- 1Department of Biological Sciences, Macquarie University, North Ryde, NSW, Australia
- 2The University of Western Australia Oceans Institute and School of Biological Sciences, University of Western Australia, Crawley, WA, Australia
- 3First Institute of Oceanography, Ministry of Natural Resources, Qingdao, China
- 4Department of Marine Sciences, University of Gothenburg, Gothenburg, Sweden
- 5Graduate School of Frontier Sciences, University of Tokyo, Kashiwa, Japan
- 6Atmosphere and Ocean Research Institute, University of Tokyo, Kashiwa, Japan
- 7Australian Institute of Marine Science, Townsville, QLD, Australia
- 8Sydney Institute of Marine Science, Sydney, NSW, Australia
- 9Ocean Tracking Network, Dalhousie University, Halifax, NS, Canada
- 10Indian Ocean Marine Research Centre, Australian Institute of Marine Science, The University of Western Australia, Crawley, WA, Australia
- 11Environmental Research Division, NOAA Southwest Fisheries Science Center, Monterey, CA, United States
- 12College of Science and Engineering, James Cook University, Townsville, QLD, Australia
- 13Institute for Antarctic and Marine Studies, University of Tasmania, Hobart, TAS, Australia
- 14Department of Ecology and Evolutionary Biology, University of California, Santa Cruz, Santa Cruz, CA, United States
- 15Department of Biology, Stanford University, Hopkins Marine Station, Pacific Grove, CA, United States
- 16Instituto de Oceanografia, Universidade Federal do Rio Grande, RioGrande, Brazil
- 17Institute for Marine and Antarctic Studies, UTAS Hobart, Hobart, TAS, Australia
- 18NOAA, U.S. IOOS Office, ATN National Coordinator, Silver Spring, MD, United States
- 19Office of Naval Research, Arlington, VA, United States
- 20Technical University of Denmark, National Institute of Aquatic Resources, Silkeborg, Denmark
- 21Fram Centre, Norwegian Polar Institute, Tromsø, Norway
- 22Institute of Marine Research, Tromsø, Norway
- 23Scottish Oceans Institute, University of St. Andrews, St. Andrews, United Kingdom
- 24ENSTA Bretagne, Lab-STICC (UMR CNRS 6285), Brest, France
- 25Sorbonne Université, UMR 7159 CNRS-IRD-MNHN, LOCEAN-IPSL, Paris, France
- 26Fish Ecology and Conservation Physiology Laboratory, Carleton University, Ottawa, ON, Canada
- 27South African Institute for Aquatic Biodiversity, Grahamstown, South Africa
- 28Department of Zoology and Entomology, Mammal Research Institute, University of Pretoria, Hatfield, South Africa
- 29Fisheries and Oceans Canada, Institut Maurice-Lamontagne, Mont-Joli, QC, Canada
- 30Red Sea Research Centre and Computational Biology Research Center, King Abdullah University of Science and Technology, Thuwal, Saudi Arabia
- 31Instituto de Física Interdisciplinar y Sistemas Complejos IFISC (CSIC-UIB), Palma de Mallorca, Spain
- 32National Institute of Water and Atmospheric Research, Wellington, New Zealand
- 33Centre d'Etudes Biologiques de Chizé UMR 7273 CNRS - Université de la Rochelle, Villiers en Bois, France
- 34School of Life and Environmental Sciences, Deakin University, Geelong, VIC, Australia
- 35School of Biological Sciences, Flinders University, Adelaide, SA, Australia
- 36Ocean Tracking Network, Department of Biology, Dalhousie University, Halifax, NS, Canada
- 37Institute of Oceanography and Environment, Universiti Malaysia Terengganu, Kuala Nerus, Malaysia
- 38Phuket Marine Biological Center, Phuket, Thailand
- 39Integrated Marine Observing System, University of Tasmania, Hobart, TAS, Australia
- 40Aeronautical Technology Directorate, Japan Aerospace Exploration Agency, Chofu, Japan
- 41Centro de Investigação em Biodiversidade e Recursos Genéticos/Research Network in Biodiversity and Evolutionary Biology, Campus Agrário de Vairão, Universidade do Porto, Porto, Portugal
- 42LOCEAN, SU/CNRS/IRD/MNHN, Paris, France
- 43Marine Biological Association of the UK, Plymouth, United Kingdom
- 44School of Ocean and Earth Science, University of Southampton, Southampton, United Kingdom
- 45Norwegian Institute for Nature Research, Trondheim, Norway
- 46Department of Oceanography and Marine Research Institute (Ma-Re), University of Cape Town, Cape Town, South Africa
- 47Marine Mammal Research Unit, Institute for the Oceans and Fisheries, University of British Columbia, Vancouver, BC, Canada
- 48Institute for Marine and Antarctic Studies, University of Tasmania, UTAS, Hobart, TAS, Australia
Animal telemetry is a powerful tool for observing marine animals and the physical environments that they inhabit, from coastal and continental shelf ecosystems to polar seas and open oceans. Satellite-linked biologgers and networks of acoustic receivers allow animals to be reliably monitored over scales of tens of meters to thousands of kilometers, giving insight into their habitat use, home range size, the phenology of migratory patterns and the biotic and abiotic factors that drive their distributions. Furthermore, physical environmental variables can be collected using animals as autonomous sampling platforms, increasing spatial and temporal coverage of global oceanographic observation systems. The use of animal telemetry, therefore, has the capacity to provide measures from a suite of essential ocean variables (EOVs) for improved monitoring of Earth's oceans. Here we outline the design features of animal telemetry systems, describe current applications and their benefits and challenges, and discuss future directions. We describe new analytical techniques that improve our ability to not only quantify animal movements but to also provide a powerful framework for comparative studies across taxa. We discuss the application of animal telemetry and its capacity to collect biotic and abiotic data, how the data collected can be incorporated into ocean observing systems, and the role these data can play in improved ocean management.
Background
Animal telemetry is a powerful tool for observing marine animals and their environments (Bograd et al., 2010; Costa et al., 2010a,b, 2012; Hussey et al., 2015). Animal telemetry can provide important ecological insights into animals' habitat preferences and home range sizes (Aarts et al., 2008; Block et al., 2011; Raymond et al., 2015), behavior states (Jonsen et al., 2005), physiology (Metcalfe et al., 2012), the timing of long-term movements and migrations (McConnell and Fedak, 1996; Hays et al., 2006; Shaffer et al., 2006; Aarestrup et al., 2009; Cherry et al., 2013; Whitlock et al., 2015), and the biotic and abiotic factors that shape their current and potential abundances and distributions (Laidre et al., 2008; Hawkes et al., 2009; Costa et al., 2010b; Hazen et al., 2013a,b; Hindell et al., 2016). These ecological parameters can be monitored to provide insight into changes in the underlying state of the oceans and their ecosystems (Hazen et al., 2019).
In addition to these ecological insights, there has been growing acceptance by physical oceanographers that animal-borne sensors can provide useful abiotic data from regions otherwise difficult to sample (Boehlert et al., 2001; Charrassin et al., 2008; Fedak, 2013; Ohshima et al., 2013). For example, assimilating animal telemetry data into ocean circulation models has resulted in significant improvements in representations of major ocean systems (Roquet et al., 2013; Treasure et al., 2017). While animal-borne sensors are limited to where animals go, and therefore, do not provide the same spatial and temporal coverage as artificial observing systems, the broad scope and immense data collection capabilities [e.g., multiple Conductivity Temperature Depth (CTD) casts per day] provide a uniquely valuable opportunity for collaboration among biologists and earth scientists to promote improved observation of ocean systems (Fedak, 2004).
Many streams of animal-borne telemetry data are now routinely included in online data portals that are available to researchers in a broad range of scientific fields. We contend that these data streams provide reliable observations of Essential Ocean Variables (EOVs). For example, the Global Ocean Observing system (GOOS) is interested in surface and sub-surface temperature and salinity profiles provided by animal-borne sensors, as these variables are crucial for the detection and attribution of changes in the marine environment that are relevant on a global scale.
At the OceanObs Conference in 2009, the potential of animal borne sensors for ocean observation was well received, including their role in collecting observations of EOVs (Costa et al., 2010a). In the decade since this meeting, the application of animal borne sensors has further matured, and the use of animal telemetry continues to grow exponentially (Hussey et al., 2015). This has been made possible by advances in tag technology, including miniaturization, improvements in battery efficiency and memory capability, and large reductions in unit costs. These advances are being complemented by an expanding range of sophisticated sensors that provide unprecedented insight into the lives of marine animals and their environments, from coastal and continental shelf ecosystems through to polar seas and open oceans (Guinet et al., 2013; Kays et al., 2015; Lennox et al., 2017). In this paper, we (1) review the status of animal telemetry systems in the context of global ocean observing, (2) describe current and new applications of this technology for marine ecological studies and collection of ocean observations including EOVs, and (3) review new approaches to analyzing these increasingly large and complex data sets, and comment on future directions.
An Overview of Animal Telemetry Systems
There are several animal telemetry technologies that are used to collect data in the marine environment (Figure 1). Some systems are archival, collecting streams of data (e.g., an animal's location, physiological and behavioral states, and environmental conditions) and storing them on the tag for later recovery of the device. Other systems are able to relay data via radio (e.g., satellite) or acoustic signals.
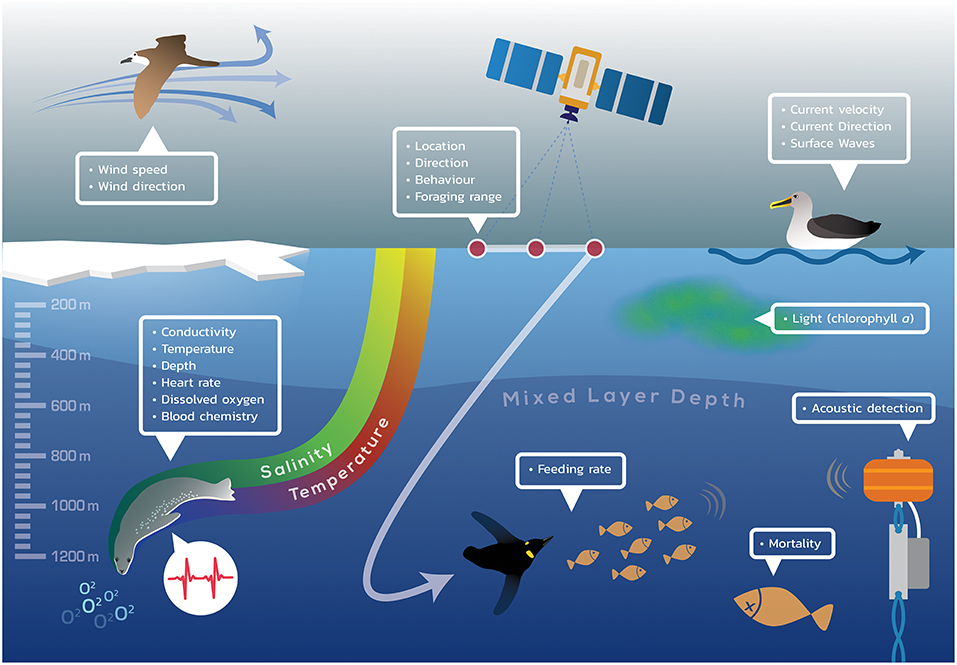
Figure 1. Schematic illustrating the many parameters that can be measured by animal-borne packages, using archival, acoustic, or satellite telemetry. The environmental, physiological, and ecological data collected by the illustrative marine animals (penguin, seabird, fish, seal) may be measured in multiple ways and stored or transmitted or both.
Archival tags are generally used for animals that are able to be easily recaptured. These include central-place foragers, such as seabirds and pinnipeds, that return to a known location either between foraging trips or after longer migrations. Body size and attachment technique may also necessitate the use of archival tags, which are often smaller than relay tags due to lower power requirements. In cases where tags are less likely to be recovered or where near real-time information is desired, data may still be archived on the tag, but are also relayed periodically through data transfer systems. For air-breathing species, such as marine mammals, seabirds and turtles, radio or satellite tags attempt to relay data each time the animal surfaces, via an antenna that must be exposed to the air. For non-air-breathing species, tags can be fitted on a dorsal fin or attached with tethers, so that the antenna occasionally emerges from the water when the animal swims near the surface. For animals that never surface, a pop-up archival tag can be deployed, which logs information for extended periods before “popping off” when a triggering mechanism releases the tag from the animal. The tag then floats to the surface where it can transmit data to a satellite (Block et al., 1998; Block, 2005). Depending on the size of the package, archival tags range from simple coarse resolution location data (Global Location Sensing-GLS) to high resolution (Global Positioning System-GPS) location data with multiple ancillary sensors (e.g., depth, temperature, conductivity, dissolved oxygen, accelerometry, see Figure 1).
Satellite-relay systems have limited bandwidth and are, therefore, often unable to transmit all of the large volumes of data that sophisticated, multiple sensor tags collect and archive. This constraint is a function of tag energy requirements, the amount of time an animal spends at the surface, i.e., access to a satellite, and satellite availability. Therefore, these tags often transmit either a pre-programmed data summary (e.g., average values over preselected time periods), or a random subsample of detailed data (Fedak et al., 2002; Block et al., 2011), whenever their signals reach a satellite. Consequently, there is a significant benefit in recovering tags where possible, to access the full stream of high-resolution archived data.
Acoustic telemetry is widely used for small marine animals that do not surface to breathe and are unlikely to be reliably recaptured (Donaldson et al., 2014). Typically, acoustic transmitters are small, light, implantable and cost-effective, facilitating large sample sizes. However, acoustic telemetry is limited by the fact that data transmission occurs through the water via acoustic data packages that can only be detected within the range of a receiver (rarely > 800 m). In most cases, receivers must also be physically recovered from the ocean to download the archived data, limiting real time data acquisition. However, units that transmit data from receivers over mobile phone networks, or via satellite telemetry, are becoming increasingly common, paving the way for the development of novel observation platforms especially in near-shore regions. Mobile acoustic receivers mounted on gliders (Lennox et al., 2018) or attached to free-swimming animals (Lidgard et al., 2014) are also now starting to be deployed, increasing the spatial coverage of the acoustic network. Emerging coordination among research organizations with compatible receivers enables acoustic telemetry data to be linked across scales of tens of meters to thousands of kilometers (Heupel et al., 2006; Brodie et al., 2018; Griffin et al., 2018).
Analysis of Animal Telemetry Data for Movement Ecology
A variety of methods have been used to analyse different types of animal telemetry data. For example, for satellite telemetry and light-level geolocation, state-space models (Patterson et al., 2008; Schick et al., 2008; Jonsen et al., 2013; McClintock et al., 2014), including hidden Markov models (e.g., Langrock et al., 2012), have been widely used to infer animal movements and to quality-control locations from error-prone telemetry data (Jonsen et al., 2005; Johnson et al., 2008). Such models allow inference of unobservable states or behaviors (e.g., horizontal location, foraging bouts) from time-series observations. Simultaneously, these models separate variability arising from an animal changing speed and direction as it moves through different habitats, from artificial noise introduced by the observation process (e.g., through the distortion or disruption of animal-borne tag transmissions to an orbiting satellite). State-space models have been used to advance knowledge based on large telemetry datasets (Block et al., 2011; Strøm et al., 2018), to infer unobservable behaviors (Leos-Barajas et al., 2017; Michelot et al., 2017), and to understand how movement behaviors are influenced by environmental drivers (Patterson et al., 2009; McClintock et al., 2012; Bestley et al., 2013; Jonsen et al., 2018).
