- 1Oceans Graduate School and The UWA Oceans Institute, The University of Western Australia, Crawley, WA, Australia
- 2The Australian Institute of Marine Science, Crawley, WA, Australia
- 3Centre for Sustainable Aquatic Ecosystems, Harry Butler Institute, Murdoch University, Murdoch, WA, Australia
- 4College of Science, Health, Engineering and Education, Environment and Conservation Sciences, Murdoch University, Murdoch, WA, Australia
- 5Hopkins Marine Station, Stanford University, Pacific Grove, CA, United States
An understanding of the role that large marine predators play in structuring trophic flow and nutrient cycling in marine ecosystems requires knowledge of their fine-scale (m-km) movement behaviors. In this study, biologging tags were used to reveal new insights into the three-dimensional fine-scale movement ecology of tiger sharks (Galeocerdo cuvier) at Ningaloo Reef, Western Australia. Tags deployed on 21 sharks in April-May 2017 for durations of 5–48 h recorded both physical parameters such as depth and temperature, and, through the use of accelerometers, gyroscopes and compasses, in-situ measurements of animal trajectory and locomotion. Animal-borne-video enabled the validation of behavioral signatures, mapping of habitat, and recording of interactions with prey. Collectively, these data were used to examine the link between vertical (oscillations) and horizontal (tortuosity) movements, and link sensor data to prey interactions recorded by the video. This biologging approach revealed complex movements that would otherwise be invisible within the time-depth records provided by traditional tagging techniques. The rate of horizontal turning was not related to vertical oscillations, suggesting that vertical movements occur independently of searching behaviors in tiger sharks. These animals displayed tortuous movements possibly associated with prey searching for 27% of their tracks, and interactions with prey elicited varied responses including highly tortuous paths and burst movements. Accurate speed measurements and GPS anchor points will considerably enhance the value of magnetometer data in future studies by facilitating more accurate dead-reckoning and geo-referencing of area-restricted search behaviors.
Introduction
The movement patterns of large predatory marine fishes such as tiger sharks (Galeocerdo cuvier) have typically been sampled using acoustic telemetry and satellite tagging approaches (Andrews et al., 2009; Papastamatiou et al., 2009, 2015; Barnett et al., 2010; Brunnschweiler et al., 2010; Vaudo et al., 2014; Comfort and Weng, 2015; Heupel and Simpfendorfer, 2015). These studies generate either presence/absence data sets (acoustic telemetry), or movement patterns of animals over large horizontal spatial scales (satellite tagging, 10–1,000s km). Although such studies continue to transform our understanding of the ecology and biology of these animals (Chapman et al., 2015), they have two major shortcomings. First, they provide limited opportunities to identify and categorize behavioral modes at the fine spatial scales (m–km) relevant to predator-prey and other inter and intraspecific interactions (competition, cannibalism etc.; Gleiss et al., 2009a). Second, the time-depth records that are usually recorded by these techniques offer only coarse resolution of the patterns of vertical movements of these predators through the water column (Ryan et al., 2004). Given that the behavior of large marine predators is thought to have a major role in structuring trophic flows and nutrient cycling within marine ecosystems (Heithaus et al., 2008; Lavery et al., 2010), it is imperative that we link their large-scale movement patterns with their day-to-day behaviors within the environment.
Biologging approaches offer a means to achieve this aim. Tags such as the Daily Diary (Wilson et al., 2008) incorporate a range of sensors including accelerometers and physical sensors, and can be used to characterize fundamental aspects of the behavior of individuals through quantitative measurement of body kinematics. The combination of these sensors can therefore record detailed movements of a target species as well as the environmental context in which they occur. First used on Adélie penguins in the late 1990s (Yoda et al., 1999), tri-axial accelerometers are often incorporated into biologging devices, and have allowed researchers to categorize the behaviors of sharks, including swimming, bursting, resting, and mating, and have also been used to quantify activity patterns and the swimming energetics of vertical movements (Whitney et al., 2010, 2012, 2018; Gleiss et al., 2011; Meekan et al., 2015). However, to link these fine-scale behaviors to larger-scale movements, we need to understand how they relate to an animal's path through the environment. Accelerometers do not provide information on animal heading, limiting most analysis of behaviors to a two-dimensional plane. Furthermore, the direct observation and consequent validation of behaviors recorded by these sensors is almost impossible for a number of large species of shark due to their high mobility and cryptic nature. The recent addition of magnetometers and animal-borne cameras to biologging tags overcomes these issues. When used in tandem with accelerometers and pressure sensors, tri-axial magnetometers enable the reconstruction of movements in three dimensions through the process of dead-reckoning (Wilson et al., 2008; Walker et al., 2015; Williams et al., 2017). Animal-borne cameras add the ability to validate classifications of behavioral signatures recorded by tri-axial sensors (Davis et al., 1999; Heithaus et al., 2001; Narazaki et al., 2013) and enable interactions with prey to be recorded (Heithaus et al., 2002a; Nakamura et al., 2015; Papastamatiou et al., 2018a).
Here, we use a biologging approach to examine the fine-scale movement and behavior of tiger sharks at Ningaloo Reef, Western Australia. Tiger sharks are a partial migrator, where some individuals remain resident in coastal areas for long periods of time and others undertake long distance movements (Papastamatiou et al., 2013; Ferreira et al., 2015; Acuña-Marrero et al., 2017). Previous studies have revealed that this species continuously oscillates through the water column, presumably to search for benthic prey on descent, and silhouetted air-breathing prey on ascent (Heithaus et al., 2002a; Nakamura et al., 2011).
In this study, we explore the extent to which multiple sensors in biologging tags reveal new insights into the fine-scale movement ecology of tiger sharks. Our tags combined video, environmental sensors, tri-axial accelerometers, magnetometers, and gyroscopes, allowing us to classify behavioral signatures in vertical movements, validated by the video, and reconstruct three-dimensional paths of these animals while concurrently recording the environmental context in which they occurred. This allowed us to (1) examine the relationship between vertical and horizontal movements, and (2) link sensor data, including tailbeats, burst acceleration and tortuosity, to prey interactions recorded on video, collectively identifying likely prey-searching behaviors.
Materials and Methods
Data Collection
Tiger sharks were captured using baited drumlines inside the reef lagoon at Ningaloo, Western Australia (22.99°S, 113.8°E, Figure 1A) in April-May 2017. Drumlines were equipped with a single 20/0 circle hook baited with fish scraps. Three drumlines were deployed ~100 m apart between 07:00 and 16:00, with lines checked every hour for captures. Once a shark was caught, it was secured alongside a 5.8 m vessel with the leader and a tail rope. Each shark was measured (pre-caudal length, fork length, total length and maximum girth) and its sex recorded, before a biologging tag was clamped to the base of its dorsal fin (see below). The dorsal fin of each shark was also photographed before and after tagging for identification purposes and to assess any potential tag effects.
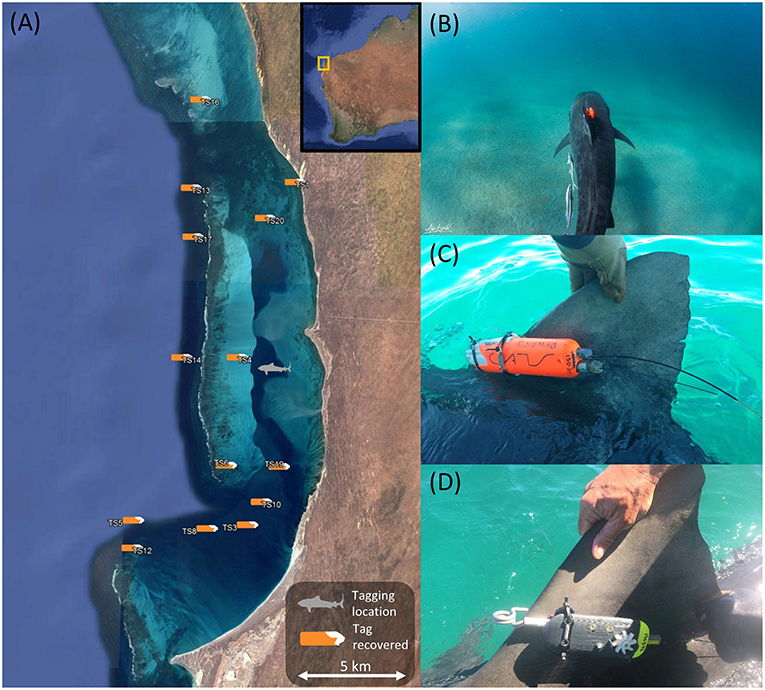
Figure 1. Tagged tiger sharks at Ningaloo Reef. (A) Location of tag deployments and recovery. Eight tags were recovered outside of the bounds of this map to the west and north. (B) Tiger shark post-release. Photo courtesy of Alex Kydd. (C) CATS Cam tag clamped to the dorsal fin. (D) CATS Diary tag clamped to the dorsal fin.
