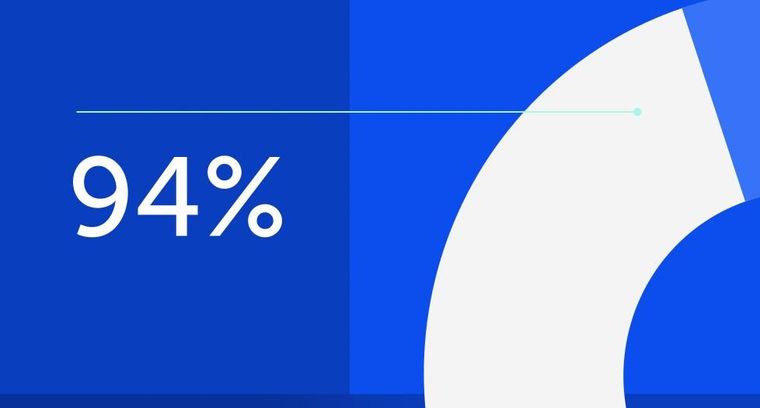
94% of researchers rate our articles as excellent or good
Learn more about the work of our research integrity team to safeguard the quality of each article we publish.
Find out more
SYSTEMATIC REVIEW article
Front. Mar. Sci., 04 July 2019
Sec. Marine Ecosystem Ecology
Volume 6 - 2019 | https://doi.org/10.3389/fmars.2019.00317
This article is part of the Research TopicOceanobs'19: An Ocean of OpportunityView all 136 articles
In coastal waters around the world, the dominant primary producers are benthic macrophytes, including seagrasses and macroalgae, that provide habitat structure and food for diverse and abundant biological communities and drive ecosystem processes. Seagrass meadows and macroalgal forests play key roles for coastal societies, contributing to fishery yields, storm protection, biogeochemical cycling and storage, and important cultural values. These socio-economically valuable services are threatened worldwide by human activities, with substantial areas of seagrass and macroalgal forests lost over the last half-century. Tracking the status and trends in marine macrophyte cover and quality is an emerging priority for ocean and coastal management, but doing so has been challenged by limited coordination across the numerous efforts to monitor macrophytes, which vary widely in goals, methodologies, scales, capacity, governance approaches, and data availability. Here, we present a consensus assessment and recommendations on the current state of and opportunities for advancing global marine macrophyte observations, integrating contributions from a community of researchers with broad geographic and disciplinary expertise. With the increasing scale of human impacts, the time is ripe to harmonize marine macrophyte observations by building on existing networks and identifying a core set of common metrics and approaches in sampling design, field measurements, governance, capacity building, and data management. We recommend a tiered observation system, with improvement of remote sensing and remote underwater imaging to expand capacity to capture broad-scale extent at intervals of several years, coordinated with stratified in situ sampling annually to characterize the key variables of cover and taxonomic or functional group composition, and to provide ground-truth. A robust networked system of macrophyte observations will be facilitated by establishing best practices, including standard protocols, documentation, and sharing of resources at all stages of workflow, and secure archiving of open-access data. Because such a network is necessarily distributed, sustaining it depends on close engagement of local stakeholders and focusing on building and long-term maintenance of local capacity, particularly in the developing world. Realizing these recommendations will produce more effective, efficient, and responsive observing, a more accurate global picture of change in vegetated coastal systems, and stronger international capacity for sustaining observations.
Seagrasses and macroalgae (macrophytes) are the foundation of submerged vegetated ecosystems in shallow coastal waters throughout the world. They are among the most productive habitats on land or sea, provide critical habitat for a diverse range of animals, including commercial, and subsistence fisheries and species of concern (Heck et al., 2003; Hamilton and Konar, 2007; Hughes et al., 2009; Unsworth R. et al., 2018; Lefcheck et al., 2019), and provide coastal protection, uptake of terrestrial nutrient runoff, and carbon storage. These habitats and the services they provide are threatened by a range of interacting human activities, notably coastal development, declining water quality, invasive species, climate warming, sea level rise, and storms (Carpenter et al., 2008; Waycott et al., 2009; Polidoro et al., 2010; Filbee-Dexter and Wernberg, 2018). Large, perennial organisms such as seagrasses and canopy-forming macroalgae (Laminariales, Tilopteridales, Desmarestiales, and Fucales, commonly known as kelp and fucoids or rockweeds) are especially vulnerable to human disturbance and, under repeated and interacting impacts, they often yield dominance to faster-growing opportunistic algae (Duarte, 1995; Bonsdorff et al., 1997; Filbee-Dexter and Wernberg, 2018). Growing understanding of the valuable services provided by seagrass and macroalgal stands has strengthened interest in conserving them. Originally, these concerns were based primarily on contributions of coastal vegetated ecosystems to commercial fisheries but have expanded to include their importance to biodiversity and threatened species, artisanal fisheries (Nordlund et al., 2018), good water quality, resilience against climate change, and cultural significance (Macreadie et al., 2017; Wernberg et al., 2019).
As a result of their ecological value and vulnerability, protection of coastal macrophyte habitats is mandated by many national and international conventions and policies, including the international Ramsar Convention on Wetlands of International Importance and Convention on Biological Diversity (Miloslavich et al., 2018) as well as the USEPA 2003 Clean Water Act, the European Union 1992 Habitat Directive, the 2000 Water Framework Directive, and the 2008 Marine Strategy Framework Directive. Effective resource management requires understanding the extent, conditions, and trends in the marine ecosystems that support them. Moreover, because drivers of change interact, ecosystem-level responses to environmental forcing can be complex, emphasizing the need for tracking both environmental conditions and key biological components of these systems (Duffy et al., 2013a). For example, long-term field monitoring and experiments in the Baltic Sea suggest that seagrass decline resulted from combined nutrient loading and the cascading effects of fishing (Baden et al., 2010; Eriksson et al., 2011; Östman et al., 2016). The interdependency of coastal habitat quality and offshore fisheries in this region would not have been recognized without long-term monitoring. Such interactions also highlight the importance of connecting monitoring to ecological theory, experiments, and modeling to evaluate mechanisms and the generality of system dynamics (Duffy et al., 2013b). For example, both shallow rooted macrophytes (Short and Burdick, 1996; Greening et al., 2018) and macroalgal forests (Benedetti-Cecchi et al., 2015; Filbee-Dexter and Wernberg, 2018) can shift rapidly to unvegetated or alternate vegetation states, with important management implications. Experiments and theory help predict the warning signs and mechanisms of such transitions. Knowledge derived from both monitoring macrophytes and mechanistic research is critical to informing policy and helping to design and implement effective management actions. Similarly, macrophyte species differ widely in morphology, but these traits often covary predictably with physiology (Duarte, 1991). Forecasting the responses of macrophyte communities to perturbations can be strengthened by incorporating mechanistic trait-based approaches, as has proven successful in terrestrial plants (Wright et al., 2004) and corals (Darling et al., 2012; Madin et al., 2014).
More than 45 programs worldwide conduct repeated observations of submerged vegetation at regional to global scales (Table S1), providing key indicators of marine ecosystem change (Marbà et al., 2013; Krumhansl et al., 2016; Miloslavich et al., 2018). Most programs operate in isolation and are restricted in space and duration (Krumhansl et al., 2016). This constrains our perception of status, trends, and dynamics of macrophyte ecosystems on the scales necessary for informing effective management and policy, particularly on national and international scales. The Global Ocean Observing System (GOOS), launched in 2009, oversaw a community process to identify a set of core physical, biogeochemical, and more recently biological “Essential Ocean Variables” (EOVs), aimed at providing data to inform requirements for international reporting and assessments (Lindstrom et al., 2012). Seagrass and macroalgal canopy cover and composition were identified as two of the seven biological EOVs based on their scientific and societal relevance and feasibility for global implementation (Miloslavich et al., 2018). However, coordinated observations of these coastal macrophytes have lagged behind those of pelagic phytoplankton and coral reefs. GOOS is collaborating with the Partnership for the Observation of the Global Ocean (POGO), the Marine Biodiversity Observation Network (MBON) (Muller-Karger et al., 2018), and other parties to draft plans for long-term, large-scale implementation of the EOVs. The immediate goal is recommendations for consolidating existing data and metadata toward a globally coherent system under the FAIR (findable, accessible, interoperable, and reusable) data principle (Wilkinson et al., 2016).
This white paper presents a consensus assessment and recommendations for advancing observation of marine macrophytes, integrating contributions from researchers with broad geographic and disciplinary expertise. We carried out an in-depth analysis of the current knowledge of seagrass and macroalgae monitoring efforts worldwide. Based on this review, we summarize the status of marine macrophyte habitats, focusing on seagrasses, macroalgal forests, and pelagic Sargassum; the services they provide to humanity; and the threats facing them. We then outline the rationale for considering macrophyte abundance and composition as Essential Ocean Variables as well as steps toward more effectively incorporating them into global observing systems that inform policy and management needs. We close with recommendations for establishing a coordinated global observing system for marine macrophytes.
Seagrasses are a group of 72 species of flowering plants that spend all or most of their lives submerged in seawater (Short et al., 2011). Most seagrasses root in shallow sediment bottoms (exceptions include the rocky shore surfgrasses, Phyllospadix spp., as well genera like Amphibolis, Thalassodendron, Cymodocea, and Posidonia, which occur on rocky bottoms under some conditions). Seagrass depth limits are set by sufficient light to support net positive growth—generally <20 m depth, but deeper in oceanic waters (e.g., >30 m in the Canary Islands) and much shallower in turbid estuaries. Seagrasses form dense populations in estuarine and protected coastal waters from the equator to high latitudes on all continents except Antarctica, and are most diverse in southeast Asia and Western Australia (Lamit et al., 2017). Six seagrass bioregions have been recognized (Figure 1), encompassing all the oceans of the world, across both tropical and temperate waters (Short et al., 2007). The seagrass Atlas (Green and Short, 2003) was able to identify and confirm only around 150,000 km2 of seagrass meadows globally. But the global area of habitat suitable for seagrasses has recently been estimated at around 1.6 million km2 based on environmental models (Jayathilake and Costello, 2018), and the total coastal area with sufficient light for seagrass growth is estimated at 4.32 million km2 (Gattuso et al., 2006; Duarte, 2017), not taking into account other habitat requirements such as suitable substrate conditions. Modeled estimates of seagrass potential extent can cover much larger areas than in situ measurements but have high uncertainty, particularly on local scales, as they are based on environmental conditions considered suitable rather than on direct evidence of seagrass presence.
Figure 1. Locations of current long-term seagrass observing networks and seagrass geographic bioregions. (1) Temperate North Atlantic, (2) Tropical Atlantic, (3) Mediterranean, (4) Temperate North Pacific, (5) Tropical Indo-Pacific, (6) Temperate Southern Oceans. Observing systems are shown as 2 degree grids.
Seagrasses support biotic communities that are often considerably more diverse and productive than in surrounding unvegetated sediments as a result of the physical structure of seagrass meadows and the high productivity of associated algae (Orth and Van Montfrans, 1984; Duffy et al., 2013b). Seagrass meadows are especially important as nursery habitats for juvenile life stages of fishes and larger invertebrates (Beck et al., 2001; Heck et al., 2003; Lefcheck et al., 2019) and provide feeding and breeding habitats for several threatened species, including sea turtles and sirenians (dugongs and manatees). However, much seagrass production is ungrazed and flows into detritus food webs or is buried in sediments, making seagrass meadows important sites of blue carbon burial (Fourqurean et al., 2012; Duarte et al., 2013). Exported seagrass material may also contribute to carbon sequestration in deeper oceanic sinks distant from seagrass habitats (Duarte and Krause-Jensen, 2017).
A host of global and local stressors affect seagrasses, including sediment and nutrient runoff, physical disturbance, algal blooms, invasive species, climate warming, and disease (Orth et al., 2006; Waycott et al., 2009). The sheltered coastal and island waters in which seagrasses grow best are prime real estate for coastal and harbor development, imposing pressures from land reclamation, deforestation, aquaculture, fishing, and marine debris (Unsworth R. et al., 2018). A principal local stressor throughout the world is poor water quality resulting from nutrient pollution (eutrophication) and/or sediment loading from land runoff (Cloern, 2001; Burkholder et al., 2007). Requirements for clear water and low nutrient concentrations make seagrasses vulnerable to eutrophication, as nutrient and sediment loading reduce light availability and favor faster-growing algae (Burkholder et al., 2007). Recently, massive influxes of pelagic Sargassum spp. have caused loss of near-coastal seagrass meadows in the Caribbean (van Tussenbroek et al., 2017), and invasive macroalgae can threaten both seagrasses and canopy macroalgae elsewhere as well (e.g., Lophocladia lallemandii in the Mediterranean Sea, Ballesteros et al., 2007a). Disruption of coastal food webs can also threaten seagrass ecosystems, both by altering grazing by megaherbivores and through cascading effects of overfishing (Eriksson et al., 2011) and hunting (Hughes et al., 2013). Climate change is a growing concern for seagrass ecosystems (Waycott et al., 2011; Short et al., 2016; Fortes et al., 2018). Many temperate seagrasses are sensitive to high temperatures, and warming-induced mortality has been observed over recent decades in several regions (Short and Neckles, 1999; Reusch et al., 2005; Moore and Jarvis, 2008; Hammer et al., 2018). While warming can be particularly detrimental near the equatorial end of the distribution range (e.g., major declines of Zostera marina at its southern range in SW Iberia; Cunha et al., 2013), seagrasses and macroalgae may instead benefit from warming at the polar end of the distribution range (Mieszkowska et al., 2006, 2014; Kortsch et al., 2012; Krause-Jensen et al., 2012). Shallow seagrasses are also vulnerable to die-off under hypersaline conditions (> 45 psu) resulting from combined low precipitation and elevated water temperatures (Walker and McComb, 1990; Koch et al., 2007; Johnson et al., 2018). Large-scale (> 50 km2) seagrass die-off associated with hypersaline conditions is a recurring problem in Florida Bay in the USA, in the French Mediterranean Sea, and southern Australia (Robblee et al., 1991; Seddon et al., 2000; Greve et al., 2003), and is expected to become more frequent and widespread under future warming. Another serious concern is the frequency of disease epidemics, which are associated with warming in many systems (Harvell et al., 2002; Altizer et al., 2013; Kaldy, 2014; Sullivan et al., 2017).
In part as a result of these multifarious stressors, ten of the 72 currently known seagrass species are at elevated risk of extinction and three species are classified as Endangered (Short et al., 2011). In the Caribbean Sea, the CARICOMP regional monitoring study detected long-term declines in the relative abundance of the robust, slow-growing seagrass Thalassia testudinum in 43% of 35 long-term monitoring stations consistent with environmental deterioration (van Tussenbroek et al., 2014). In the Mediterranean, dramatic losses in cover of the seagrasses Posidonia and Cymodocea have been predicted (Chefaoui et al., 2018) and observed, and many local extinctions were reported in SW Iberia (Cunha et al., 2013). Many tropical Pacific seagrasses are also declining, mainly in populated areas, due to increased nutrient loading and sedimentation, the two most common stressors of seagrasses worldwide (Short et al., 2014). It is likely that ongoing environmental change will similarly lead to seagrass declines in other locations including the diverse tropical regions where robust environmental protection is often poorly implemented. The wide uncertainty in estimates of global seagrass area, and particularly the geographic bias in our knowledge, makes estimates of threats and projected losses speculative, but there is widespread concern about losing seagrass meadows that have not yet been identified or assessed. This makes establishing a globally harmonized approach for monitoring these coastal ecosystems all the more important and urgent.
A variety of large macroalgae live in dense populations that can be described as marine forests (Wernberg and Filbee-Dexter, 2019). Among the most prominent and widely distributed are large brown algae (Ochrophyta) known as kelps and rockweeds (orders Laminariales, Tilopteridales, Desmarestiales, and Fucales), the largest being the giant kelp Macrocystis pyrifera, which can reach >45 m in length. Large green (Chlorophyta) and red (Rhodophyta) macroalgae can also form marine forests. Macroalgal forests vary greatly in height, structure, and function and, like seagrasses, provide three-dimensional landscape structure that generally supports dense communities of other algae, invertebrates, fishes, and some marine mammals—most of which could not persist in the absence of the canopy (Tegner and Dayton, 2000; Krumhansl and Scheibling, 2012; Teagle et al., 2017).
Macroalgal forests are confined to hard substrata, typically rocky reefs, and are generally found from the intertidal zone to 15–25 meters in depth, although the lower limit can exceed 50–100 m in clear water (Graham et al., 2007; Marzinelli et al., 2015b; Assis et al., 2016, 2018). Tidal height, water clarity, wave exposure, and herbivory, particularly by sea urchins, often limit their spatial distribution. Macroalgal forests are particularly prominent along temperate and polar latitudes (Steneck and Johnson, 2013; Wernberg et al., 2019), but laminarian kelp forests occur near the equator in clear, nutrient-rich water below the thermocline (>30 meters) (Graham et al., 2007), and extensive Sargassum forests are common in many shallow tropical and subtropical environments (Fulton et al., 2014). Macroalgal forests dominate at least 25% of the world's coastlines (Steneck and Johnson, 2013; Wernberg et al., 2019).
Macroalgal forests are impacted by a variety of stressors, including sediment and nutrient loading (Foster and Schiel, 2010); direct harvesting (Vásquez et al., 2014); fishing (Ling et al., 2009); climate change in the form of rising water temperatures, ocean acidification, extreme weather events, melting glaciers (Mieszkowska et al., 2006; Wernberg et al., 2012; Araujo et al., 2016); pollution by heavy metals and organic chemicals (Thibaut et al., 2005; Coleman et al., 2008; Fowles et al., 2018); harvesting for food and the phycocolloid industry (Rebours et al., 2014; Buschmann et al., 2017); aquaculture (Yang et al., 2015); and disease (Altizer et al., 2013; Marzinelli et al., 2015a). Climate warming has directly affected the abundance, distribution, and geographic range of many macroalgal species (Johnson et al., 2011; Nicastro et al., 2013; Assis et al., 2014; Brodie et al., 2014; Neiva et al., 2015; Filbee-Dexter et al., 2016; Lourenco et al., 2016; Wernberg et al., 2016; Martinez et al., 2018). Near the poles, climate warming is likely to stimulate the expansion of macroalgal forests (Krause-Jensen and Duarte, 2014). Disturbances to food webs have also threatened macroalgal forests. Overfishing has led indirectly to loss of canopy-forming macroalgae by releasing sea urchins from predatory control and allowing them to overgraze kelps (Wilmers et al., 2012; Filbee-Dexter and Scheibling, 2014; Konar et al., 2014; Ling et al., 2015; Estes et al., 2016). Such trophic cascades are expected to increase in the future. Other herbivores can similarly overgraze macroalgal forests (Chenelot and Konar, 2007; Gianni et al., 2017). Over the last decade or so, tropical herbivores have expanded into temperate regions, increasing the abundance, diversity, and feeding pressure of herbivores and in some cases eradicating macroalgal forests (Johnson et al., 2013; Vergés et al., 2014; Araujo et al., 2016).
As a result of these multiple stressors, macroalgal communities are declining worldwide, particularly laminarian kelp forests. Among macroalgal time series extending >20 years, 61% are in decline and only 5% are increasing (Krumhansl et al., 2016; Wernberg et al., 2019). Losses of canopy-forming macroalgae are predicted to increase with rising global temperatures and more frequent and severe weather events (Oliver et al., 2018). Unfortunately, 66% of the bioregions with laminarian kelp forests have no time series data (Mieszkowska et al., 2006; Krumhansl et al., 2016). In many regions, kelps and other canopy-forming macroalgae have been in transition to dominance by turf and coralline algae over the past two decades (Benedetti-Cecchi et al., 2001; Filbee-Dexter and Scheibling, 2014; Strain et al., 2014; Filbee-Dexter and Wernberg, 2018). Community states dominated by turf or coralline algae are often maintained by multiple, complex feedbacks, suppressing their return to dominance by canopy-forming macroalgae (Benedetti-Cecchi et al., 2015; O'Brien et al., 2015; Rindi et al., 2017; Filbee-Dexter and Wernberg, 2018). This emphasizes the need to understand ecological processes as well as abundance trends.
Large macroalgae also occur in the open pelagic regions of the Atlantic Ocean, Gulf of Mexico, and Caribbean Sea, where extensive accumulations of two pelagic brown seaweeds, Sargassum fluitans and S. natans, occur with a diversity of morphological forms (Schell et al., 2015). Both species are holopelagic, reproduce by vegetative fragmentation (Huffard et al., 2014) and form “Lagrangian ecosystems” that drift with winds and currents. In addition to these naturally pelagic macroalgae, floating rafts of normally benthic algae have increased over recent years in several regions. In the East China Sea, floating seaweed rafts are formed by S. horneri, which grows on the bottom but can be detached by strong waves (Qi et al., 2017). Aggregations of detached S. horneri have recurred since 2008, along with blooms of the green macroalga Ulva prolifera in the Yellow Sea. Strategies have been developed to monitor these algal blooms that can also be applied to Sargassum blooms (Hu et al., 2017). Kelps can also separate from the substrate and drift long distances, transporting biomass and associated animals on their way through the ocean (Fraser et al., 2018).