Movement behaviors of mobile marine animals, such as foraging, resting, or fleeing, can be embedded in a complex web of movement types (straight line, looping, convoluted paths). The random walk paradigm, a standard framework for modeling individual animal movement, has also been commonly used to describe these movement patterns and to infer behaviors from simple null models that relate to theories about searching, foraging or dispersal (Nathan et al., 2008). Normally diffusing random walks, such as a correlated random walk (CRW) (Turchin, 1998), can be used to analyze navigational capacities (Bailey et al., 2018) and movements in heterogeneous landscapes (Barton et al., 2009), to test habitat selection (Sims et al., 2006), to infer behavioral states from movement paths (Jonsen et al., 2005) and as a means to aid reconstruction of marine animal paths from telemetry data (Johnson et al., 2008).
Resource Selection Functions (RSF) in random-walk models, which have been widely used to investigate species' habitat preferences in terrestrial studies, are now an emerging approach in marine ecology (Manly et al., 2002; Sousa et al., 2016; Lone et al., 2018). The objective of RSFs is to quantify the disproportionate use of a resource (or habitat) relative to its availability, often estimated by mechanistic movement models (Bastille-Rousseau et al., 2015; Queiroz et al., 2016). Improvements have also been made to RSFs, such as the addition of step- and path-selection and the estimation of movement covariates within step selection analysis, thus accounting for changes in resource availability during animal movement (Avgar et al., 2016).
Specialized random walks, including Lévy walks (Sims et al., 2008), have been used to explore commonalities in movement and potential optimality of search patterns by individuals and species across different environments (Humphries et al., 2010). A strength of quantitative random walk analysis is the opportunity it presents for testing explicit hypotheses linked to elucidating the factors driving the expression and evolution of behavior (Hays et al., 2016).
Studies using acoustic telemetry have historically been designed to generate data over limited spatial scales to address a specific research question. These studies have addressed measures of residency, movements and activity space (Heupel et al., 2006). Analyzing data over small spatial scales limits the capacity of researchers to make comparisons of patterns among study sites and to link data from the same individuals that may move among telemetry arrays. The development of broader acoustic telemetry networks and increased data sharing has resulted in vast data sets that can provide broader information on species movement (e.g., Hoenner et al., 2018). These large data sets provide challenges in data management and analysis, especially when considering that these data are typically used to calculate movement metrics, such as home range size. Standardized approaches that calculate metrics of detection, dispersal and activity space allow direct comparisons among sites Udyawer et al. (2018). Integration of multiple data streams, such as environmental variables, to help interpret movements and space-use, also enhance the value of telemetry data but present new analytical and data management challenges (see below). Finally, technological advances, including sensors integrated into transmitters, provide another layer of data complexity, while concomitantly providing an opportunity to develop a refined sense of movement in three dimensions (Simpfendorfer et al., 2012; Udyawer et al., 2015; Lee et al., 2017).
Animal Telemetry and Oceanography
Animal telemetry has contributed data on key physical environmental variables, including ocean temperature and salinity, by using oceanographic sensors integrated into animal-borne satellite transmitters. Tracked marine animals are gathering key surface and sub-surface oceanographic information in some of the harshest environments on the planet, filling important observational gaps in the global climate observing system (Fedak, 2013; Roquet et al., 2013, 2017), while simultaneously linking the behavior of these animals to these oceanographic parameters (Biuw et al., 2007). Vertical profiles of temperature and salinity, the two key observations for calculating water density are now routinely sampled in several key areas of the global ocean, such as the seasonally ice-covered sectors of the Southern Ocean (Charrassin et al., 2008). These data are central to understanding global climate processes given this region's central role in heat and CO2 uptake and unhindered (land barrier free) distribution of climate signals (Sallée, 2018). New sensors also provide expanding opportunities to monitor additional parameters, such as chlorophyll (Guinet et al., 2013) or the wind/wave surface state from accelerometry and magnetometry or hydrophony (Cazau et al., 2017). The current state of development and some of the limitations of integrating animal telemetry with oceanography are reviewed below, with specific focus on advances in technology and scientific findings.
Autonomous Oceanography With Animal-Borne Sensors
Physical oceanography has traditionally focused on the observation of the two key properties of seawater: temperature and salinity. These parameters are related to the ocean heat budget, a central element of the climate system, as well as to mechanisms of evaporation, precipitation, and sea ice formation and melting. When observed simultaneously, these two properties determine seawater density from which the geostrophic component of ocean circulation, the vertical stratification of water masses and mixing patterns can be derived. For this reason, temperature and salinity are most often profiled together using a single instrument, the CTD (Conductivity-Temperature-Depth), that combines a pressure sensor with a temperature probe and a conductivity cell from which salinity and density can be derived. Traditionally, these instruments have been deployed from research vessels.
The advent of the global Argo array of autonomous profiling floats in the early 2000s profoundly modified ocean monitoring. The Argo system provides a synoptic sampling of the upper 2000 m of the ocean in space and time that is near global albeit at a coarse scale (Roemmich et al., 2009; Riser et al., 2016). Concurrently, in 2000/01, the Sea Mammal Research Unit (SMRU, University of St. Andrews, UK) developed the CTD Satellite Relay Data Logger (CTD-SRDLs Figure 2; Lydersen et al., 2002). The CTD-SRDL is an autonomous logger incorporating a miniaturized CTD unit (Boehme et al., 2009), coupled with a satellite transmitter (Argos) that enables geo-location and data transmission. Calibration of the sensors in a labeled oceanographic calibration facility is undertaken prior to every deployment to ensure high data quality [for example following battery replacement (Goetz, 2015)]. The CTD-SRDL is most often programmed to sample water properties at 0.5 Hz during the ascent phase of an animal's dive and these CTD-profiles are then telemetered in a compressed form (binned in from 10 to 25 depth levels per profile depending on the configuration) using the ARGOS location and data collection system (Photopoulou et al., 2015), offering a life-span of about 6 to 8 months of data collection/transmission, depending on the species tagged and the scheduling applied.
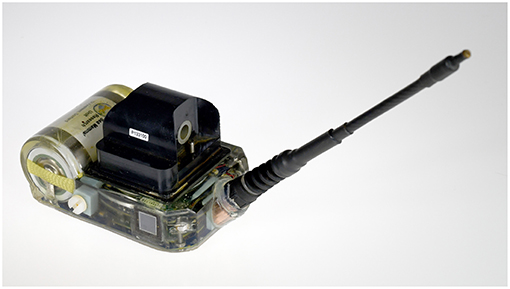
Figure 2. A CTD-SRDL tag, featuring a miniaturized CTD on top of the core unit, with the microcontroller below, the wet/dry sensor on the frontside, the battery on the rear, and the Argos antenna pointing forward.
Although there have been important modifications in the design and programming of CTD-SRDLs since their first use, the design of the CTD instruments incorporated in these tags has remained quite stable. This stability has ensured accumulation of data and improved knowledge about each of the sensors' performance, based on both laboratory calibration experiments (Boehme et al., 2009) and comparisons with ship-borne CTD profiles (Roquet et al., 2011; Frazer et al., 2018; Mensah et al., 2018). More recently, continuous recording by CTD-SRDL sensors has enabled direct comparisons between consecutive upcasts and downcasts, thereby providing new ways to assess the dynamic response of sensors (Mensah et al., 2018).
The SMRU CTD-SRDL and other loggers archive data to internal memory far more frequently than they can be transmitted and, if the instruments can be recovered, additional data at higher resolution can be downloaded. To date, about 50 finely-resolved multi-parameter tracks have been collected from polar seals [Weddell (Leptonychotes weddellii), crabeater (Lobodon carcinophagus) and southern elephant seals (Mirounga leonina)], and temperate pinnipeds [Australian (Neophoca cinerea) and California (Zalophus californianus) sea lions, Australian (Arctocephalus pusillus doriferus) and New Zealand fur seals (A. forsteri)]. In some rare instances, CTD-SRDLs have been configured to continuously log during the entire deployment period on southern elephant seals from the Kerguelen Islands. Results from a dozen such loggers offered a kilometric spatial resolution to investigate sub-mesoscale variability, as well as new opportunities to investigate CTD data quality (Mensah et al., 2018).
Oceanographic Findings Enabled by Animal Telemetry Over the Last Decade
The functionality of Argo floats is limited in polar regions due to the seasonal presence of sea ice that prevents floats from returning to the surface. Instrumenting free-ranging, air-breathing animals that move through sea-ice covered areas, such as seals, with temperature and salinity sensors can help fill data gaps in the Argo dataset in sea ice regions (Fedak, 2013; Treasure et al., 2017). To date, over 540 000 profiles have been collected by marine mammals and have been made available to the broader operational and research oceanography communities (Roquet et al., 2014) (Figure 3, http://www.meop.net/).
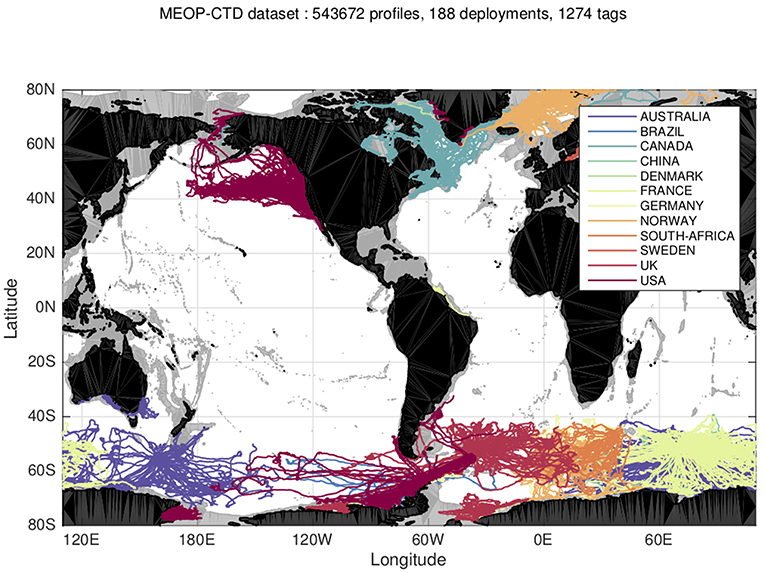
Figure 3. Distribution of hydrographic profiles currently available in the MEOP-CTD database (November 2017 version, Source: meop.net).
The great potential provided by animal-borne sensors for monitoring Southern Ocean hydrographic conditions and how animals respond to them was demonstrated following the deployments of CTD-SRDLs on southern elephant seals in 2004–2005 (Biuw et al., 2007; Charrassin et al., 2008). These early data helped refine our knowledge of the Antarctic Circumpolar Current (ACC) frontal structure and hydrography in the South Atlantic (Boehme et al., 2008; Meredith et al., 2011) and in the vicinity of the Kerguelen Plateau (Park et al., 2008; Roquet et al., 2009) with applications for tracking ACC fronts (Pauthenet et al., 2018) or for estimating rates of sea ice formation (Charrassin et al., 2008; Williams et al., 2011). In 2011, observations from animal-borne CTD-SRDLs were central to solving a 30+ year-old puzzle regarding Antarctic Bottom Water formation in the Weddell-Enderby Basin (Ohshima et al., 2013). Observations of very high salinity shelf water were linked to a new source of Antarctic Bottom Water in the intense Cape Darnley polynya. Furthermore, Williams et al. (2016) demonstrated that Prydz Bay, situated just east of Cape Darnley, makes a secondary contribution to Antarctic Bottom Water due to the production of dense shelf water near the Amery Ice Shelf (also see Xu et al., 2017). A minor source of Antarctic Bottom Water was also detected at Vincennes Bay (Kitade et al., 2014), supporting the idea that several East Antarctica polynyas contribute to Antarctic Bottom Water formation. However, the ongoing freshening by glacial melting may compromise the ability of polynyas to form this Bottom Water in the future (Williams et al., 2016; Silvano et al., 2018).
Animal-collected data have also been very successful in documenting local circulation and seasonal variability of water properties over the Antarctic continental shelf. Costa et al. (2008) analyzed the upper ocean heat content variability in the west Antarctic Peninsula using instrumented seals, providing a valuable reference to evaluate numerical circulation models. Using the maximum depth of benthic dives, Padman et al. (2010) identified troughs in the continental shelf that allow intrusions of Circumpolar Deep Water under the Wilkins Ice Shelf, accelerating its collapse (Padman et al., 2012). Animal-collected data also helped to characterize the exchange of properties across the shelf break in the Weddell Sea, linked to eddy overturning (Nost et al., 2011) and wind forcing variability (Arthun et al., 2012). Zhang et al. (2016) described intrusions of modified Circumpolar Deep Water into the continental shelf waters of the Bellingshausen Sea, with important implications for the stability of the West Antarctic Ice Sheet. Mallett et al. (2018) presented new insights on the distribution and seasonality of Circumpolar Deep-Water properties in the Amundsen Sea. More broadly, the animal collected data is limited seasonally and spatially, but by merging animal-collected data with ship-based and Argo float observations, Pellichero et al. (2017) provided the most comprehensive assessment of the seasonal cycle of Southern Ocean mixed-layer characteristics to date.
Animal CTD-SRDLs have also become a valuable data source in several sectors of the North Atlantic Ocean. Straneo et al. (2010) used hooded seal (Cystophora cristata) data from the East Greenland Shelf to estimate seasonal temperature variations of subtropical waters sitting near the entrance of a major glacial fjord. Variability of these continental shelf waters was further investigated by Sutherland et al. (2013). Instrumented ringed seals (Pusa hispida) have also proved useful to investigate freshwater runoff from the Greenland Ice Sheet (Mernild et al., 2015) and freshwater discharge plumes from glaciers in Greenland (Everett et al., 2018) by providing observations from directly adjacent to the glacier tongue. Grist et al. (2011) used Argo and marine mammal profiles to produce a gridded data set that revealed distinctive boundary current-related temperature minima in the Labrador Sea and at the East Greenland coast (Isachsen et al., 2014). Isachsen et al. (2014) used data collected by instrumented hooded seals as well as Argo floats to reveal warmer and saltier conditions over much of the Nordic Seas in 2007–2008 compared to the 1956–2006 climatology. Exchanges of warm Atlantic Water across the shelf west of Spitsbergen were found to be primarily controlled by surface heat flux through the generation of an eddy overturning (Tverberg et al., 2014).