A combination of CATS Diary tags (Customized Animal Tracking Solutions, Australia) and CATS Cam tags were deployed on tiger sharks (Figure 1). Both were equipped with tri-axial accelerometers, magnetometers, and gyroscopes, and depth, temperature and light sensors. The Cam tag additionally housed a HD video camera. Though speed sensors were also present in the Diary tags, they were not functional. The sensors continuously recorded all parameters at 20 Hz, and video was recorded at pre-programmed hours of the day for a maximum of 6 h per deployment due to memory limitations. In order to attach the tags to the dorsal fins of the sharks, CATS tags were joined to a stainless steel spring clamp (CATS, Australia) via docking pin and a corrodible galvanic timed release (GTR, Ocean Appliances, Australia). Previous work has shown that these clamps allow tags to remain rigidly attached to dorsal fins of large sharks for up to 93 h (Gleiss et al., 2009b; Chapple et al., 2015), and the GTR models used were designed to dissolve in seawater after 7–48 h (Table 1). Once the GTR dissolved, the tag released from the clamp, allowing the tag to float to the surface. Floating tag packages were tracked down using a hand-held VHF receiver operated from a vessel (Lear and Whitney, 2016). A magnesium sleeve on the clamp itself also dissolved after ~7 days, so that the clamp detached, leaving no tagging equipment attached to the shark.
Data Processing and Analysis
Depth Record
Zero offset corrections were applied to the depth trace based on periods where sharks were being tagged and known to be at the surface. The depth record was then split into vertical swimming phases (“ascending,” “descending” and “level swimming”) using vertical velocity (VV). To do this, the depth trace was firstly smoothed using a 10 s running mean and the average VV was calculated by taking the difference of this smoothed depth between successive points at 1 s intervals. Ascents and descents were defined where VV exceeded an absolute value of 0.05 m/s for more than 10 s, and level where this value was not exceeded (Whitney et al., 2016).
Tri-axial Sensor Data
Data recorded by the accelerometer (acceleration) and gyroscope (angular velocity) were analyzed using Igor Pro ver. 7.0.4.1 (Wavemetrics, Inc. Lake Oswego, USA) and Ethographer (Sakamoto et al., 2009). The gravitational component of acceleration (static acceleration) was determined using a 3 s box smoothing window on the raw acceleration data (Shepard et al., 2008). Pitch angles were derived by calculating the arcsine of the static acceleration in the surging (posterior-anterior) axis. To correct for the tag attachment angle on each individual shark, we determined the pitch when the shark was swimming at a constant depth (when VV was equal to zero), and subtracted this value from all pitch estimates (Kawatsu et al., 2009). The dynamic component of acceleration was calculated by subtracting the gravitational component from the raw acceleration for each axis. ODBA (overall dynamic body acceleration) was calculated by summing the absolute value of dynamic acceleration from all three axes (Wilson et al., 2006). Comparing ODBA with video recorded burst events allowed us to classify bursts as events where ODBA >0.2. We used a continuous wavelet transformation on the dynamic component of sway (lateral) axis to calculate the acceleration signal amplitude and frequency of tailbeats (Sakamoto et al., 2009). Using these same methods, angular velocity signal amplitude and frequency were calculated using the angular velocity data, and the resulting signals were compared with those derived from the acceleration data to determine the best measure of tailbeat frequency.
Recovery Period
We used metrics quantifying tailbeat activity calculated from tri-axial sensor data to estimate the duration of recovery from stress of capture following the methods of Whitney et al. (2016). This study found that immediately after release, the tailbeats of blacktip sharks Carcharhinus limbatus were elevated, and would slowly decline in frequency over the course of an individual's recovery (mean recovery time of ~10.5 h). Briefly, to calculate this period for tiger sharks, tailbeat cycle (the inverse of tailbeat frequency) throughout descent was summarized for 15 min windows, and plotted against time post-release. A recovery period was defined as the time it took for this metric to reach 80% of its asymptote (Whitney et al., 2016). This was calculated for all tiger sharks, with the exception of two individuals that had tag deployment durations of <5 h. The overall mean recovery period was then eliminated from the tri-axial sensor data prior to further analyses to remove potential sublethal and unnatural behaviors resulting from stress of capture by drumlines.
Shark Heading and Pseudo-Track Calculation
Acceleration and magnetometer data were used to calculate head yaw angle (hereafter referred to as “heading”) and pseudo-tracks in the software Framework 4 (Walker et al., 2015). Heading calculations required input of orientation of the sensors in the tags, and magnetometer and acceleration data. The orientation of the device was corrected using orientation specifications provided by CATS (N. Liebsch pers. comm). The coordinate systems of the accelerometer and magnetometer were perfectly aligned and consequently no adjustment was required to align these sensors. Pitch and roll calculated from the accelerometer data were used to correct for tilt (Walker et al., 2015). In addition to the calculations applied by Framework 4, we applied a 3 s box smoothing window to the heading data to filter out the dynamic movements of the sharks caused by tailbeats.
Dead-reckoning with the heading data was used to calculate horizontal movement tracks for each shark as described in Andrzejaczek et al. (2018). As no speed estimates were available, a constant speed was assumed for all tracks. In addition, no GPS positions were available to anchor each track and to account for cumulative errors derived from both unrepresentative speed estimates and the effect of ocean currents (Wilson et al., 2007; Walker et al., 2015; Andrzejaczek et al., 2018). For these reasons, tracks were used only for plotting short-term movements of the sharks in two- and three-dimensional space, and are subsequently referred to as “pseudo-tracks” for the remainder of the text given they could not be used to accurately estimate animal positions. Heading data were also used to calculate the turning angles of individuals. Data were resampled to a 1 s frequency and converted to a 0–360° scale. The minimum difference in angle between consecutive observations was used to determine turning angle.
Window Size and Statistics
The time window used for analysis was selected by determining the window for which the highest variance in turning angle was observed, while being of sufficient width to capture the longest dives in their entirety. This time window was estimated to be 15 min, and within this period, a number of vertical movement parameters were summarized including mean (± standard deviation) and maximum depth, ascent pitch, descent pitch, ascent VV and descent VV. The percent of time spent moving vertically (ascending and descending), termed the “diving ratio,” was also calculated for each window as per:
This parameter allowed us to differentiate between sampling windows when vertical movements consisted of either continuous oscillations through the water column (diving ratio close to or equal to one), or extended periods of level swimming (diving ratio close to or equal to zero) (Andrzejaczek et al., 2018). In addition, heading was used to estimate path tortuosity and direction. Firstly, turning angles were summed for each window to obtain an estimate of tortuosity. Secondly, the Circular package in R 3.4.0 (R Core Team, 2017) was used to calculate the mean resultant length, or , of the heading, a measure of the concentration of unimodal circular data (Pewsey et al., 2013). When is close to or equal to one, points are closely clustered around the mean direction and are highly directional, and as approaches zero, points spread more evenly in a circle, indicative of a tortuous path.
Video Analysis
Video recorded by the tags was analyzed in BORIS (Behavioral Observation Research Interactive Software; Friard and Gamba, 2016). This open-source software allows the user to set an ethogram and record the timing of behavioral events. We set an ethogram to mark the occurrence of prey interactions, shark responses to the presence of prey, burst swimming events, presence of seabed in the field of view, habitat type, and other notable behaviors (Supplementary Table 1). Video was also used to validate parameters recorded and calculated from the Diary data, such as tailbeat frequency, bursts, and glides.