Pelagic Sargassum occurs over vast areas of open ocean but is patchy and ephemeral. Its distribution is largely controlled by physical processes, notably ocean circulation and wind (Brooks et al., 2018). Sargassum accumulates in areas of convergence, similar to debris and other pollutants (Powers et al., 2013). Monitoring of pelagic Sargassum is constrained by lack of basic information on its life history, demography, and responses to environmental conditions. The broad distribution and drifting of Sargassum also presents challenges for monitoring. Since 2011, large rafts of Sargassum have entered the Caribbean Sea, washing ashore in massive amounts and affecting navigation and the economies of the island nations in the region, which are largely driven by tourism and, to a lesser extent, fishing. Sargassum accumulation and pollution often co-occur, threatening the endemic and other species that rely on Sargassum (Witherington et al., 2012; Powers et al., 2013). The ecological implications of these accumulations are poorly studied (van Tussenbroek et al., 2017; Gavio and Santos-Martinez, 2018). In the short term, Sargassum can suffocate coastal fauna by depleting dissolved oxygen as the algal biomass decomposes, stress coral reefs by shading their photosynthetic symbionts, and render sea turtle nesting beaches unusable. But moderate influx of biomass and marine-derived nutrients may enhance the growth of dune vegetation and stabilize coastlines. Understanding such ecological processes is necessary for informed management decisions.
Ecosystem services are benefits that humans receive from the Earth's natural systems and include provisioning, regulating, cultural, and supporting processes (Costanza et al., 1997; Rapport et al., 1998; MEA, 2005). In coastal ecosystems, marine macrophytes provide services including fisheries support, nutrient cycling, coastal protection, water purification, provision of raw materials, and carbon storage that can counteract climate change (Geider et al., 2001; Boström et al., 2003; UNEP, 2006; Bos et al., 2007; Cullen-Unsworth and Unsworth, 2013; Vassallo et al., 2013; Campagne et al., 2015; Dewsbury et al., 2016; Nordlund et al., 2016; Lamb et al., 2017; Filbee-Dexter and Wernberg, 2018). Because seagrasses are sensitive to nutrient loading and water transparency, they also serve as valuable indicators of the state of coastal ecosystems (Dennison, 1987). Seagrasses contribute to water quality by reducing pathogens that cause disease in corals and humans (Lamb et al., 2017) and by taking up nutrient runoff from land. They protect coastal lives and property by stabilizing coastal sediments via both their below-ground rhizome structure and leaf canopies (Cruz-Palacios and Van Tussenbroek, 2005; Bos et al., 2007). Seagrass ecosystems are also increasingly recognized as protecting underwater cultural heritage (Krause-Jensen et al., 2018).
Similarly, macroalgal forests provide a range of ecosystem services (Vásquez et al., 2014; Bennett et al., 2016; Macreadie et al., 2017; Blamey and Bolton, 2018), including direct support of commercial, recreational, and subsistence fisheries and aquaculture. Indirect ecosystem services include erosion control, climate change mitigation and adaptation, and biogeochemical cycling of nitrogen, carbon, and phosphorus. Intrinsic ecosystem services include cultural and religious significance, biodiversity, and scientific value. Cultural services provided by macrophytes remain understudied (Ruiz-Frau et al., 2017) but include tourism, aesthetic values, and some traditional ways of life that are intricately associated with these ecosystems for food, recreation, and spiritual fulfillment (Wyllie-Echeverria et al., 2002; de la Torre-Castro and Rönnbäck, 2004; Cullen-Unsworth and Unsworth, 2013; Filbee-Dexter and Wernberg, 2018). Few studies have valued macroalgal ecosystem services economically (Bennett et al., 2016), but laminarian kelp forests have been estimated to contribute around 1 million USD per kilometer of coastline (Filbee-Dexter and Wernberg, 2018). The true value is likely to be orders of magnitude higher if indirect and non-use values are fully considered (Bennett et al., 2016).
Marine macrophytes provide critical habitat that supports fisheries' productivity and food security across the world, especially in developing regions (Beck et al., 2001; Green and Short, 2003; Orth et al., 2006; Brun et al., 2009; Unsworth et al., 2014; Nordlund et al., 2016, 2018; Unsworth R. et al., 2018). In the Mediterranean, for example, seagrass covers <2% of the seafloor but seagrass-associated fishes and invertebrates comprise 30–40% of the total value of commercial fisheries landings (Jackson et al., 2015). Shallow seagrass meadows are easily exploitable and support subsistence, commercial, or recreational fishing in many regions, ranging from hand-gleaning to commercial trawling and targeting multiple fish and invertebrate species (Nordlund et al., 2018). Kelp forests similarly serve as nurseries for fished species in some regions (Hamilton and Konar, 2007). Some kelp ecosystems have been overfished (Tegner and Dayton, 2000; Bertocci et al., 2015), but knowledge of these fisheries is geographically limited. Other marine macrophytes are also important for fisheries (Kritzer et al., 2016), including tropical seaweed beds (Tano et al., 2016, 2017). The increasing human populations and standards of living in developing nations put a premium on maximizing the productivity and sustainability of fisheries generally and on conserving the marine macrophyte habitats that support them (Unsworth R. et al., 2018). There is a strong need to include the value of seagrass and macroalgal habitats in spatial planning and management (Nordlund et al., 2018; Unsworth R. et al., 2018). Finally, pelagic Sargassum also has been designated an “essential fish habitat” (NOAA, 1996), as it provides shelter and food for early life-stages of pelagic fishes (Wells and Rooker, 2004) and may play an important role in the recruitment dynamics of economically important species (Kingsford and Choat, 1985).
Marine ecosystems play key roles in mitigating rising atmospheric CO2 by sequestering and storing carbon in coastal plant biomass and sediments—known as “blue carbon.” Seagrass meadows, tidal marshes, and mangrove forests are key blue carbon habitats, occupying just 1% of the seafloor but storing over half the ocean's carbon (Duarte et al., 2013), sequestering much more carbon on a per-area basis than terrestrial vegetated ecosystems (Mcleod et al., 2011; Röhr et al., 2018). But the magnitude of this carbon storage is highly variable (Nelleman, 2009; Kennedy et al., 2010; Mcleod et al., 2011; Kindeberg et al., 2018; Röhr et al., 2018). Macroalgae form the most extensive of marine vegetated habitats, but until recently were overlooked as contributors to carbon sequestration because they are mostly confined to rocky substrata that do not support carbon burial in sediments (Howard et al., 2017). However, macroalgal forests can export material to carbon sinks in shelf sediments and in the deep sea where it can be sequestered (Krumhansl and Scheibling, 2012; Hill et al., 2015; Krause-Jensen and Duarte, 2016; Wernberg and Filbee-Dexter, 2018). A first-order estimate indicates that this macroalgal contribution is of the same order of magnitude as carbon sequestration by seagrasses, saltmarshes, and mangroves combined (Krause-Jensen and Duarte, 2016). The contribution of macroalgae to carbon sequestration varies among species (Trevathan-Tackett et al., 2015), but more direct estimates are needed to quantify sequestration, and this requires a paradigm shift in accounting procedures as well as development of methods to trace carbon from donor to sink habitats in the ocean (Krause-Jensen et al., 2018). As vegetated ecosystems have declined substantially in area (Waycott et al., 2009), many coastal areas have been converted from carbon sinks to sources, a shift that can, in principle, be reversed (Pendleton et al., 2012; Macreadie et al., 2015, 2017; Marbà et al., 2015; Kerrylee et al., 2018). One approach to quantifying the processes that mediate carbon storage and release from seagrass sediments is the TeaComposition H2O project, which uses widely available tea bags to measure organic carbon preservation in seagrass and other wetland sediments, currently under way across 350 nearshore marine sites. Similarly, the SUKER Network has recently completed globally distributed litterbag experiments across 40 sites between Alaska and Portugal to measure the fate of sugar kelp detritus.
Anthropogenic nitrogen inputs are a major stressor facing coastal ecosystems worldwide (Duarte et al., 2009). Historically, nitrogen has been a limiting factor for primary production in many coastal regions, but nutrient loading from agricultural runoff and coastal development has greatly increased usable nitrogen availability in coastal systems worldwide. These inputs shift the character of the vegetation and produce far-reaching impacts that ripple through coastal ecosystems (Breitburg et al., 2009). Macrophytes can help mitigate such eutrophication. Seagrass meadows suffer less decline where they are separated from terrestrial runoff by fringing marshes, probably because of denitrification and burial of terrestrial nitrogen within the marshes before it reaches the seagrasses offshore (Valiela and Cole, 2002). Seaweed aquaculture also has been proposed as a way to mitigate eutrophication impacts (Chopin et al., 2001; Neori et al., 2004), and in certain locations on the coast of China, algal cultivation has reduced nutrients and resulting algal blooms, including toxic microalgae (Yang et al., 2014). But algal aquaculture has more often been detrimental, resulting in widespread seagrass loss (Eklöf et al., 2006; Unsworth R. K. et al., 2018).
Macrophyte ecosystems provide food and habitat to a wide range of invertebrates, fishes, and some marine mammals and reptiles, including several listed under CITES [e.g., the dugong, Dugong dugon; the West Indian manatee, Trichechus manatus; and the African manatee, Trichechus senegalensis, all listed in CITES Appendix 1, and the green turtle, Chelonia mydas, classified as Endangered on the IUCN Red List and also on CITES Appendix 1 (Moore et al., 2017; Sievers et al., 2019)]. Most large animals that depend on seagrasses have declined substantially during historical times, and about 30% of named seahorse species, all of which use seagrass habitats, are included on the IUCN Red List (Hughes et al., 2009). Prior to European colonization, large seagrass-feeding vertebrates were extremely abundant in some regions. The Cayman Islands fishery in the Caribbean landed ~13,000 sea turtles each year for decades beginning in the late Seventeenth century (Jackson, 1997), and the number of dugongs along the coast of the Great Barrier Reef Region south of Cairns was much greater than it is today (Marsh et al., 2005). The formerly larger densities of megaherbivores undoubtedly had major impacts on seagrasses (Marsh et al., 2011, 2018; Vonk et al., 2015), but megaherbivore grazing can also increase seagrass productivity (Aragones and Marsh, 2000; Christianen et al., 2011; Marsh et al., 2011, 2018). Live seagrass seeds have been found in megaherbivore feces, indicating the potential for green turtles and dugongs to disperse seeds up to hundreds of kilometers (Tol et al., 2017) and excavating dugongs can also increase microbial nutrient cycling in seagrass sediments (Perry and Dennison, 1999). Megaherbivores associated with seagrasses also support provisioning and cultural ecosystem services, including hunting, fishing, tourism, and cultural values (Butler et al., 2012; Cullen-Unsworth et al., 2014).
Large-scale loss of seagrass results in mortality and reduced fecundity of seagrass-dependent megaherbivores (Marsh et al., 2011; Fuentes et al., 2016). Kelp forests of the northeastern Pacific formerly supported the largest known herbivorous marine mammal, Steller's sea cow (Hydrodamalis gigas). This giant 8–9 m relative of the dugong was hunted to extinction within a few decades of its first encounter with Europeans in 1741 (Marsh et al., 2011), and the sea otter (Enhydra lutris) nearly met a similar fate, having been eliminated from the North Pacific apart from a few remote islands of the Aleutian chain prior to protection in the 1970s. There is some evidence of a symbiotic relationship between sea otters and seagrasses (Hughes et al., 2013).
As is true of marine invertebrates generally, we have very limited knowledge of the conservation status of most macrophyte-associated invertebrates. Several gastropods and echinoderms are popular as curios and traditional medicines, and harvesting may contribute to their population decline or extinction (Hughes et al., 2009).
Pelagic Sargassum serves as nursery habitat for oceanic-stage juvenile sea turtles (Carr, 1987; Witherington et al., 2012). Young turtles likely grow faster in association with pelagic Sargassum owing to foraging on invertebrates in the algae and higher temperatures achieved by basking in surface rafts, and the thick mats may also reduce predation risk (Mansfield and Putman, 2013; Mansfield et al., 2014). In 2014, the US National Marine Fisheries Service designated waters in the U.S. Exclusive Economic Zone with abundant pelagic Sargassum as a “critical habitat” for the loggerhead sea turtle (Caretta caretta) in the Northwest Atlantic Ocean. There is also growing evidence that small sea turtles of several species actively orient toward pelagic Sargassum (Mansfield and Putman, 2013; Putman and Mansfield, 2015). New satellite-based mapping (Figures 2A,C) now makes it possible to integrate Sargassum distribution with models of sea turtle behavior and movement (Putman et al., 2012) to explore its role in turtle population dynamics.
Figure 2. Tracking of pelagic Sargassum density and movement in the Caribbean region. (A) Sargassum area density map (0.5° × 0.5°) in July 2018. The red color indicate pixels where the area density (% cover) exceeds 0.1 units. (B) Trajectories of spatial drifters deployed by NOAA/AOML in the Atlantic. Each color represents a type of drifter. The trajectories of drifters with transmitters attached to real and artificial Sargassum are shown in green. (C) Cumulative weekly AFAI field from VIIRS&MODIS (20 June 2018). The structures in green/red may correspond to Sargassum patches, which extended over large areas in the Caribbean Sea during that period. Clouds are masked to black.
In addition to their role in climate change mitigation through carbon storage, marine macrophytes also contribute to climate change adaptation e.g., by dampening the wave energy and stimulating sedimentation, thereby protecting coastlines against rising water levels and storms (Duarte et al., 2013). Coastal wetlands, including seagrass meadows and some macroalgal forests, form protective barriers that shelter coastal land from erosion and storm surge by attenuating waves and reducing property damage and human deaths. Both living plants and their dead biomass accreted as peat strengthen shorelines and provide a robust barrier that protects coastal land against sea level rise (Gedan et al., 2011), severe storms, and wave action. Analysis of 34 major US hurricanes found that economic damage declined with greater area of wetland in the storm zone, and that wind speed and wetland area together explained 60% of the variation in damage; coastal wetlands were estimated to provide more than 23 billion USD per year in storm protection in the USA (Costanza et al., 2008). And studies in the UK concluded that maintenance of natural marsh is much less expensive than building and maintaining sea walls as protection against erosion (King and Lester, 1995; Rupprecht et al., 2017).
Mapping the risk of storm and wave hazards along the coastline of the USA shows that, under several projected climate scenarios, the number of people and the total value of residential property exposed to hazards could be reduced by half by preserving existing coastal habitats (Arkema et al., 2013). Models predict that climate change will increase wave heights, especially at higher latitudes (Izaguirre et al., 2011). In Northern Europe, the intensity of destructive storms has already increased more than 3-fold since 1990 (Gregow et al., 2017), and monthly mean wave heights have risen up to 0.6 m in the North Atlantic during the latter twentieth century (Woolf et al., 2002). Natural infrastructure provided by coastal habitats holds strong but understudied potential to mitigate such hazards and increase coastal resilience under climate change (Sutton-Grier et al., 2015).
To examine the current status of marine macrophyte observing, we collated information on monitoring programs with more than three repeated observation events over a period >3 years, with a least one observation event in the last decade (2008–2018). This eliminated the large number of localized, short-term monitoring projects. Of the 20 active long-term seagrass observing programs meeting these criteria (Table S1), the largest were the global programs SeagrassNet and Seagrass-Watch. Together these programs include long-term monitoring across five of the six seagrass bioregions (Figure 1), with 21 countries currently participating in SeagrassNet and 11 in Seagrass-Watch. One of the largest regional networks is in the temperate North Atlantic region, where 23 countries monitor seagrass via 11 programs (e.g., COMBINE; PMN) (Marbà et al., 2013) in compliance with the pan-European Water Framework Directive (WFD, 2000/60/EC) (Foden and Brazier, 2007). The WFD stimulated widespread monitoring of macroalgae and seagrasses in Europe by prescribing assessment of ecological status based on biological elements, including aquatic plants. However, while the WFD requires that ecological status is defined relative to a reference, and that status classifications are intercalibrated within ecoregions, member states are free to develop their own indicators, which has resulted in a proliferation of seagrass indicators that are often not comparable (Krause-Jensen et al., 2005; Marbà et al., 2013).
The motivations for existing seagrass observing networks range from broad—increasing general scientific knowledge and tracking the status of seagrass—to more narrow efforts to support conservation and regulatory agreements. The book Global Seagrass Research Methods (Short and Coles, 2001) provides detailed methodology for assessing all aspects of seagrass ecosystems. Two broad approaches are currently used to monitor seagrass ecosystems. The first involves air-borne or satellite remote sensing to map seagrass and estimate broad-scale changes in extent over time. The second approach quantifies indicators of seagrass condition in situ at smaller spatial scales, often to assess the effects of stressors. These approaches have been powerfully combined in a hierarchical framework for monitoring based on spatial extent, frequency of monitoring, and scope (Neckles et al., 2012). The first tier within the hierarchical framework characterizes overall distribution of seagrasses across a region of interest. This approach is widely used to assess status and trends at broad scales, over long time periods, with low observing frequency. Aerial and hyperspectral satellite imagery are well-established techniques at such scales, but such remote technology restricts observations to shallow depths and is often unworkable in the complex multi-habitat seascapes and turbid waters where seagrasses are most abundant (Knudby and Nordlund, 2011). Measures derived from remote sensing are often limited to presence/absence and extent. Rapidly evolving technological advances may offer partial solutions, with improvements in resolution and availability of both satellite sensors and high-quality drone and autonomous or remotely operated sensors (both above and within water) increasing the environmental window of opportunity for observing and quantifying seagrass. Currently, there is no consistent approach or recommended sensor for seagrass globally.
Some observing networks supplement remote sensing with in situ manual sampling of species identity and abundance, which constitute the second and subsequent tiers within a hierarchical monitoring framework. In situ sampling characterizes seagrass condition by selecting statistically rigorous sampling units and monitoring frequency, e.g., SeagrassNet. These measurements are predominantly in situ and done by hand, providing more detailed indicators of spatiotemporal variation in species composition, size, and abundance (e.g., cover, density, biomass). These second-tier measures involve a greater number of sampling units, a higher temporal frequency, and higher resolution than the first tier of remote sensing. Finer-scale tiers generally focus more specifically on evaluating drivers of change at higher frequency, with more metrics, and a smaller number of sites. These in situ methods are far more accurate and detailed than remote sensing but accordingly more labor intensive and thus restricted in scope.
The GOOS has proposed harmonization of monitoring protocols toward development of seagrass cover and composition as an Essential Ocean Variable (Miloslavich et al., 2018). Several collective decisions need to be made to achieve this goal. First, there is currently no widely accepted standard for site selection, and observing networks vary widely in the types, sizes, and replication of sampling and reporting units. Most networks use some version of stratified random sampling, with or without constraints. For example, areas of investigation are often divided using expert knowledge into subpopulations—e.g., by water depth—that maximize variation between and minimize the variation within units. Sampling units are then randomly drawn and may be allocated proportionately to provide robust estimates of variance (Neckles et al., 2012; see also FKMMP, Texas Seagrass Monitoring program). This approach aims to balance reporting area size, level of detail necessary, accessibility, legacy, safety, and budget.
Once the reporting unit or site is established, the second decision to be considered involves the frequency of assessment and sampling design. Current long-term observing networks generally monitor annually, with a few including seasonal assessments. Networks that use a hierarchical framework generally make observations at a lower frequency for the first large-scale tier (e.g., 3–5 years), with annual and seasonal monitoring for the smaller-scale sampling tiers. Some networks (e.g., Seagrass-Watch) implement an adaptive monitoring approach, altering the frequency of monitoring and data collection when rates of change differ from those initially anticipated, or when the focus of investigation changes. The most popular spatial study design generally involves sampling along a transect. This design has advantages over random sampling in being easier, more cost effective, in guaranteeing that measurements are evenly spread, and in being able to sample a given plot repeatedly through time. The number of samples necessary to characterize a reporting unit also depends on the variance of the indicator of interest.
The third decision involves selecting among the many indicators used to evaluate seagrass ecosystem status across spatial scales, species, and habitats. The plethora of indicators and indices (Marbà et al., 2013) complicates both comparisons across ecosystems and the choice of optimal monitoring strategy. But a recent analysis of the sensitivity and response time of various indicators to ecosystem degradation and recovery formed the basis for a decision tree for selecting indicators to assist managers for specific management goals (Roca et al., 2016). The best established and most commonly used measure of seagrass abundance is percent cover estimated visually. Percent cover has wide application and can reduce overall sampling error because it is simple and promotes replication. Estimating cover can be subjective, but use of common reference cards, QA/QC procedures, and clear criteria can greatly improve the accuracy of cover estimates (Finn et al., 2010). Quadrat measurements of percent cover are more efficient in detecting change in seagrass cover than seagrass blade counts or above- or below-ground biomass measures, and the latter require destructive sampling (Heidelbaugh and Nelson, 1996). It is possible to estimate seagrass biomass from cover after field quantification of biomass-cover relationships (Carstensen et al., 2016). Additional indicators measured within the sampling unit may include seagrass species identity and diversity, shoot characteristics, chemical constituents, and associated flora and fauna. A few programs include process indicators such as productivity, herbivore pressure, flowering reproductive effort, and/or or recovery capacity via seed banks or shoot recruitment. Finally, some seagrass functional types and traits are more sensitive to stressors than others, which can be used in an evidence-based approach to selecting appropriate and reliable indicators for specific management goals (Roca et al., 2016).