Autonomous Sensing of the Air-Sea Interface With Seabirds
The air-sea interface couples the ocean and atmosphere through exchanges of momentum, heat, gas, water, and micro-particles, thus it plays an important role in determining daily weather conditions and also in driving global climate change and biogeochemical cycles. Wind and current observations are necessary to understand the processes mediated at this interface. Although there have been remarkable advances in technology to observe the air-sea interface such as satellites, drifters, and autonomous surface vehicles, measurements have been so coarse spatially or temporally that the air-sea fluxes often remain uncertain, decreasing the accuracy of forecasting unusual weather events. Physical observing approaches that sample at fine spatio-temporal resolutions are required to improve the accuracy of atmosphere and ocean nowcasts and forecasts.
The development of a small motion logger that can be attached to a bird's back and contains a micro-controller, a GPS receiver, an inertial sensor, a 3-axis geomagnetic sensor, and a Li-Ion battery charger (Yoda et al., 2014; Yonehara et al., 2016) has revealed that observations of soaring seabirds, such as the streaked shearwater (Calonectris leucomelas) and albatross, can measure winds over the sea (Yonehara et al., 2016; Goto et al., 2017; Figure 4), surface currents (Yoda et al., 2014), and surface waves. Winds are particularly under-sampled in open ocean areas due to infrequent (twice/day) satellite observations and sparse buoy measurements, and in coastal zones where the topography is complex (Pickett et al., 2003; He et al., 2004; Albert et al., 2010). Extensive travel distance and prolonged flight duration of soaring seabirds enabled fine-scale resolution and wide geographic range estimation of wind speed and direction covering temporal and spatial gaps between the remote-sensing measurements.
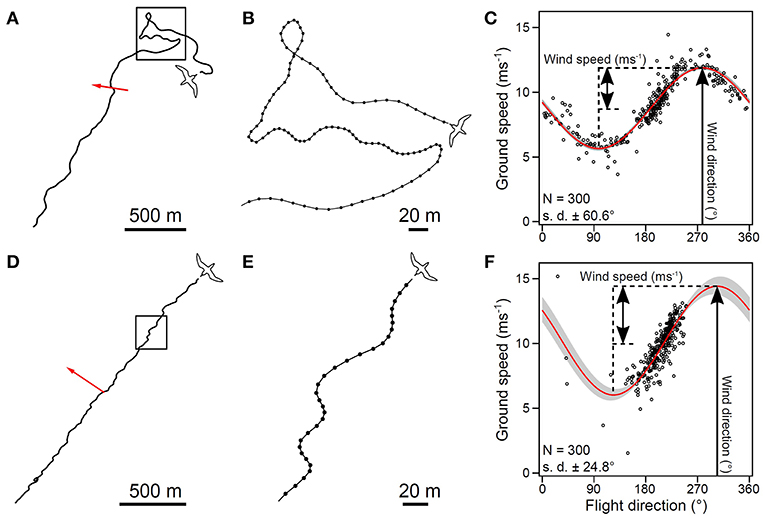
Figure 4. (A) An example of a 5-min section of the flight path of a streaked shearwater. The red arrow indicates the estimated wind velocity. (B) Enlarged view of a meandering path shown in (A). (D) Another example of a 5-min section of a flight path of a streaked shearwater when the bird seemed to travel in a certain direction. (E) Enlarged view of (D) showing repeated zigzag movement from a soaring maneuver. (C,F) The relationship between flight direction and ground speed of the path section in (A) [estimated wind speed of fitted curve, 3.11 m s−1; upper confidence interval (CI), 3.13 m s−1; and lower CI, 3.10 m s s−1; estimated wind direction of fitted curve, 278°; upper CI, 280°; and lower CI, 277°] and D (estimated wind speed of fitted curve, 4.20 s−1; upper CI, 4.34 s−1; and lower CI, 4.10 s−1; estimated wind direction of fitted curve, 304°; upper CI, 310°; and lower CI, 298°), respectively. Angular SDs of the flight direction are (C) 60.6° and (F) 24.8°, respectively. The red curve is the fitted sinusoidal curve. Gray area represents the 95% CI of the fitted sinusoidal curve. Estimated wind speed and direction is indicated by black arrows. The figures adapted from data presented in Yonehara et al. (2016).
Surface currents consist of geostrophic and ageostrophic currents, such as Ekman and Stokes drifts, which play important roles in transporting heat, organic matter, and inorganic matter, and therefore strongly influence marine ecosystems. Current measurements are typically conducted by in situ observations using either ship-board acoustic Doppler current profilers (ADCP), moored current-meters, Lagrangian drifters, or remote-sensing, such as satellite altimetry and High Frequency (HF) radar. However, these in situ methods have spatial or temporal limitations and HF radar observation in particular is limited to coastal regions. Satellite altimetry offers wider spatial scope for observations and can observe global surface geostrophic currents, but has disadvantages including spatial and temporal resolutions of 7 km and 10 days, respectively. Accuracy of altimetry decreases in the coastal regions due to tidal effects and importantly, satellite altimetry cannot measure ageostrophic currents.
Yoda et al. (2014) developed a new method to measure in situ currents by exploiting the behavior of seabirds equipped with GPS loggers. This method estimated surface current velocity from GPS track data collected by streaked shearwaters when they drifted passively at the sea surface (Figure 5). The GPS logger consisted of a GPS receiver with an antenna (GiPSy, Technosmart, Rome, Italy) that was programmed to record a position every 1 min. Measurements of wind speed and direction using this method were similar to those observed by ship-borne ADCPs, although further quality control is needed to improve estimates of current velocity because birds are susceptible to slip at the surface depending upon wind conditions (Fossette et al., 2012; Yoda et al., 2014; Sánchez-Román et al., 2019). Miyazawa et al. (2015) showed the feasibility of assimilating high-resolution surface current data (Figure 6), obtained by streaked shearwaters with GPS loggers (Yoda et al., 2014) into an operational ocean forecast system during the Japan Coastal Ocean Predictability Experiment 2 (JCOPE27; see jamstec.go.jp/jcope/for real-time forecast). Furthermore, seabirds are not only passive drifters at the sea surface, they also adaptively search for prey in different prey fields (Yoda et al., 2014; Carroll et al., 2017). This feeding behavior improves the ability to monitor surface currents intensively in highly productive regions where seabirds commonly feed.
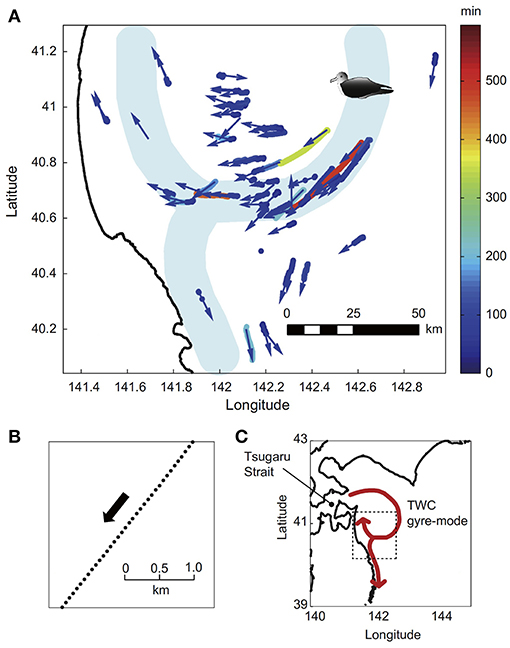
Figure 5. (A) Passive drift movements of streaked shearwaters tracked in September 2010. The color bar indicates duration of drifting on seawater. Most drift tracks were shorter than 100 min, but some lasted several hours (pale blue to red tracks). The blue arrows indicate directions of drifting. (B) One example of drifting of a streaked shearwater. The black arrow indicates drift direction. We defined drifting as resting on water for more than 30 min with smoothness and consistency of movement direction. (C) The box in (C) is enlarged in (A). The bold red line in (C) and the pale blue region in (A) are the Tsugaru Warm Current (TWC) on 10 September 2010 derived from ship-board ADCPs reported by the Marine Information Service Office, Japan Coast Guard (JCG) (URL: http://www1.kaiho.mlit.go.jp/). Reprinted from Progress in Oceanography, 122, Ken Yoda, Kozue Shiomi, Katsufumi Sato, Foraging spots of streaked shearwaters in relation to ocean surface currents as identified using their drift movements, 54-64, Copyright (2014), with permission from Elsevier.
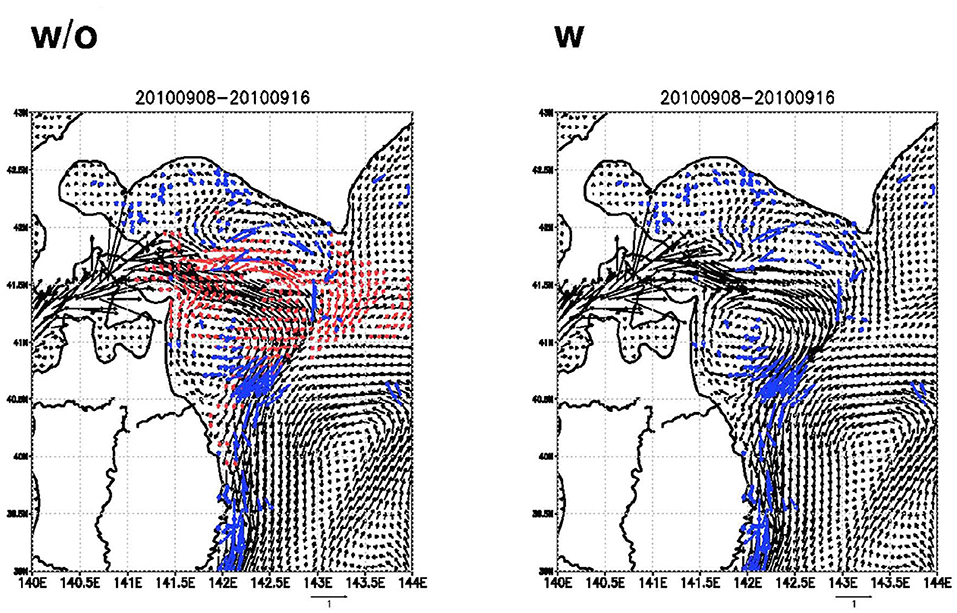
Figure 6. Model current distributions at 5 m depth averaged during the target period. Left (right) panels indicate the results without (with) the assimilation of the seabird drift in the 2010 season. The composite drift data are represented by the blue colored vectors. Difference larger than 0.1 m s−1 in magnitude of the flows with and without the assimilation is indicated by the red colored vectors in the left panels. Numerics at the top of the figure denote the period. The figures adapted from data presented in Miyazawa et al. (2015) which is licensed under a Creative Commons Attribution 4.0 International License.
Animal Telemetry and Essential Ocean Variables
Essential Ocean Variables (EOVs) are a suite of parameters that have been identified by the United Nation's Intergovernmental Oceanographic Commission's Global Ocean Observing system (GOOS) BioEco and Physics and Climate panels (Miloslavich et al., 2018). These parameters are considered crucial for the detection and attribution of change in the marine environment relevant to the global scale. Historically, GOOS has coordinated the collection of data on physical and chemical oceanographic indicators (i.e., the physical environment), and is now working to expand the monitoring to cover ecosystem and biological (EcoBio) indicators that track variation and trends in key biota and ecosystem processes (Miloslavich et al., 2018).
In addition to providing biological information about tagged individuals, animal telemetry data can provide robust, reliable, and comparable information on EcoBio EOVs. Although EcoBio EOVs are currently in development, a number of candidate variables have been proposed that relate to animal diet, phenology, and abundance (Constable et al., 2016). Foraging range is an important candidate EcoBio EOV, that is often derived from animal telemetry and can be informative about the distribution of prey and its effect on marine predators. For example, the Marine Mammals Exploring Oceans Pole-to-Pole (MEOP) program is likely to be an important source of EcoBio EOV data in the Southern Ocean (Roquet et al., 2017; Treasure et al., 2017). Parameters, such as foraging ranges, trip durations and habitat use obtained by tracking elephant seals (Mirounga sp), humpback whales (Megaptera novaeangliae), and king (Aptenodytes patagonicus), emperor (Aptenodytes forsteri), Adélie (Pygoscelis adeliae) and Gentoo (Pygoscelis papua) penguins, have been identified as a cost effective way to monitor the distribution of mesopelagic fish and krill in the ecosystem (Constable et al., 2016; Xavier et al., 2018). Because species have different habitat and dietary requirements, the species to be monitored as indicators of global ocean health need to be carefully considered.
The use of animal telemetry data for EOVs is facilitated by standardization across regional networks such as the Animal Telemetry Network (ATN, USA), Ocean Tracking Network (OTN, Canada and Global), European Telemetry Network (ETN), Acoustic Tracking Array Platform (ATAP, South Africa), and Integrated Marine Observation System Animal Tracking Facility (IMOS ATF; Australia). The adoption of common and coordinated analytical metrics (e.g., IODE OBIS; Benson et al., 2018; Table 1) by these regional facilities promotes the application of EOVs to global problems.
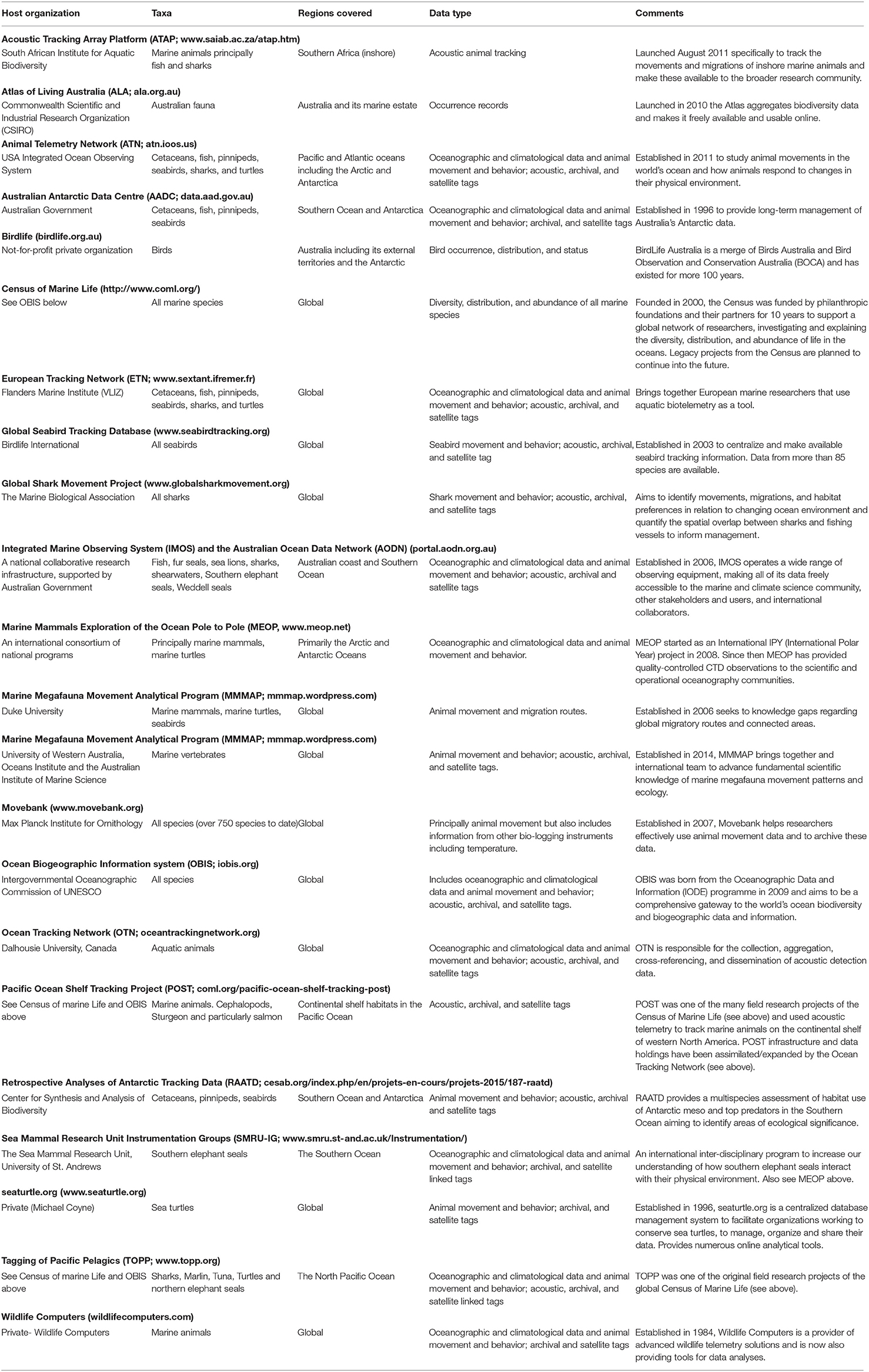
Table 1. Summary of marine data repositories for animal-borne oceanographic sensors and observation of animal movement.