Movement Classification
Hierarchical cluster analysis was used to categorize both vertical movements and path tortuosity for each analysis window. Vertical movements were classified using mean ascent and descent pitch and diving ratio, and the path tortuosity using the sum of the turning angles and . These variables were scaled using the scale function in R and a cluster analysis was applied using the hclust function, based on a dissimilarity matrix produced from Euclidean distances and complete linkage as the agglomeration method. We chose the number of clusters by looking at the gradient of reduction in within groups sum of squares with additional number of clusters added. Groups resulting from the clustering of vertical movements were labeled as “V-groups” and from the clustering of path tortuosity as “T-groups.” The resulting V-groups were compared through qualitative analysis and correlation coefficients with T-groups to investigate if more tortuous movements were related to more oscillatory movements. Where movements were qualitatively identified to be tortuous, available behavioral records from concurrent videos were examined.
Generalized Linear Mixed Models
To further investigate relationships between oscillatory and tortuous movements, generalized linear mixed models (GLMMs) were built in R using the nlme package (Pinheiro et al., 2017). Diving ratio was set as the response variable, and sum of turning angles were sequentially set as explanatory variables (due to correlation >0.6 between these variables), and tiger shark identity was set as a random variable. Diving ratio was logit transformed prior to analysis. We used the corAR1 function to account for temporal auto-correlation in our datasets (Zuur et al., 2009). The resulting models were compared with null models using Akaike's information criterion (AIC).
Results
A total of 22 tiger sharks ranging in length from 2.65 to 3.80 m TL were caught and tagged (14 Cam tag and 8 Daily Diary tag deployments) (Table 1). Tags were attached for a mean duration of 15 h (range 4.5–48 h) and recorded ~410 h of Diary data and 50 h of video footage. One Diary tag failed to record any data. One shark was recaptured after 11 days and was re-tagged (TS17 and TS24 in Table 1). Based on the analysis of tailbeats, we calculated a mean recovery period from capture and tagging by the sharks of 4 h and for this reason, the first 4 h of each dataset were excluded from further analysis (Supplementary Figure 1). Evidence for recovery after this time was also provided by the video records, which showed investigations of potential prey and consumption of a discarded fish head by sharks within 2 h of tagging and release (Supplementary Video). The angular velocity data produced the clearest tailbeat signal and was therefore used for further data exploration.
Vertical Movements
The seabed was observed in videos at least every 15 min in all but 14 (~2%) sampling windows where the water visibility was very poor, or in one case, during a period of extended surface swimming by a shark while offshore. As a result, we assumed that vertical oscillations were depth-limited and could be used to map the approximate depth of the seabed throughout the tracks (Supplementary Figure 2). Tagged tiger sharks swam at a mean depth of 11.6 ± 17.5 m, predominately remaining in inshore habitats, with four tiger sharks moving into offshore habitats and diving to a maximum depth of 94 m. Video analysis showed tiger sharks transiting a variety of habitats, including sand, macroalgal reefs, coral reef, bare reef, pelagic, and edge habitats (Bancroft, 2003) (Supplementary Video 1).
The cluster analysis revealed six vertical-groupings (V-groups) as the dominant vertical movement modes, with an additional six V-groups describing <8% of the data (Figure 2, Supplementary Table 2). Diving ratio increased from 0.5 ± 0.8% in V-group 1 to 80 ± 6% in Group 6. Groups 4, 5 and 6 were characterized by mean diving ratio and/or mean pitch that were greater than overall mean values, and examination of tracks associated with these groups demonstrated that they were associated with a higher frequency of oscillations throughout the water column. Offshore periods were only clustered into these three V-groups, whereas all modes of vertical movement were displayed by inshore sharks.
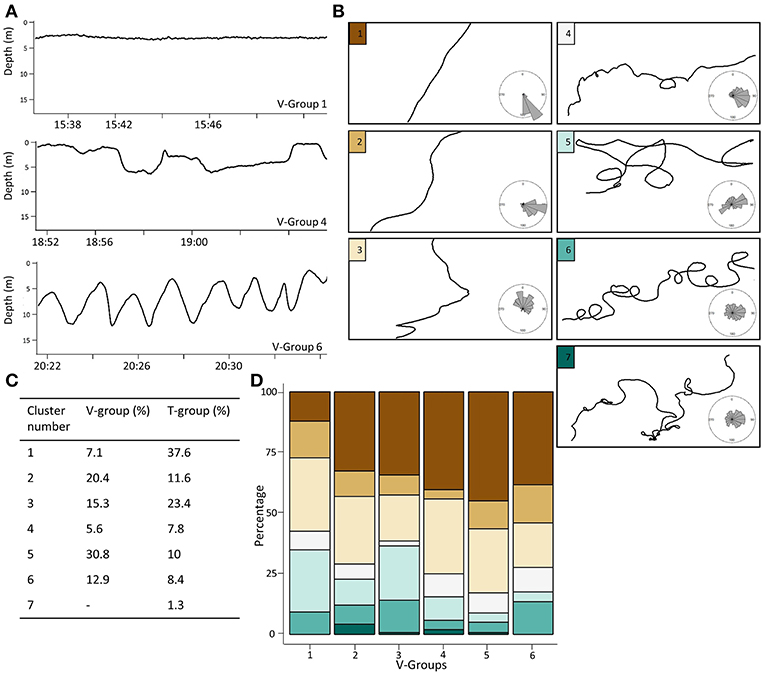
Figure 2. Comparison of dominant vertical movement clusters (V-groups) and path tortuosity clusters (T-groups) from hierarchical cluster analysis. (A) 15 min depth profiles representative of V-groups 1, 4, and 8, demonstrating the increase in oscillatory movements with V-group. (B) 5–15 min pseudo-tracks representative of each T-group, demonstrating the increase in tortuosity with T-group. Each pseudo-plot is displayed in approximately the same amount of area, resulting in directional tracks moving outside of the given area after 5 min. As constant speed was used to estimate pseudo-tracks, we caution that speed changes throughout these windows by tagged sharks may slightly influence the shape of resulting pseudo-tracks. Polar plot on bottom right of each track displays example of heading variance for each group over the entire 15 min window. (C) The % windows found in each cluster. (D) The % T-group composition of each dominant V-group. Colors are T-groups from (B).
Horizontal Path Tortuosity and Prey Interactions
The classification of path tortuosity generated seven tortuosity-groups (T-groups) (Figure 2, Supplementary Table 3). Movements increased in tortuosity from T-groups 1–7, ranging from highly directional with low turning to almost uniform distributions of direction with high levels of turning. A total of 72.6% of windows were classified in T-groups 1–3 where movements were subjectively categorized as more directional and the remaining 27.4% in T-groups 4–7 were categorized as more tortuous. All T-groups were present in both inshore and offshore habitats.
Video data were available for 21 of the windows assessed to have more tortuous paths (T-groups 4–7). Tiger sharks were located on the shallow sandflats (<3 m in depth) for almost half of these windows (43%). Six tortuous windows were associated with investigations of turtles. For example, TS15 was tracking through coral reef habitat when a loggerhead turtle (Caretta caretta) was sighted on the far right of the field of view (Figure 3, Supplementary Video 1). After this first observation, the shark turned sharply and the turtle was again spotted in mid-water, tightly turning away from the shark with its shell perpendicular to the shark. The shark then approached the surface and descended before circling the same area of coral reef for 25 min, remaining on the seabed and following the structure of the reef (Figure 3, Supplementary Video 1). The turtle was not observed again in the field of view for the remainder of this 25 min period.