Remote sensing and in situ sampling provide complementary views of seagrass habitat. Remote sensing across heterogeneous reporting areas often cannot detect habitat distribution changes <30% (Lee Long et al., 1996; Unsworth et al., 2009; Hossain et al., 2010; Schultz et al., 2015). Such programs require ground-truthing to increase precision and accuracy. The level of change and accuracy of detection vary across programs. There is an urgent need to design more effective monitoring capable of detecting change of 10% or less (Duarte, 2002). Approaches that use fixed plots have higher statistical power for detecting small changes compared with random-plot methods (Schultz et al., 2015). The tiered approach that links remote sensing to in situ sampling systematically offers promising solutions to this challenge (Neckles et al., 2012).
Numerous environmental drivers influence seagrass occurrence and persistence in an area (Short et al., 2014). These include the biophysical parameters that regulate physiological activity and morphology (water depth, salinity, light, temperature, nutrients, etc.), biological parameters such as herbivory and diseases, and anthropogenic impacts that inhibit plant growth such as excess nutrients and sediments. Almost all observing networks include some measure of water quality, including salinity, temperature, and/or light. Some networks implement continuous monitoring of key pressures (e.g., temperature and light) via relatively inexpensive loggers, which improves interpretation of environmental influence on seagrass condition. Other measures commonly collected include sediment condition, such as grain size and organic content (Short and Coles, 2001).
Numerous programs monitor macroalgal forests (Table S1), but coverage is patchy. Many regions have not been surveyed (Krumhansl et al., 2016) because they do not have the required infrastructure or funding and/or are inaccessible. Surveying these areas is difficult because many occur in cold, turbid, deep, and/or wave-exposed environments far from road access. For mapping distributions, some of these challenges can be overcome with remote surveying, including drones (Konar and Iken, 2018) and aerial photography. Satellites are promising for mapping of intertidal algae in particular (Brodie et al., 2018; Setyawidati et al., 2018). For kelps that form surface canopies, the Landsat series of satellites provides a record back to 1983 at 30-m resolution (Cavanaugh et al., 2011; Nijland et al., 2019) and more recent satellites provide even higher resolution (Cavanaugh et al., 2010). However, canopy-forming kelps on low-contrast bottoms or in deeper or turbid water can be difficult to see from the air, and some canopies (e.g., Nereocystis leutkeana) vary in visibility with tides. Satellite imagery has limited effectiveness for the many areas without surface kelp. Kelps can now be detected to a depth of 6 m (Uhl et al., 2016), but this only covers a portion of their depth range. Sidescan sonar is one promising technique for differentiating species (Cochrane and Lafferty, 2002; Dijkstra et al., 2017). Automated sensing of macrophyte beds by autonomous underwater vehicles (AUVs) also shows promise. Aerial UAVs have proven effective for macroalgae monitoring on intertidal reefs (Murfitt et al., 2017). As with remote sensing generally, ground-truthing is often needed to determine what lies beneath the surface.
While the general features of intertidal macroalgal communities can be surveyed from boats or aerial imagery, e.g., via the CARLIT method used in the Mediterranean (Blanfun et al., 2016), most benthic macroalgal forests cannot be detected from the air and efficient underwater surveying methods are essential. Large-scale mapping of the most conspicuous species can be done with drop-down camera imagery from a boat, video surveys by SCUBA divers aided by diver propulsion vehicles (DPVs) (Kimura et al., 2012), and AUVs (Barrett and Edgar, 2010; Bewley et al., 2015). SCUBA diver-operated cameras may be more versatile, but AUVs have more autonomy in remote areas. For long and remote coastlines, predictive modeling of marine macrophyte species distributions can be a strong aid to indicate the most likely places for species to occur as a basis for the spatial design of field campaigns and ground-truthing (Bekkby et al., 2009). Finally, programs based on volunteers can be useful analyzing or taking images for presence/absence (e.g., www.marineforests.com; https://seagrassspotter.org). Such citizen science initiatives can cover broad spatial areas because they allow participation by persons anywhere in the world as long as they have access to the internet and can photograph the appropriate organisms.
Remote surveys based on imagery often cannot distinguish species or quantify their abundances. For such purposes, scuba is usually necessary, which entails finer spatial scales. As is true for seagrasses, many surveys of macroalgae apply transect approaches with nested quadrat sampling. Recording abundances in the field saves time and space in sample processing. However, complete surveys of seaweed diversity or small recruit density can only be done with microscopic analyses. Thus, if the goals include both broad and fine-scale biodiversity or recruitment surveys, the optimal solution might be a stratified sampling design, with different resolution of sampling at different spatial scales, as described above for seagrasses (Neckles et al., 2012).
Many macroalgal forests are highly dynamic across time and space (Krumhansl et al., 2016), so long-term monitoring is needed to parse out spatial and temporal variability from longer-term directional change. As is also true for seagrasses, most macroalgal monitoring programs follow protocols that fit their local situation logistically and financially, and thus use different sampling designs, replication, taxonomic resolution, and frequency, making inter-regional comparisons difficult. Lack of consensus on the nature and the need for reference conditions has also resulted in inconsistencies in the way anthropogenic effects have been assessed and interpreted. For example, the CARLIT approach uses macroalgal assemblages from pristine areas (e.g., marine protected areas in Sardinia and the Balearic Islands in the Mediterranean) as a reference condition for assemblages on the mainland (Ballesteros et al., 2007b; Asnaghi et al., 2009). Although this can be a powerful approach to identify relatively pristine locations (as suggested by high similarity between focal and reference assemblages), it ignores the potential confounding effect of geographic segregation and the inherent differences between islands and the mainland (Benedetti-Cecchi et al., 2003). This contrasts with design-based approaches, such as Before-After/Control-Impact (BACI) designs and their evolution (Underwood, 1994), that require the interspersion of focal and reference conditions to tease apart anthropogenic influences from the effect of spatially confounding factors. From 2000–2010, the Census of Marine Life attempted to compare intertidal and shallow subtidal macroalgal forests globally using a common protocol that quantified biomass. This was very expensive and time-consuming, but resulted in some global comparisons (Cruz-Motta et al., 2010; Konar et al., 2010), with some sites still sampled today.
Due to its vast and largely inaccessible habitat in the open ocean, pelagic Sargassum is monitored mostly by satellite (Brooks et al., 2018). Satellite sensors are a valuable tool in a wide range of applications, including coastal mapping, ocean circulation monitoring, resource management, and extreme event forecasting (Table 1). The combination of spatial, temporal, spectral, and radiometric resolution of different satellite sensors helps define the potential uses of each sensor. Usually, and for ocean dynamic monitoring purposes, the feature size stretches between submesoscale (0.1–10 kilometers) and mesoscale (10–100 kilometers), with required revisit times between a few hours to a few days in most cases (Sentinel-3: ESA's Global Land and Ocean Mission for GMES Operational Services-ESA SP-1322/3, October 2012).
Practical monitoring and tracking of pelagic Sargassum uses two main satellite-derived products, the Alternative Floating Algae Index (AFAI) and the Maximum Chlorophyll Index (MCI). Both are based on the radiance/reflectance measured above a baseline interpolated between 2 neighboring spectral bands (Gower and King, 2011; Wang and Hu, 2016). The objective is to detect surface algal accumulations, identifying the patches and long lines of Sargassum. This information could then be used to study Sargassum distribution and variability and to predict beaching events. To obtain accurate and reliable results, invalid pixels must be masked for clouds, cloud shadows, sun-glint areas, sensor zenith areas, etc. The satellite core observational component (Table 1) consists of various sensors, such as the Moderate Resolution Imaging Spectroradiometer (MODIS) on Terra and Aqua and the Medium Resolution Imaging Spectrometer (MERIS) onboard the Envisat-1 satellite. Follow-up ocean color missions ensure the continuity of the time series, especially after Envisat-1 was lost in April 2012, and both MODIS satellites have exceeded their expected lifetime by several years. The Ocean Land Color Instrument (OLCI) onboard the Sentinel-3 constellation and the Visible Infrared Imaging Radiometer Suite (VIIRS) on the Joint Polar Satellite System (JPSS) satellites are multispectral radiometers that, besides their other multiple ocean color applications, also contribute to providing global and low latency information on Sargassum (Wang and Hu, 2018).
Satellite data miss small patches of Sargassum at subpixel scale. Higher resolution satellite fields, such as the Floating Algae Index (FAI) obtained from OLI on Landsat-8 and other pseudo-color imagery decrease this gap at the cost of reducing coverage, revisiting times, and often larger latencies and processing times. An optimized solution would require implementing a synergistic approach in the integration of diverse datasets, including high-resolution, low-altitude airborne measurements.
The Satellite-based Sargassum Watch System (SaWS) at the University of South Florida (USF) relies on near-real-time satellite and modeling results to detect and track pelagic Sargassum fields, which serve to create monthly outlook bulletins, showing the distribution and coverage maps in the Central West Atlantic (CWA) and Caribbean regions (Hu et al., 2016). These monthly Sargassum density maps (Figure 2A) are used to predict Sargassum blooms in the Caribbean Sea from AFAI observations in a hotspot region in the CWA (Wang and Hu, 2016). USF AFAI fields are also served through the Atlantic OceanWatch node, hosted at AOML, which provides cumulative daily, 3-day, and weekly datasets within an interoperable framework (Figure 2B).
As is true for seagrasses and kelp forests, field observations are essential to calibrating and validating satellite measurements, reducing the uncertainties and adding value to the satellite products (e.g., tuning regional algorithms). For pelagic Sargassum, geolocated visual observations (i.e., from ships, aircrafts, shore) serve as a valuable proxy of ground-truthing to test the satellite algorithms. Sites such as the one implemented by the Gulf Coast Research Laboratory collect Sargassum observation details online. The samples of Sargassum provide information about the abundance and distribution, and the opportunity to carry out genetic and morphological analysis. The Sea Education Association (SEA) has been collecting Sargassum samples using dip nets and surface neuston tows for more than 40 years in the Sargasso Sea, Caribbean and Gulf of Mexico. This dataset contributes to the study of the annual and interannual distribution of pelagic Sargassum.
As mentioned above, the effects of currents and winds in the distribution of pelagic Sargassum are not well-understood. The Atlantic Oceanographic and Meteorological Laboratory (NOAA/AOML) is currently conducting an experiment (Figure 2B) where undrogued drifters of various sizes and shapes, simulating common debris and Sargassum, were deployed in the Atlantic to assess the impact of wind and currents on their trajectories. These drifters are tracked in real time using GPS transmissions.
The scale of satellite remote sensing of drifting marine macrophytes requires coordinated, ongoing efforts involving multiple connecting stakeholders across government, academia, industry, and civil society, some of which have already been organized. Through a coordinated multi-disciplinary initiative, including interaction between scientists, data providers, environmental managers and decision makers, a practical monitoring system and accompanying Sargassum warning strategies are in development. The Spatial Data Infrastructure (SDI) component relies on products obtained from in situ and remote sensing data, specifically developed to detect and track Sargassum; numerical prediction models to determine potential trajectories and volumes; and interoperable tools. The benefits of this framework span essential economic, social, and environmental domains, defining the baseline needed to coordinate future science-driven monitoring and evaluation efforts. A pilot project for this effort is currently in place and led by IOCARIBE of IOC UNESCO, the GEO Blue Planet Initiative, UNDP Barbados and the Organization of East Caribbean States with partners from government agencies, intergovernmental initiatives, and academia, and with continued improvements expected to benefit the populations and economies of the countries in the region and beyond.
Primary goals of macrophyte observing programs include tracking status and trends in macrophyte abundance and extent as well as understanding the environmental and anthropogenic forcing of these patterns. Generally, this approach involves spatial and temporal analyses to detect change relative to benchmarks and to predict future trajectories. Observation systems take a variety of approaches depending on goals and targeted species and often differ in spatial and temporal scales, frequency of sampling, and taxonomic resolution (Tables S1a,b). Most programs for benthic macrophytes employ some form of area-based sampling, typically using quadrats of 0.25 m2 or more, nested within larger transects of 20–100 meters. The size of the sampled patches and transects depends greatly on programmatic goals and the physical and biological characteristics of the systems. Temporal coverage also varies (Tables S1a,b), with most observation systems focused primarily on longitudinal surveys at regional or sub-regional scales over many years (or seasons; e.g., MexCal). Other programs have focused on broad spatial coverage at some expense to temporal coverage (e.g., Reef Life Survey, RLS). Repeated surveys are done through fixed transects in some programs, such as the Santa Barbara Coastal Long Term Ecological Research (SBC LTER) Project and the Channel Islands Kelp Forest Monitoring, ensuring the same spaces are re-sampled annually. Other programs use stratified random sampling within sites to assess variability over time at site, but not small scales, such as in MarClim (Mieszkowska et al., 2006, 2014) and the Kelp Ecosystems Ecology Network (KEEN; www.kelpecosystems.org).
Public funding for macrophyte monitoring is ultimately motivated by interest in management of fisheries and other ecosystem services to humans. Robust Ecosystem-Based Management (Curtin and Prellezo, 2010) requires consideration of interactions among organisms within food webs. Indicators applied in EBM typically include abundances of fishes and larger invertebrates, and in some cases metrics of trophic transfer, functional diversity, and population sizes of key species. These variables generally require large logistical effort and often destructive sampling. Visual survey techniques are commonly used for fishes and include quantitative diver transects (Edgar and Stuart-Smith, 2014; Norderhaug et al., 2015), various versions of Remote Underwater Video (Langlois, 2006; Perry, 2018), and video captured by AUVs (Ling et al., 2016). Acoustic monitoring is also increasingly possible (Kaartvedt et al., 2009). As for most sampling, a combination of methods can reduce biases of individual methods (Edgar et al., 2016) and likely provides the best strategy for sampling a diverse range of biota. The requirements for effective ecosystem-based management of macrophyte systems are beyond the scope of this review, but we note that this is an important goal of macrophyte observing and should inform strategies for system design (Personnic et al., 2014; Thibaut et al., 2017).
To be useful to management, a biodiversity observing system must produce integrated data products and concise, intuitive ways to convey variability and uncertainty to non-scientific audiences. Managers generally need integrated data summaries with concrete information related to the missions of their agencies. Users of such products include institutional decision makers, environmental managers, and stakeholders in the fisheries, aquaculture, and tourism sectors. Common information needs are for status and trends in extent and condition of habitat and of commercially important, charismatic, or protected species and quality of water and environment. The Chesapeake Bay Program and the Great Barrier Reef Marine Monitoring Programme exemplify successful efforts to communicate results of seagrass status and trends to the wider general public using easily interpretable scorecards (McKenzie et al., 2017). Such approaches are invaluable for maintaining support and buy-in from stakeholders and the general public.
The usefulness of monitoring for decision makers depends on a framework for interpreting indicators of change in systems or species relevant to the question of interest (Markiewicz and Patrick, 2015). For example, do changes to a system have positive or negative consequences for people, what are the causes, and can they be reversed or mitigated? In addition to the magnitude and direction of changes, managers need guidance on risks, opportunities, and likelihood of success of different mitigating and remediating actions. Observing systems can also inform proactive planning for sustainable development and conservation by providing the spatial data necessary to evaluate benefits and trade-offs associated with different management options. For instance, Strategic Environmental Assessments (SEAs) and Environmental Impact Assessments (EIAs) require access to such data in order to inform policies and programs related to spatial planning, and whether to permit proposed development activities. Other management programs that require information generated by long-term sustained monitoring efforts include the Condition Reports produced by the National Marine Sanctuaries, and the Integrated Ecosystem Assessment (IEA) developed by the National Oceanic and Atmospheric Administration (NOAA) in the USA. These considerations emphasize that a key aspect of designing and managing an integrated observing network is involving stakeholders and a sustained focus on how the field measurements are translated to informative and useful indicators for management.
Molecular tools show promise for tackling several long-standing challenges in macrophyte monitoring. Molecular tools can screen environmental samples where no macrophytes are visible, identify species presence, and even roughly quantify them under certain conditions (Chariton et al., 2010; Pawlowski et al., 2011). DNA fingerprinting of individual organisms after local extinctions can identify whether new recruits arise from the local population or from immigration (Assis et al., 2017). A key need in realizing the great potential for screening environmental samples is developing improved reference libraries for marine macrophytes.
An inherent challenge to long-term biodiversity monitoring is changing taxonomy, which is happening rapidly for macroalgae as a result of new insights from DNA-based analyses and improved collections. Cryptic species, which may be indistinguishable without molecular methods, are common in macroalgae as they are in marine invertebrates (Knowlton, 1993). Molecular tools can be integrated into observing systems to provide sharper identification, revealing new macroalgal species along coastlines studied by PISCO in California (Neiva et al., 2017) and MarClim in the UK (Zardi et al., 2015) for example.
A related application of molecular tools is environmental DNA (eDNA) obtained from water or other environmental samples that originated in biological materials shed by organisms (Bourlat et al., 2013; Thomsen and Willerslev, 2015). Where sufficient sequence libraries exist to identify this material, eDNA can be used to confirm current or recent presence of organisms not detected by other methods. In the marine environment, eDNA has been used primarily to detect microbes and viruses, eukaryotic phytoplankton, zooplankton, macro-invertebrates, and vertebrates (Djurhuus et al., 2018; Goodwin et al., 2019). But eDNA has also been used to confirm the historic presence of seagrass at locations where it no longer exists (Hamaguchi et al., 2018). The only other study using eDNA from marine plants of which we are aware estimated the contribution of seagrasses and macroalgae to carbon stocks in sediments (Reef et al., 2017). Developing DNA barcoding resources for seagrasses and macroalgae that are suitable for eDNA approaches could greatly advance macrophyte observing systems. It is essential that such studies are cross-referenced with high quality sequence libraries connected to voucher specimens lodged in museums or herbaria (Dormontt et al., 2018). A complete DNA reference library for seagrasses is being developed by the Global Initiative to Barcode Seagrass (GIBS) (http://barcoding.seagrassonline.org/) and is more than 50% completed.
Molecular tools are also promising for inferring drivers of spatial connectivity important in management, such as those observed for giant kelp in the Santa Barbara Channel Long-Term Ecological Research site (Alberto et al., 2011; Johansson et al., 2015); for seagrasses in the Caribbean (van Dijk et al., 2018) and North Atlantic (Olsen et al., 2004); and for locating particularly rich and threatened biodiversity hotspots (Assis et al., 2018). Analysis of molecular markers in a phylogeographic context has helped understand pathways of marine animal migration (Taberlet et al., 2012) and can be similarly useful for tracking drifting macrophytes (Fraser et al., 2018; Smith et al., 2018). Molecular tools may also prove useful in characterizing genetic diversity and changes in seaweed species used in aquaculture (Valero et al., 2017).
Remote sensing provides unique opportunities for observing marine macrophytes. First, while in situ monitoring protocols are well-established for seagrasses, slow change in some species results in shifting baselines (Pauly, 1995) that make detecting change difficult (Unsworth et al., 2014), and in situ studies are highly patchy in time and space. Improvements in and lower cost of remote sensing technology, and accessibility of satellite imagery are advancing the ability to map seagrass extent (Fortes et al., 2018). Remote sensing is a promising means of mapping seagrass cover in shallow areas with clear water (Kendrick et al., 2002; McCarthy et al., 2018; Traganos and Reinartz, 2018), but it is much more difficult in the optically complex environments of turbid estuaries and where smaller species dominate. This is a key frontier for future research and technology development.
Second, remote sensing provides one of the only realistic ways to get approximate estimates of macrophyte extent in remote or poorly resourced regions. In recent decades, satellite sensor technology has developed rapidly, as has the availability of high-resolution multispectral imagery (Hossain et al., 2015). Further research is expected to improve the application of such technologies to seagrass remote sensing. No single technology can currently measure all seagrass parameters of interest, particularly at small scales, but knowledge of seagrass distribution is increasing rapidly as a result of more widely available high-resolution imagery and increasing interest in seagrass worldwide. Remote sensing may help address the key frontier of knowledge inequality between the global North and South if approaches can be better developed to suit the conditions in poorly known and resourced regions of the southern hemisphere, including high seagrass diversity, complex multi-habitat seascapes, and deep water. This approach will also require active participation of local contributors.
In Southeast Asia, for example, regional-scale estimates of seagrass extent have only recently emerged. This is primarily a result of advancements in remote sensing technology and well-funded regional projects such as the UNEP/GEF South China Sea Project, the Bay of Bengal Large Marine Ecosystem Project, and the JSPS-Asian CORE Project (Fortes et al., 2018) and the Blue Carbon Project of the Coral Triangle. Large-scale assessments are often extrapolated based on environmental conditions, with relatively low resolution (often 10 km pixels), and can produce suspect estimates of macrophyte cover and habitat suitability on local scales, particularly for animals. Such estimates need refinement but are a valuable start for poorly known and resourced regions.