To ensure that all EOVs are relevant to Ocean Observing, GOOS has highlighted four metrics against which EOVs, including those derived from animal telemetry data, must be assessed:
• Implementation metrics deal with the feasibility of the approaches used to measure the EOVs and the reliability of the technology used. Land-breeding species (such as seabirds and most pinnipeds) are relatively easy to access and to instrument, ensuring regular opportunities for deployment of tracking equipment. The cost of instrumentation has declined substantially over the last decade, its reliability is high and continues to improve, analysis approaches are becoming fast and efficient, and it is now possible to instrument large samples of animals with GPS and light-level geolocation devices (Auger-Méthé et al., 2017). It is increasingly recognized that deployment of tags that are too large or too heavy can impede animal performance, compromising both the individual animal's welfare and the quality of the data that is collected (Vandenabeele et al., 2012; McIntyre, 2014). Careful selection of tags to minimize the effects on both individuals and populations prior to large scale deployments is critical. Given these caveats, though, depending on device type, tags can provide data for periods of days, weeks (high spatial resolution GPS loggers) up to a period of years currently topping out at a decade (low resolution geolocation devices, implanted acoustic tags). This means that the nature of the monitoring required will influence the choice of instrumentation.
• Performance metrics quantify how the observations satisfactorily represent the phenomena of interest. For example, animal tracking can address the proposed foraging range EOV, and is often the only viable tool for addressing this question, particularly during the non-breeding winter period when ship-based and aerial surveys are impractical in remote areas such as polar regions.
• Data delivery metrics quantify how efficiently and adequately the data from the tags are transferred to users. Some types of tracking data are available in near-real time. For example, the Argos system, when it is in contact with a tag, calculates the position of animals and provides this information to the user within 24 h. Several user groups automatically upload Argos positions for use by the broader community (e.g., IMOS). Tracking devices that rely on archived data, such as geolocation tags and some types of GPS tags, cannot deliver data until the device has been retrieved or has come within range of a base-station and the data have been downloaded. In the case of light-level geolocation, there is also a need to process the downloaded data in order to obtain position estimates. This necessarily places limits on the temporal utility of these technologies where there may be a lag of several years from deployment until the data becomes available.
• Impact metrics quantify use of data, information, and products for societal benefit. Examples include the number of peer-reviewed publications and research projects. The animal telemetry community has a well-established culture of publishing its work. Since the 1980s, there has been a steady increase in the number of research projects and publications from the use of marine animal telemetry (Hussey et al., 2015). In recent years there have also been increasing numbers of collaborative syntheses analyzing the many diverse data sets compiled by hundreds of individual tracking studies. These syntheses provide genuinely synergetic insights into ecological processes, such as animal foraging ranges and the factors that underpin them (Block et al., 2011; Brodie et al., 2018; Sequeira et al., 2018).
Benefits and Challenges of Animal Telemetry as a Tool For Global Ocean Observation
Benefits for Local Conservation
Animal telemetry has immense value for aiding understanding and conservation of ocean habitats (Hays et al., 2019). At the same time as measuring animal movement, tracking devices can collect important observations on poorly monitored physical environments through which these animals' transit. The complex and diverse marine systems in tropical regions support a rich diversity of life including tens of marine mammal and sea turtle species, many of which are endangered. Compared to the relatively abundant data available in many other regions (e.g., Hussey et al., 2015; Sequeira et al., 2018), information about ocean use by marine fauna in tropical Asia is limited. To address these data and knowledge gaps, researchers have started data collection using available technologies. We present two examples of relatively nascent studies from tropical Asia, spanning the Pacific, and Indian Oceans to illustrate the recent application of these ideas.
In tropical Asia the green turtle (Chelonia mydas) is listed as Endangered (Seminoff, 2004) and the Bryde's whale (Balaenoptera edeni) listed as Least Concern (Cooke and Brownell, 2018) in the IUCN Red List of Threatened Species. Tropical Asia hosts more than a third of the 32 known important nesting areas (Index Sites) of green turtles, and more than half of the subpopulations nesting at these sites have been declining in the region (Seminoff, 2004). Although Bryde's whales are distributed throughout tropical Asian waters, limited population data are available and only for the upper Gulf of Thailand (Cherdsukjai et al., 2015). Satellite tracking of turtle and whale movement in the region has described space use and migration patterns, as well as having documented the need for a regional integrated effort for conservation of these megafauna. Satellite tagged green turtles at Redang Island (Liew et al., 1995), Khram Island (Chantrapornsyl et al., 2002), and Ma'Daerah Sanctuary (Van de Merwe et al., 2009), all within registered Index Sites, migrated after nesting, traveling back to their forage grounds up to 2,900 km away and spanning coastal waters of five countries. The tracked turtle routes show that the nesting population spent considerable time outside existing protected areas (e.g., no trawl zones), and hence are likely exposed to bycatch from fishing activities (Van de Merwe et al., 2009). This supports findings elsewhere, for example, recent analyses from the Mediterranean using large sample sizes showed that conservation planning that did not include turtle tracks would perform poorly, missing important habitats (Mazor et al., 2016). Similarly, satellite tracking indicated that Bryde's whales' movements in the Upper Gulf of Thailand can cover the entire Gulf (bordered by three countries) in a single week (Cherdsukjai et al., 2016). This exemplifies the need for immediate coordinated efforts in conservation planning and actions for protection of these species in this region.
The Importance of Collaboration and Data Sharing
Due to the cost and challenges associated with deploying tags and recovering data, benefits arising from the increasingly large volume of telemetry data being collected worldwide can be maximized if data are pooled together and can be made accessible to a broad array of researchers. Initiatives focusing on data pooling and collaborative, multidisciplinary research have created tools to greatly increase our understanding of animal movement and behavior and have led to scientific breakthroughs in the discipline of movement ecology (Hussey et al., 2015). Such breakthroughs have been made by collaborative initiatives, such as the Tagging of Pelagic Predators (TOPP; https://oceanview.pfeg.noaa.gov/topp/map), the aforementioned MEOP and IMOS ATF and the Marine Megafauna Movement Analytical Program (MMMAP; mmmap.wordpress.com), among others (Table 1). For example, TOPP analyzed data from 1791 individual tracks collated as part of the Census of Marine Life programme and provided a quantitative assessment of the space use, hotspots, migration pathways, and niche partitioning by multiple predator species (fish, pinnipeds, cetaceans, turtles, seabirds) in the Pacific Ocean (Costa et al., 2010b; Block et al., 2011). Data collated by IMOS-ATF led to the identification of four functional movement classes of marine animals within Australian waters based on the analysis of 2181 individuals from 92 species (including fish, sharks, and turtles; Brodie et al., 2018). The understanding of circumpolar species habitat use has been transformed by MEOP (e.g., southern elephant seals; Hindell et al., 2016). MMMAP compared global movement patterns across >2,500 individuals from 50 marine vertebrates including whales, sharks, seals, seabirds, polar bears, sirenians, and turtles, showing a remarkable convergence between movements within the coastal and open ocean (Sequeira et al., 2018).
The value of data sharing has been identified by the animal telemetry community (Nguyen et al., 2017), and the outputs from large collaborative efforts collating and analyzing tracking data are revealing that many of the key questions in animal movement (Hays et al., 2016) can only be answered through data pooling. To assist the development of such studies, a select group of credible online data repositories is now emerging (Table 1; Campbell et al., 2016). These include, for example, OTN, BirdLife International (2012) and Movebank (Wikelski and Kays, 2010). Collectively, such repositories are globally accessible, strive to use open source software and common (or at least easily mappable) formats, and amass and curate large quantities of movement data and metadata across thousands of species extending across most regions of the globe (Hussey et al., 2015; Kays et al., 2015). Analysis of the large spatial and temporal datasets now available will allow establishment of baselines from which large-scale (1000s km) and long-term (decadal) changes can be identified, which in turn will provide conservation benefits through informed planning and management (Allen and Singh, 2016; Fraser et al., 2018), and will “increase global communication, scope for collaboration, intellectual advancement, and funding opportunities” (Hussey et al., 2015).
Pooling datasets collected in disparate ways with different instruments, or secondarily derived from other primary data (e.g., model simulation outputs), will, however, bring new analytical challenges. A potential way to address some of these challenges is through multi-disciplinary approaches, promoting the engagement of researchers from disparate fields, including ecologists, physiologists, physicists, mathematicians, and computer and visualization scientists. A combination of existing and new analytical techniques will most likely be required to realize the full potential of the large amount of telemetry data now in existence (Hussey et al., 2015) and facilitate the use of big data approaches to significantly enhance our understanding of animal movement (Hampton et al., 2013; Meekan et al., 2017; Rodríguez et al., 2017; Thums et al., 2018).
For studies that integrate observations from telemetry data into oceanographic observing, there are clear mutual benefits of collaboration between ecologists who generally deploy tags, and oceanographers who are also end-users of the data. The need for international coordination of deployment of animal-borne sensors to address data coverage gaps was recognized more than 15 years ago, in particular the challenge of coordinating resources to enable simultaneous deployments across several sub-Antarctic sites. To address this, the project SEaOS (Southern Elephant seals as Oceanographic Samplers) was launched in 2003 with participants from five national groups. This led to the MEOP program that began during the International Polar Year period in 2008–2009.
MEOP has now transformed into a large consortium that acts to bridge the scientific teams deploying the tags and those using the data (Treasure et al., 2017). The MEOP data (Figure 3) are made available to the global scientific community through the data portal (http://www.meop.net). Similar efforts are underway within other components of the global animal telemetry and oceanographic community, including the US Animal Telemetry Network (https://atn.ioos.us /, Block et al., 2016), the EuroGOOS Animal-Borne Instrument (ABI) Task Team in Europe, the Integrated Marine Observing System (through its Animal Tracking Facility (IMOS ATF; imos.org.au), the Canadian Ocean Tracking Network (oceantrackingnetwork.org/), and the framework provided by the Observations Coordination Group of the Joint WMO-IOC Technical Commission for Oceanography and Marine Meteorology (JCOMM-OCG; Table 1). Future success in integrating data from animal-borne instruments into ocean observing systems will depend on three key requirements: sufficient data quality, data standardization and robust data delivery.
Challenges of Incorporating Data From Animal-Borne Platforms Into Operational Oceanography Systems
Despite the successes of international and cross-disciplinary collaboration between ecologists and oceanographers, hydrographic information collected from animal-borne platforms have too rarely been used routinely for operational oceanography. A primary reason for this has been the difficulty to obtain dedicated resources in ocean data centers for the processing of animal data, encompassing the registration of the individual tags (also known as “platforms”), the data processing and the subsequent uptake of their data through the Global Telecommunication System (GTS) to, among others, the climate operations community. For example, data from CTD-SRDLs are directly managed by the instrument manufacturer (SMRU, University of St. Andrews), where the data are decoded and the profiles rebuilt before distribution through the British Oceanographic Data Center (BODC). At the BODC, the data are then automatically converted into either TESAC or BUFR formats and sent to the GTS for operational use. However, in contrast to what is done for Argo float data, BODC has to date no dedicated resources to quality control and flag the animal data before transmission to the GTS, limiting importantly the usability of the data for data assimilation.
The complexity of encoding, decoding, and transmitting these data and posting them on the GTS is in part the result of unavoidable constraints imposed by both the animals' behavior, the small size of the tags and the CLS Argos data transmission system. Tags on animals must be as small as possible so as to not adversely affect the animal; therefore, the energy available to the tags is extremely limited. The animals themselves further constrain the way data is processed and sent because of their behavior, largely because they vary their diving and surfacing behavior in an unpredictable way, only returning to the surface infrequently for brief periods which imposes further bandwidth limitations (see Photopoulou et al., 2015 for details). Data received from the tags may be transmitted hours or even days after their collection, further delaying data reception and data delivery. This is because the data is buffered for transmission and the success rates of transmission depend on how long the animal stays at the surface and the availability of satellites at the time of surfacing. Taken together, these complexities mean that real time processing and data dissemination may not be as straightforward to implement as that from the more predictable data stream from Argo floats.
An important component of data uptake, use and integration is access to the individual platform metadata. Currently animal metadata are not centralized or easily accessed and given that the Joint Technical Commission for Oceanography (JCOMMs) in situ Observing Programmes Support Center (JCOMMOPS) already manages platform metadata for gliders, Argo float and sensors on ships, it follows that as part of the broader and globally comprehensive ocean observing system, the physical environment data from animal-borne tags could also be managed by JCOMMOPS. The basic metadata associated with the observations would ideally include: World Meteorological Organization (WMO) identifying numbers, the principal investigator's name, species name, sensor information from the manufacturer, tag program configurations, sensor calibration, diagnostics and data adjustment coefficients and the quality control details and quality flags. Additional effort on the part of projects deploying the animal tags is needed to improve metadata availability.