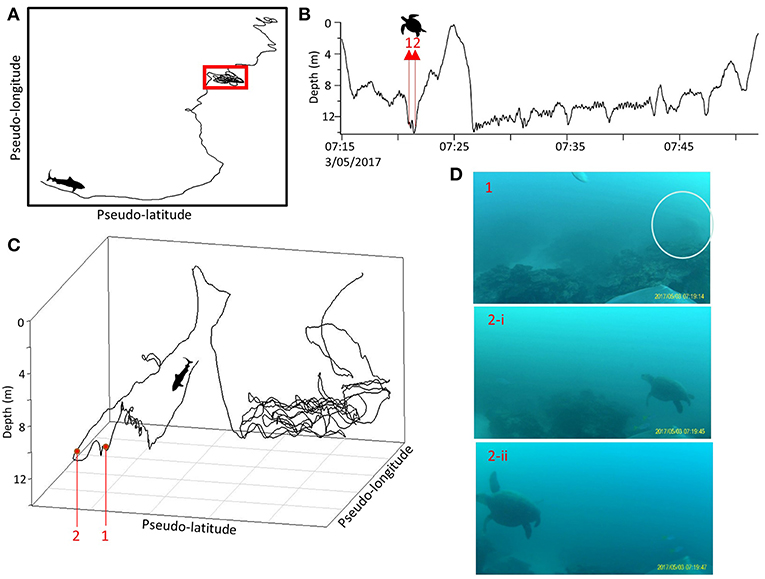
Figure 3. Tiger shark and loggerhead turtle interaction. (A) One-hour long pseudo-track from TS15. Red square denotes 35 min area of prey interaction displayed in (B,C). (B) Depth track. (C) 3D track. (D) Screenshots from video of the interaction. 1 and 2 refer to where interaction takes place in depth and 3D tracks. See Supplementary Video 1 for full video of interaction.
A number of other interactions with prey were recorded on the cameras (Supplementary Table 4), however none of these involved successful foraging on live prey. Most interactions (92%) occurred when sharks were level swimming with the seabed in the field of view of the camera and included investigations and possible stalking of several lutjanid fish (Symphorus nematophorus), in at least one case prompting a rapid color change, 17 interactions with turtles, in at least four cases eliciting tight turning and shell up responses from turtles, and potential investigations of a tawny nurse shark (Nebrius ferrugineus) and another tiger shark (Supplementary Table 4 and Video 1). Several investigations of prey were immediately preceded by burst, stalking and/or turning behaviors (Figure 4). Sharks did not pursue vigilant or fleeing prey, and no bursts were observed in the direction of prey when prey were in the field of view.
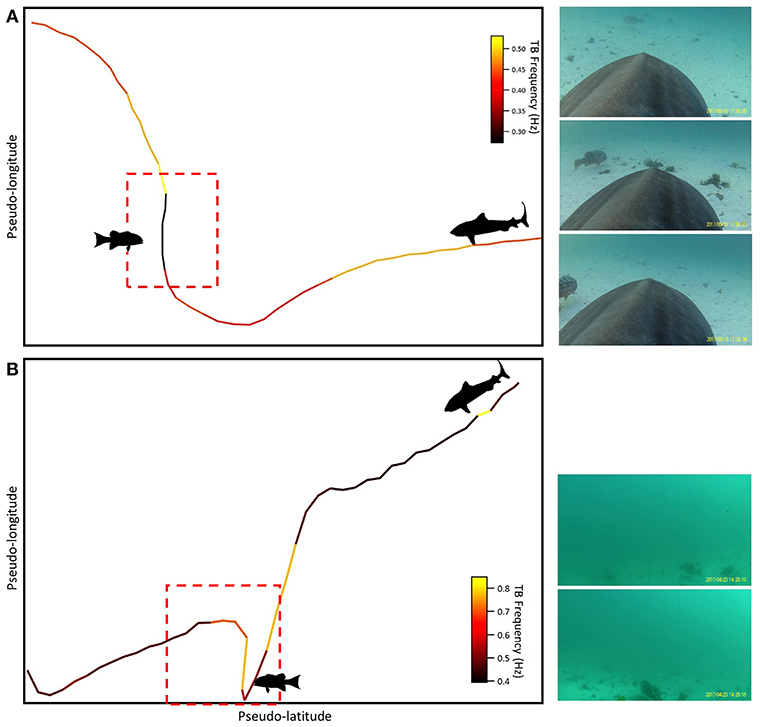
Figure 4. One minute pseudo-tracks of tiger shark interactions with Chinamanfish (Symphorus nematophorus). Track line is colored by tailbeat frequency (Hz). Red dashed square indicates where the fish was observed in the video field of view (displayed in screenshots to the right of the pseudo-track). X and Y-axes represent arbitrary units of latitude and longitude created by magnetometer and accelerometer data whilst a constant speed is assumed. (A) Example of an interaction where the tailbeat of the shark slows upon encountering the fish. (B) Example of an interaction where the tailbeat of the shark quickens upon encountering the fish. As constant speed was used to estimate pseudo-tracks, we caution that speed changes throughout the sampling period by tagged sharks may slightly influence the shape of resulting pseudo-tracks.
No extended periods of bursting that may be indicative of headshaking behavior (bursts >2 s in length; Brewster et al., 2018) were observed in any part of the dataset. Burst behavior was highly variable with a mean 3 ± 15 bursts occurring in a 15 min window. Highly tortuous windows (T-groups 6 and 7) were associated with higher than average bursts (e.g., Figure 5), however further analysis of bursting was confounded by artificially high ODBA levels when swimming at the surface due to the effects of chop.
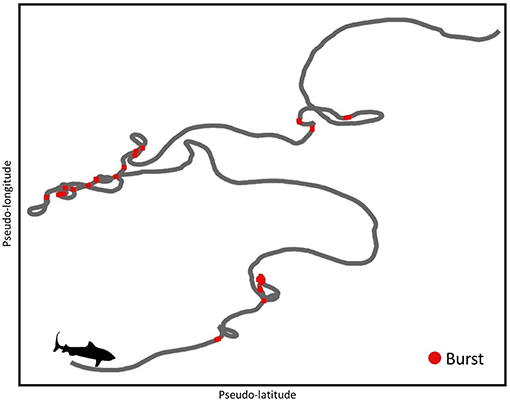
Figure 5. Fifteen minute pseudo-track throughout a tortuous window in TS20. Track is colored by bursts (Overall Dyamic Body Acceleration >0.2) and takes place at ~21:30 in water ~2 m in depth. As constant speed was used to estimate pseudo-tracks, we caution that speed changes throughout these windows by tagged sharks may slightly influence the shape of resulting pseudo-tracks.
Oscillations and Tortuosity
There were no strong correlations between the diving ratio and tortuosity variables ( and the sum of turning angles; all r < 0.3), and almost all vertical movements displayed all levels of tortuosity. For example, highly oscillatory movements classified in V-group 8 were classified in both T-group 1, with directional swimming, and in T-group 6, where movements were determined to be highly tortuous (Figure 6). Conversely, windows containing entirely level swimming in V-group 1 were also classified in both T-groups 1 and 6. In addition, generalized linear mixed models revealed no relationships between diving ratio and variables describing tortuosity ( and sum of turning angles, Table 2).
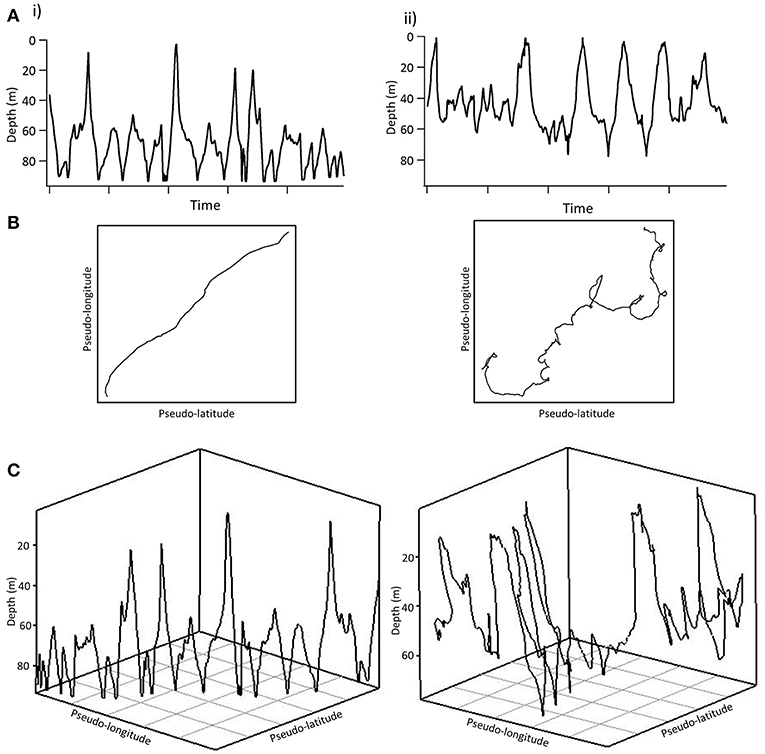
Figure 6. Two tiger sharks (i and ii) with very similar oscillatory depth tracks but different horizontal tracks in offshore environments over a 2.5 h period. (A) Depth track. (B) Pseudo-track. (C) 3D track. Each pseudo-plot and 3D track is displayed in approximately the same amount of horizontal area. As constant speed was used to estimate pseudo-tracks, we caution that speed changes by tagged sharks may slightly influence the shape of resulting pseudo-tracks.