Remote sensing is the primary means of tracking pelagic Sargassum, estimating monthly mean biomass of at least 4.4 million tons drifting in the Caribbean Sea and Central West Atlantic in July 2015 (Hu et al., 2015; Wang et al., 2018). Combining molecular markers with oceanographic circulation models can identify movement and dispersal patterns of drifting algae and associated organisms across the ocean surface (Fraser et al., 2018). Tracking mats of Sargassum, as well as other drifting macrophytes, could be facilitated with attached GPS devices that relay position via satellite, particularly by deploying paired drifters alongside Sargassum mats, drogued at different depths to track and quantify divergence between Sargassum and ocean currents (Figures 2B,C). Similar work conducted with small sea turtles (Putman and Mansfield, 2015) greatly improved predictions of their distribution in particle-tracking dispersal models (Putman et al., 2015). Such coordination of targeted in situ sampling with remotely sensed observations of Sargassum and numerical modeling would help refine and validate inferences from satellite-based observations. For pelagic Sargassum, a key research area is determining the effects of transport within the upper few meters of the ocean, prioritizing research through sensitivity analyses (Putman et al., 2018). For example, predicting the timing and location of Sargassum beaching over a period of a few weeks might be highly sensitive to wind activity in the Caribbean Sea. Such modeling analyses could help prioritize aspects of transport for empirical investigations.
The advent of new massive data collection systems, computers with enhanced processing and storage capabilities, and algorithms to parse and structure data offers a set of powerful emerging technologies that can be deployed in biodiversity observations. Machine Learning (ML) is a subset of Artificial Intelligence and aims to identify meaningful patterns and associations in data and use them to produce models that can predict future outcomes. One of the most promising ML techniques is Deep Learning, usually linked to its popular architecture, Deep Neural Networks (DNN). New ML frameworks such as TensorFlow and PyTorch implement DNNs that can be easily applied to diverse classification and regression problems. In oceanography and satellite data processing, artificial neural networks have been applied intensively only in the last few years. Multiple applications include modeling and predicting pathogen outbreaks (Wang and Deng, 2016), SAR image classification (Bentes et al., 2015), fish detection and recognition (Villon et al., 2016), ocean color product generation (Hieronymi et al., 2017), satellite biogeographic seascape classifications (Kavanaugh et al., 2016), carbon flux estimates (Laruelle et al., 2017), the drift paths of massive blooms of Ulva prolifera (Hu et al., 2018), and species recognition in images from diver visual surveys (Edgar et al., 2016). For pelagic Sargassum monitoring, ML techniques could be applied to estimate total biomass, analyze environmental factors driving growth and distribution, develop “sensorless” classification models to identify Sargassum in remote imagery, and predict trajectories.
Access to relevant, high-quality data is critical to informing sustainable management and use of the ocean. But there are many challenges to achieving consistent and intercomparable data flows at the scale and accuracy required, including differing quality, time frames, scales, and resolutions. Methods of data collection may affect the interoperability and interpretation of data for use in decision-making. Biodiversity monitoring efforts tend to be widely distributed, challenging the production of a global, or even regional understanding of ecosystem states. Conceptual frameworks such as the Essential Biodiversity Variables (EBVs) and the EOVs can help to generate interoperable, multi-purpose data based on common monitoring protocols (Muller-Karger et al., 2018). These data can be made available in centrally accessible, open-access repositories such as IOC-UNESCO's Ocean Biogeographic Information System (OBIS; www.iobis.org), which is linked to more than 20 regional OBIS nodes and 500 organizations worldwide, facilitating integration of observations—in this case, more than 45 million—to support marine biodiversity assessments. Data uploaded into OBIS have the additional benefit of becoming automatically available on the Global Biodiversity Information Facility (GBIF). These global databases are integrated with herbarium data, literature record compilations, and citizen science image-based records at www.marineforests.com. In any such large-scale database it is important to consult taxonomic specialists and regional experts to curate and validate data before using, because misidentification of species in the published literature is a challenging and common problem in many macrophyte groups.
One challenge to better integration and standardization of biodiversity data is defining a common language. Protocols such as the Extended Darwin Core, adopted by OBIS and GBIF, offer standardized and stable sets of terms to facilitate publishing and sharing of information about biological diversity by providing reference definitions and examples (Wieczorek et al., 2012). The Darwin Core Archive (DwC-A) provides taxonomic and spatially explicit information on species occurrences, while the “extended” version provides flexibility to adapt the Darwin Core to include additional information. Standards such as these should be complemented by detailed metadata, specification of data sources and methods used, and the attributed license and any use restrictions. Recent progress has been made toward fewer and more user-friendly data licenses, reducing the uncertainty surrounding how data may be used. Most notably, Creative Commons licenses (http://creativecommons.org) have made licenses more readily accessible, understandable and easily adopted by content providers, including those who distribute data.
Another major challenge to realizing a world of open data is fair credit for work and data generation (Wilkinson et al., 2016). Given the proliferation of data, it has become difficult to track the use of data in subsequent work and for data providers to demonstrate impact in the same way as those who publish peer-reviewed papers, despite the considerable effort involved in generating these data. Increasingly, digital object identifiers (DOIs) have been adopted by data publishers to provide permanent links to the original sources of data, helping to track how these data are being used in subsequent research through citations. The attribution of DOIs by data publishers thereby offers data contributors the ability to track and demonstrate their impact. This practice is also being adopted by journals (e.g., Nature Scientific Data, which provides both a paper DOI and a data DOI). Ultimately, we need multi-purpose, interoperable approaches that allow consolidation of data in meaningful ways while ensuring appropriate attribution for data providers and clarity regarding how these data can be used. Recent advances toward establishing consistent approaches globally will help to make quality data available and inform decision making at multiple scales.
Macrophytes are diverse, and the observation systems that study them similarly vary widely in nature and sophistication (Miloslavich et al., 2018). Some are run by governments, with strong training, retention of personnel, and long-term stability. Others are collaborative networks of multiple academic institutions (e.g., Partnership for Interdisciplinary Studies of Coastal Oceans, or PISCO). Some are spearheaded by a few key personnel (e.g., MarClim) and work with local partners and citizen scientists (e.g., RLS, Seagrass Watch, www.marineforests.com). Our review suggests several themes for advancing these diverse efforts toward a global network that achieves more than the sum of its parts.
Tremendous efforts are already ongoing to monitor seagrass, kelp, and pelagic Sargassum abundance and distribution, but these efforts are poorly coordinated and use a wide variety of methods that are often not comparable. There is much added value to be gained with relatively modest investment in building and sustaining platforms for easy communication, collaboration, knowledge sharing, and training. An important lesson from the Census of Marine Life is the need for common protocols to capture change across multiple scales in space and time. Only by ensuring comparability can we generate the expansive datasets needed to address variation at scales relevant to environmental management, including the pervasive effects of global climate change.
The long-term seagrass observing networks that have persisted are those that have purpose-built approaches and methods that can be consistently conducted with modest funding and person-power (Table S1). Approaches that are robust and usable across a variety of seascapes, taxa, and habitats are most accessible to diverse contributors and regions. A simple, standardized core field sampling architecture can then provide a scaffolding for integration—and ground-truthing—of new technology, including remote sensing, machine learning techniques for species recognition from imagery, and eDNA, when and where resources are available. Given the number of methods available, aims need to be clearly defined to assess trade-offs between data resolution and monitoring feasibility.
Achieving widespread community buy-in for coordinated monitoring of marine macrophytes will be advanced by clearly linking observing activities to the needs of local participants, policy makers, and decision makers. The data collected need to be scientifically rigorous enough for acceptance by policy makers but simple enough to be conducted widely and communicated clearly to the general public. Coordination among efforts will also increase efficiency and add value. Better coordination and mapping of macrophyte extent and quality would advance initiatives to develop integrated seagrass accounts that map ecosystem services and value them as natural capital. Building a clearly articulated natural capital base for marine macrophytes could open doors to new funding streams by linking ecosystem services to end users (e.g., fishers, carbon traders, tourist industry). For example, Essential Ocean Variables for marine macrophytes could link to blue carbon initiatives, which have the potential to develop a commercial user base and supplementary funding.
Any long-term project will experience lapses in funding, leadership, and/or other interruptions, and most rely on substantial leveraging of in-kind support from participants. To sustain an observing system under such circumstances, it is imperative that the work addresses local needs, builds capacity and community, and provides opportunities across career levels, including future generations. Local participants must also feel ownership and a degree of control if efforts are to be sustained. These results can be met with an approach based on a standardized but flexible sampling design and protocols that provide a scaffolding on which additional activities of local interest can be built and that catalyzes and adds value to those activities. For example, implementing a standard seagrass monitoring protocol often provides opportunities for participants to quantify commercially important species during the same surveys, provides educational opportunities to local students, and so on. An important component of all observing networks is feedback and outreach. Not only is this critical for networks that rely on voluntary data contributions, but it ensures project outputs remain useful for evidence-based policy and management decisions. Sustaining a network of monitoring sites ultimately requires strong collaborations in addition to knowledge and technology transfer among participating observatories. This is especially urgent in Small Island Developing States and Least Developed Countries, where the knowledge gaps are largest and coastal ecosystems are particularly vulnerable. This is a core goal of the Group on Earth Observations Biodiversity Observation Networks (Pereira et al., 2013). Similarly, GOOS aims to tackle this challenge by promoting standardized protocols and data management best practices (Bax et al., 2018).
In many regions, Local Ecological Knowledge (LEK) can provide unique data to establish baselines and to understand historical change (Johannes et al., 2000; Beaudreau and Levin, 2014; Frans and Auge, 2016; Aswani et al., 2018), as it often incorporates observations over longer time periods and preserves memories of rare and extreme events (Moller et al., 2004). LEK can also be used to direct exploratory scientific sampling efforts. Engaging fishers and other users specifically can take advantage of their regular access to the sea (Hashim et al., 2017; Cullen-Unsworth et al., 2018). A combination of science and LEK can provide data across larger temporal and spatial scales. LEK and associated citizen science have been increasingly applied to help map and monitor marine habitats, including seagrasses, at global scales in cost-effective ways (Titilola, 1990; Moller et al., 2004; Jones et al., 2017) and to help design marine protected areas (Ban et al., 2009). Such approaches also provide opportunities for engagement, empowerment, and an improved sense of well-being. The capacity for LEK to contribute to large-scale ocean observation systems remains largely untapped. Finally, citizen-based monitoring can provide valuable data under the right circumstances (McKenzie et al., 2000; Short et al., 2006), although it is difficult to implement in regions where macrophytes are submerged and inaccessible.
Reliable replication over space and time and rigorous standardization are essential in any monitoring program aimed at detecting changes. Providing support, training, coordination, and data management for monitoring is a challenge for all long-term observing networks as they face periods of reduced funding, which can compromise data quality. A key part of support is consistent and sustainable training tools and protocols for new technicians and other members to ensure consistent application of methods over time and space. Some networks have institutionalized training or require passing courses (e.g., KEEN, PISCO, Reef Life Survey, and MBON Pole to Pole), a model that should be widely adopted.
Sustaining observing efforts over the long term requires plans for continuity of leadership, funding, training, and data management. In most networks, a small number of champions act as a driving force, and there is little planning for succession and turnover. Such plans are needed to sustain any program through the long-term. The Kelp Forest Monitoring program provides a valuable model in that it has successfully turned over leadership twice in its multi-year lifespan, in part due to such planning.
Nearly all monitoring programs are conducted by marine scientists or environmental practitioners. However, over the last couple of decades, citizen science has also begun to contribute significantly to science, education, and policy (Jones et al., 2017). Citizen monitoring is most successful when it requires minimal specialized equipment and resources. Nearly a third of the current long-term seagrass observing networks include some level of citizen science, including SeagrassWatch and SeagrassSpotter.org as well as TeaComposition H2O. The incorporation of approaches based on Information and Communication Technology (ICT) into citizen science expands its reach. By using a web and phone app approach, SeagrassSpotter.org has to date collected data in 75 countries and included observations of 36 species, and www.marineforests.com includes thousands of photographic records around the world. Citizen science is also included in the Kelp Ecosystem Ecology Network. In Southeast Asia, citizen initiatives are key to the effective linkage between science, policy, and practice, which is the core of coastal natural resources management (Fortes et al., 2018).
Observing systems are only as good as the data produced. Collection, storage, and use of data must be designed with a long-term vision, engaging global institutions to ensure data security and accessibility. To assure users of data quality, observing networks should implement clear, formal, and transparent practices for quality assurance and quality control (QA/QC) and data management. Data management is opaque for many current long-term monitoring programs, with only a few having centralized systems and even fewer with clear QA/QC protocols. Clear and transparent policies on data ownership and procedures for data access are also critical for any observing network (Wilkinson et al., 2016), particularly when it includes multiple contributors from many organizations. Implementing standard data agreements facilitates confidence among data collectors, stewards, and users.
Comparisons across space and time can be challenging where taxonomic expertise is inadequate. Some observation systems target only major structure-forming taxa, while others sample a much broader taxonomic range. Increasing taxonomic depth is time consuming and expensive, and the inherent tradeoff between sampling scale and taxonomic depth is a key hurdle to harmonizing biological observation efforts. The level of taxonomic resolution also depends strongly on the knowledge of system participants. One possible solution involves strong, independent cross-verification of identifications based on archived voucher collections. Methods based on imagery have the advantage of being independently verified by other observers in future analyses. Photographs and voucher collections have been particularly important in cases where species names change as cryptic species are discovered (Zardi et al., 2015; Neiva et al., 2017). Thus, archiving images and voucher collections makes surveys more valuable. Formal systems for classifying functional groups (Althaus et al., 2015) would advance cross-system comparisons, including across temperate and tropical systems. Achieving harmonized strategies for aligning taxonomic or functional levels, good management of leadership, methodologies, training, and data management, should not only produce more effective and efficient observing in practice but will facilitate a more accurate global picture of change in macrophyte systems. Such efforts should incorporate rigorous documentation and open-access archiving of protocols and all stages of workflow, from field surveys to data management (e.g., through Ocean Best Practices), and provide open access data.
Over the last decade or so, fisheries have increasingly explored, and sometimes adopted, an ecosystems approach (Travis et al., 2014; Patrick and Link, 2015), recognizing that harvested species are connected via a web of complex interactions with abiotic forcing and other species that may confound management based on simpler models. The same situation holds for coastal vegetation (Duffy et al., 2013b). Long-term observations have confirmed that decline in coastal macrophyte ecosystems resulted from both excess nutrient loading and altered food webs resulting from harvesting in the Baltic Sea (Eriksson et al., 2011) and California (Hughes et al., 2013). These examples emphasize the need for an ecosystem-based approach to coastal resource management and that this requires management—and monitoring—of multiple biological and environmental variables.
The substantial prior efforts devoted to monitoring and research on seagrasses, macroalgal forests, and pelagic Sargassum offer several valuable lessons for envisioning a more ambitious, coordinated effort in support of a global observing system. Successful observing programs are driven by tractable questions with rigorous, common statistical designs that meet specific scientific and policy needs. Long-term observation projects for coastal seagrasses and macroalgae have been substantially under-resourced relative to observations of oceanic phytoplankton despite the outsized importance of coastal vegetation in fisheries support, coastal protection, global carbon dynamics, and other ecosystem services. A key, achievable goal is to reach consensus on when, where, and how methods can be harmonized to provide a minimal set of common metrics, agreed sampling designs, and data reporting mechanisms and standards that build toward global coverage. Implementing a robust large-scale observation network should consider the past, with the wealth of legacy data and LEK available, the shifting baselines of the present, and focus attention proactively on the range of possible future outcomes for the health and ecosystem services provided by seagrass meadows, macroalgal forests, and pelagic Sargassum.
JD, LB-C, JT, and FM-K developed the project idea and coordinated writing and editing. LM and JB led assembly of network data for Table S1. All authors contributed to manuscript writing and/or editing.
This review was supported by US National Science Foundation award OCE-1829922 to JD, as well as a large number of sources to the 47 co-authors.
SY was employed by DHI Water & Environment.
The remaining authors declare that the research was conducted in the absence of any commercial or financial relationships that could be construed as a potential conflict of interest.
This is contribution 34 from the Smithsonian's MarineGEO Network. JT was funded by NOAA/OceanWatch & GG was funded by NOAA/AOML. This manuscript is also a contribution to the Marine Biodiversity Observation Network (MBON) of the Group on Earth Observations Biodiversity Observation Network, and to the Integrated Marine Biosphere Research (IMBeR) project, which is supported by the Scientific Committee on Oceanic Research (SCOR) and Future Earth. The work leading up to the manuscript was funded in part under the US National Ocean Partnership Program (NOPP RFP NOAA-NOS-IOOS-2014-2003803 in partnership between NOAA, BOEM, NASA, the US Integrated Ocean Observing System (IOOS) Program Office, and NSF. Specifically, the MBON work was funded through NASA grant NNX14AP62A to FM-K [National Marine Sanctuaries as Sentinel Sites for a Demonstration Marine Biodiversity Observation Network (MBON)].