Recent Technological Advances and Future Directions
Developments in Telemetry Technology
In the decade since OceanObs 09, the application of animal telemetry has provided significant amounts of data from otherwise difficult to sample situations. Furthermore, ocean observing using animal-borne platforms has demonstrated the importance of animal telemetry data to a broader understanding of ocean processes (Roquet et al., 2013). Thus, improvements in accuracy and reliability as well as expanding the range of sensors on animal tags is clearly important as is broadening applicability more and varied species. Developments include new, small, low-power sensors that sample additional parameters capable at extremely low duty cycles to allow for continuous monitoring of animals. Sensors for fluorescence (Guinet et al., 2013), oxygen (Bailleul et al., 2015), light levels (Teo et al., 2004), sound (Cazau et al., 2017), acceleration (Carroll et al., 2014, 2016; Cox et al., 2018), and active sonar (Lawson et al., 2015) have all been deployed (Figure 1) but most require refinement. Each of these contribute to a better understanding of the link between physical, biogeochemical, and ecological processes.
Revolutions in sensor technology are also being translated into a new generation of sensors to track marine life, with significantly reduced footprints and power requirements, including the capacity to harvest power from the environment, better accommodating animal anatomy and movement and containing significantly enhanced capacities for data storage and analysis. Examples of these revolutionary sensors include the “marine skin,” a flexible-stretchable silicon-printed sensor system that brings the concept of wearable to marine animal tags (Nassar et al., 2018), new magnetic sensors to monitor animal behaviors in detail (Kaidarova et al., 2018a), and graphene-based flexible, ultrathin, and light salinity sensors to acquire oceanographic information (Kaidarova et al., 2018b).
Improved Metadata Standardization
For data systems, community-wide data standards for the exchange of tracking data are well advanced (e.g., Benson et al., 2018; IODE OBIS Event Data workshop on Animal Tagging Tracking F. w., 2018), but not yet universally accepted. The development of coordinated systems that provide information on animal movements and distributions globally, in (near) real-time, are a necessity given the rapid expansion in the use of the technology and would be a strong asset for GOOS. Globally, there are already a number of extant but independently evolved web-based platforms for the management and analysis of animal movement data (Table 1). Collating data into these repositories provides internal standardization and accessibility. For example, Zoatrack is hosted on the Atlas of Living Australia and has a relational data model based on animal identifiers, timestamps and locations, for satellite or GPS based movement data that are provided via direct file uploads or automated feed from the Argos satellite network (Dwyer et al., 2015). It also has a spatial interface where environmental spatial layers can be added, and data can be discovered by species or location. The Atlas of Living Australia typically ingests data in Simple Darwin Core, a flat file format, which accommodates simple occurrence data well (Newman et al., 2018). ZoaTrack records are summarized into this format by representing a track as an occurrence record with a summary footprint over a date range.
An implementation like this as a metadata catalog allows tracking data to be discoverable and begins opening possibilities for API development and machine interoperability between other external systems and users. However, there will be challenges in accomplishing the latter as data fields and vocabularies vary among the different systems due to their independent evolution. Recently, a roadmap indicating a positive way to negotiate these inconsistencies has been proposed (Sequeira et al., under review). It will also be important to ensure that data are not available to individuals or organizations who could use it to exploit or otherwise disturb or harass tagged wildlife or their untagged conspecifics (Cooke et al., 2017), and new frameworks are being developed to address these sensitive issues (Lennox et al., under review).
A Step Change in Analysis—Big Data
Challenges are arising for analysis of the dramatically increasing amount of acoustic and satellite tracking data. The telemetry field is growing in a similar way to other fields of research where “big data” analyses are now commonplace (e.g., human mobility, Meekan et al., 2017). For animal telemetry, this starts with finding approaches to permit faster and more efficient computing and model fitting (e.g., Whoriskey et al., 2017; Jonsen et al., 2018). The fields of Statistical Physics and Complex Systems have been developing and applying techniques that deal with big data of movement. These techniques have developed from classical work focused on single trajectories and how analyzing collective movements (e.g., Vicsek and Zafeiris, 2012; Morin et al., 2017) can improve an understanding of search strategies and their properties (e.g., Benichou et al., 2011). Collective movement has also been studied using network-based techniques, either focusing on animal social networks (e.g., Krause et al., 2013; Daniels et al., 2017) and leadership (e.g., Jacoby et al., 2016; Mwaffo et al., 2018), detecting patterns and drivers of movement across multiple individuals (e.g., Abrahms et al., 2017; Rodríguez et al., 2017), or by trying to understand communication and transmission of culture (e.g., Carroll et al., 2015; Sasaki and Biro, 2017). Advances made using such approaches are generating new insights about animal movements and their drivers (Rodríguez et al., 2017). The real-time nature of marine animal telemetry can also be used to inform real-time management actions, such as interventions with ship traffic to avoid collisions between marine animals and vessels, and warning beach goers of risk of shark presence (Hazen et al., 2017; Pirotta et al., 2019). This requires real-time integration and analyses of massive amounts of data, something only possible with the assistance of machine learning tools.
Animal Tracking as an Ocean Observing Tool 2019–2029
The advancement of human technologies has made the ocean the next great frontier for industrial development (McCauley et al., 2015; Ogburn et al., 2017; World Economic Forum, 2017). For this “Blue Growth” agenda to be sustainable, and to ensure that rapidly expanding marine developments do not compromise the existing socioeconomic benefits and essential ecosystem services humanity derives from the ocean, managers and policy makers need to be informed by comprehensive monitoring of the ocean. Animal telemetry has a crucial role to play in this task. The species selected for tracking are typically of high value either because of their role in the human food supply or because of the roles they play in ecosystems (Cooke, 2008; Fraser et al., 2018). Many of these animals move on scales ranging from local (a few meters to tens of kms) to entire ocean basins (thousands of kms), in order to meet their needs.
Identifying the pathways that they follow and the habitats they use is important for informed management and implementing effective conservation actions. Telemetry is an ideal tool to provide this information. Meanwhile, environmental conditions in the ocean are changing in ways not completely understood, driven largely by anthropogenic climate forcing among other causes. Animal habitats and movement pathways will change with these environmental characteristics, as will the environmental services the ocean provides, and the risks posed to people who live and work on the ocean. Greater and better observations of the physical environment are required to manage these risks, on a scale comparable with what has been achieved for terrestrial weather forecasting. Enlisting “animal oceanographers” cost-effectively provides relevant information in areas that are difficult to reach and need to be sampled. Sensor technology is rapidly evolving, and the creation of new, miniaturized sensors and data flows will allow scientists to address emerging issues, such as ocean acidification. However, the large amount of data to be generated through these technological improvements will need a refocus of attention and resources directed toward the challenge of storing, curating, analyzing, and communicating the knowledge, stemming from our greatly expanded observations.
Observations obtained through animal telemetry now span oceanography and physics through to ecology and animal physiology and provide critical information for detecting and attributing change in the marine environment. The high quality of the data and its temporal and spatial breadth is critical to GOOS and forms the basis of the proposed new EcoBio EOVs. Technological advances and the recent exponential growth of animal telemetry enabling the sampling of a wide range of environments (ranging from coastal to open oceans and from tropical to polar regions) has made observations from animal borne tags a mature contributor to ocean observation.
Author Contributions
RH, AS, FR, KKo, XZ, and MF: conception, core authorship, senior editing and writing. MHe, CS, CM, FW, MMe, GC, SB, MHi, and IJ: editing and written contribution. DPC, BB, MMu, BW, MW, KA, MB, LB, SJB, DC, J-BC, SJC, PC, PJNdB, TJ, CD, VE, LF, JF-G, KG, YG, CG, MHa, GH, EH, LH, CH, SI, SJ, KKi, KMK, CL, TM, MN, LP, BP, NQ, GR, KS, DWS, ET, MT, AMT, AWT, GW, and YY: written contribution.
Conflict of Interest Statement
The authors declare that the research was conducted in the absence of any commercial or financial relationships that could be construed as a potential conflict of interest.
References
Aarestrup, K., Økland, F., Hansen, M. M., Righton, D., Gargan, P., Castonguay, M., et al. (2009). Oceanic spawning migration of the European eel (Anguilla anguilla). Science 325, 1660–1660. doi: 10.1126/science.1178120
Aarts, G., MacKenzie, M., McConnell, B., Fedak, M., and Matthiopoulos, J. (2008). Estimating space-use and habitat preference from wildlife telemetry data. Ecography 31, 140–160. doi: 10.1111/j.2007.0906-7590.05236.x
Abrahms, B., Seidel, D. P., Dougherty, E., Hazen, E. L., Bograd, S. J., Wilson, A. M., et al. (2017). Suite of simple metrics reveals common movement syndromes across vertebrate taxa. Mov. Ecol. 5:2. doi: 10.1186/s40462-017-0104-2
Albert, A., Echevin, V., Lévy, M., and Aumont, O. (2010). Impact of nearshore wind stress curl on coastal circulation and primary productivity in the Peru upwelling system. J. Geophys. Res. 115:C12033. doi: 10.1029/2010JC006569
Allen, A. M., and Singh, N. J. (2016). Linking movement ecology with wildlife management and conservation. Front. Ecol. Evol. 3:155. doi: 10.3389/fevo.2015.00155
Arthun, M., Nicholls, K. W., Makinson, K., Fedak, M. A., and Boehme, L. (2012). Seasonal inflow of warm water onto the southern Weddell Sea continental shelf, Antarctica. Geophys. Res. Lett. 39:L17601. doi: 10.1029/2012GL052856
Auger-Méthé, M., Albertsen, C. M., Jonsen, I. D., Derocher, A. E., Lidgard, D. C., Studholme, K. R., et al. (2017). Spatiotemporal modelling of marine movement data using Template Model Builder (TMB). Mar. Ecol. Prog. Ser. 565, 237–249. doi: 10.3354/meps12019
Avgar, T., Potts, J. R., Lewis, M. A, and Boyce, M. S. (2016). Integrated step selection analysis: bridging the gap between resource selection and animal movement. Methods Ecol. Evol. 7, 619–630. doi: 10.1111/2041-210X.12528
Bailey, J. D., Wallis, J., and Codling, E. A. (2018). Navigational efficiency in a biased and correlated random walk model of individual animal movement. Ecology 99, 217–223. doi: 10.1002/ecy.2076
Bailleul, F., Vacquie-Garcia, J., and Guinet, C. (2015). Dissolved oxygen sensor in animal-borne instruments: an innovation for monitoring the health of oceans and investigating the functioning of marine ecosystems. PLoS ONE 10:e0132681. doi: 10.1371/journal.pone.0132681
Barton, K. A., Phillips, B. L., Morales, J. M., and Travis, J. M. J. (2009). The evolution of an 'intelligent' dispersal strategy: biased, correlated random walks in patchy landscapes. Oikos 118, 309–319. doi: 10.1111/j.1600-0706.2008.16936.x
Bastille-Rousseau, G., Potts, J. R., Schaefer, J. A., Lewis, M. A., Ellington, E. H., Rayl, N. D., et al. (2015). Unveiling trade-offs in resource selection of migratory caribou using a mechanistic movement model of availability. Ecography 38, 1049–1059. doi: 10.1111/ecog.01305
Benichou, O., Loverdo, C., Moreau, M., and Voituriez, R. (2011). Intermittent search strategies. Rev. Modern Phys. 83:81. doi: 10.1103/RevModPhys.83.81
Benson, A., Appeltans, W., Bajona, L., Bosch, S., Cowley, P., De Pooter, D., et al. (2018). Outcomes of the international oceanographic data and information exchange ocean biogeographic information system OBIS-event-data workshop on animal tagging and tracking. Biodivers. Inform. Sci. Standards 2:e25728. doi: 10.3897/biss.2.25728
Bestley, S., Jonsen, I. D., Hindell, M. A., Guinet, C., and Charrassin, J. B. (2013). Integrative modelling of animal movement: incorporating in situ habitat and behavioural information for a migratory marine predator. Proc. R. Soc. Biol. Sci. 280:20122262. doi: 10.1098/rspb.2012.2262
BirdLife International (2012). Tracking Devices Provide New Insights Into Seabird Migration. Available online at: http://www.birdlife.org on 07/11/2018
Biuw, M., Boehme, L., Guinet, C., Hindell, M., Costa, D., Charrassin, J. B., et al. (2007). Variations in behavior and condition of a Southern Ocean top predator in relation to in situ oceanographic conditions. Proc. Natl. Acad. Sci. 104, 13705–13710. doi: 10.1073/pnas.0701121104
Block, B. A. (2005). Physiological ecology in the 21st century: advancements in biologging science. Integr. Compar. Biol. 45, 305–320. doi: 10.1093/icb/45.2.305
Block, B. A., Dewar, H., Farwell, C., and Prince, E. D. (1998). A new satellite technology for tracking the movements of Atlantic bluefin tuna. Proc. Natl. Acad. Sci. 95, 9384–9389. doi: 10.1073/pnas.95.16.9384
Block, B. A., Holbrook, C. M., Simmons, S. E., Holland, K. N., Ault, J. S., Costa, D. P., et al. (2016). Toward a national animal telemetry network for aquatic observations in the United States. Anim. Biotelemetry 4:6. doi: 10.1186/s40317-015-0092-1
Block, B. A., Jonsen, D. I., Jorgensen, S. J., Winship, A. J., Shaffer, S. A., Bograd, S. J., et al. (2011). Tracking apex marine predator movements in a dynamic ocean. Nature 475, 86–90. doi: 10.1038/nature10082
Boehlert, G. W., Costa, D. P., Crocker, D. E., Green, P., O'Brien, T., Levitus, S., et al. (2001). Autonomous pinniped environmental samplers: using instrumented animals as oceanographic data collectors. J. Atmos. Oceanic Technol. 18, 1882–1893. doi: 10.1175/1520-0426(2001)018andlt;1882:APESUIandgt;2.0.CO;2
Boehme, L., Lovell, P., Biuw, M., Roquet, F., Nicholson, J., Thorpe, S. E., et al. (2009). Technical note: animal-borne CTD-Satellite Relay Data Loggers for real-time oceanographic data collection. Ocean Sci. 5, 685–695. doi: 10.5194/os-5-685-2009
Boehme, L., Meredith, M., Thorpe, S., Biuw, M., and Fedak, M. A. (2008). C9.The ACC frontal system in the South Atlantic: monitoring using merged Argo and animal-borne sensor data. J. Geophys. Res. 113:C9. doi: 10.1029/2007JC004647
Bograd, S. J., Block, B. A., Costa, D. P., and Godley, B. J. (2010). Biologging technologies: new tools for conservation. Introduction Endangered Species Res. 10, 1–7. doi: 10.3354/esr00269
Brodie, S., Lédée, E. J. I., Heupel, M. R., Babcock, R. C., Campbell, H. A., Gledhill, D. C., et al. (2018). Continental-scale animal tracking reveals functional movement classes across marine taxa. Sci. Rep. 8:5. doi: 10.1038/s41598-018-21988-5
Campbell, H. A., Urbano, F., Davidson, S., Dettki, H., and Cagnacci, F. (2016). A plea for standards in reporting data collected by animal-borne electronic devices. Anim. Biotelemetry 4:96. doi: 10.1186/s40317-015-0096-x
Carroll, E. L., Baker, C. S., Watson, M., Alderman, R., Bannister, J., Gaggiotti, O. E., et al. (2015). Cultural traditions across a migratory network shape the genetic structure of southern right whales around Australia and New Zealand. Sci. Rep. 5:16182. doi: 10.1038/srep16182
Carroll, G., Cox, M., Harcourt, R., Pitcher, B., Slip, D., and Jonsen, I. (2017). Hierarchical influences of prey distribution on patterns of prey capture by a marine predator. Funct. Ecol. 31, 1750–1760. doi: 10.1111/1365-2435.12873
Carroll, G., Everett, J. D., Harcourt, R., Slip, D., and Jonsen, I. (2016). High sea surface temperatures driven by a strengthening current reduce foraging success by penguins. Sci. Rep. 6:22236. doi: 10.1038/srep22236
Carroll, G., Slip, D., Jonsen, I., and Harcourt, R. (2014). Supervised accelerometry analysis can identify prey capture by penguins at sea. J. Exp. Biol. 217, 4295–4302. doi: 10.1242/jeb.113076
Cazau, D., Bonnel, J., Jouma'a, J., Le Bras, Y., and Guinet, C. (2017). Measuring the marine soundscape of the Indian Ocean with southern elephant seals used as acoustic gliders of opportunity. J. Atmos. Oceanic Technol. 34, 207–223. doi: 10.1175/JTECH-D-16-0124.1
Chantrapornsyl, S., Charuchinda, M., Sakamoto, W., Arai, N., Klom-In, W., Kittiwattanawong, K., et al. (2002). Migration Tracking of Green Turtles (Chelonia mydas) Using Platform Transmitter Terminals. Available online at: https://www.dmcr.go.th/upload/dt/file/file-612-966145833.pdf
Charrassin, J. B., Hindell, M., Rintoul, S. R., Roquet, F., Sokolov, S., Biuw, M., et al. (2008). Southern Ocean frontal structure and sea-ice formation rates revealed by elephant seals. Proc. Natl. Acad. Sci. 105, 11634–11639. doi: 10.1073/pnas.0800790105
Cherdsukjai, P., Thongsukdee, S., Passada, S., and Prempree, T. (2015). Population sizes of Bryde's whale (Balaenoptera edeni) in the Upper Gulf of Thailand, estimated by mark and recapture method. Proc. Des. Symp. Conser. Ecosystem 3. doi: 10.14989/198821
Cherdsukjai, P., Thongsukdee, S., Passada, S., Prempree, T., and Yaovasuta, P. (2016). “Satellite tracking of Bryde's whale (Balaenoptera edeni), in the Upper Gulf of Thailand,” in Proceedings of 5th Marine Science Conference. 1-3 June 2016, Bangkok. 104–114.