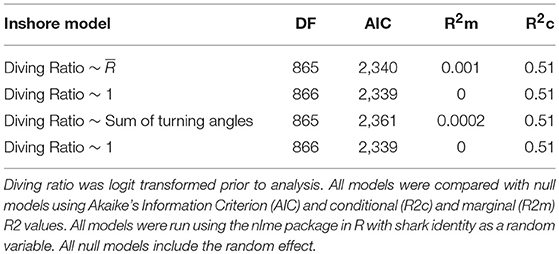
Table 2. Results of generalized linear mixed models testing the relationship between diving ratio and indicators of horizontal path tortuosity.
Discussion
Our study shows how a multi-sensor biologging approach may be used to investigate foraging behavior of sharks as large, top-order predators. This information is essential if we are to understand the keystone role that these animals are thought to play in marine ecosystems.
Foraging Behavior and Tortuosity
We found evidence of foraging behavior based on path tortuosity and video recorded encounters with potential prey. Tiger sharks displayed tortuous horizontal paths for an estimated 27% of their tracks. As straight-line directional movement has been calculated to be the most energetically efficient form of travel (Wilson et al., 2013), the initiation of tortuous movements should provide some form of benefit to offset the energetic costs of turning. Tortuous movements have been linked with searching behavior and increased foraging success in many marine animals, including sharks, and are thought to indicate area-restricted searching (Austin et al., 2006; Papastamatiou et al., 2009, 2012; Towner et al., 2016; Adachi et al., 2017). For this reason, we argue that the tortuous tracks we recorded represented prey searching, despite the fact that we did not witness any consumption of prey. It should also be noted that the direct observation of natural predation events by marine predators tends to be very rare (Pitman et al., 2014; Papastamatiou et al., 2018a), particularly for ectothermic shark species that are likely to feed less frequently compared to marine mammals and seabirds (Papastamatiou et al., 2018a). Given that video recordings were limited to a maximum of six daylight hours per tag due to data storage and lighting constraints, it is perhaps not surprising that we did not record any predation events involving live prey. Alternatively, tiger sharks may forage less frequently than other, smaller, shark species. Mass specific metabolic rate will decline with increasing shark size (Sims, 2000), and as a result, a larger tiger shark would theoretically need to eat fewer items of larger prey. Animal-borne camera tags deployed on two gray reef sharks (Carcharhinus amblyrhynchos; <1.6 m total length), for example, recorded nine foraging attempts across just 22 h of footage (Papastamatiou et al., 2018b). Longer recording durations of camera tags would enable foraging attempts in larger shark species, including tiger sharks, to be recorded and for associated acceleration signals to be validated. Collectively, this will facilitate further comparisons of foraging rates among ectothermic shark species.
In addition, previous observations of foraging behavior suggest that tiger sharks have a preference for weakened and/or injured prey and rarely predate on vigilant animals (Heithaus et al., 2002a; Hammerschlag et al., 2016). In several cases prey investigations were followed by either escape maneuvers performed by turtles, or color displays from demersal fish, all of which culminated with the shark continuing on its course without attempting to predate on the alert individual. It is also possible that some of these foraging behaviors may not have been focused on the potential prey species that were seen in the video, particularly in the case of benthic fishes. Facultative scavenging is an important feature of the behavior of tiger sharks (Clua et al., 2013; Hammerschlag et al., 2016) and in some cases sharks may have been drawn to approach mesopredatory benthic fishes simply by the potential opportunity to scavenge food, i.e., kleptoparasitism. This might explain why burst acceleration occurred only sporadically during prey investigations (Figure 4).
Video data showed that tortuous movements were often associated with shallow sandflat habitats, a location where tiger sharks at Ningaloo Reef have frequently been observed hunting prey by ecotourism operators (pers. comm. Frazer McGregor, Supplementary Figure 3). It is possible that tiger sharks may be showing a pattern of area-restricted search after detecting potential prey, either to investigate this prey source or due to the high probability of encountering other prey items nearby (Weimerskirch et al., 2007), or when entering areas linked to high foraging success based on past learning experiences (Adachi et al., 2017). Similar patterns in exploratory foraging have been found in the movements of lions (Panthera leo), where GPS-collared individuals exhibited more tortuous and slower movements when in the vicinity of waterholes, a pattern that suggested area-restricted searching in an area with potentially higher prey density (Valeix et al., 2010). In our study, sandflats may be preferred habitats for foraging by tiger sharks because prey here have less room to perform evasive maneuvers and to escape (Heithaus et al., 2002b), increasing the efficiency of hunting.
Three-Dimensional Movements
Fine-scale movement behaviors were invisible in the time-depth record. A comparison of vertical and horizontal tracks of tagged tiger sharks found no relationship between vertical movements and path tortuosity, and some tracks with the same time-depth record displayed very different horizontal paths. Level or oscillatory swimming occurred while sharks displayed either directional or tortuous swimming, suggesting that vertical movements may not change significantly when tiger sharks undergo area-restricted search behaviors. This pattern contrasts to that observed in sandbar sharks (Carcharhinus plumbeus), where tortuous movements were associated with extended periods of level swimming on the seabed and therefore lower diving ratios (Andrzejaczek et al., 2018). Dietary preferences may be responsible for the differences in fine-scale movement patterns between these two species. Sandbar sharks feed primarily on demersal prey (McElroy et al., 2006), while tiger sharks are facultative scavengers with a preference for both air-breathing and benthic prey (Simpfendorfer et al., 2001; Hammerschlag et al., 2016). This suggests that the vertical distribution of the preferred prey of these predators may influence their three-dimensional search patterns. Inter-specific differences in foraging strategy may also drive differences in fine-scale movement behaviors, however, further data is required for evaluation of this hypothesis.
The majority of interactions with potential prey occurred when tiger sharks were level-swimming (92%), with several of these events taking place during tortuous paths. Oscillatory behavior was often observed prior to prey investigation events. These combined behaviors suggest that tiger sharks may have been attempting to increase their likelihood of encountering visual or olfactory cues through the water column (Carey et al., 1990; Klimley et al., 2002; Nakamura et al., 2011), and once triggered, began tortuous paths in conjunction with level swimming in order to locate prey and remain in a potentially profitable area. For example, one shark encountered a loggerhead turtle on a small coral reef and then circled the same area for 25 min, despite the prey item not being re-encountered on video (Figure 3). These changes in behaviors indicate that future studies should investigate how both fine-scale horizontal and vertical movements vary before and after prey are encountered in order to better understand search strategies in sharks. The variance in behaviors observed in similar depth profiles also highlights the need for a combination of high-resolution tri-axial movement, video, and depth sensors to quantify the behavior of these animals, as time-depth records alone would be insufficient to distinguish prey searching and fine-scale habitat use. A similar conclusion was reached by Davis et al. (2003), who used high-resolution three-dimensional movement data and animal-borne video to classify dive types of Weddell seals (Leptonychotes weddellii). These additional data streams facilitated accurate classification of dive functionality (i.e., foraging or exploring) in comparison to previous studies that had only used time-depth recorders to classify dives (Davis et al., 2003).
Challenges and Future Considerations
Although biologging approaches provide a wealth of new information, there are many challenges in processing, analyzing, visualizing and interpreting the large amount of data recorded by these tags (Whitney et al., 2018). Manipulation of the datasets can require access to large computers and proficiency in multiple types of specialist software, and challenges also arise in visualizing the data to display biologically relevant patterns within very complex data sets. Here, many of the different data streams were shown to be important in understanding the fine-scale movement behavior of tiger sharks. Pseudo-tracks and three-dimensional plots presented a relatively simple means to visualize the data, and both heading and tailbeat kinematic data were demonstrated to be useful in describing interactions with prey. This multi-faceted approach is likely to be of use for investigating and comparing these behaviors among other species of marine animals, where differences in foraging ecology may drive differences in prey searching behaviors and therefore three-dimensional movement patterns.