The Supplementary Material for this article can be found online at: https://www.frontiersin.org/articles/10.3389/fmars.2019.00317/full#supplementary-material
Alberto, F., Raimondi, P. T., Reed, D. C., Watson, J. R., Siegel, D. A., Mitarai, S., et al. (2011). Isolation by oceanographic distance explains genetic structure for Macrocystis pyrifera in the santa barbara channel. Molecul. Ecol. 20, 2543–2554. doi: 10.1111/j.1365-294X.2011.05117.x
Althaus, F., Hill, N., Ferrari, R., Edwards, L., Przeslawski, R., Schönberg, C. H. L., et al. (2015). A standardised vocabulary for identifying benthic biota and substrata from underwater imagery: the CATAMI classification scheme. PLoS ONE 10:e0141039. doi: 10.1371/journal.pone.0141039
Altizer, S., Ostfeld, R. S., Johnson, P. T. J., Kutz, S., and Harvell, C. D. (2013). Climate change and infectious diseases: from evidence to a predictive framework. Science 341, 514–519. doi: 10.1126/science.1239401
Aragones, L., and Marsh, H. (2000). Impact of dugong grazing and turtle cropping on tropical seagrass communities. Pacific Conserv. Biol. 5, 286–288. doi: 10.1071/PC000277
Araujo, R. M., Assis, J., Aguillar, R., Airoldi, L., Barbara, I., Bartsch, I., et al. (2016). Status, trends and drivers of kelp forests in Europe: an expert assessment. Biodivers. Conserv. 25, 1319–1348. doi: 10.1007/s10531-016-1141-7
Arkema, K. K., Guannel, G., Verutes, G., Wood, S. A., Guerry, A., Ruckelshaus, M., et al. (2013). Coastal habitats shield people and property from sea-level rise and storms. Nat. Clim. Chang. 3, 913–918. doi: 10.1038/nclimate1944
Asnaghi, V., Chiantore, M., Bertolotto, R. M., Parravicini, V., Cattaneo-Vietti, R., Gaino, F., et al. (2009). Implementation of the European water framework directive: natural variability associated with the CARLIT method on the rocky shores of the Ligurian Sea (Italy). Mar. Ecol. Evol. Persp 30, 505–513. doi: 10.1111/j.1439-0485.2009.00346.x
Assis, J., Araujo, M. B., and Serrao, E. A. (2018). Projected climate changes threaten ancient refugia of kelp forests in the North Atlantic. Global Change Biol. 24, E55–E66. doi: 10.1111/gcb.13818
Assis, J., Berecibar, E., Claro, B., Alberto, F., Reed, D., Raimondi, P., et al. (2017). Major shifts at the range edge of marine forests: the combined effects of climate changes and limited dispersal. Sci. Rep. 7. doi: 10.1038/srep44348
Assis, J., Coelho, N. C., Lamy, T., Valero, M., Alberto, F., and Serrao, E. A. (2016). Deep reefs are climatic refugia for genetic diversity of marine forests. J. Biogeogr. 43, 833–844. doi: 10.1111/jbi.12677
Assis, J., Serrao, E. A., Claro, B., Perrin, C., and Pearson, G. A. (2014). Climate-driven range shifts explain the distribution of extant gene pools and predict future loss of unique lineages in a marine brown alga. Mol. Ecol. 23, 2797–2810. doi: 10.1111/mec.12772
Aswani, S., Lemahieu, A., and Sauer, W. (2018). Global trends of local ecological knowledge and future implications. PLoS ONE 13:e0195440. doi: 10.1371/journal.pone.0195440
Baden, S., Boström, C., Tobiasson, S., Arponen, H., and Moksnes, P.-O. (2010). Relative importance of trophic interactions and nutrient enrichment in seagrass ecosystems: a broad-scale field experiment in the Baltic-Skagerrak area. Limnol. Oceanogr. 55:1435. doi: 10.4319/lo.2010.55.3.1435
Ballesteros, E., Cebrian, E., and Alcoverro, T. (2007a). Mortality of shoots of Posidonia oceanica following meadow invasion by the red alga Lophocladia lallemandii. Botan Marina 50, 8–13. doi: 10.1515/BOT.2007.002
Ballesteros, E., Torras, X., Pinedo, S., García, M., Mangialajo, L., and de Torres, M. (2007b). A new methodology based on littoral community cartography dominated by macroalgae for the implementation of the European Water Framework Directive. Mar. Pollut. Bull. 55, 172–180. doi: 10.1016/j.marpolbul.2006.08.038
Ban, N. C., Picard, C. R., and Vincent, A. C. J. (2009). Comparing and integrating community-based and science-based approaches to prioritizing marine areas for protection. Conserv. Biol. 23, 899–910. doi: 10.1111/j.1523-1739.2009.01185.x
Barrett, N. S., and Edgar, G. J. (2010). Distribution of benthic communities in the fjord-like Bathurst Channel ecosystem, south-western Tasmania, a globally anomalous estuarine protected area. Aquat. Conserv. 20, 397–406. doi: 10.1002/aqc.1085
Bax, N. J., Appletans, W., Brainard, R., Duffy, J. E., Dunstan, P., Hanich, Q., et al. (2018). Linking capacity development to monitoring networks to achieve sustained ocean observation. Front. Mar. Sci. 5:346. doi: 10.3389/fmars.2018.00346
Beaudreau, A. H., and Levin, P. S. (2014). Advancing the use of local ecological knowledge for assessing data-poor species in coastal ecosystems. Ecol. Appl. 24, 244–256. doi: 10.1890/13-0817.1
Beck, M. W., Heck, K. L Jr, Able, K. W., Childers, D. L., Eggleston, D. B., Gillanders, B. M., et al. (2001). The identification, conservation, and management of estuarine and marine nurseries for fish and invertebrates. Bioscience 51, 633–641. doi: 10.1641/0006-3568(2001)051<0633:TICAMO>2.0.CO;2
Bekkby, T., Rinde, E., Erikstad, L., and Bakkestuen, V. (2009). Spatial predictive distribution modelling of the kelp species Laminaria hyperborea. ICES J. Mar. Sci. 66, 2106–2115. doi: 10.1093/icesjms/fsp195
Benedetti-Cecchi, L., Maggi, E., Bertocci, I., Vaselli, S., Micheli, F., Osio, G. C., et al. (2003). Variation in rocky shore assemblages in the northwestern Mediterranean: contrasts between islands and the mainland. J. Exp. Mar. Biol. Ecol. 293, 193–215. doi: 10.1016/S0022-0981(03)00220-X
Benedetti-Cecchi, L., Pannacciulli, F., Bulleri, F., Moschella, P. S., Airoldi, L., Relini, G., et al. (2001). Predicting the consequences of anthropogenic disturbance: large-scale effects of loss of canopy algae on rocky shores. Mar. Ecol. Prog. Ser. 214, 137–150. doi: 10.3354/meps214137
Benedetti-Cecchi, L., Tamburello, L., Maggi, E., and Bulleri, F. (2015). Experimental perturbations modify the performance of early warning indicators of regime shift. Curr. Biol. 25, 1867–1872. doi: 10.1016/j.cub.2015.05.035
Bennett, S., Wernberg, T., Connell, S. D., Hobday, A. J., Johnson, C. R., and Poloczanska, E. S. (2016). The ‘Great Southern Reef': social, ecological and economic value of Australia's neglected kelp forests. Mar. Freshwat. Res. 67, 47–56. doi: 10.1071/MF15232
Bentes, C., Velotto, D., and Lehner, S. (2015). “Target classification in oceanographic SAR images with deep neural networks: Architecture and initial results,” in 2015 IEEE International Geoscience and Remote Sensing Symposium (IGARSS), 3703–3706. doi: 10.1109/IGARSS.2015.7326627
Bertocci, I., Araujo, R., Oliveira, P., and Sousa-Pinto, I. (2015). REVIEW: potential effects of kelp species on local fisheries. J. Appl. Ecol. 52, 1216–1226. doi: 10.1111/1365-2664.12483
Bewley, M. S., Nourani-Vatani, N., Rao, D., Douillard, B., Pizarro, O., and Williams, S. B. (2015). Hierarchical classification in AUV imagery. Springer Trac. Adv. R. 105, 3–16. doi: 10.1007/978-3-319-07488-7_1
Blamey, L. K., and Bolton, J. J. (2018). The economic value of South African kelp forests and temperate reefs: past, present and future. J. Mar. Syst. 188, 172–181. doi: 10.1016/j.jmarsys.2017.06.003
Blanfun,é, A., Boudouresque, C. F., Verlaque, M., Beqiraj, S., Kashta, L., Nasto, I., et al. (2016). Response of rocky shore communities to anthropogenic pressures in Albania (Mediterranean Sea): ecological status assessment through the CARLIT method. Mar. Pollut. Bull. 109, 409–418. doi: 10.1016/j.marpolbul.2016.05.041
Bonsdorff, E., Blomqvist, E. M., Mattila, J., and Norkko, A. (1997). Coastal eutrophication: causes, consequences and perspectives in the Archipelago areas of the northern Baltic Sea. Est. Coast. Shelf Sci. 44, 63–72. doi: 10.1016/S0272-7714(97)80008-X
Bos, A. R., Bouma, T. J., de Kort, G. L. J., and van Katwijk, M. M. (2007). Ecosystem engineering by annual intertidal seagrass beds: Sediment accretion and modification. Est. Coast. Shelf Sci. 74, 344–348. doi: 10.1016/j.ecss.2007.04.006
Boström, C., Baden, S., and Krause-Jensen, D. (2003). “The seagrasses of Scandinavia and the Baltic Sea,” in World Atlas of Seagrasses: Present Status and Future Conservation, eds E. Green, F. Short, and M. Spalding (Berkley: California Press, 27–37.
Bourlat, S. J., Borja, A., Gilbert, J., Taylor, M. I., Davies, N., Weisberg, S. B., et al. (2013). Genomics in marine monitoring: new opportunities for assessing marine health status. Mar. Pollut. Bull. 74, 19–31. doi: 10.1016/j.marpolbul.2013.05.042
Breitburg, D. L., Craig, J. K., Fulford, R. S., Rose, K. A., Boynton, W. R., Brady, D. C., et al. (2009). Nutrient enrichment and fisheries exploitation: interactive effects on estuarine living resources and their management. Hydrobiologia 629, 31–47. doi: 10.1007/s10750-009-9762-4
Brodie, J., Ash, L. V., Tittley, I., and Yesson, C. (2018). A comparison of multispectral aerial and satellite imagery for mapping intertidal seaweed communities. Aquat. Conserv. 28, 872–881. doi: 10.1002/aqc.2905
Brodie, J., Williamson, C. J., Smale, D. A., Kamenos, N. A., Mieszkowska, N., Santos, R., et al. (2014). The future of the northeast Atlantic benthic flora in a high CO 2world. Ecol. Evol. 4, 2787–2798. doi: 10.1002/ece3.1105
Brooks, M. T., Coles, V. J., Hood, R. R., and Gower, J. F. (2018). Factors controlling the seasonal distribution of pelagic Sargassum. Mar. Ecol. Prog. Ser. 599, 1–18. doi: 10.3354/meps12646
Brun, F. G., van Zetten, E., Cacabelos, E., and Bouma, T. J. (2009). Role of two contrasting ecosystem engineers (Zostera noltiiand Cymodocea nodosa) on the food intake rate of Cerastoderma edule. Helgol. Mar. Res. 63, 19–25. doi: 10.1007/s10152-008-0134-7
Burkholder, J., Tomasko, D., and Touchette, B. (2007). Seagrasses and eutrophication. J. Exp. Mar. Biol. Ecol. 350, 46–72. doi: 10.1016/j.jembe.2007.06.024
Buschmann, A. H., Camus, C., Infante, J., Neori, A., Israel, A., Hernandez-Gonzalez, M. C., et al. (2017). Seaweed production: overview of the global state of exploitation, farming and emerging research activity. Eur. J. Phycol. 52, 391–406. doi: 10.1080/09670262.2017.1365175
Butler, J. R. A., Tawake, A., Skewes, T., Tawake, L., and McGrath, V. (2012). Integrating traditional ecological knowledge and fisheries management in the torres strait, Australia: the catalytic role of turtles and dugong as cultural keystone species. Ecol. Soc. 17:34. doi: 10.5751/ES-05165-170434
Campagne, C. S., Salles, J.-M., Boissery, P., and Deter, J. (2015). The seagrass Posidonia oceanica: ecosystem services identification and economic evaluation of goods and benefits. Mar. Pollut. Bull. 97, 391–400. doi: 10.1016/j.marpolbul.2015.05.061
Carpenter, K. E., Abrar, M., Aeby, G., Aronson, R. B., Banks, S., Bruckner, A., et al. (2008). One-third of reef-building corals face elevated extinction risk from climate change and local impacts. Science 321, 560–563. doi: 10.1126/science.1159196
Carr, A. (1987). New perspectives on the pelagic stage of sea turtle development. Conserv. Biol. 1, 103–121. doi: 10.1111/j.1523-1739.1987.tb00020.x
Carstensen, J., Krause-Jensen, D., and Balsby, T. J. S. (2016). Biomass-cover relationship for eelgrass meadows. Est. Coasts 39, 440–450. doi: 10.1007/s12237-015-9995-6
Cavanaugh, K., Cavanaugh, K. C., Siegel, D. A., and Reed, D. C. (2011). Environmental controls of giant-kelp biomass in the Santa Barbara Channel, California. Mar. Ecol. Progr. Seri. Oldendorf. 429, 1–17. doi: 10.3354/meps09141
Cavanaugh, K. C., Cavanaugh, K., Siegel, D., and Kinlan, B. P. (2010). Scaling giant kelp field measurements to regional scales using satellite observations. Mar. Ecol. Progr. Seri. Oldendorf. 403, 13–27. doi: 10.3354/meps08467
Chariton, A. A., Court, L. N., Hartley, D. M., Colloff, M. J., and Hardy, C. M. (2010). Ecological assessment of estuarine sediments by pyrosequencing eukaryotic ribosomal DNA. Front. Ecol. Environ. 8, 233–238. doi: 10.1890/090115
Chefaoui, R. M., Duarte, C. M., and Serrão, E. A. (2018). Dramatic loss of seagrass habitat under projected climate change in the Mediterranean Sea. Glob. Chang. Biol. 24, 4919–4928. doi: 10.1111/gcb.14401
Chenelot, H., and Konar, B. (2007). Lacuna vincta (Mollusca, Neotaenioglossa) herbivory on juvenile and adult Nereocystis luetkeana (Heterokontophyta, Laminariales). Hydrobiologia 583, 107–118. doi: 10.1007/s10750-006-0484-6
Chopin, T., Buschmann, A. H., Halling, C., Troell, M., Kautsky, N., Neori, A., et al. (2001). Integrating seaweeds into marine aquaculture systems: a key toward sustainability. J. Phycol. 37, 975–986. doi: 10.1046/j.1529-8817.2001.01137.x
Christianen, M. J. A., Govers, L. L., Bouma, T. J., Kiswara, W., Roelofs, J. G. M., Lamers, L. P. M., et al. (2011). Marine megaherbivore grazing may increase seagrass tolerance to high nutrient loads. J. Ecol. 100, 546–560. doi: 10.1111/j.1365-2745.2011.01900.x
Cloern, J. (2001). Our evolving conceptual model of the coastal eutrophication problem. Mar. Ecol. Prog. Ser. 210, 223–253. doi: 10.3354/meps210223
Cochrane, G. R., and Lafferty, K. D. (2002). Use of acoustic classification of sidescan sonar data for mapping benthic habitat in the Northern Channel Islands, California. Cont. Shelf Res. 22, 683–690. doi: 10.1016/S0278-4343(01)00089-9
Coleman, M. A., Kelaher, B. P., Steinberg, P. D., and Millar, A. J. K. (2008). Absence of a large brown macroalga on urbanized rocky reefs around Sydney, Australia, and evidence for historical decline. J. Phycol. 44, 897–901. doi: 10.1111/j.1529-8817.2008.00541.x
Costanza, R., dArge, R., deGroot, R., Farber, S., Grasso, M., Hannon, B., et al. (1997). The value of the world's ecosystem services and natural capital. Nature 387, 253–260. doi: 10.1038/387253a0
Costanza, R., Pérez-Maqueo, O., Martinez, M. L., Sutton, P., Anderson, S. J., and Mulder, K. (2008). The value of coastal wetlands for hurricane protection. Ambio 37, 241–248. doi: 10.1579/0044-7447(2008)37<241:TVOCWF>2.0.CO;2
Cruz-Motta, J. J., Miloslavich, P., Palomo, G., Iken, K., Konar, B., Pohle, G., et al. (2010). Patterns of spatial variation of assemblages associated with intertidal rocky shores: a global perspective. PLoS ONE 5:e14354. doi: 10.1371/journal.pone.0014354
Cruz-Palacios, V., and Van Tussenbroek, B. I. (2005). Simulation of hurricane-like disturbances on a Caribbean seagrass bed. J. Exp. Mar. Biol. Ecol. 324, 44–60. doi: 10.1016/j.jembe.2005.04.002
Cullen-Unsworth, L., Jones, B., Seary, R., Newman, R., and Unsworth, R. (2018). Reasons for seagrass optimism: local ecological knowledge confirms presence of dugongs. Mar. Pollut. Bull.134, 118–122. doi: 10.1016/j.marpolbul.2017.11.007
Cullen-Unsworth, L., and Unsworth, R. (2013). Seagrass meadows, ecosystem services, and sustainability. Environment 55, 14–27. doi: 10.1080/00139157.2013.785864
Cullen-Unsworth, L. C., Nordlund, L. M., Paddock, J., Baker, S., McKenzie, L. J., and Unsworth, R. K. F. (2014). Seagrass meadows globally as a coupled social-ecological system: Implications for human wellbeing. Mar. Pollut. Bull. 83, 387–397. doi: 10.1016/j.marpolbul.2013.06.001
Cunha, A. H., Assis, J. F., and Serrão, E. A. (2013). Seagrasses in Portugal: a most endangered marine habitat. Aquat. Bot. 104, 193–203. doi: 10.1016/j.aquabot.2011.08.007
Curtin, R., and Prellezo, R. (2010). Understanding marine ecosystem based management: a literature review. Mar. Policy 34, 821–830. doi: 10.1016/j.marpol.2010.01.003
Darling, E. S., Alvarez-Filip, L., Oliver, T. A., Mcclanahan, T. R., and Côté, I. M. (2012). Evaluating life-history strategies of reef corals from species traits. Ecol. Lett. 15, 1378–1386. doi: 10.1111/j.1461-0248.2012.01861.x
de la Torre-Castro, M., and Rönnbäck, P. (2004). Links between humans and seagrasses - an example from tropical East Africa. Ocean Coast. Manage. 47, 361–387. doi: 10.1016/j.ocecoaman.2004.07.005
Dennison, W. C. (1987). Effects of light on seagrass photosynthesis, growth, and depth distribution. Aquat. Bot. 27, 15–26. doi: 10.1016/0304-3770(87)90083-0
Dewsbury, B. M., Bhat, M., and Fourqurean, J. W. (2016). A review of seagrass economic valuations: gaps and progress in valuation approaches. Ecosyst. Serv. 18, 68–77. doi: 10.1016/j.ecoser.2016.02.010
Dijkstra, J. A., Harris, L. G., Mello, K., Litterer, A., Wells, C., and Ware, C. (2017). Invasive seaweeds transform habitat structure and increase biodiversity of associated species. J. Ecol. 105, 1668–1678. doi: 10.1111/1365-2745.12775
Djurhuus, A., Pitz, K., Sawaya, N. A., Rojas-Márquez, J., Michaud, B., Montes, E., et al. (2018). Evaluation of marine zooplankton community structure through environmental DNA metabarcoding. Limnol. Oceanogr. 16, 209–221. doi: 10.1002/lom3.10237
Dormontt, E. E., Van Dijk, K., Bell, K. L., Biffin, E., Breed, M. F., Byrne, M., et al. (2018). Advancing DNA metabarcoding applications for plants requires systematic analysis of herbarium collections-an Australian perspective. Front. Ecol. Evol. 6:134. doi: 10.3389/fevo.2018.00134
Duarte, C. (1991). Allometric scaling of seagrass form and productivity. Mar. Ecol. Progr. Seri. 77, 289–300. doi: 10.3354/meps077289
Duarte, C. M. (1995). Submerged aquatic vegetation in relation to different nutrient regimes. Ophelia 41, 87–112. doi: 10.1080/00785236.1995.10422039
Duarte, C. M. (2002). The future of seagrass meadows. Environ. Conserv. 29, 192–206. doi: 10.1017/S0376892902000127
Duarte, C. M. (2017). Reviews and syntheses: Hidden forests, the role of vegetated coastal habitats in the ocean carbon budget. Biogeosciences 14, 301–310. doi: 10.5194/bg-14-301-2017
Duarte, C. M., Conley, D. J., Carstensen, J., and Sánchez-Camacho, M. (2009). Return to Neverland: shifting baselines affect eutrophication restoration targets. Estuar. Coasts 32, 29–36. doi: 10.1007/s12237-008-9111-2
Duarte, C. M., and Krause-Jensen, D. (2017). Export from seagrass meadows contributes to marine carbon sequestration. Front. Marine Sci. 4:13. doi: 10.3389/fmars.2017.00013
Duarte, C. M., Losada, I. J., Hendriks, I. E., Mazarrasa, I., and Marbà, N. (2013). The role of coastal plant communities for climate change mitigation and adaptation. Nat. Clim. Chang. 3, 961–968. doi: 10.1038/nclimate1970
Duffy, J. E., Amaral-Zettler, L. A., Fautin, D. G., Paulay, G., Rynearson, T. A., Sosik, H. M., et al. (2013a). Envisioning a marine biodiversity observation network. Bioscience 63, 350–361. doi: 10.1525/bio.2013.63.5.8
Duffy, J. E., Moksnes, P.-O., and Hughes, A. R. (2013b). “Ecology of Seagrass Communities,” in Marine Community Ecology and Conservation, eds M. D. Bertness, J. F. Bruno, B. R. Silliman, and J. J. Stachowicz (Sunderland, MA: Sinauer Associates), 271–297.