Cherry, S. G., Derocher, A. E., Thiemann, G. W., and Lunn, N. J. (2013). Migration phenology and seasonal fidelity of an Arctic marine predator in relation to sea ice dynamics. J. Anim. Ecol. 82, 912–921. doi: 10.1111/1365-2656.12050
Constable, A. J., Costa, D. P., Schofield, O., Newman, L., Urban, E. R., Fulton, E. A., et al. (2016). Developing priority variables (“ecosystem Essential Ocean Variables” - eEOVs) for observing dynamics and change in Southern Ocean ecosystems. J. Mar. Syst. 161, 26–41. doi: 10.1016/j.jmarsys.2016.05.003
Cooke, J. G., and Brownell, R. L. Jr. (2018). Balaenoptera edeni. The IUCN Red List of Threatened Species 2018:e.T2476A50349178.
Cooke, S. J. (2008). Biotelemetry and biologging in endangered species research and animal conservation: relevance to regional, national, and IUCN Red List threat assessments. Endangered Species Res. 4, 165–185. doi: 10.3354/esr00063
Cooke, S. J., Nguyen, V. M., Kessel, S. T., Hussey, N., Young, N., and Ford, A. T. (2017). Troubling and unanticipated issues at the frontier of animal tracking for conservation and management. Conserv. Biol. 31, 1205–1207. doi: 10.1111/cobi.12895
Costa, D. P., Block, B. A., Bograd, S., Fedak, M. A., and Gunn, J. S. (2010a). TOPP as a marine life observatory: using electronic tags to monitor the movements, behaviour and habitats of marine vertebrates. Proc. OceanObs 9, 21–25. doi: 10.5270/OceanObs09.cwp.19
Costa, D. P., Breed, G. A., and Robinson, P. W. (2012). New insights into pelagic migrations: implications for ecology and conservation. Ann. Rev. Ecol. Evol. Syst. 43, 73–96. doi: 10.1146/annurev-ecolsys-102710-145045
Costa, D. P., Huckstadt, L. A., Crocker, D. E., McDonald, B. I., Goebel, M. E., and Fedak, M. A. (2010b). Approaches to studying climatic change and its role on the habitat selection of antarctic pinnipeds. Integr. Comput. Biol. 50, 1018–1030. doi: 10.1093/icb/icq054
Costa, D. P., Klinck, J., Hofmann, E. E., Dinniman, M., and Burns, J. M. (2008). Upper ocean variability in west Antarctic Peninsula continental shelf waters as measured using instrumented seals. Deep Sea Res. 55, 323–337. doi: 10.1016/j.dsr2.2007.11.003
Cox, S. L., Orgeret, F., Gesta, M., Rodde, C., Heizer, I., Weimerskirch, H., et al. (2018). Processing of acceleration and dive data on-board satellite relay tags to investigate diving and foraging behaviour in free-ranging marine predators. Methods Ecol. Evol. 9, 64–77. doi: 10.1111/2041-210X.12845
Daniels, B. C., Krakauer, D. C., and Flack, J. C. (2017). Control of finite critical behaviour in a small-scale social system. Nat. Commun. 8:14301. doi: 10.1038/ncomms14301
Donaldson, M. R., Hinch, S. G., Suski, C. D., Fisk, A. T., Heupel, M. R., and Cooke, S. J. (2014). Making connections in aquatic ecosystems with acoustic telemetry monitoring. Front. Ecol. Environ. 12, 565–573. doi: 10.1890/130283
Dwyer, R. G., Brooking, C., Brimblecombe, W., Campbell, H. A., Hunter, J., Watts, M., et al. (2015). An open web-based system for the analysis and sharing and analysis of animal tracking data. Anim. Biotelemetry 3:8. doi: 10.1186/s40317-014-0021-8
Everett, A., Kohler, J., Sundfjord, A., Kovacs, K. M., Torsvik, T., Pramanik, A., et al. (2018). Subglacial discharge plume behaviour revealed by CTD-instrumented ringed seals. Sci. Rep. 8:13467. doi: 10.1038/s41598-018-31875-8
Fedak, M., Lovell, P., McConnell, B., and Hunter, C. (2002). Overcoming the constraints of long range radio telemetry from animals: getting more useful data from smaller packages. Integr. Compar. Biol. 42, 3–10. doi: 10.1093/icb/42.1.3
Fedak, M. A. (2004). Marine animals as platforms for oceanographic sampling: a “win/win” situation for biology and operational oceanography. Memoirs Natl. Institute Polar Res. 58, 133–147.
Fedak, M. A. (2013). The impact of animal platforms on polar ocean observation. Deep Sea Res. 88, 7–13. doi: 10.1016/j.dsr2.2012.07.007
Fossette, S., Putman, N. F., Lohmann, K. J., Marsh, R., and Hays, G. C. (2012). A biologist's guide to assessing ocean currents: a review. Mar. Ecol. Prog. Ser. 457, 285–301. doi: 10.3354/meps09581
Fraser, K. C., Davies, K. T. A., Davy, C. M., Ford, A. T., Tyler Flockhard, D. T., and Martins, G. C. (2018). Tracking the conservation promise of movement ecology. Front. Ecol. Evol. 6:150. doi: 10.3389/fevo.2018.00150
Frazer, E. K., Langhorne, P. J., Williams, M. J. M., Goetz, K. T., and Costa, D. P. (2018). A method for correcting seal-borne oceanographic data and application to the estimation of regional sea ice thickness. J. Mar. Syst. 187, 250–259. doi: 10.1016/j.jmarsys.2018.08.002
Goetz, K. T. (2015). Movement, Habitat, and Foraging Behavior of Weddell Seals (Leptonychotes weddellii) In the Western Ross Sea, Antarctica. Dissertation. University of California, Santa Cruz, CA.
Goto, Y., Yoda, K., and Sato, K. (2017). Asymmetry hidden in birds' tracks reveals wind, heading, and orientation ability over the ocean. Sci. Adv. 3:e1700097. doi: 10.1126/sciadv.1700097
Griffin, L. P., Brownscombe, J. W., Adams, A. J., Boucek, R. E., Finn, J. T., Heithaus, M. R., et al. (2018). Keeping up with the Silver King: using cooperative acoustic telemetry networks to quantify the movements of Atlantic tarpon (Megalops atlanticus) in the coastal waters of the southeastern United States. Fish. Res. 205, 65–76. doi: 10.1016/j.fishres.2018.04.008
Grist, J. P., Josey, S. A., Boehme, L., Meredith, M. P., Davidson, F. J. M., Stenson, G. B., et al. (2011). Temperature signature of high latitude Atlantic boundary currents revealed by marine mammal-borne sensor and Argo data. Geophys. Res. Lett. 38:15. doi: 10.1029/2011GL048204
Guinet, C., Xing, X., Walker, E., Monestiez, P., Marchand, S., Picard, B., et al. (2013). Calibration procedures and first data set of Southern Ocean chlorophyll a profiles collected by elephant seals equipped with a newly developed CTD-fluorescence tags. Earth Syst. Sci. Data 5, 15–29. doi: 10.5194/essd-5-15-2013
Hampton, S. E., Strasser, C. A., Tewksbury, J. J., Gram, W. K., Budden, A. E., Batcheller, A. L., et al. (2013). Big data and the future of ecology. Front. Ecol. Environ. 11, 156–162. doi: 10.1890/120103
Hawkes, L. A., Broderick, A. C., Godfrey, M. H., and Godley, B. J. (2009). Climate change and marine turtles. Endangered Species Res. 7, 137–154. doi: 10.3354/esr00198
Hays, G. C., Bailey, H., Bograd, S. J., Bowen, W. D., Campagna, C., Carmichael, R. H., et al. (2019). Translating marine animal tracking data into conservation policy and management. Trends Ecol. Evol. 34, 385-486, e1–e2. doi: 10.1016/j.tree.2019.01.009
Hays, G. C., Ferreira, L. C., Sequeira, A. M. M., Meekan, M. G., Duarte, C. M., Bailey, H., et al. (2016). Key questions in marine megafauna movement ecology. Trends Ecol. Evol. 31, 463–475. doi: 10.1016/j.tree.2016.02.015
Hays, G. C., Hobson, V. J., Metcalfe, J. D., Righton, D., and Sims, D. W. (2006). Flexible foraging movements of leatherback turtles across the North Atlantic Ocean. Ecology 87, 2647–2656. doi: 10.1890/0012-9658(2006)87[2647:FFMOLT]2.0.CO;2
Hazen, E. L., Abrahms, B., Brodie, S., Carroll, G., Jacox, M. G., Savoca, M. S., et al. (2019). Marine top predators as climate and ecosystem sentinels. Front. Ecol. Environ. (accepted)
Hazen, E. L., Jorgensen, S., Rykaczewski, R. R., Bograd, S. J., Foley, D. G., Jonsen, I. D., et al. (2013b). Predicted habitat shifts of Pacific top predators in a changing climate. Nat. Climate Change 3:234. doi: 10.1038/nclimate1686
Hazen, E. L., Maxwell, S. M., Bailey, H., Bograd, S. J., Hamann, M., Gaspar, P., et al. (2013a). Ontogeny in marine tagging and tracking science: technologies and data gaps. Mar. Ecol. Prog. Ser. 457, 221–240. doi: 10.3354/meps09857
Hazen, E. L., Palacios, D. M., Forney, K. A., Howell, E. A., Becker, E., Hoover, A. L., et al. (2017). WhaleWatch: a dynamic management tool for predicting blue whale density in the California Current. J. Appl. Ecol. 54, 1415–1428. doi: 10.1111/1365-2664.12820
He, R., Liu, Y., and Weisberg, R. H. (2004). Coastal ocean wind fields gauged against the performance of an ocean circulation model. Geophys. Res. Lett. 31:L14303. doi: 10.1029/2003GL019261
Heupel, M. R., Semmens, J. M., and Hobday, A. J. (2006). Automated acoustic tracking of aquatic animals: scales, design and deployment of listening station arrays. Mar. Freshw. Res. 57, 1–13. doi: 10.1071/MF05091
Hindell, M. A., McMahon, C. R., Bester, M. N., Boehme, L., Costa, D., Fedak, M. A., et al. (2016). Circumpolar habitat use in the southern elephant seal: implications for foraging success and population trajectories. Ecosphere 7:e01213. doi: 10.1002/ecs2.1213
Hoenner, X., Huveneers, C., Steckenreuter, A., Simpfendorfer, C., Tattersall, K., Jaine, F., et al. (2018). Australia's continental-scale acoustic tracking database and its automated quality control process. Sci. Data 5:206. doi: 10.1038/sdata.2017.206
Humphries, N. E., Queiroz, N., Dyer, J. R., Pade, N. G., Musyl, M. K., Schaefer, K. M., et al. (2010). Environmental context explains Lévy and Brownian movement patterns of marine predators. Nature 465, 1066–1069. doi: 10.1038/nature09116
Hussey, N. E., Kessel, S. T., Aarestrup, K., Cooke, S. J., Cowley, P. D., Fisk, A. T., et al. (2015). Aquatic animal telemetry: a panoramic window into the underwater world. Science 348, 1221–1231. doi: 10.1126/science.1255642
IODE OBIS Event Data workshop on Animal Tagging and Tracking F. w. (2018). Reports of Meetings of Experts and Equivalent Bodies.