Despite our biologging approach revealing detailed insights into movements and behaviors of tiger sharks, interpretation of data sets was hampered by several issues. Firstly, the field of view of the camera often limited our interpretation of behaviors, and was not likely to record all interactions of sharks with prey. A wider field of view, or optimally cameras capable of filming across 360° may enable further behavioral insights. Secondly, storage and battery constraints and the need to recover the tags in order to download archived data sets constrained tag deployments to relatively short periods (a few days at most). Delaying video activation until after predicted recovery periods for sharks could assist in extending tag recording time. However, there is no current solution to the need to recover the tags, which makes it difficult to place the fine-scale movement patterns we described into a long-term context. Lastly, accurate speed measurements and GPS anchor points would considerably increase the value of the magnetometer data in allowing us to calculate more accurate fine-scale habitat preferences in both space and time (Walker et al., 2015). Such data would enable a shift from the more qualitative interpretation of three-dimensional fine-scale habitat use reported here, to quantitative analyses such as spherical first passage time (Bailleul et al., 2010), which would allow for an objective classification of area-restricted search behavior. Accurate measurements of spatial scale will also reveal how sharks adjust their movement paths according to distributions of food resources and environmental factors (Fritz et al., 2003), further enabling identification of drivers of movement patterns.
Conclusion
Our study demonstrates the utility of multi-sensor biologging tags in classifying the fine-scale movements and behaviors of a keystone marine predator. Our results showed that recording movement in two-dimensions alone, as is the case with traditional time-depth recorders, is not sufficient in distinguishing among fine-scale behavioral modes. Data obtained from the combination of magnetometers, accelerometers, and video effectively described predator-prey interactions and habitat use, providing important information that will enable a greater understanding of the role these predators play in coral reef ecosystems.
Ethics Statement
All methods were used in accordance with approved guidelines by the University of Western Australia Animal Ethics Committee (RA/3/100/1437), and under permit numbers 2881 (WA Department of Fisheries) and 08-000322-3 (WA Department of Parks and Wildlife).
Author Contributions
SA, MM, and AG conceived the study. SA, AG, TC, and KL performed fieldwork/data collection. SA, AG, CP, and MM analyzed and interpreted the data, and SA led the writing of the manuscript. All authors read and approved the final manuscript.
Funding
Funding for this research was provided by crowdfunding on the Experiment platform (10.18258/7190), a Holsworth Wildlife Research Endowment and a UWA Graduate Research School fieldwork award. SA was funded by an Australian Postgraduate Award and UWA top-up scholarship. Two CATS tags were provided by Big Wave Productions.
Conflict of Interest Statement
The authors declare that the research was conducted in the absence of any commercial or financial relationships that could be construed as a potential conflict of interest.
Acknowledgments
We thank Murdoch University and Coral Bay Research Station for accommodation and vessel use while conducting fieldwork. This work would not have been possible without the generous support of numerous volunteers, particularly: Frazer McGregor, Abraham Sianipar, Adam Jolly, Blair Bentley, Evan Byrnes, Garry Teesdale and Michael Tropiano providing valuable field support; Olivia Seeger and Abraham Sianipar who helped with the video analysis. Nikolai Liebsch provided invaluable assistance with tag functioning. Alex Kydd, David Palfrey and Daniel Thomas-Browne generously contributed photos and video. Ryan Daly supplied helpful assistance for the analysis. We would also like to thank Yuuki Watanabe, Yannis Papastamatiou and Culum Brown for providing constructive and insightful comments that improved the manuscript.
Supplementary Material
The Supplementary Material for this article can be found online at: https://www.frontiersin.org/articles/10.3389/fmars.2019.00229/full#supplementary-material
References
Acuña-Marrero, D., Smith, A. N. H., Hammerschlag, N., Hearn, A., Anderson, M. J., Calich, H., et al. (2017). Residency and movement patterns of an apex predatory shark (Galeocerdo cuvier) at the Galapagos Marine Reserve. PLoS ONE 12:e0183669. doi: 10.1371/journal.pone.0183669
Adachi, T., Costa, D. P., Robinson, P. W., Peterson, S. H., Yamamichi, M., Naito, Y., et al. (2017). Searching for prey in a three-dimensional environment: hierarchical movements enhance foraging success in northern elephant seals. Funct. Ecol. 31, 361–369. doi: 10.1111/1365-2435.12686
Andrews, K. S., Williams, G. D., Farrer, D., Tolimieri, N., Harvey, C. J., Bargmann, G., et al. (2009). Diel activity patterns of sixgill sharks, Hexanchus griseus: the ups and downs of an apex predator. Anim. Behav. 78, 525–536. doi: 10.1016/j.anbehav.2009.05.027
Andrzejaczek, S., Gleiss, A. C., Pattiaratchi, C. B., and Meekan, M. G. (2018). First insights into the fine-scale movements of the sandbar shark, Carcharhinus plumbeus. Front. Mar. Sci. 5:483. doi: 10.3389/fmars.2018.00483
Austin, D., Bowen, W. D., McMillan, J. I., and Iverson, S. J. (2006). Linking movement, diving, and habitat to foraging success in a large marine predator. Ecology 87, 3095–3108. doi: 10.1890/0012-9658(2006)87[3095:LMDAHT]2.0.CO;2
Bailleul, F., Lesage, V., and Hammill, M. O. (2010). Spherical first passage time: a tool to investigate area-restricted search in three-dimensional movements. Ecol. Modell. 221, 1665–1673. doi: 10.1016/j.ecolmodel.2010.04.001
Bancroft, K. (2003). A Standardised Classification Scheme for the Mapping of Shallow-Water Marine Habitats in Western Australia. Fremantle, WA: Marine Conservation Branch, Department of Conservation and Land Management, Report MCB-05/2003.
Barnett, A., Abrantes, K. G., Stevens, J. D., Bruce, B. D., and Semmens, J. M. (2010). Fine-scale movements of the broadnose sevengill shark and its main prey, the gummy shark. PLoS ONE 5:e15464. doi: 10.1371/journal.pone.0015464
Brewster, L. R., Dale, J. J., Guttridge, T. L., Gruber, S. H., Hansell, A. C., Elliott, M., et al. (2018). Development and application of a machine learning algorithm for classification of elasmobranch behaviour from accelerometry data. Mar. Biol. 165:62. doi: 10.1007/s00227-018-3318-y
Brunnschweiler, J., Queiroz, N., and Sims, D. (2010). Oceans apart? Short-term movements and behaviour of adult bull sharks Carcharhinus leucas in Atlantic and Pacific Oceans determined from pop-off satellite archival tagging. J. Fish Biol. 77, 1343–1358. doi: 10.1111/j.1095-8649.2010.02757.x
Carey, F., Scharold, J., and Kalmijn, A. J. (1990). Movements of blue sharks (Prionace glauca) in depth and course. Mar. Biol. 106, 329–342. doi: 10.1007/BF01344309
Chapman, D. D., Feldheim, K. A., Papastamatiou, Y. P., and Hueter, R. E. (2015). There and back again: a review of residency and return migrations in sharks, with implications for population structure and management. Ann. Rev. Mar. Sci. 7, 547–570. doi: 10.1146/annurev-marine-010814-015730
Chapple, T. K., Gleiss, A. C., Jewell, O. J. D., Wikelski, M., and Block, B. A. (2015). Tracking sharks without teeth: a non-invasive rigid tag attachment for large predatory sharks. Anim. Biotelemetry 3:14. doi: 10.?1186/?s40317-015-0044-9
Clua, E., Chauvet, C., Read, T., Werry, J. M., and Lee, S. Y. (2013). Behavioural patterns of a tiger shark (Galeocerdo cuvier) feeding aggregation at a blue whale carcass in Prony Bay, New Caledonia. Mar. Freshw. Behav. Physiol. 46, 1–20. doi: 10.1080/10236244.2013.773127
Comfort, C. M., and Weng, K. C. (2015). Vertical habitat and behaviour of the bluntnose sixgill shark in Hawaii. Deep Sea Res. II 115, 116–126. doi: 10.1016/j.dsr2.2014.04.005
Davis, R. W., Fuiman, L. A., Williams, T. M., Collier, S. O., Hagey, W. P., Kanatous, S. B., et al. (1999). Hunting behavior of a marine mammal beneath the Antarctic fast ice. Science 283:993.