Edgar, G. J., Bates, A. E., Bird, T. J., Jones, A. H., Kininmonth, S., Stuart-Smith, R. D., et al. (2016). New approaches to marine conservation through the scaling up of ecological data. Annu. Rev. Mar. Sci. 8, 435–436. doi: 10.1146/annurev-marine-122414-033921
Edgar, G. J., and Stuart-Smith, R. D. (2014). Systematic global assessment of reef fish communities by the Reef Life Survey program. Scientific Data 1:140007. doi: 10.1038/sdata.2014.7
Eklöf, J. S., Henriksson, R., and Kautsky, N. (2006). Effects of tropical open-water seaweed farming on seagrass ecosystem structure and function. Mar. Ecol. Prog. Ser. 325, 73–84. doi: 10.3354/meps325073
Eriksson, B. K., Sieben, K., Eklöf, J., Ljunggren, L., Olsson, J., Casini, M., et al. (2011). Effects of altered offshore food webs on coastal ecosystems emphasize the need for cross-ecosystem management. AMBIO 40, 786–797. doi: 10.1007/s13280-011-0158-0
Estes, J. A., Heithaus, M., McCauley, D. J., Rasher, D. B., and Worm, B. (2016). Megafaunal impacts on structure and function of ocean ecosystems. Annu. Rev. Environ. Resourc. 41, 83–116. doi: 10.1146/annurev-environ-110615-085622
Filbee-Dexter, K., Feehan, C. J., and Scheibling, R. E. (2016). Large-scale degradation of a kelp ecosystem in an ocean warming hotspot. Mar. Ecol. Prog. Ser. 543, 141–152. doi: 10.3354/meps11554
Filbee-Dexter, K., and Scheibling, R. E. (2014). Sea urchin barrens as alternative stable states of collapsed kelp ecosystems. Mar. Ecol. Prog. Ser. 495, 1–25. doi: 10.3354/meps10573
Filbee-Dexter, K., and Wernberg, T. (2018). Rise of turfs: a new battlefront for globally declining kelp forests. Bioscience 68, 64–76. doi: 10.1093/biosci/bix147
Finn, P., Udy, N. S., Baltais, S. J., Price, K., and Coles, L. (2010). Assessing the quality of seagrass data collected by community volunteers in moreton bay marine park, Australia. Environ. Conserv. 37, 83–89. doi: 10.1017/S0376892910000251
Foden, J., and Brazier, D. P. (2007). Angiosperms (seagrass) within the EU water framework directive: a UK perspective. Mar. Pollut. Bull. 55, 181–195. doi: 10.1016/j.marpolbul.2006.08.021
Fortes, M. D., Ooi, J. L. S., Tan, Y. M., Prathep, A., Bujang, J. S., and Yaakub, S. M. (2018). Seagrass in Southeast Asia: a review of status and knowledge gaps, and a road map for conservation. Bot. Marina 61, 269–288. doi: 10.1515/bot-2018-0008
Foster, M. S., and Schiel, D. R. (2010). Loss of predators and the collapse of southern California kelp forests (?): Alternatives, explanations and generalizations. J. Exp. Mar. Biol. Ecol. 393, 59–70. doi: 10.1016/j.jembe.2010.07.002
Fourqurean, J. W., Duarte, C. M., Kennedy, H., Marbà, N., Holmer, M., Mateo, M. A., et al. (2012). Seagrass ecosystems as a globally significant carbon stock. Nat. Geosci. 5, 1–5. doi: 10.1038/ngeo1477
Fowles, A. E., Stuart-Smith, R. D., Stuart-Smith, J. F., Hill, N. A., Kirkpatrick, J. B., and Edgar, G. J. (2018). Effects of urbanisation on macroalgae and sessile invertebrates in southeast Australian estuaries. Estuar. Coast. Shelf. 205, 30–39. doi: 10.1016/j.ecss.2018.02.010
Frans, V. F., and Auge, A. A. (2016). Use of local ecological knowledge to investigate endangered baleen whale recovery in the Falkland Islands. Biol. Conserv. 202, 127–137. doi: 10.1016/j.biocon.2016.08.017
Fraser, C. I., Morrison, A. K., Hogg, A. M., Macaya, E. C., van Sebille, E., Ryan, P. G., et al. (2018). Antarctica's ecological isolation will be broken by storm-driven dispersal and warming. Nat. Clim. Chang. 8, 704–708. doi: 10.1038/s41558-018-0209-7
Fuentes, M., Delean, S., Grayson, J., Lavender, S., Logan, M., and Marsh, H. (2016). Spatial and temporal variation in the effects of climatic variables on dugong calf production. PLoS ONE 11:e0155675. doi: 10.1371/journal.pone.0155675
Fulton, C. J., Depczynski, M., Holmes, T. H., Noble, M. M., Radford, B., Wernberg, T., et al. (2014). Sea temperature shapes seasonal fluctuations in seaweed biomass within the Ningaloo coral reef ecosystem. Limnol. Oceanogr. 59, 156–166. doi: 10.4319/lo.2014.59.1.0156
Gattuso, J.-P., Gentili, B., Duarte, C. M., Kleypas, J. A., Middelburg, J. J., and Antoine, D. (2006). Light availability in the coastal ocean: impact on the distribution of benthic photosynthetic organisms and contribution to primary production. Biogeosci. Discuss. 3, 895–959. doi: 10.5194/bgd-3-895-2006
Gavio, B., and Santos-Martinez, A. (2018). Floating Sargassum in Serranilla Bank, Caribbean Colombia, may jeopardize the race to the ocean of baby sea turtles. Acta Biol. Colomb. 23, 311–313. doi: 10.15446/abc.v23n3.68113
Gedan, K. B., Kirwan, M. L., Wolanski, E., Barbier, E. B., and Silliman, B. R. (2011). The present and future role of coastal wetland vegetation in protecting shorelines: answering recent challenges to the paradigm. Clim. Change 106, 7–29. doi: 10.1007/s10584-010-0003-7
Geider, R. J., Delucia, E. H., Falkowski, P. G., Finzi, A. C., Grime, J. P., Grace, J., et al. (2001). Primary productivity of planet earth: biological determinants and physical constraints in terrestrial and aquatic habitats. Glob. Chang. Biol. 7, 849–882. doi: 10.1046/j.1365-2486.2001.00448.x
Gianni, F., Bartolini, F., Pey, A., Laurent, M., Martins, G. M., Airoldi, L., et al. (2017). Threats to large brown algal forests in temperate seas: the overlooked role of native herbivorous fish. Sci. Rep. 7:6012. doi: 10.1038/s41598-017-06394-7
Goodwin, K. D., Muller-Karger, F. E., Djurhuus, A., Allen, L. Z., Allen, A. E., McCrow, J. P., et al. (2019). “Molecular Approaches for an Operational Marine Biodiversity Observation Network,” in World Seas: an Environmental Evaluation, ed C. Shepherd (Amsterdam: Elsevier), 613–631. doi: 10.1016/B978-0-12-805052-1.00032-2
Gower, J. F., and King, S. A. (2011). Distribution of floating Sargassum in the Gulf of Mexico and the Atlantic Ocean mapped using MERIS. Int. J. Remote Sens. 32, 1917–1929. doi: 10.1080/01431161003639660
Graham, M. H., Kinlan, B. P., Druehl, L. D., Garske, L. E., and Banks, S. (2007). Deep-water kelp refugia as potential hotspots of tropical marine diversity and productivity. Proc. Natl. Acad. Sci. U.S.A. 104, 16576–16580. doi: 10.1073/pnas.0704778104
Green, E. P., and Short, F. T. (2003). World Atlas of Seagrasses. University of California Press Available online at: http://books.google.com/books?hl=en&lr=&id=dHV0NA3m2AIC&oi=fnd&pg=PA1&dq=world+atlas+of+seagrasses+short&ots=wxBQHQxIJP&sig=U3kliAAUH0Jf4H-yDtfsLZ5PLzw
Greening, H., Janicki, A., and Sherwood, E. T. (2018). “Seagrass recovery in tampa Bay, Florida (USA),” in The Wetland Book: II: Distribution, Description, and Conservation (Dordrecht: Springer), 495–506. doi: 10.1007/978-94-007-4001-3_269
Gregow, H., Laaksonen, A., and Alper, M. E. (2017). Increasing large scale windstorm damage in Western, Central and Northern European forests, 1951–2010. Sci. Rep. 7:46397. doi: 10.1038/srep46397
Greve, T. M., Borum, J., and Pedersen, O. (2003). Meristematic oxygen variability in eelgrass (Zostera marina). Limnol. Oceanogr. 48, 210–216. doi: 10.4319/lo.2003.48.1.0210
Hamaguchi, M., Shimabukuro, H., Hori, M., Yoshida, G., Terada, T., and Miyajima, T. (2018). Quantitative real-time polymerase chain reaction (PCR) and droplet digital PCR duplex assays for detecting Zostera marina DNA in coastal sediments. Limnol. Oceanogr. 16, 253–264. doi: 10.1002/lom3.10242
Hamilton, J., and Konar, B. (2007). Implications of substrate complexity and kelp variability for south-central Alaskan nearshore fish communities. Fish B-Noaa 105, 189–196.
Hammer, K. J., Borum, J., Hasler-Sheetal, H., Shields, E. C., Sand-Jensen, K., and Moore, K. A. (2018). High temperatures cause reduced growth, plant death and metabolic changes in eelgrass Zostera marina. Mar. Ecol. Prog. Ser. 604, 121–132. doi: 10.3354/meps12740
Harvell, C. D., Mitchell, C. E., Ward, J. R., Altizer, S., Dobson, A. P., Ostfeld, R. S., et al. (2002). Climate warming and disease risks for terrestrial and marine biota. Science 296, 2158–2162. doi: 10.1126/science.1063699
Hashim, M., Ito, S., Numata, S., Hosaka, T., Hossain, M. S., Misbari, S., et al. (2017). Using fisher knowledge, mapping population, habitat suitability and risk for the conservation of dugongs in Johor Straits of Malaysia. Marine Policy 78, 18–25. doi: 10.1016/j.marpol.2017.01.002
Heck, K., Hays, G., and Orth, R. (2003). Critical evaluation of the nursery role hypothesis for seagrass meadows. Mar. Ecol. Prog. Ser. 253, 123–136. doi: 10.3354/meps253123
Heidelbaugh, W. S., and Nelson, W. G. (1996). A power analysis of methods for assessment of change in seagrass cover. Aquat. Bot. 53, 227–243. doi: 10.1016/0304-3770(95)01000-9
Hieronymi, M., Müller, D., and Doerffer, R. (2017). The OLCI neural network swarm (ONNS): a bio-geo-optical algorithm for open ocean and coastal waters. Front. Marine Sci. 4:138. doi: 10.3389/fmars.2017.00140
Hill, R., Bellgrove, A., Macreadie, P., Petrou, K., Beardall, J., Steven, A., et al. (2015). Can macroalgae contribute to blue carbon? An Australian perspective. Limnology and Oceanography 60, 1689–1706. doi: 10.1002/lno.10128
Hossain, M., Rogers, K., and Saintilan, N. (2010). Variation in seagrass biomass estimates in low and high density settings: implications for the selection of sample size. Environ. Indicat. 5, 17–27.
Hossain, M. S., Bujang, J. S., Zakaria, M. H., and Hashim, M. (2015). The application of remote sensing to seagrass ecosystems: an overview and future research prospects. International Journal of … 36, 61–113. doi: 10.1080/01431161.2014.990649
Howard, J., Sutton-Grier, A., Herr, D., Kleypas, J., Landis, E., Mcleod, E., et al. (2017). Clarifying the role of coastal and marine systems in climate mitigation. Front. Ecol. Environ. 15, 42–50. doi: 10.1002/fee.1451
Hu, C., Feng, L., Hardy, R. F., and Hochberg, E. J. (2015). Spectral and spatial requirements of remote measurements of pelagic Sargassum macroalgae. Remote Sens. Environ. 167, 229–246. doi: 10.1016/j.rse.2015.05.022
Hu, C., Murch, B., Barnes, B. B., Wang, M., Maréchal, J.-P., Franks, J., et al. (2016). Sargassum watch warns of incoming seaweed. EOS 97, 10–15. doi: 10.1029/2016EO058355
Hu, L., Hu, C., and Ming-Xia, H. E. (2017). Remote estimation of biomass of Ulva prolifera macroalgae in the Yellow Sea. Remote Sens. Environ. 192, 217–227. doi: 10.1016/j.rse.2017.01.037
Hu, P., Liu, Y., Hou, Y., and Yin, Y. (2018). An early forecasting method for the drift path of green tides: a case study in the Yellow Sea, China. Int. J. Appl. Earth Observ. Geoinform. 71, 121–131. doi: 10.1016/j.jag.2018.05.001
Huffard, C. L., von Thun, S., Sherman, A. D., Sealey, K., and Smith, K. L. (2014). Pelagic Sargassum community change over a 40-year period: temporal and spatial variability. Mar. Biol. 161, 2735–2751. doi: 10.1007/s00227-014-2539-y
Hughes, A. R., Williams, S. L., Duarte, C. M., Heck, K. L., and Waycott, M. (2009). Associations of concern: declining seagrasses and threatened dependent species. Front. Ecol. Environ. 7, 242–246. doi: 10.1890/080041
Hughes, B. B., Eby, R., Van Dyke, E., Tinker, M. T., Marks, C. I., Johnson, K. S., et al. (2013). Recovery of a top predator mediates negative eutrophic effects on seagrass. Proc. Natl. Acad. Sci. U.S.A. 110, 15313–15318. doi: 10.1073/pnas.1302805110
Izaguirre, C., Méndez, F. J., Menéndez, M., and Losada, I. J. (2011). Global extreme wave height variability based on satellite data. Geophys. Res. Lett. 38:L10607. doi: 10.1029/2011GL047302
Jackson, E. L., Rees, S. E., Wilding, C., and Attrill, M. J. (2015). Use of a seagrass residency index to apportion commercial fishery landing values and recreation fisheries expenditure to seagrass habitat service. Conserv. Biol. 29, 899–909. doi: 10.1111/cobi.12436
Jayathilake, D. R. M., and Costello, M. J. (2018). A modelled global distribution of the seagrass biome. Biol. Conserv. 226, 120–126. doi: 10.1016/j.biocon.2018.07.009
Johannes, R. E., Freeman, M. M. R., and Hamilton, R. J. (2000). Ignore fishers' knowledge and miss the boat. Fish Fish. 1, 257–271. doi: 10.1046/j.1467-2979.2000.00019.x
Johansson, M. L., Alberto, F., Reed, D. C., Raimondi, P. T., Coelho, N. C., Young, M. A., et al. (2015). Seascape drivers of M acrocystis pyrifera population genetic structure in the northeast P acific. Mol. Ecol. 24, 4866–4885. doi: 10.1111/mec.13371
Johnson, C. R., Banks, S. C., Barrett, N. S., Cazassus, F., Dunstan, P. K., Edgar, G. J., et al. (2011). Climate change cascades: Shifts in oceanography, species' ranges and subtidal marine community dynamics in eastern Tasmania. J. Exp. Mar. Biol. Ecol. 400, 17–32. doi: 10.1016/j.jembe.2011.02.032
Johnson, C. R., Koch, M. S., Pedersen, O., and Madden, C. J. (2018). Hypersalinity as a trigger of seagrass (Thalassia testudinum) die-off events in Florida Bay: evidence based on shoot meristem O 2 and H 2 S dynamics. J. Exp. Mar. Biol. Ecol. 504, 47–52. doi: 10.1016/j.jembe.2018.03.007
Johnson, C. R., Ling, S. D., and Strain, E. M. A. (2013). “Is “barrens” habitat good for sea urchins?,” in Echinoderms in a Changing World (Boca Raton, FL), 254–255.
Jones, B. L., Unsworth, R. K. F., McKenzie, L. J., Yoshida, R. L., and Cullen-Unsworth, L. C. (2017). Crowdsourcing conservation: the role of citizen science in securing a future for seagrass. Mar. Pollut. Bull. 134, 210–215. doi: 10.1016/j.marpolbul.2017.11.005
Kaartvedt, S., Rostad, A., Klevjer, T. A., and Staby, A. (2009). Use of bottom-mounted echo sounders in exploring behavior of mesopelagic fishes. Mar. Ecol. Prog. Ser. 395, 109–118. doi: 10.3354/meps08174
Kaldy, J. E. (2014). Effect of temperature and nutrient manipulations on eelgrass Zostera marina L. from the Pacific Northwest, USA. J. Exp. Mar. Biol. Ecol. 453, 108–115. doi: 10.1016/j.jembe.2013.12.020
Kavanaugh, M. T., Oliver, M. J., Chavez, F. P., Letelier, R. M., Muller-Karger, F. E., and Doney, S. C. (2016). Seascapes as a new vernacular for pelagic ocean monitoring, management and conservation. ICES J. Marine Sci. 73, 1839–1850. doi: 10.1093/icesjms/fsw086
Kendrick, G., Aylward, M., Hegge, B., Cambridge, M., Hillman, K., Wyllie, A., et al. (2002). Changes in seagrass coverage in Cockburn Sound, Western Australia between 1967 and 1999. Aquat. Bot. 73, 75–87. doi: 10.1016/S0304-3770(02)00005-0
Kennedy, H., Beggins, J., Duarte, C. M., Fourqurean, J. W., Holmer, M., Marbà, N., et al. (2010). Seagrass sediments as a global carbon sink: Isotopic constraints. Global Biogeochem. Cycles 24:GB4026. doi: 10.1029/2010GB003848
Kerrylee, R., Macreadie, P., Kelleway, J., and Saintilan, N. (2018). Blue carbon in coastal landscapes: a spatial framework for assessment of stocks and additionality. Sustainability Science 14:453–467. doi: 10.1007/s11625-018-0575-0
Kimura, S., Cox, G., Carroll, J., Pengilley, S., and Harmer, A. (2012). Going the Distance: Use of Diver Propulsion Units, Underwater Acoustic Navigation, and Three-Way Wireless Communication to Survey Kelp Forest Habitats. American Academy of Underwater Sciences.
Kindeberg, T., Ørberg, S. B., Röhr, M. E., Holmer, M., and Krause-Jensen, D. (2018). Sediment stocks of carbon, nitrogen, and phosphorus in Danish eelgrass meadows. Front. Mar. Sci. 5:474. doi: 10.3389/fmars.2018.00474
King, S. A., and Lester, J. N. (1995). The value of salt marsh as a sea defence. Mar. Pollut. Bull. 30, 180–189. doi: 10.1016/0025-326X(94)00173-7
Kingsford, M. J., and Choat, J. H. (1985). The fauna associated with drift algae captured with a plankton-mesh purse seine net1. Limnol. Oceanogr. 30, 618–630. doi: 10.4319/lo.1985.30.3.0618
Knowlton, N. (1993). Sibling species in the sea. Annu. Rev. Ecol. Syst. Available online at: http://www.jstor.org/stable/2097177
Knudby, A., and Nordlund, L. (2011). Remote sensing of seagrasses in a patchy multi-species environment. Int. J. Remote Sens. 32, 2227–2244. doi: 10.1080/01431161003692057
Koch, M. S., Schopmeyer, S. A., Kyhn-Hansen, C., Madden, C. J., and Peters, J. S. (2007). Tropical seagrass species tolerance to hypersalinity stress. Aquat. Bot. 86, 14–24. doi: 10.1016/j.aquabot.2006.08.003
Konar, B., Edwards, M. S., and Estes, J. A. (2014). Biological interactions maintain the boundaries between kelp forests and urchin barrens in the Aleutian Archipelago. Hydrobiologia 724, 91–107. doi: 10.1007/s10750-013-1727-y
Konar, B., and Iken, K. (2018). The use of unmanned aerial vehicle imagery in intertidal monitoring. Deep-Sea Res. 147, 79–86. doi: 10.1016/j.dsr2.2017.04.010
Konar, B., Iken, K., Cruz-Motta, J. J., Benedetti-Cecchi, L., Knowlton, A., Pohle, G., et al. (2010). Current patterns of macroalgal diversity and biomass in northern hemisphere rocky shores. PLoS ONE 5. doi: 10.1371/journal.pone.0013195
Kortsch, S., Primicerio, R., Beuchel, F., Renaud, P. E., Rodrigues, J., Lønne, O. J., et al. (2012). Climate-driven regime shifts in Arctic marine benthos. Proc. Natl. Acad. Sci. U.S.A. 109, 14052–14057. doi: 10.1073/pnas.1207509109
Krause-Jensen, D., and Duarte, C. M. (2014). Expansion of vegetated coastal ecosystems in the future Arctic. Front. Mar. Sci. 1:77. doi: 10.3389/fmars.2014.00077
Krause-Jensen, D., and Duarte, C. M. (2016). Substantial role of macroalgae in marine carbon sequestration. Nat. Geosci. 9, 737–738. doi: 10.1038/ngeo2790
Krause-Jensen, D., Greve, T. M., and Nielsen, K. (2005). Eelgrass as a bioindicator under the european water framework directive. Water Resour. Manage 19, 63–75. doi: 10.1007/s11269-005-0293-0
Krause-Jensen, D., Marbà, N., Olesen, B., Sejr, M. K., Christensen, P. B., Rodrigues, J., et al. (2012). Seasonal sea ice cover as principal driver of spatial and temporal variation in depth extension and annual production of kelp in Greenland. Glob. Chang. Biol. 18, 2981–2994. doi: 10.1111/j.1365-2486.2012.02765.x
Krause-Jensen, D., Serrano, O., Apostolaki, E. T., Gregory, D. J., and Duarte, C. M. (2018). Seagrass sedimentary deposits as security vaults and time capsules of the human past. Ambio 48, 325–335. doi: 10.1007/s13280-018-1083-2
Kritzer, J. P., DeLucia, M.-B., Greene, E., Shumway, C., Topolski, M. F., Thomas-Blate, J., et al. (2016). The importance of benthic habitats for coastal fisheries. Bioscience 66, 274–284. doi: 10.1093/biosci/biw014
Krumhansl, K. A., Okamoto, D. K., Rassweiler, A., Novak, M., Bolton, J. J., Cavanaugh, K. C., et al. (2016). Global patterns of kelp forest change over the past half-century. Proc. Natl. Acad. Sci. U.S.A. 113, 13785–13790. doi: 10.1073/pnas.1606102113
Krumhansl, K. A., and Scheibling, R. E. (2012). Production and fate of kelp detritus. Mar. Ecol. Prog. Ser. 467, 281–302. doi: 10.3354/meps09940
Lamb, J. B., van de Water, J. A. J. M., Bourne, D. G., Altier, C., Hein, M. Y., Fiorenza, E. A., et al. (2017). Seagrass ecosystems reduce exposure to bacterial pathogens of humans, fishes, and invertebrates. Science 355, 731–733. doi: 10.1126/science.aal1956
Lamit, N., Tanaka, Y., Majid, H., and Link, G. B. (2017). Seagrass diversity in Brunei Darussalam: first records of three species. Sci. Bruneiana 16, 48–52.
Langlois, T. C. P. (2006). Baited underwater video for assessing reef fish populations in marine reserves. SPC Fish. Newslett. 118:53.
Laruelle, G. G., Landschützer, P., Gruber, N., Tison, J. L., Delille, B., and Regnier, P. (2017). Global high-resolution monthly pCO2 climatology for the coastal ocean derived from neural network interpolation. Biogeosciences 14, 4545–4561. doi: 10.5194/bg-14-4545-2017
Lee Long, W. J., McKenzie, L. J., Rasheed, M. A., and Coles, R. G. (1996). “Monitoring Seagrasses in Tropical Ports and Harbours,” in Seagrass Biology: Proceedings of an International Workshop, Rottnest Island, Western Australia 25-29 January, 1996, eds J. Kuo, R. C. Phillips, D. I. Walker, and H. Kirkman (Perth, WA: Faculty of Sciences, The University of Western Australia, 345–350.