Isachsen, P. E., Sørlie, S. R., Mauritzen, C., Lydersen, C., Dodd, P., and Kovacs, K. M. (2014). Upper-ocean hydrography of the Nordic Seas during the International Polar Year (2007–2008) as observed by instrumented seals and Argo floats. Deep Sea Res. I 93, 41–59. doi: 10.1016/j.dsr.2014.06.012
Jacoby, D. M., Papastamatiou, Y. P., and Freeman, R. (2016). Inferring animal social networks and leadership: applications for passive monitoring arrays. J. R. Soc. Interface 13:676. doi: 10.1098/rsif.2016.0676
Johnson, D. S., London, J. M., Lea, M. A., and Durban, J. W. (2008). Continuous-time correlated random walk model for animal telemetry data. Ecology 89, 1208–1215. doi: 10.1890/07-1032.1
Jonsen, I., McMahon, C., Patterson, T., Auger-Methe, M., Harcourt, R., Hindell, M., et al. (2018). Movement behaviour responses to environment: fast inference of individual variation with a mixed effects model. Ecology 100:e02566. doi: 10.1002/ecy.2566
Jonsen, I. D., Basson, M., Bestley, S., Bravington, M. V., Patterson, T. A., Pedersen, M. W., et al. (2013). State-space models for bio-loggers: a methodological road map. Deep Sea Res. II 88–89, 34–46. doi: 10.1016/j.dsr2.2012.07.008
Jonsen, I. D., Flemming, J. M., and Myers, R. A. (2005). Robust state-space modelling of animal movement data. Ecology 86, 2874–2880. doi: 10.1890/04-1852
Kaidarova, A., Khan, M. A., Amara, S., Geraldi, N., Shamim, K. A., Wilson, R. P., et al. (2018a). Tunable, Flexible composite magnets for marine monitoring applications. Adv. Eng. Mater. 2018:229. doi: 10.1002/adem.201800229
Kaidarova, A., Marengo, M., Marinaro, G., Geraldi, N., Duarte, C. M., and Kosel, J. (2018b). Flexible and biofouling independent salinity sensor. Adv. Mater. Interfaces 2018:1801110. doi: 10.1002/admi.201801110
Kays, R., Crofoot, C., Jetz, W., and Wikelski, M. (2015). Terrestrial animal tracking as an eye on life and planet. Science 348, 1222–1231. doi: 10.1126/science.aaa2478
Kitade, Y., Shimada, K., Tamura, T., Williams, G. D., Aoki, S., Fukamachi, Y., et al. (2014). Antarctic bottom water production from the Vincennes Bay Polynya, East Antarctica. Geophys. Res. Lett.41:2014GL059971 doi: 10.1002/2014GL059971
Krause, J., Krause, S., Arlinghaus, R., Psorakis, I., Roberts, S., and Rutz, C. (2013). Reality mining of animal social systems. Trends Ecol. Evol. 28, 541–551. doi: 10.1016/j.tree.2013.06.002
Laidre, K. L., Stirling, I., Lowry, L. F., Wiig, O., Heide-Jørgensen, M. P., and Ferguson, S. H. (2008). Quantifying the sensitivity of Arctic marine mammals to climate-induced habitat change. Ecol. Appl. 18:sp2. doi: 10.1890/06-0546.1
Langrock, R., King, R., Matthiopoulos, J., Thomas, L., Fortin, D., and Morales, J. M. (2012). Flexible and practical modeling of animal telemetry data: hidden Markov models and extensions. Ecology 93, 2336–2342. doi: 10.1890/11-2241.1
Lawson, G. L., Hückstädt, L. A., Lavery, A. C., Jaffré, F. M., Wiebe, P. H., Fincke, J. R., et al. (2015). Development of an animal-borne “sonar tag” for quantifying prey availability: test deployments on northern elephant seals. Anim. Biotelemetry 3:22. doi: 10.1186/s40317-015-0054-7
Lee, K. A., Huveneers, C., Duong, T., and Harcourt, R. G. (2017). The ocean has depth: two-versus three-dimensional space use estimators in a demersal reef fish. Mar. Ecol. Prog. Ser. 572, 223–241 doi: 10.3354/meps12097
Lennox, R. J., Aarestrup, K., Cooke, S. J., Cowley, P. D., Deng, Z. D., Fisk, A. T., et al. (2017). Envisioning the future of aquatic animal tracking: technology, science, and application. Bioscience 67, 884–896. doi: 10.1093/biosci/bix098
Lennox, R. J., Engler-Palma, C., Kowarski, K., Filous, A., Whitlock, R., Cooke, S. J., et al. (2018). Optimizing marine spatial plans with animal tracking data. Canad. J. Fish. Aquat. Sci. 76, 497–509. doi: 10.1139/cjfas-2017-0495
Leos-Barajas, V., Photopoulou, T., Langrock, R., Patterson, T. A., Watanabe, Y. Y., Murgatroyd, M., et al. (2017). Analysis of animal accelerometer data using hidden Markov models. Methods Ecol. Evol. 8, 161–173. doi: 10.1111/2041-210X.12657
Lidgard, D. C., Bowen, W. D., Jonsen, I. D., McConnell, B. J., Lovell, P., Webber, D. M., et al. (2014). Transmitting species-interaction data from animal-borne transceivers through Service Argos using Bluetooth communication. Methods Ecol. Evol. 5, 864–871. doi: 10.1111/2041-210X.12235
Liew, H. C., Chan, E. H., Papi, F., and Luschi, P. (1995). “Long distance migration of green turtles from Redang Island: the need for regional cooperation in sea turtle conservation,” in Proceedings of the International Congress of Chelonian Conservation. 6-10 July 1995, ed. B. Devaux, Gonfaron, 73–75.
Lone, K., Merkel, B., Lydersen, C., Kovacs, K. M., and Aars, J. (2018). Sea ice resource selection models for polar bears in the Barents Sea subpopulation. Ecography 41, 567–578. doi: 10.1111/ecog.03020
Lydersen, C., Nøst, O. A., Lovell, P., McConnell, B. J., Gammelsrød, T., Hunter, C., et al. (2002). Salinity and temperature structure of a freezing Arctic fjord - monitored by white whales (Delphinapterus leucas). Geophys. Res. Lett. 29:2119. doi: 10.1029/2002GL015462
Mallett, H. K. W., Boehme, L., Fedak, M. A., Heywood, K. J., Stevens, D. P., and Roquet, F. (2018). Variation in the distribution and properties of Circumpolar Deep Water in the eastern Amundsen Sea, on seasonal timescales, using seal-borne tags. Geophys. Res. Lett. 2018:77430. doi: 10.1029/2018GL077430
Manly, B., McDonald, L., Thomas, D., McDonald, T., and Erickson, W. (2002). Resource Selection by Animals: Statistical Design and Analysis for Field Studies. Dordrecht: Kluwer.
Mazor, T., Beger, M., McGowan, J., Possingham, H. P., and Kark, S. (2016). The value of migration information for conservation prioritization of sea turtles in the Mediterranean. Glob Ecol Biogeogr. 25, 540–552. doi: 10.1111/geb.12434
McCauley, D. J., Pinsky, M. L., Palumbi, S. R., Estes, J. A., Joyce, F. H., and Warner, R. R. (2015). Marine defaunation: animal loss in the global ocean. Science 347:1255641. doi: 10.1126/science.1255641
McClintock, B., King, R., Thomas, L., Matthiopoulos, J., McConnell, B., and Morales, J. (2012). A general discrete-time modelling framework for animal movement using multi-scale random walks. Ecol. Monogr. 82, 335–349. doi: 10.1890/11-0326.1
McClintock, B. T., Johnson, D. S., Hooten, M. B., Ver Hoef, J. M., and Morales, J. M. (2014). When to be discrete: the importance of time formulation in understanding animal movement. Mov. Ecol. 2:21. doi: 10.1186/PREACCEPT-1254967273135270
McConnell, B. J., and Fedak, M. A. (1996). Movements of southern elephant seals. Canad. J. Zool. 74, 1485–1496. doi: 10.1139/z96-163
McIntyre, T. (2014). Trends in tagging of marine mammals: a review of marine mammal biologging studies. Afr. J. Mar. Sci. 36, 409–422. doi: 10.2989/1814232X.2014.976655
Meekan, M. G., Duarte, C. M., Fernández-Gracia, J., Thums, M., Sequeira, A. M. M., Harcourt, R., et al. (2017). The ecology of human mobility. Trends Ecol. Evol. 32, 198–210. doi: 10.1016/j.tree.2016.12.006
Mensah, V., Roquet, F., Siegelman-Charbit, L., Picard, B., Pauthenet, E., and Guinet, C. (2018). A Correction for the thermal mass–induced errors of CTD tags mounted on marine mammals. J. Atmos. Oceanic Technol. 35, 1237–1252 doi: 10.1175/JTECH-D-17-0141.1
Meredith, M. P., Nicholls, K. W., Renfrew, I. A., Boehme, L., Biuw, M., and Fedak, M. (2011). Seasonal evolution of the upper-ocean adjacent to the South Orkney Islands, Southern Ocean: Results from a “lazy biological mooring”. Deep Sea Res II 58, 1569–1579. doi: 10.1016/j.dsr2.2009.07.008
Mernild, S. H., Holland, D. M., Holland, D., Rosing-Asvid, A., Yde, J. C., Liston, G. E., et al. (2015). Freshwater flux and spatiotemporal simulated runoff variability into ilulissat icefjord, west greenland, linked to salinity and temperature observations near tidewater glacier margins obtained using instrumented ringed seals. J. Phys. Oceanogr. 45, 1426–1445. doi: 10.1175/JPO-D-14-0217.1
Metcalfe, J. D., Le Quesne, W. J., Cheung, W. W., and Righton, D. A. (2012). Conservation physiology for applied management of marine fish: an overview with perspectives on the role and value of telemetry. Philos. Trans. R. Soc. Lond. B 367, 1746–1756. doi: 10.1098/rstb.2012.0017
Michelot, T., Langrock, R., Bestley, S., Jonsen, I. D., Photopoulou, T., and Patterson, T. A. (2017). Estimation and simulation of foraging trips in land-based marine predators. Ecology 98, 1932–1944. doi: 10.1002/ecy.1880
Miloslavich, P., Bax, N. J., Simmons, S. E., Klein, E., Appeltans, W., Aburto-Oropeza, O., et al. (2018). Essential ocean variables for global sustained observations of biodiversity and ecosystem changes. Global Change Biol. 24, 2416–2433. doi: 10.1111/gcb.14108
Miyazawa, Y., Guo, X., Varlamov, S. M., Miyama, T., Yoda, K., Sato, K., et al. (2015). Assimilation of the seabird and ship drift data in the north-eastern sea of Japan into an operational ocean nowcast/forecast system. Sci. Rep. 5:17672. doi: 10.1038/srep17672
Morin, A., Desreumaux, N., Caussin, J. B., and Bartolo, D. (2017). Distortion and destruction of colloidal flocks in disordered environments. Nat. Phys. 13, 63–67. doi: 10.1038/nphys3903
Mwaffo, V., Keshavan, J., and Humbert, S. (2018). Detecting intermittent switching leadership in coupled dynamical systems. Sci. Rep. 8:10338. doi: 10.1038/s41598-018-28285-1
Nassar, J. M., Khan, S. M., Velling, S. J., Gaxiola, A. D., Shaikh, S. F., Geraldi, N. R., et al. (2018). Compliant lightweight non-invasive standalone “Marine Skin” tagging system. Nat. Flexible Electron. 2:13. doi: 10.1038/s41528-018-0025-1
Nathan, R., Getz, W. M., Revilla, E., Holyoak, M., Kadmon, R., Saltz, D., et al. (2008). A movement ecology paradigm for unifying organismal movement research. Proc. Natl. Acad. Sci. 105, 19052–19059. doi: 10.1073/pnas.0800375105
Newman, P., Dettki, H., and Belbin, L. (2018). What went where when? Representing animal movements as simple darwin core occurrences. Biodivers. Inform. Sci. Standards 2:e25664. doi: 10.3897/biss.2.25664
Nguyen, V., Brooks, J. L., Young, L., Lennox, N., Haddaway, R., et al. (2017). To share or not to share in the emerging era of big data: perspectives from fish telemetry researchers on data sharing. Canad. J. Fish. Aquat. Sci. 74, 1260–1274. doi: 10.1139/cjfas-2016-0261
Nost, O. A., Biuw, M., Tverberg, V., Lydersen, C., Hattermann, T., Zhou, Q., et al. (2011). Eddy overturning of the Antarctic Slope Front controls glacial melting in the Eastern Weddell Sea. J. Geophys. Res. 116:C11. doi: 10.1029/2011JC006965
Ogburn, M. B., Harrison, A.-L., Whoriskey, F., Cooke, S. J., Mills-Flemming, J. E., and Torres, L. G. (2017). Addressing challenges in the application of animal movement ecology to aquatic conservation and management. Front. Mar. Sci. 4:70. doi: 10.3389/fmars.2017.00070
Ohshima, K. I., Fukamachi, Y., Williams, G. D., Nihashi, S., Roquet, F., Kitade, Y., et al. (2013). Antarctic Bottom Water production by intense sea-ice formation in the Cape Darnley polynya. Nat. Geosci. 6:235. doi: 10.1038/ngeo1738
Padman, L., Costa, D. P., Bolmer, S. T., Goebel, M. E., Huckstadt, L. A., Jenkins, A., et al. (2010). Seals map bathymetry of the Antarctic continental shelf. Geophys. Res. Lett. 37:21. doi: 10.1029/2010GL044921
Padman, L., Costa, D. P., Dinniman, M. S., Fricker, H. A., Goebel, M. E., Huckstadt, L. A., et al. (2012). Oceanic controls on the mass balance of Wilkins Ice Shelf, Antarctica. J. Geophys. Res Oceans 117:C1. doi: 10.1029/2011JC007301
Park, Y.-H., Roquet, F., Durand, I., and Fuda, J.-L. (2008). Large-scale circulation over and around the Northern Kerguelen Plateau. Deep Sea Res. II 55, 566–581. doi: 10.1016/j.dsr2.2007.12.030
Patterson, T. A., Basson, M., Bravington, M. V., and Gunn, J. (2009). Classifying movement behaviour in relation to environmental conditions using hidden Markov models. J. Anim. Ecol. 78, 1113–1123. doi: 10.1111/j.1365-2656.2009.01583.x
Patterson, T. A., Thomas, L., Wilcox, C., Ovaskainen, O., and Matthiopoulos, J. (2008). State-space models of individual animal movement. Trends Ecol. Evol. 23, 87–94. doi: 10.1016/j.tree.2007.10.009
Pauthenet, E., Roquet, F., Madec, G., Guinet, C., Hindell, M., McMahon, C. R., et al. (2018). Seasonal meandering of the Polar Front upstream of the Kerguelen Plateau. Geophys. Res. Lett. 45:614. doi: 10.1029/2018GL079614
Pellichero, V., Sallée, J.-B., Schmidtko, S., Roquet, F., and Charrassin, J.-B. (2017). The ocean mixed layer under Southern Ocean sea-ice: Seasonal cycle and forcing. J. Geophys. Res. Oceans 122, 1608–1633. doi: 10.1002/2016JC011970
Photopoulou, T., Fedak, M. A., Matthiopoulos, J., McConnell, B., and Lovell, P. (2015). The generalized data management and collection protocol for conductivity-temperature-depth satellite relay data loggers. Anim. Biotelemetry 3:21. doi: 10.1186/s40317-015-0053-8
Pickett, M. H., Tang, W., Rosenfeld, L. K., and Wash, C. H. (2003). QuikSCAT satellite comparisons with nearshore buoy wind data off the U.S. west coast. J. Atmos. Ocean Technol. 20, 1869–1879. doi: 10.1175/1520-0426(2003)020andlt;1869:QSCWNBandgt;2.0.CO;2
Pirotta, V., Grech, A., Jonsen, I. D., Laurance, W. F., and Harcourt, R. G. (2019). Consequences of global shipping traffic for marine giants. Front. Ecol. Environ. 17, 39–47. doi: 10.1002/fee.1987
Queiroz, N., Humphries, N. E., Mucientes, G., Hammerschlag, N., Lima, F. P., Scales, K. L., et al. (2016). Ocean-wide tracking of pelagic sharks reveals extent of overlap with longline fishing hotspots. Proc. Natl. Acad. Sci. U.S.A. 113, 1582–1587. doi: 10.1073/pnas.1510090113
Raymond, B., Lea, M. A., Patterson, T., Andrews-Goff, V., Sharples, R., Charrassin, J. B., et al. (2015). Important marine habitat off east Antarctica revealed by two decades of multi-species predator tracking. Ecography 38, 121–129. doi: 10.1111/ecog.01021
Riser, S. C., Freeland, H. J., Roemmich, D., Wijffels, S., Troisi, A., Belbéoch, M., et al. (2016). Fifteen years of ocean observations with the global Argo array. Nat. Climate Change 6:145. doi: 10.1038/nclimate2872
Rodríguez, J. P., Fernández-Gracia, J., Thums, M., Hindell, M. A., Sequeira, A. M., Meekan, M. G., et al. (2017). Big data analyses reveal patterns and drivers of the movements of southern elephant seals. Sci. Rep. 7:112. doi: 10.1038/s41598-017-00165-0
Roemmich, D., Johnson, G. C., Riser, S., Davis, R., Gilson, J., Owens, W. B., et al. (2009). The Argo Program: Observing the global ocean with profiling floats. Oceanography 22, 34–43. doi: 10.5670/oceanog.2009.36
Roquet, F., Boehme, L., Block, B., Charrassin, J. B., Costa, D., Guinet, C., et al. (2017). Ocean observations using tagged animals. Oceanography 30:139. doi: 10.5670/oceanog.2017.235
Roquet, F., Charrassin, J. B., Marchand, S., Boehme, L., Fedak, M., Reverdin, G., et al. (2011). Delayed-mode calibration of hydrographic data obtained from animal-borne satellite relay data loggers. J. Atmos. Ocean Technol. 28, 787–801. doi: 10.1175/2010JTECHO801.1
Roquet, F., Park, Y.-H., Guinet, C., Bailleul, F., and Charrassin, J.-B. (2009). Observations of the Fawn Trough Current over the Kerguelen Plateau from instrumented elephant seals. J. Mar. Syst. 78, 377–393. doi: 10.1016/j.jmarsys.2008.11.017
Roquet, F., Williams, G. D., Hindell, M., Harcourt, R., McMahon, C., Guinet, C., et al. (2014). A Southern Indian Ocean database of hydrographic profiles obtained with instrumented elephant seals. Nat. Sci. Data 1:140028. doi: 10.1038/sdata.2014.28
Roquet, F., Wunsch, C., Forget, G., Heimbach, P., Guinet, C., Reverdin, G., et al. (2013). Estimates of the Southern Ocean general circulation improved by animal-borne instruments. Geophys. Res. Lett. 40, 6176–6180. doi: 10.1002/2013GL058304
Sánchez-Román, A., Gómez-Navarro, L., Fablet, R., Oro, D., Mason, E., Arcos, J. M., et al. (2019). Rafting behaviour of seabirds as a proxy to describe surface ocean currents in the Balearic Sea. Sci. Rep. 9:17775. doi: 10.1038/s41598-018-36819-w
Sasaki, T., and Biro, D. (2017). Cumulative culture can emerge from collective intelligence in animal groups. Nat. Commun. 8:15049. doi: 10.1038/ncomms15049
Schick, R. S., Loarie, S. R., Colchero, F., Best, B. D., Boustany, A., Conde, D. A., et al. (2008). Understanding movement data and movement processes: current and emerging directions. Ecol. Lett. 11, 1338–1350. doi: 10.1111/j.1461-0248.2008.01249.x
Seminoff, J. A. (2004). Chelonia mydas. The IUCN Red List of Threatened Species 2004:e.T4615A11037468.