Davis, R. W., Fuiman, L. A., Williams, T. M., Horning, M., and Hagey, W. P. (2003). Classification of Weddell seal dives based on 3-dimensional movements and video-recorded observations. Mar. Ecol. Prog. Ser. 264, 109–122. doi: 10.3354/meps264109
Ferreira, L. C., Thums, M., Meeuwig, J. J., Vianna, G. M. S., Stevens, J., McAuley, R., et al. (2015). Crossing latitudes—long-distance tracking of an apex predator. PLoS ONE 10:e0116916. doi: 10.1371/journal.pone.0116916
Friard, O., and Gamba, M. (2016). BORIS: a free, versatile open-source event-logging software for video/audio coding and live observations. Methods Ecol. Evol. 7, 1325–1330. doi: 10.1111/2041-210x.12584
Fritz, H., Said, S., and Weimerskirch, H. (2003). Scale–dependent hierarchical adjustments of movement patterns in a long–range foraging seabird. Proc. R. Soc. 270, 1143–1148. doi: 10.1098/rspb.2003.2350
Gleiss, A. C., Gruber, S. H., and Wilson, R. P. (2009a). “Multi-channel data-logging: towards determination of behaviour and metabolic rate in free-swimming sharks,” in Tagging and Tracking of Marine Animals With Electronic Devices, eds J. L. Nielsen, H. Arrizabalaga, N. Fragoso, A. J. Hobday, M. Lutcavage, and J. Sibert (New York, NY: Springer), 211–228.
Gleiss, A. C., Norman, B., Liebsch, N., Francis, C., and Wilson, R. P. (2009b). A new prospect for tagging large free-swimming sharks with motion-sensitive data-loggers. Fish. Res. 97, 11–16. doi: 10.1016/j.fishres.2008.12.012
Gleiss, A. C., Norman, B., and Wilson, R. P. (2011). Moved by that sinking feeling: variable diving geometry underlies movement strategies in whale sharks. Funct. Ecol. 25, 595–607. doi: 10.1111/j.1365-2435.2010.01801.x
Hammerschlag, N., Bell, I., Fitzpatrick, R., Gallagher, A. J., Hawkes, L. A., Meekan, M. G., et al. (2016). Behavioral evidence suggests facultative scavenging by a marine apex predator during a food pulse. Behav. Ecol. Sociobiol. 70, 1777–1788. doi: 10.1007/s00265-016-2183-2
Heithaus, M., Dill, L., Marshall, G., and Buhleier, B. (2002a). Habitat use and foraging behavior of tiger sharks (Galeocerdo cuvier) in a seagrass ecosystem. Mar. Biol. 140, 237–248. doi: 10.1007/s00227-001-0711-7
Heithaus, M., Frid, A., and Dill, L. (2002b). Shark-inflicted injury frequencies, escape ability, and habitat use of green and loggerhead turtles. Mar. Biol. 140, 229–236. doi: 10.1007/s00227-001-0712-6
Heithaus, M. R., Frid, A., Wirsing, A. J., and Worm, B. (2008). Predicting ecological consequences of marine top predator declines. Trends Ecol. Evol. 23, 202–210. doi: 10.1016/j.tree.2008.01.003
Heithaus, M. R., Marshall, G. J., Buhleier, B. M., and Dill, L. M. (2001). Employing Crittercam to study habitat use and behavior of large sharks. Mar. Ecol. Prog. Ser. 209, 307–310. doi: 10.3354/meps209307
Heupel, M. R., and Simpfendorfer, C. A. (2015). Long-term movement patterns of a coral reef predator. Coral Reefs 34, 679–691. doi: 10.1007/s00338-015-1272-4
Kawatsu, S., Sato, K., Watanabe, Y., Hyodo, S., Breves, J. P., Fox, B. K., et al. (2009). A new method to calibrate attachment angles of data loggers in swimming sharks. EURASIP J. Adv. Signal Process. 2010:732586. doi: 10.1155/2010/732586
Klimley, A. P., Beavers, S. C., Curtis, T. H., and Jorgensen, S. J. (2002). Movements and swimming behavior of three species of sharks in La Jolla Canyon, California. Environ. Biol. Fishes 63, 117–135. doi: 10.1023/A:1014200301213
Lavery, T. J., Roudnew, B., Gill, P., Seymour, J., Seuront, L., Johnson, G., et al. (2010). Iron defecation by sperm whales stimulates carbon export in the Southern Ocean. Proc. R. Soc. 277, 3527–3531. doi: 10.1098/rspb.2010.0863
Lear, K. O., and Whitney, N. M. (2016). Bringing data to the surface: recovering data loggers for large sample sizes from marine vertebrates. Anim. Biotelemetry 4:12. doi: 10.1186/s40317-016-0105-8
McElroy, W. D., Wetherbee, B. M., Mostello, C. S., Lowe, C. G., Crow, G. L., and Wass, R. C. (2006). Food habits and ontogenetic changes in the diet of the sandbar shark, Carcharhinus plumbeus, in Hawaii. Environ. Biol. Fishes 76, 81–92. doi: 10.1007/s10641-006-9010-y
Meekan, M., Fuiman, L., Davis, R., Berger, Y., and Thums, M. (2015). Swimming strategy and body plan of the world's largest fish: implications for foraging efficiency and thermoregulation. Front. Mar. Sci. 2:64. doi: 10.3389/fmars.2015.00064
Nakamura, I., Goto, Y., and Sato, K. (2015). Ocean sunfish rewarm at the surface after deep excursions to forage for siphonophores. J. Anim. Ecol. 84, 590–603. doi: 10.1111/1365-2656.12346
Nakamura, I., Watanabe, Y. Y., Papastamatiou, Y. P., Sato, K., and Meyer, C. G. (2011). Yo-yo vertical movements suggest a foraging strategy for tiger sharks Galeocerdo cuvier. Mar. Ecol. Prog. Ser. 424, 237–246. doi: 10.3354/meps08980
Narazaki, T., Sato, K., Abernathy, K. J., Marshall, G. J., and Miyazaki, N. (2013). Loggerhead turtles (Caretta caretta) use vision to forage on gelatinous prey in mid-water. PLoS ONE 8:e66043. doi: 10.1371/journal.pone.0066043
Papastamatiou, Y., Meyer, C. G., Kosaki, R. K., Wallsgrove, N. J., and Popp, B. N. (2015). Movements and foraging of predators associated with mesophotic coral reefs and their potential for linking ecological habitats. Mar. Ecol. Prog. Ser. 521, 155–170. doi: 10.3354/meps11110
Papastamatiou, Y. P., DeSalles, P. A., and McCauley, D. J. (2012). Area-restricted searching by manta rays and their response to spatial scale in lagoon habitats. Mar. Ecol. Prog. Ser. 456, 233–244. doi: 10.3354/meps09721
Papastamatiou, Y. P., Lowe, C. G., Caselle, J. E., and Friedlander, A. M. (2009). Scale-dependent effects of habitat on movements and path structure of reef sharks at a predator-dominated atoll. Ecology 90, 996–1008. doi: 10.1890/08-0491.1
Papastamatiou, Y. P., Meyer, C. G., Carvalho, C., Dale, J. J., Hutchinson, M. R., and Holland, K. N. (2013). Partial migration in tiger sharks. Bull. Ecol. Soc. Am. 94, 250–251. doi: 10.1890/0012-9623-94.3.250
Papastamatiou, Y. P., Meyer, C. G., Watanabe, Y. Y., and Heithaus, M. R. (2018a). “Animal-borne video cameras and their use to study shark ecology and conservation,” in Shark Research: Emerging Technologies and Applications for the Field and Laboratory, eds J. C. Carrier, M. R. Heithaus, and C. A. Simpfendorfer. (Boca Raton, FL: CRC Press), 83–92.
Papastamatiou, Y. P., Watanabe, Y. Y., Demšar, U., Leos-Barajas, V., Bradley, D., Langrock, R., et al. (2018b). Activity seascapes highlight central place foraging strategies in marine predators that never stop swimming. Mov. Ecol. 6:9. doi: 10.1186/s40462-018-0127-3
Pewsey, A., Neuhäuser, M., and Ruxton, G. D. (2013). Circular Statistics in R. Oxford, UK: Oxford University Press.