Lefcheck, J. S., Hughes, B. B., Johnson, A. J., Pfirrman, B. W., Rasher, D. B., Smyth, A. R., et al. (2019). Are coastal habitats important nurseries? A meta-analysis. Conserv. Lett. e12645. doi: 10.1111/conl.12645
Lindstrom, E., Gunn, J., Fischer, A., McCurdy, A., and Glover, L. K. (2012). A Framework for Ocean Observing. By the Task Team for an Integrated Framework for Sustained Ocean Observing. doi: 10.5270/OceanObs09-FOO
Ling, S. D., Johnson, C. R., Frusher, S. D., and Ridgway, K. R. (2009). Overfishing reduces resilience of kelp beds to climate-driven catastrophic phase shift. Proc. Natl. Acad. Sci. U.S.A. 106, 22341–22345. doi: 10.1073/pnas.0907529106
Ling, S. D., Mahon, I., Marzloff, M. P., Pizarro, O., Johnson, C. R., and Williams, S. B. (2016). Stereo-imaging AUV detects trends in sea urchin abundance on deep overgrazed reefs. Limnol. Oceanogr. 14, 293–304. doi: 10.1002/lom3.10089
Ling, S. D., Scheibling, R. E., Rassweiler, A., Johnson, C. R., Shears, N., Connell, S. D., et al. (2015). Global regime shift dynamics of catastrophic sea urchin overgrazing. Philosoph. Transac. R. Soc. Biol. Sci. 370:20130269. doi: 10.1098/rstb.2013.0269
Lourenco, C. R., Zardi, G. I., McQuaid, C. D., Serrao, E. A., Pearson, G. A., Jacinto, R., et al. (2016). Upwelling areas as climate change refugia for the distribution and genetic diversity of a marine macroalga. J. Biogeogr. 43, 1595–1607. doi: 10.1111/jbi.12744
Macreadie, P., Trevathan-Tackett, S., Skilbeck, C., Sanderman, J., Curlevski, N., Jacobsen, G., et al. (2015). Losses and recovery of organic carbon from a seagrass ecosystem following disturbance. Proc. R. Soc. London B 282, 1–6. doi: 10.1098/rspb.2015.1537
Macreadie, P. I., Jarvis, J., Trevathan-Tackett, S. M., and Bellgrove, A. (2017). “Seagrasses and Macroalgae: Importance, Vulnerability and Impacts,” in Climate Change Impacts on Fisheries and Aquaculture: A Global Analysis (Wiley-Blackwell), 729–770. doi: 10.1002/9781119154051.ch22
Madin, J. S., Baird, A. H., Dornelas, M., and Connolly, S. R. (2014). Mechanical vulnerability explains size-dependent mortality of reef corals. Ecol. Lett. 17, 1008–1015. doi: 10.1111/ele.12306
Mansfield, K. L., and Putman, N. F. (2013). Oceanic habits and habitats. Biol. Sea Turtles 3, 189–211. doi: 10.1201/b13895-9
Mansfield, K. L., Wyneken, J., Porter, W. P., and Luo, J. (2014). First satellite tracks of neonate sea turtles redefine the ‘lost years' oceanic niche. Proce. R. Soc. Lond. B 281:20133039. doi: 10.1098/rspb.2013.3039
Marbà, N., Arias Ortiz, A., Masqué, P., Kendrick, G. A., Mazarrasa, I., Bastyan, G. R., et al. (2015). Impact of seagrass loss and subsequent revegetation on carbon sequestration and stocks. J. Ecol. 103, 296–302. doi: 10.1111/1365-2745.12370
Marbà, N., Krause-Jensen, D., Alcoverro, T., Birk, S., Pedersen, A., Neto, J. M., et al. (2013). Diversity of European seagrass indicators: patterns within and across regions. Hydrobiologia 704, 265–278. doi: 10.1007/s10750-012-1403-7
Markiewicz, A., and Patrick, I. (2015). Developing Monitoring and Evaluation Frameworks. Thousand Oaks, CA: SAGE Publications.
Marsh, H., De'ath, G., Gribble, N., and Lane, B. (2005). Historical marine population estimates: triggers or targets for conservation? The dugongcase study. Ecol. Appl. 15, 481–492. doi: 10.1890/04-0673
Marsh, H., Grech, A., and McMahon, K. (2018). “Dugongs: Seagrass Community Specialists.,” in Seagrasses of Australia (Cham: Springer). doi: 10.1007/978-3-319-71354-0_19
Marsh, H., O'Shea, T., and Reynolds, J. (2011). Ecology and Conservation of the Sirenia: Dugongs and Manatees. No. 18. Cambridge University Press. doi: 10.1017/CBO9781139013277
Martinez, B., Wernberg, T., Radford, B., Thomsen, M. S., Russell, B. D., Connell, S. D., et al. (2018). Distribution models predict large contractions in habitat-forming seaweeds in response to ocean warming. Divers. Distribut. 24, 1350–1366. doi: 10.1111/ddi.12767
Marzinelli, E. M., Campbell, A. H., Valdes, E. Z., Verges, A., Nielsen, S., Wernberg, T., et al. (2015a). Continental-scale variation in seaweed host-associated bacterial communities is a function of host condition, not geography. Environ. Microbiol. 17, 4078–4088. doi: 10.1111/1462-2920.12972
Marzinelli, E. M., Williams, S. B., Babcock, R. C., Barrett, N. S., Johnson, C. R., Jordan, A., et al. (2015b). Large-scale geographic variation in distribution and abundance of australian deep-water kelp forests. PLoS ONE 10:e0118390. doi: 10.1371/journal.pone.0118390
McCarthy, M. J., Radabaugh, K. R., Moyer, R. P., and Muller-Karger, F. E. (2018). Enabling efficient, large-scale high-spatial resolution wetland mapping using satellites. Remote Sens. Environ. 208, 189–201. doi: 10.1016/j.rse.2018.02.021
McKenzie, L., Collier, C., Langlois, L., Yoshida, R., Smith, N., and Waycott, M. (2017). Marine Monitoring Program. Annual Report for Inshore Seagrass Monitoring: 2015 to 2016, Great Barrier Reef Marine Park Authority, Townsville.
McKenzie, L. J., Long, L., and Marina, R. C. B. (2000). Seagrass-watch: community based monitoring of seagrass resources. Biol. Mar. Medit. 7, 393–396.
Mcleod, E., Chmura, G. L., Bouillon, S., Salm, R., Björk, M., Duarte, C. M., et al. (2011). A blueprint for blue carbon: toward an improved understanding of the role of vegetated coastal habitats in sequestering CO 2. Front. Ecol. Environ. 9, 552–560. doi: 10.1890/110004
MEA (2005). Millennium Ecosystem Assessment. Ecosystems and Human Well-being: Synthesis. Washington, DC: Island Press.
Mieszkowska, N., Kendall, M., Hawkins, S., Leaper, R., Williamson, P., Hardman-Mountford, N., et al. (2006). Changes in the range of some common rocky shore species in Britain–a response to climate change? Hydrobiologia 555, 241–251. doi: 10.1007/s10750-005-1120-6
Mieszkowska, N., Sugden, H., Firth, L. B., and Hawkins, S. J. (2014). The role of sustained observations in tracking impacts of environmental change on marine biodiversity and ecosystems. Phil. Trans. R. Soc. A 372 :20130339. doi: 10.1098/rsta.2013.0339
Miloslavich, P., Bax, N. J., Simmons, S. E., Klein, E., Appeltans, W., Aburto-Oropeza, O., et al. (2018). Essential ocean variables for global sustained observations of biodiversity and ecosystem changes. Glob. Chang. Biol. 105, 10456–10418. doi: 10.1111/gcb.14108
Moller, H., Berkes, F., Lyver, P. O., and Kislalioglu, M. (2004). Combining science and traditional ecological knowledge: monitoring populations for co-management. Ecol. Soc. 9:2. doi: 10.5751/ES-00675-090302
Moore, A. M., Ambo-Rappe, R., and Ali, Y. (2017). “The Lost Princess (putri duyung)” of the small islands: dugongs around sulawesi in the anthropocene. Front. Mar. Sci. 4:284. doi: 10.3389/fmars.2017.00284
Moore, K. A., and Jarvis, J. C. (2008). Environmental factors affecting recent summertime eelgrass diebacks in the lower chesapeake bay: implications for long-term persistence. J. Coastal Res. 55, 135–147. doi: 10.2112/SI55-014
Muller-Karger, F. E., Miloslavich, P., Bax, N. J., Simmons, S., Costello, M. J., Sousa-Pinto, I., et al. (2018). Advancing marine biological observations and data requirements of the complementary essential ocean variables (EOVs) and essential biodiversity variables (EBVs) frameworks. Front. Marine Sci. 5:e0141039–e0141015. doi: 10.3389/fmars.2018.00211
Murfitt, S. L., Allan, B. M., Bellgrove, A., Rattray, A., Young, M. A., and Ierodiaconou, D. (2017). Applications of unmanned aerial vehicles in intertidal reef monitoring. Sci. Rep. 7:10259. doi: 10.1038/s41598-017-10818-9
Neckles, H. A., Kopp, B. S., Peterson, B. J., and Pooler, P. S. (2012). Integrating scales of seagrass monitoring to meet conservation needs. Estuar. Coasts 35, 23–46. doi: 10.1007/s12237-011-9410-x
Neiva, J., Assis, J., Coelho, N. C., Fernandes, F., Pearson, G. A., and Serrao, E. A. (2015). Genes left behind: climate change threatens cryptic genetic diversity in the canopy-forming seaweed bifurcaria bifurcata. PLoS ONE 10:e0131530. doi: 10.1371/journal.pone.0131530
Neiva, J., Serrão, E. A., Anderson, L., Raimondi, P. T., Martins, N., Gouveia, L., et al. (2017). Cryptic diversity, geographical endemism and allopolyploidy in NE Pacific seaweeds. BMC Evol. Biol. 17:30. doi: 10.1186/s12862-017-0878-2
Nelleman, C. (2009). BLUE CARBON. The Role of Healthy Oceans in Binding Carbon. United Nations Environment Programme, 1–80.
Neori, A., Chopin, T., Troell, M., Buschmann, A. H., Kraemer, G. P., Halling, C., et al. (2004). Integrated aquaculture: rationale, evolution and state of the art emphasizing seaweed biofiltration in modern mariculture. Aquaculture 231, 361–391. doi: 10.1016/j.aquaculture.2003.11.015
Nicastro, K. R., Zardi, G. I., Teixeira, S., Neiva, J., Serrao, E. A., and Pearson, G. A. (2013). Shift happens: trailing edge contraction associated with recent warming trends threatens a distinct genetic lineage in the marine macroalga Fucus vesiculosus. BMC Biol. 11:6. doi: 10.1186/1741-7007-11-6
Nijland, W., Reshitnyk, L., and Rubidge, E. (2019). Satellite remote sensing of canopy-forming kelp on a complex coastline: a novel procedure using the Landsat image archive. Remote Sens. Environ. 220, 41–50. doi: 10.1016/j.rse.2018.10.032
NOAA (1996). Magnuson-Stevens Fishery Conservation and Management Act, as Amended Through Oct. 11, 1996. NOAA Tech. Mem. NMFS-F/SPO-23. 121 p.
Norderhaug, K. M., Gundersen, H., Pedersen, A., Moy, F., Green, N., Walday, M. G., et al. (2015). Effects of climate and eutrophication on the diversity of hard bottom communities on the Skagerrak coast 1990-2010. Mar. Ecol. Prog. Ser. 530, 29–46. doi: 10.3354/meps11306
Nordlund, L., Unsworth, R. K. F., Gullström, M., and Cullen-Unsworth, L. C. (2018). Global significance of seagrass fishery activity. Fish Fish. 19, 399–412. doi: 10.1111/faf.12259
Nordlund, L. M., Koch, E. W., Barbier, E. B., and Creed, J. C. (2016). Seagrass ecosystem services and their variability across genera and geographical regions. PLoS ONE 11:e0163091–e0163023. doi: 10.1371/journal.pone.0163091
O'Brien, J. M., Scheibling, R. E., and Krumhansl, K. A. (2015). Positive feedback between large-scale disturbance and density-dependent grazing decreases resilience of a kelp bed ecosystem. Mar. Ecol. Prog. Ser. 522, 1–13. doi: 10.3354/meps11193
Oliver, E. C. J., Donat, M. G., Burrows, M. T., Moore, P. J., Smale, D. A., Alexander, L. V., et al. (2018). Longer and more frequent marine heatwaves over the past century. Nat. Commun. 9:1324. doi: 10.1038/s41467-018-03732-9
Olsen, J. L., Stam, W. T., Coyer, J. A., Reusch, T. B., Billingham, M., Boström, C., et al. (2004). North Atlantic phylogeography and large-scale population differentiation of the seagrass Zostera marina L. Mol. Ecol. 13, 1923–1941. doi: 10.1111/j.1365-294X.2004.02205.x
Orth, R., Carruthers, T., Dennison, W., Duarte, C., Fourqurean, J., Heck, K. Jr, et al. (2006). A global crisis for seagrass ecosystems. Bioscience 56, 987–996. doi: 10.1641/0006-3568(2006)56<987:AGCFSE>2.0.CO;2
Orth, R., and Van Montfrans, J. (1984). Epiphyte-seagrass relationships with an emphasis on the role of micrograzing: a review. Aquat. Bot. 18, 43–69. doi: 10.1016/0304-3770(84)90080-9
Östman, Ö., Eklöf, J., Eriksson, B. K., Olsson, J., Moksnes, P.-O., and Bergström, U. (2016). Top-down control as important as eutrophication effects in North Atlantic coastal ecosystems. J. Appl. Ecol. 53, 1138–1147. doi: 10.1111/1365-2664.12654
Patrick, W. S., and Link, J. S. (2015). Myths that continue to impede progress in ecosystem-based fisheries management. Fisheries 40, 155–160. doi: 10.1080/03632415.2015.1024308
Pauly, D. (1995). Anecdotes and the shifting baseline syndrome of fisheries. Trends Ecol. Evol. 10:430. doi: 10.1016/S0169-5347(00)89171-5
Pawlowski, J., Christen, R., Lecroq, B., Bachar, D., Shahbazkia, H. R., Amaral-Zettler, L., et al. (2011). Eukaryotic richness in the abyss: insights from pyrotag sequencing. PLoS ONE 6:e18169. doi: 10.1371/journal.pone.0018169
Pendleton, L., Donato, D. C., Murray, B. C., Crooks, S., Jenkins, W. A., Sifleet, S., et al. (2012). Estimating global “blue carbon” emissions from conversion and degradation of vegetated coastal ecosystems. PLoS ONE 7:e43542. doi: 10.1371/journal.pone.0043542
Pereira, H. M., Ferrier, S., Walters, M., Geller, G. N., Jongman, R. H. G., Scholes, R. J., et al. (2013). Essential biodiversity variables. Science 339, 277–278. doi: 10.1126/science.1229931
Perry, C. J., and Dennison, W. C. (1999). Microbial nutrient cycling in seagrass sediment. J. Aust. Geol. Geophys. 17, 227–231.
Perry, D. S. T. (2018). Habitat connectivity of fish in temperate shallow-water seascapes. Front. Mar. Sci. 4:440. doi: 10.3389/fmars.2017.00440
Personnic, S., Boudouresque, C. F., Astruch, P., Ballesteros, E., Blouet, S., Bellan-Santini, D., et al. (2014). An ecosystem-based approach to assess the status of a Mediterranean ecosystem, the Posidonia oceanica seagrass meadow. PLoS ONE 9:e98994. doi: 10.1371/journal.pone.0098994
Polidoro, B. A., Carpenter, K. E., Collins, L., Duke, N. C., Ellison, A. M., Ellison, J. C., et al. (2010). The loss of species: mangrove extinction risk and geographic areas of global concern. PLoS ONE 5:e10095. doi: 10.1371/journal.pone.0010095
Powers, S. P., Hernandez, F. J., Condon, R. H., Drymon, J. M., and Free, C. M. (2013). Novel pathways for injury from offshore oil spills: direct, sublethal and indirect effects of the deepwater horizon oil spill on pelagic sargassum communities. PLoS ONE 8:e74802. doi: 10.1371/journal.pone.0074802
Putman, N. F., Abreu-Grobois, F. A., Iturbe-Darkistade, I., Putman, E. M., Richards, P. M., and Verley, P. (2015). Deepwater Horizon oil spill impacts on sea turtles could span the Atlantic. Biol. Lett. 11:20150596. doi: 10.1098/rsbl.2015.0596
Putman, N. F., Goni, G. J., Gramer, L. J., Hu, C., Johns, E. M., Trinanes, J., et al. (2018). Simulating transport pathways of pelagic Sargassum from the equatorial atlantic into the Caribbean Sea. Prog. Oceanogr. 165, 205–214. doi: 10.1016/j.pocean.2018.06.009
Putman, N. F., and Mansfield, K. L. (2015). Direct evidence of swimming demonstrates active dispersal in the sea turtle “lost years.” Curr. Biol. 25, 1221–1227. doi: 10.1016/j.cub.2015.03.014
Putman, N. F., Verley, P., Shay, T. J., and Lohmann, K. J. (2012). Simulating transoceanic migrations of young loggerhead sea turtles: merging magnetic navigation behavior with an ocean circulation model. J. Exp. Biol. 215, 1863–1870. doi: 10.1242/jeb.067587
Qi, L., Hu, C., Wang, M., Shang, S., and Wilson, C. (2017). Floating algae blooms in the East China Sea. Geophys. Res. Lett. 44, 11,501–11,509. doi: 10.1002/2017GL075525
Rapport, D., Gaudet, C., Constanza, R., Epstein, R., and Levins, R. (1998). “Defining Ecosystem Health,” in Ecosystem Health: Principles and Practice, eds D. Rapport, C. Gaudet, R. Constanza, R. Epstein, and R. Levins (Oxford: Blackwell Science, 18–33.
Rebours, C., Marinho-Soriano, E., Zertuche-Gonzalez, J. A., Hayashi, L., Vasquez, J. A., Kradolfer, P., et al. (2014). Seaweeds: an opportunity for wealth and sustainable livelihood for coastal communities. J. Appl. Phycol. 26, 1939–1951. doi: 10.1007/s10811-014-0304-8
Reef, R., Atwood, T. B., Samper-Villarreal, J., Adame, M. F., Sampayo, E. M., and Lovelock, C. E. (2017). Using eDNA to determine the source of organic carbon in seagrass meadows. Limnol. Oceanogr. 62, 1254–1265. doi: 10.1002/lno.10499
Reusch, T. B. H., Ehlers, A., Hämmerli, A., and Worm, B. (2005). Ecosystem recovery after climatic extremes enhanced by genotypic diversity. Proc. Natl. Acad. Sci. U.S.A. 102, 2826–2831. doi: 10.1073/pnas.0500008102
Rindi, L., Dal Bello, M., Dai, L., Gore, J., and Benedetti-Cecchi, L. (2017). Direct observation of increasing recovery length before collapse of a marine benthic ecosystem. Nat. Ecol. Evol. 1:0153. doi: 10.1038/s41559-017-0153
Robblee, M. B., Barber, T. R., Carlson, P. R Jr, Durako, M. J., Fourqurean, J. W., Muehlstein, L. K., et al. (1991). Mass mortality of the tropical seagrass Thalassia testudinum in Florida Bay (USA). Mar Ecol Progr Seri. 71, 297–299. doi: 10.3354/meps071297
Roca, G., Alcoverro, T., Krause-Jensen, D., Balsby, T. J. S., van Katwijk, M. M., Marba, N., et al. (2016). Response of seagrass indicators to shifts in environmental stressors: a global review and management synthesis. Ecol. Indic. 63, 310–323. doi: 10.1016/j.ecolind.2015.12.007
Röhr, M. E., Holmer, M., Baum, J. K., Björk, M., Chin, D., Chalifour, L., et al. (2018). Blue carbon storage capacity of temperate eelgrass (Zostera marina) meadows. Glob. Biogeochem. Cycles 32, 1457. doi: 10.1029/2018GB005941
Ruiz-Frau, A., Gelcich, S., Hendriks, I. E., Duarte, C. M., and Marba, N. (2017). Current state of seagrass ecosystem services: research and policy integration. Ocean Coastal Manage. 149, 107–115. doi: 10.1016/j.ocecoaman.2017.10.004
Rupprecht, F., Möller, I., Paul, M., Kudella, M., Spencer, T., Van Wesenbeeck, B. K., et al. (2017). Vegetation-wave interactions in salt marshes under storm surge conditions. Ecol. Eng. 100, 301–315. doi: 10.1016/j.ecoleng.2016.12.030
Schell, J., Goodwin, D., and Siuda, A. (2015). Recent sargassum inundation events in the caribbean: shipboard observations reveal dominance of a previously rare form. Oceanography 8, 8–10. doi: 10.5670/oceanog.2015.70
Schultz, S. T., Kruschel, C., Bakran-Petricioli, T., and Petricioli, D. (2015). Error, power, and blind sentinels: the statistics of seagrass monitoring. PLoS ONE 10:e0138378. doi: 10.1371/journal.pone.0138378
Seddon, S., Connolly, R. M., and Edyvane, K. S. (2000). Large-scale seagrass dieback in northern Spencer Gulf, South Australia. Aquat. Bot. 66, 297–310. doi: 10.1016/S0304-3770(99)00080-7
Setyawidati, N., Kaimuddin, A. H., Wati, I. P., Helmi, M., Widowati, I., Rossi, N., et al. (2018). Percentage cover, biomass, distribution, and potential habitat mapping of natural macroalgae, based on high-resolution satellite data and in situ monitoring, at Libukang Island, Malasoro Bay, Indonesia. J. Appl. Phycol. 30, 159–171. doi: 10.1007/s10811-017-1208-1
Short, F., and Burdick, D. (1996). Quantifying eelgrass habitat loss in relation to housing development and nitrogen loading in Waquoit Bay, Massachusetts. Estuar. Coasts 19, 730–739. doi: 10.2307/1352532
Short, F., Carruthers, T., Dennison, W., and Waycott, M. (2007). Global seagrass distribution and diversity: a bioregional model. J. Exp. Mar. Biol. Ecol. 350, 3–20. doi: 10.1016/j.jembe.2007.06.012
Short, F., McKenzie, L. J., Coles, R., Vidler, K., and Gaeckle, J. (2006). SeagrassNet Manual for Scientific Monitoring of Seagrass Habitat, Worldwide Edition. Durham, NH: The University of New Hampshire.