Sequeira, A. M. M., Rodríguez, J. P., Eguíluz, V. M., Harcourt, R., Hindell, M., Sims, D. W., et al. (2018). Convergence of marine megafauna movement patterns in coastal and open oceans. Proc. Natl. Acad. Sci. 115, 3072–3077. doi: 10.1073/pnas.1716137115
Shaffer, S. A., Tremblay, Y., Weimerskirch, H., Scott, D., Thompson, D. R., Sagar, P. M., et al. (2006). Migratory shearwaters integrate oceanic resources across the Pacific Ocean in an endless summer. Proc. Natl. Acad. Sci. 103, 12799–12802. doi: 10.1073/pnas.0603715103
Silvano, A., Rintoul, S. R., Peña-Molino, B., Hobbs, W. R., van Wijk, E., Aoki, S., et al. (2018). Freshening by glacial meltwater enhances melting of ice shelves and reduces formation of Antarctic Bottom Water. Sci. Adv. 4:eaap9467. doi: 10.1126/sciadv.aap9467
Simpfendorfer, C. A., Olsen, E. M., Heupel, M. R., and Moland, E. (2012). Three-dimensional kernel utilization distributions improve estimates of space use in aquatic animals. Canad. J. Fish. Aquat. Sci. 69, 565–572. doi: 10.1139/f2011-179
Sims, D. W., Southall, E. J., Humphries, N. E., Hays, G. C., Bradshaw, C. J., Pitchford, J. W., et al. (2008). Scaling laws of marine predator search behaviour. Nature 451, 1098–1103. doi: 10.1038/nature06518
Sims, D. W., Witt, M. J., Richardson, A. J., Southall, E. J., and Metcalfe, J. D. (2006). Encounter success of free-ranging marine predator movements across a dynamic prey landscape. Proc. R. Soc. B Biol. Sci. 273, 1195–1201. doi: 10.1098/rspb.2005.3444
Sousa, L., Queiroz, N., Mucientes, G., Humphries, N., and Sims, D. (2016). Environmental influence on the seasonal movements of satellite-tracked ocean sunfish Mola mola in the north-east Atlantic. Anim. Biotelemetry 4:2. doi: 10.1186/s40317-016-0099-2
Straneo, F., Hamilton, G. S., Sutherland, D. A., Stearns, L. A., Davidson, F., Hammill, M. O., et al (2010). Rapid circulation of warm subtropical waters in a major glacial fjord in East Greenland. Nat. Geosci. 3, 182–186. doi: 10.1038/ngeo764
Strøm, J. F., Thorstad, E. B., Hedger, R. D., and Rikardsen, A. H. (2018). Revealing the full ocean migration of individual Atlantic salmon. Anim. Biotelemetry 6:2. doi: 10.1186/s40317-018-0148-0
Sutherland, D. A., Straneo, F., Stenson, G. B., Davidson, F. J. M., Hammill, M. O., and Rosing-Asvid, A. (2013). Atlantic water variability on the SE Greenland continental shelf and its relationship to SST and bathymetry. J. Geophys. Res. Oceans 118, 847–855. doi: 10.1029/2012JC008354
Teo, S. L., Boustany, A., Blackwell, S., Walli, A., Weng, K. C., and Block, B. A. (2004). Validation of geolocation estimates based on light level and sea surface temperature from electronic tags. Mar. Ecol. Prog. Ser. 283, 81–98. doi: 10.3354/meps283081
Thums, M., Fernández-Gracia, J., Sequeira, A. M. M., Eguíluz, V. M., Duarte, C. M., and Meekan, M. G. (2018). How big data fast tracked human mobility research and the lessons for animal movement ecology. Front. Mar. Sci. 2018:21. doi: 10.3389/fmars.2018.00021
Treasure, A. M., Roquet, F., Ansorge, I. J., Bester, M. N., Boehme, L., Bornemann, H., et al. (2017). Marine mammals exploring the oceans pole to pole a review of the MEOP consortium. Oceanography 30, 132–138. doi: 10.5670/oceanog.2017.234
Tverberg, V., Nøst, O. A., Lydersen, C., and Kovacs, K. M. (2014). Winter sea ice melting in the Atlantic Water subduction area, Svalbard Norway. J. Geophys. Res. Oceans 119, 5945–5967. doi: 10.1002/2014JC010013
Udyawer, V., Dwyer, R., Hoenner, X., Babcock, R., Brodie, S., Campbell, H., et al. (2018). Movement ecology in an era of big data: a framework for standardising analysis of animal telemetry data. Anim. Biotelemetry. 6:17. doi: 10.1186/s40317-018-0162-2
Udyawer, V., Simpfendorfer, C., and Heupel, M. (2015). Diel patterns in the three-dimensional use of space by sea snakes. Anim. Biotelemetry 3:6. doi: 10.1186/s40317-015-0063-6
Van de Merwe, J., Ibrahim, K., Lee, S. Y., and Whittier, J. M. (2009). Habitat use by green turtles (Chelonia mydas) nesting in Peninsular Malaysia: local and regional conservation implications. Wildlife Res. 36, 637–645. doi: 10.1071/WR09099
Vandenabeele, S. P., Shepard, E. L., Grogan, A., and Wilson, R. P. (2012). When three per cent may not be three per cent; device-equipped seabirds experience variable flight constraints. Mar. Biol. 159, 1–14. doi: 10.1007/s00227-011-1784-6
Vicsek, T., and Zafeiris, A. (2012). Collective motion. Phys. Rep. Rev. Sec. Phys. Lett. 517, 71–140. doi: 10.1016/j.physrep.2012.03.004
Whitlock, R. E., Hazen, E. L., Walli, A., Farwell, C., Bograd, S. J., Foley, D. G., et al. (2015). Direct quantification of energy intake in an apex marine predator suggests physiology is a key driver of migrations. Sci. Adv. 1:e1400270. doi: 10.1126/sciadv.1400270
Whoriskey, K., Auger-Methé, M., Albertsen, C. M., Whoriskey, F. G., Binder, T. R., Krueger, C. C., et al. (2017). A hidden Markov movement model for rapidly identifying behavioral states from animal tracks. Ecol. Evol. 7, 2112–2121. doi: 10.1002/ece3.2795
Wikelski, M., and Kays, R. (2010). Movebank: Archive, Analysis and Sharing of Animal Movement Data. Available online at: https://www.movebank.org/
Williams, G. D., Herraiz-Borreguero, L., Roquet, F., Tamura, T., Ohshima, K. I., Fukamachi, Y., et al. (2016). The suppression of Antarctic bottom water formation by melting ice shelves in Prydz Bay. Nat. Commun. 7:12577doi: 10.1038/ncomms12577
Williams, G. D., Hindell, M., Houssais, M.-N., Tamura, T., and Field, I. C. (2011). Upper ocean stratification and sea ice growth rates during the summer-fall transition, as revealed by Elephant seal foraging in the Adelie Depression, East Antarctica. Ocean Sci. 7, 185–202. doi: 10.5194/os-7-185-2011
World Economic Forum (2017). Harnessing the Fourth Industrial Revolution for Oceans. 25. Available online at: http://www3.weforum.org/docs/WEF_Harnessing_4IR_Oceans.pdf
Xavier, J. C., Cherel, Y., Ceia, F. R., Queirós, J. P., Guimarães, B., Rosa, R., et al. (2018). Eastern rockhopper penguins Eudyptes filholi as biological samplers of juvenile and sub-adult cephalopods around Campbell Island, New Zealand. Polar Biol. 41, 1937–1949. doi: 10.1007/s00300-018-2333-2
Xu, Z., Gao, G., Xu, J., and Shi, M. (2017). The evolution of water property in the Mackenzie Bay polynya during Antarctic winter. J. Ocean Univ. China 16, 766–774. doi: 10.1007/s11802-017-3286-8
Yoda, K., Shiomi, K., and Sato, K. (2014). Foraging spots of streaked shearwaters in relation to ocean surface currents as identified using their drift movements. Prog. Oceanogr. 122, 54–64. doi: 10.1016/j.pocean.2013.12.002
Yonehara, Y., Goto, Y., Yoda, K., Watanuki, Y., Young, L. C., Weimerskirch, H., et al. (2016). Flight paths of seabirds soaring over the ocean surface enable measurement of fine-scale wind speed and direction. Proc. Natl. Acad. Sci. 113, 9039–9044. doi: 10.1073/pnas.1523853113
Keywords: ocean observing, animal telemetry, animal movement, movement analysis, EOV
Citation: Harcourt R, Sequeira AMM, Zhang X, Roquet F, Komatsu K, Heupel M, McMahon C, Whoriskey F, Meekan M, Carroll G, Brodie S, Simpfendorfer C, Hindell M, Jonsen I, Costa DP, Block B, Muelbert M, Woodward B, Weise M, Aarestrup K, Biuw M, Boehme L, Bograd SJ, Cazau D, Charrassin J-B, Cooke SJ, Cowley P, de Bruyn PJN, Jeanniard du Dot T, Duarte C, Eguíluz VM, Ferreira LC, Fernández-Gracia J, Goetz K, Goto Y, Guinet C, Hammill M, Hays GC, Hazen EL, Hückstädt LA, Huveneers C, Iverson S, Jaaman SA, Kittiwattanawong K, Kovacs KM, Lydersen C, Moltmann T, Naruoka M, Phillips L, Picard B, Queiroz N, Reverdin G, Sato K, Sims DW, Thorstad EB, Thums M, Treasure AM, Trites AW, Williams GD, Yonehara Y and Fedak MA (2019) Animal-Borne Telemetry: An Integral Component of the Ocean Observing Toolkit. Front. Mar. Sci. 6:326. doi: 10.3389/fmars.2019.00326
Received: 15 November 2018; Accepted: 28 May 2019;
Published: 26 June 2019.
Edited by:
Laura Lorenzoni, University of South Florida, United StatesReviewed by:
Andreas Fahlman, Fundación Oceanogràfic de la Comunitat Valenciana, SpainRachael Orben, Hatfield Marine Science Center, Oregon State University, United States
Copyright © 2019 Harcourt, Sequeira, Zhang, Roquet, Komatsu, Heupel, McMahon, Whoriskey, Meekan, Carroll, Brodie, Simpfendorfer, Hindell, Jonsen, Costa, Block, Muelbert, Woodward, Weise, Aarestrup, Biuw, Boehme, Bograd, Cazau, Charrassin, Cooke, Cowley, de Bruyn, Jeanniard du Dot, Duarte, Eguíluz, Ferreira, Fernández-Gracia, Goetz, Goto, Guinet, Hammill, Hays, Hazen, Hückstädt, Huveneers, Iverson, Jaaman, Kittiwattanawong, Kovacs, Lydersen, Moltmann, Naruoka, Phillips, Picard, Queiroz, Reverdin, Sato, Sims, Thorstad, Thums, Treasure, Trites, Williams, Yonehara and Fedak. This is an open-access article distributed under the terms of the Creative Commons Attribution License (CC BY). The use, distribution or reproduction in other forums is permitted, provided the original author(s) and the copyright owner(s) are credited and that the original publication in this journal is cited, in accordance with accepted academic practice. No use, distribution or reproduction is permitted which does not comply with these terms.
*Correspondence: Rob Harcourt, cm9iZXJ0LmhhcmNvdXJ0QG1xLmVkdS5hdQ==