Pinheiro, J., Bates, D., DebRoy, S., Sarkar, D., Heisterkamp, S., Van Willigen, B., et al. (2017). Package ‘nlme’. Linear and Nonlinear Mixed Effects Models, version, 3–1.
Pitman, R. L., Totterdell, J. A., Fearnbach, H., Ballance, L. T., Durban, J. W., and Kemps, H. (2014). Whale killers: prevalence and ecological implications of killer whale predation on humpback whale calves off Western Australia. Mar. Mamm. Sci. 31, 629–657. doi: 10.1111/mms,.12182
R Core Team (2017). “R: language and environment for statistical computing,” in R Foundation for Statistical Computing. (Vienna). Available online at: https://www.R-project.org/
Ryan, P. G., Petersen, S. L., Peters, G., and Grémillet, D. (2004). GPS tracking a marine predator: the effects of precision, resolution and sampling rate on foraging tracks of African penguins. Mar. Biol. 145, 215–223. doi: 10.1007/s00227-004-1328-4
Sakamoto, K. Q., Sato, K., Ishizuka, M., Watanuki, Y., Takahashi, A., Daunt, F., et al. (2009). Can ethograms be automatically generated using body acceleration data from free-ranging birds. PLoS ONE 4:e5379. doi: 10.1371/journal.pone.0005379
Shepard, E. L. C., Wilson, R. P., Halsey, L. G., Quintana, F., Laich, A. G., Gleiss, A. C., et al. (2008). Derivation of body motion via appropriate smoothing of acceleration data. Aquat. Biol. 4, 235–241. doi: 10.3354/ab00104
Simpfendorfer, C., Goodreid, A., and McAuley, R. (2001). Size, sex and geographic variation in the diet of the tiger shark, Galeocerdo cuvier, from Western Australian waters. Environ. Biol. Fishes 61, 37–46. doi: 10.1023/a:1011021710183
Sims, D. W. (2000). Can threshold foraging responses of basking sharks be used to estimate their metabolic rate? Mar. Ecol. Prog. Ser. 200, 289–296. doi: 10.3354/meps200289
Towner, A. V., Leos-Barajas, V., Langrock, R., Schick, R. S., Smale, M. J., Kaschke, T., et al. (2016). Sex-specific and individual preferences for hunting strategies in white sharks. Funct. Ecol. 30, 1397–1407. doi: 10.1111/1365-2435.12613
Valeix, M., Loveridge, A. J., Davidson, Z., Madzikanda, H., Fritz, H., and Macdonald, D. W. (2010). How key habitat features influence large terrestrial carnivore movements: waterholes and African lions in a semi-arid savanna of north-western Zimbabwe. Landsc. Ecol. 25, 337–351. doi: 10.1007/s10980-009-9425-x
Vaudo, J. J., Wetherbee, B. M., Harvey, G., Nemeth, R. S., Aming, C., Burnie, N., et al. (2014). Intraspecific variation in vertical habitat use by tiger sharks (Galeocerdo cuvier) in the western North Atlantic. Ecol. Evol. 4, 1768–1786. doi: 10.1002/ece3.1053
Walker, J. S., Jones, M. W., Laramee, R. S., Holton, M. D., Shepard, E. L. C., Williams, H. J., et al. (2015). Prying into the intimate secrets of animal lives; software beyond hardware for comprehensive annotation in ‘Daily Diary’ tags. Mov. Ecol. 3:29. doi: 10.1186/s40462-015-0056-3
Weimerskirch, H., Pinaud, D., Pawlowski, F., and Bost, C. A. (2007). Does prey capture induce area-restricted search? A fine-scale study using GPS in a marine predator, the wandering albatross. Am. Nat. 170, 734–743. doi: 10.1086/522059
Whitney, N. M., Lear, K. O., Gleiss, A. C., Payne, N., and White, C. F. (2018). “Advances in the application of high-resolution biologgers to elasmobranch fishes,” in Shark Research: Emerging Technologies and Applications for the Field and Laboratory, eds J. C. Carrier, M. R. Heithaus, and C. Simpfendorfer. (Boca Raton, FL: CRC Press), 45–70.
Whitney, N. M., Papastamatiou, Y. P., and Gleiss, A. C. (2012). “Integrative multi-sensor tagging of elasmobranchs: emerging techniques to quantify behavior, physiology, and ecology,” in Biology of Sharks and Their Relatives, eds J. Carrier, J. A. Musick, and M. Heithaus (Boca Raton, FL: CRC Press).
Whitney, N. M., Pratt, H. L. Jr, Pratt, T. C., and Carrier, J. C. (2010). Identifying shark mating behaviour using three-dimensional acceleration loggers. Endanger. Species Res. 10, 71–82. doi: 10.3354/esr00247
Whitney, N. M., White, C. F., Gleiss, A. C., Schwieterman, G. D., Anderson, P., Hueter, R. E., et al. (2016). A novel method for determining post-release mortality, behavior, and recovery period using acceleration data loggers. Fish. Res. 183, 210–221. doi: 10.1016/j.fishres.2016.06.003
Williams, H. J., Holton, M. D., Shepard, E. L. C., Largey, N., Norman, B., Ryan, P. G., et al. (2017). Identification of animal movement patterns using tri-axial magnetometry. Mov. Ecol. 5:6. doi: 10.1186/s40462-017-0097-x
Wilson, R. P., Griffiths, I. W., Legg, P. A., Friswell, M. I., Bidder, O. R., Halsey, L. G., et al. (2013). Turn costs change the value of animal search paths. Ecol. Lett. 16, 1145–1150. doi: 10.1111/ele.12149
Wilson, R. P., Liebsch, N., Davies, I. M., Quintana, F., Weimerskirch, H., Storch, S., et al. (2007). All at sea with animal tracks; methodological and analytical solutions for the resolution of movement. Deep Sea Res. II 54, 193–210. doi: 10.1016/j.dsr2.2006.11.017
Wilson, R. P., Shepard, E. L. C., and Liebsch, N. (2008). Prying into the intimate details of animal lives: use of a daily diary on animals. Endanger. Species Res. 4, 123–137. doi: 10.3354/esr00064
Wilson, R. P., White, C. R., Quintana, F., Halsey, L. G., Liebsch, N., Martin, G. R., et al. (2006). Moving towards acceleration for estimates of activity-specific metabolic rate in free-living animals: the case of the cormorant. J. Anim. Ecol. 75, 1081–1090. doi: 10.1111/j.1365-2656.2006.01127.x
Yoda, K., Sato, K., Niizuma, Y., Kurita, M., Bost, C., Le Maho, Y., et al. (1999). Precise monitoring of porpoising behaviour of Adélie penguins determined using acceleration data loggers. J. Exp. Biol. 202, 3121–3126. http://jeb.biologists.org/content/202/22/3121.short
Keywords: vertical movement, behavior, tortuosity, top predator, predator-prey interaction
Citation: Andrzejaczek S, Gleiss AC, Lear KO, Pattiaratchi CB, Chapple TK and Meekan MG (2019) Biologging Tags Reveal Links Between Fine-Scale Horizontal and Vertical Movement Behaviors in Tiger Sharks (Galeocerdo cuvier). Front. Mar. Sci. 6:229. doi: 10.3389/fmars.2019.00229
Received: 14 January 2019; Accepted: 12 April 2019;
Published: 14 May 2019.
Edited by:
Alastair Martin Mitri Baylis, South Atlantic Environmental Research Institute, Falkland IslandsReviewed by:
Yannis Peter Papastamatiou, Florida International University, United StatesYuuki Watanabe, National Institute of Polar Research, Japan
Copyright © 2019 Andrzejaczek, Gleiss, Lear, Pattiaratchi, Chapple and Meekan. This is an open-access article distributed under the terms of the Creative Commons Attribution License (CC BY). The use, distribution or reproduction in other forums is permitted, provided the original author(s) and the copyright owner(s) are credited and that the original publication in this journal is cited, in accordance with accepted academic practice. No use, distribution or reproduction is permitted which does not comply with these terms.
*Correspondence: Samantha Andrzejaczek, sandrzejaczek@gmail.com