Short, F., and Neckles, H. (1999). The effects of global climate change on seagrasses. Aquat. Bot. 63, 169–196. doi: 10.1016/S0304-3770(98)00117-X
Short, F. T., Coles, R., Fortes, M. D., Victor, S., Salik, M., Isnain, I., et al. (2014). Monitoring in the Western Pacific region shows evidence of seagrass decline in line with global trends. Mar. Pollut. Bull. 83, 408–416. doi: 10.1016/j.marpolbul.2014.03.036
Short, F. T., and Coles, R. G. (2001). Global Seagrass Research Methods, 33. Available online at: http://www.worldcat.org/title/global-seagrass-research-methods/oclc/935245623
Short, F. T., Kosten, S., Morgan, P. A., Malone, S., and Moore, G. E. (2016). Impacts of climate change on submerged and emergent wetland plants. Aquat. Bot. 135, 3–17. doi: 10.1016/j.aquabot.2016.06.006
Short, F. T., Polidoro, B., Livingstone, S. R., Carpenter, K. E., Bandeira, S., Bujang, J. S., et al. (2011). Extinction risk assessment of the world's seagrass species. Biol. Conserv. 144, 1961–1971. doi: 10.1016/j.biocon.2011.04.010
Sievers, M., Brown, C. J., Tulloch, V. J., Pearson, R. M., Haig, J. A., Turschwell, M. P., et al. (2019). The role of vegetated coastal wetlands for marine megafauna conservation. Trends Ecol. Evol. doi: 10.1016/j.tree.2019.04.004. [Epub ahead of print].
Smith, T. M., York, P. H., Broitman, B. R., Thiel, M., Hays, G. C., van Sebille, E., et al. (2018). Rare long-distance dispersal of a marine angiosperm across the Pacific Ocean. Glob. Ecol. Biogeogr. 27, 487–496. doi: 10.1111/geb.12713
Steneck, R. S., and Johnson, C. R. (2013). “Kelp forests: dynamic patterns, processes and feedbacks,” in Marine Community Ecology, eds M. Bertness, B. Silliman, and J. Stachowitz (Sunderland, MA: Sinaur Associates Inc., 315–336.
Strain, E. M., Thomson, R. J., Micheli, F., Mancuso, F. P., and Airoldi, L. (2014). Identifying the interacting roles of stressors in driving the global loss of canopy-forming to mat-forming algae in marine ecosystems. Glob. Change Biol. 20, 3300–3312. doi: 10.1111/gcb.12619
Sullivan, B. K., Trevathan-Tackett, S. M., Neuhauser, S., and Govers, L. L. (2017). Review: Host-pathogen dynamics of seagrass diseases under future global change. Mar. Pollut. Bull. 134, 75–88. doi: 10.1016/j.marpolbul.2017.09.030
Sutton-Grier, A. E., Wowk, K., and Bamford, H. (2015). Future of our coasts: the potential for natural and hybrid infrastructure to enhance the resilience of our coastal communities, economies and ecosystems. Environ. Sci. Policy 51, 137–148. doi: 10.1016/j.envsci.2015.04.006
Taberlet, P., Coissac, E., Pompanon, F., Brochmann, C., and Willerslev, E. (2012). Towards next-generation biodiversity assessment using DNA metabarcoding. Mol. Ecol. 21, 2045–2050. doi: 10.1111/j.1365-294X.2012.05470.x
Tano, S., Eggertsen, M., Wikstrom, S. A., Berkstrom, C., Buriyo, A. S., and Hailing, C. (2016). Tropical seaweed beds are important habitats for mobile invertebrate epifauna. Estuar. Coast. Shelf Sci. 183, 1–12. doi: 10.1016/j.ecss.2016.10.010
Tano, S. A., Eggertsen, M., Wikstrom, S. A., Berkstrom, C., Buriyo, A. S., and Halling, C. (2017). Tropical seaweed beds as important habitats for juvenile fish. Mar. Freshw. Res. 68, 1921–1934. doi: 10.1071/MF16153
Teagle, H., Hawkins, S. J., Moore, P. J., and Smale, D. A. (2017). The role of kelp species as biogenic habitat formers in coastal marine ecosystems. J. Exp. Mar. Biol. Ecol. 492, 81–98. doi: 10.1016/j.jembe.2017.01.017
Tegner, M., and Dayton, P. K. (2000). Ecosystem effects of fishing in kelp forest communities. ICES J. Mar. Sci. 57, 579–589. doi: 10.1006/jmsc.2000.0715
Thibaut, T., Blanfuné, A., Boudouresque, C. F., Personnic, S., Ruitton, S., Ballesteros, E., et al. (2017). An ecosystem-based approach to assess the status of Mediterranean algae-dominated shallow rocky reefs. Mar. Pollut. Bull. 117, 311–329. doi: 10.1016/j.marpolbul.2017.01.029
Thibaut, T., Pinedo, S., Torras, X., and Ballesteros, E. (2005). Long-term decline of the populations of Fucales (Cystoseira spp. and Sargassum spp.) in the Alberes coast (France, North-western Mediterranean). Mar. Pollut. Bull. 50, 1472–1489. doi: 10.1016/j.marpolbul.2005.06.014
Thomsen, P. F., and Willerslev, E. (2015). Environmental DNA – An emerging tool in conservation for monitoring past and present biodiversity. Biol. Conserv. 183, 4–18. doi: 10.1016/j.biocon.2014.11.019
Titilola, S. (1990). The Economics of Incorporating Indigenous Knowledge Systems Into Agricultural Development: A Model And Analytical Framework. Studies in technology and social change (USA). Available online at: http://agris.fao.org/agris-search/search.do?recordID=US9404376
Tol, S. J., Jarvis, J. C., York, P. H., Grech, A., Congdon, B. C., and Coles, R. G. (2017). Long distance biotic dispersal of tropical seagrass seeds by marine mega-herbivores. Sci. Rep. 7:4458. doi: 10.1038/s41598-017-04421-1
Traganos, D., and Reinartz, P. (2018). Mapping Mediterranean seagrasses with Sentinel-2 imagery. Mar. Pollut. Bull. 134, 197–209. doi: 10.1016/j.marpolbul.2017.06.075
Travis, J., Coleman, F. C., Auster, P. J., Cury, P. M., Estes, J. A., Orensanz, J., et al. (2014). Integrating the invisible fabric of nature into fisheries management. Proc. Natl. Acad. Sci. U.S.A. 111, 581–584. doi: 10.1073/pnas.1305853111
Trevathan-Tackett, S. M., Kelleway, J., Macreadie, P. I., Beardall, J., Ralph, P., and Bellgrove, A. (2015). Comparison of marine macrophytes for their contributions to blue carbon sequestration. Ecology 96, 3043–3057. doi: 10.1890/15-0149.1
Uhl, F., Bartsch, I., and Oppelt, N. (2016). Submerged kelp detection with hyperspectral data. Remote Sens. 8:487. doi: 10.3390/rs8060487
Underwood, A. J. (1994). On beyond baci - sampling designs that might reliably detect environmental disturbances. Ecol. Appl. 4, 3–15. doi: 10.2307/1942110
UNEP (2006). Marine and Coastal Ecosystems and Human Well-Being: A Synthesis Report Based on the Findings of the Millennium Ecosystem Assessment. United Nations Environmental Programme.
Unsworth, R., Hinder, S. L., Bodger, O. G., and Cullen-Unsworth, L. C. (2014). Food supply depends on seagrass meadows in the coral triangle. Environ. Res. Lett. 9. doi: 10.1088/1748-9326/9/9/094005
Unsworth, R., Nordlund, L. M., and Cullen-Unsworth, L. C. (2018). Seagrass meadows support global fisheries production. Conserv. Lett. 8:e12566 doi: 10.1111/conl.12566
Unsworth, R., Rasheed, M. A., and Taylor, H. A. (2009). Port of Townsville Long Term Seagrass Monitoring, October 2008. DEEDI Publication PR09-4330. Cairns: QPIF.
Unsworth, R. K., Ambo-Rappe, R., Jones, B. L., La Nafie, Y. A., Irawan, A., Hernawan, U. E., et al. (2018). Indonesia's globally significant seagrass meadows are under widespread threat. Sci. Total Environ. 634, 279–286. doi: 10.1016/j.scitotenv.2018.03.315
Valero, M., Guillemin, M.-L., Destombe, C., Jacquemin, B., Gachon, C. M., Badis, Y., et al. (2017). Perspectives on domestication research for sustainable seaweed aquaculture. Perspect. Phycol. 33–46. doi: 10.1127/pip/2017/0066
Valiela, I., and Cole, M. (2002). Comparative evidence that salt marshes and mangroves may protect seagrass meadows from land-derived nitrogen loads. Ecosystems 5, 92–102. doi: 10.1007/s10021-001-0058-4
van Dijk, K., Bricker, E., van Tussenbroek, B. I., and Waycott, M. (2018). Range-wide population genetic structure of the Caribbean marine angiosperm Thalassia testudinum. Ecol. Evol. 8, 9478–9490. doi: 10.1002/ece3.4443
van Tussenbroek, B. I., Arana, H. A. H., Rodríguez-Martínez, R. E., Espinoza-Avalos, J., Canizales-Flores, H. M., González-Godoy, C. E., et al. (2017). Severe impacts of brown tides caused by Sargassum spp. on near-shore Caribbean seagrass communities. Mar. Poll. Bull. 122, 272–281. doi: 10.1016/j.marpolbul.2017.06.057
van Tussenbroek, B. I., Cortés, J., Collin, R., Fonseca, A. C., Gayle, P. M. H., Guzmán, H. M., et al. (2014). Caribbean-wide, long-term study of seagrass beds reveals local variations, shifts in community structure and occasional collapse. PLoS ONE 9:e90600. doi: 10.1371/journal.pone.0090600
Vásquez, J., Zuñiga, S., Tala, F., Piaget, N., Rodríguez, D., and Vega, J. M. A. (2014). Economic valuation of kelp forests in northern Chile: values of goods and services of the ecosystem. J. Appl. Phycol. 26, 1081–1088. doi: 10.1007/s10811-013-0173-6
Vassallo, P., Paoli, C., Rovere, A., Montefalcone, M., Morri, C., and Bianchi, C. N. (2013). The value of the seagrass Posidonia oceanica: a natural capital assessment. Mar. Pollut. Bull. 75, 157–167. doi: 10.1016/j.marpolbul.2013.07.044
Vergés, A., Steinberg, P. D., Hay, M. E., Poore, A. G. B., Campbell, A. H., Ballesteros, E., et al. (2014). The tropicalization of temperate marine ecosystems: climate-mediated changes in herbivory and community phase shifts. Proc. R. Soc. B 281:20140846. doi: 10.1098/rspb.2014.0846
Villon, S., Chaumont, M., Subsol, G., Villéger, S., Claverie, T., and Mouillot, D. (2016). “Coral reef fish detection and recognition in underwater videos by supervised machine learning: comparison between deep learning and HOG+SVM methods,” in Advanced Concepts for Intelligent Vision Systems, eds J. Blanc-Talon, C. Distante, W. Philips, D. Popescu, and P. Scheunders (Springer International Publishing), 160–171. doi: 10.1007/978-3-319-48680-2_15
Vonk, J. A., Christianen, M. J. A., Stapel, J., and O'Brien, K. R. (2015). What lies beneath: why knowledge of belowground biomass dynamics is crucial to effective seagrass management. Ecol. Indic. 57, 259–267. doi: 10.1016/j.ecolind.2015.05.008
Walker, D. I., and McComb, A. J. (1990). Salinity response of the seagrass Amphibolis antarctica (Labill.) sonder et aschers.: an experimental validation of field results. Aquat. Bot. 36, 359–366. doi: 10.1016/0304-3770(90)90052-M
Wang, J., and Deng, Z. (2016). Modeling and prediction of oyster norovirus outbreaks along gulf of mexico coast. Environ. Health Perspect. 124, 627–633. doi: 10.1289/ehp.1509764
Wang, M., and Hu, C. (2016). Mapping and quantifying Sargassum distribution and coverage in the Central West Atlantic using MODIS observations. Remote Sens. Environ. 183, 350–367. doi: 10.1016/j.rse.2016.04.019
Wang, M., and Hu, C. (2018). On the continuity of quantifying floating algae of the Central West Atlantic between MODIS and VIIRS. Int. J. Remote Sens. 39, 3852–3869. doi: 10.1080/01431161.2018.1447161
Wang, M., Hu, C., Cannizzaro, J., English, D., Han, X., Naar, D., et al. (2018). Remote sensing of Sargassum biomass, nutrients, and pigments. Geophys. Res. Lett. 45, 12,359–12,367. doi: 10.1029/2018GL078858
Waycott, M., Duarte, C. M., Carruthers, T. J. B., Orth, R. J., Dennison, W. C., Olyarnik, S., et al. (2009). Accelerating loss of seagrasses across the globe threatens coastal ecosystems. Proc. Natl. Acad. Sci. U.S.A. 106, 12377–12381. doi: 10.1073/pnas.0905620106
Waycott, M., McKenzie, L., Mellors, J. E., Ellison, J. C., Sheaves, M. T., Collier, C., et al. (2011). “Vulnerability of mangroves, seagrasses and intertidal flats in the tropical Pacific to climate change,” in Vulnerability of Tropical Pacific Fisheries and Aquaculture to Climate Change, eds D. Johann, J. E. Johnson, and A. J. Hobday (Noumea: Secretariat of the Pacific Community), 297–368.
Wells, R. J., and Rooker, J. R. (2004). Spatial and temporal patterns of habitat use by fishes associated with Sargassum mats in the northwestern Gulf of Mexico. Bull. Mar. Sci. 74, 81–99.
Wernberg, T., Bennett, S., Babcock, R. C., de Bettignies, T., Cure, K., Depczynski, M., et al. (2016). Climate-driven regime shift of a temperate marine ecosystem. Science 353, 169–172. doi: 10.1126/science.aad8745
Wernberg, T., and Filbee-Dexter, K. (2018). Grazers extend blue carbon transfer by slowing sinking speeds of kelp detritus. Sci. Rep. 8:17180. doi: 10.1038/s41598-018-34721-z
Wernberg, T., and Filbee-Dexter, K. (2019). Missing the marine forest for the trees. Mar. Ecol. Progr. Seri. 612, p209–215. doi: 10.3354/meps12867
Wernberg, T., Krumhansl, K., Filbee-Dexter, K., and Pedersen, M. F. (2019). “Status and trends for the world's kelp forests,” in World Seas: an Environmental Evaluation, ed C. Shepherd (Amsterdam: Elsevier), 57–78. doi: 10.1016/B978-0-12-805052-1.00003-6
Wernberg, T., Smale, D. A., and Thomsen, M. S. (2012). A decade of climate change experiments on marine organisms: procedures, patterns and problems. Glob. Chang. Biol. 18, 1491–1498. doi: 10.1111/j.1365-2486.2012.02656.x
Wieczorek, J., Bloom, D., Guralnick, R., Blum, S., Döring, M., Giovanni, R., et al. (2012). Darwin core: an evolving community-developed biodiversity data standard. PLoS ONE 7:e29715. doi: 10.1371/journal.pone.0029715
Wilkinson, M. D., Dumontier, M., Aalbersberg, I. J., Appleton, G., Axton, M., Baak, A., et al. (2016). The FAIR Guiding Principles for scientific data management and stewardship. Sci. Data 3, 160018–160019. doi: 10.1038/sdata.2016.18
Wilmers, C. C., Estes, J. A., Edwards, M., Laidre, K. L., and Konar, B. (2012). Do trophic cascades affect the storage and flux of atmospheric carbon? An analysis of sea otters and kelp forests. Front. Ecol. Environ. 10, 409–415. doi: 10.1890/110176
Witherington, B., Hirama, S., and Hardy, R. (2012). Young sea turtles of the pelagic Sargassum-dominated drift community: Habitat use, population density, and threats. 63, 1–22. doi: 10.3354/meps09970
Woolf, D. K., Challenor, P. G., and Cotton, P. D. (2002). Variability and predictability of the North Atlantic wave climate. J. Geophys. Res. 107:3145. doi: 10.1029/2001JC001124
Wright, I. J., Reich, P. B., Westoby, M., Ackerly, D. D., Baruch, Z., Bongers, F., et al. (2004). The worldwide leaf economics spectrum. Nature 428, 821–827. doi: 10.1038/nature02403
Wyllie-Echeverria, S., Gunnarsson, K., Mateo, M. A., Borg, J. A., Renom, P., Kuo, J., et al. (2002). Protecting the seagrass biome: report from the traditional seagrass knowledge working group. Bull. Mar. Sci. 71, 1415–1416.
Yang, F., Long, L., Sun, X., Wu, H., Li, T., and Xiang, W. (2014). Optimization of medium using response surface methodology for lipid production by Scenedesmus sp. Mar. Drugs 12, 1245–1257. doi: 10.3390/md12031245
Yang, Y. F., Chai, Z. Y., Wang, Q., Chen, W. Z., He, Z. L., and Jiang, S. J. (2015). Cultivation of seaweed Gracilaria in Chinese coastal waters and its contribution to environmental improvements. Algal Res. 9, 236–244. doi: 10.1016/j.algal.2015.03.017
Keywords: biodiversity, seagrass, network, macroalgae, biodiversity observation network (BON), essential ocean variables (EOV)
Citation: Duffy JE, Benedetti-Cecchi L, Trinanes J, Muller-Karger FE, Ambo-Rappe R, Boström C, Buschmann AH, Byrnes J, Coles RG, Creed J, Cullen-Unsworth LC, Diaz-Pulido G, Duarte CM, Edgar GJ, Fortes M, Goni G, Hu C, Huang X, Hurd CL, Johnson C, Konar B, Krause-Jensen D, Krumhansl K, Macreadie P, Marsh H, McKenzie LJ, Mieszkowska N, Miloslavich P, Montes E, Nakaoka M, Norderhaug KM, Norlund LM, Orth RJ, Prathep A, Putman NF, Samper-Villarreal J, Serrao EA, Short F, Pinto IS, Steinberg P, Stuart-Smith R, Unsworth RKF, van Keulen M, van Tussenbroek BI, Wang M, Waycott M, Weatherdon LV, Wernberg T and Yaakub SM (2019) Toward a Coordinated Global Observing System for Seagrasses and Marine Macroalgae. Front. Mar. Sci. 6:317. doi: 10.3389/fmars.2019.00317
Received: 07 November 2018; Accepted: 27 May 2019;
Published: 04 July 2019.
Edited by:
Christos Dimitrios Arvanitidis, Hellenic Centre for Marine Research (HCMR), GreeceReviewed by:
Sotiris Orfanidis, Institute of Fisheries Research, GreeceCopyright © 2019 Duffy, Benedetti-Cecchi, Trinanes, Muller-Karger, Ambo-Rappe, Boström, Buschmann, Byrnes, Coles, Creed, Cullen-Unsworth, Diaz-Pulido, Duarte, Edgar, Fortes, Goni, Hu, Huang, Hurd, Johnson, Konar, Krause-Jensen, Krumhansl, Macreadie, Marsh, McKenzie, Mieszkowska, Miloslavich, Montes, Nakaoka, Norderhaug, Norlund, Orth, Prathep, Putman, Samper-Villarreal, Serrao, Short, Pinto, Steinberg, Stuart-Smith, Unsworth, van Keulen, van Tussenbroek, Wang, Waycott, Weatherdon, Wernberg and Yaakub. This is an open-access article distributed under the terms of the Creative Commons Attribution License (CC BY). The use, distribution or reproduction in other forums is permitted, provided the original author(s) and the copyright owner(s) are credited and that the original publication in this journal is cited, in accordance with accepted academic practice. No use, distribution or reproduction is permitted which does not comply with these terms.
*Correspondence: J. Emmett Duffy, ZHVmZnllQHNpLmVkdQ==
Disclaimer: All claims expressed in this article are solely those of the authors and do not necessarily represent those of their affiliated organizations, or those of the publisher, the editors and the reviewers. Any product that may be evaluated in this article or claim that may be made by its manufacturer is not guaranteed or endorsed by the publisher.
Research integrity at Frontiers
Learn more about the work of our research integrity team to safeguard the quality of each article we publish.