- Department of Marine Sciences, The University of North Carolina at Chapel Hill, Chapel Hill, NC, United States
The small subunit 18S rRNA (18S) gene is the most commonly used marker for taxonomic identification in eukaryotes. However, protists may harbor substantial variation in their 18S gene copy number, which can lead to a rapid decline in concordance between 18S gene sequences and actual organismal abundances. Here we used a computational method to estimate 18S gene copy number in seven species of marine eukaryotic phytoplankton and found large interspecies and strain-level differences across and within the examined species. Our results emphasize that variations in 18S gene copy number need to be taken into consideration and that corrections can improve the accuracy of quantitative eukaryotic microbial community profiles.
Introduction
With substantial reductions in DNA sequencing costs combined with higher sequence yields, amplicon sequencing has revolutionized our view of microbial ecology (Sogin et al., 2006). It produces a culture-independent molecular characterization of the microbial community composition, and application of amplicon sequencing has successfully discovered novel microbes and characterized microbial diversity from a wide range of environments (Caron et al., 2012).
Due to its high specificity and sequence conservation, the 18S rRNA gene has become the most commonly used marker to explore eukaryotic protist community structure in both aquatic and terrestrial environments (Countway et al., 2005; de Vargas et al., 2015). However, 18S gene sequencing has inherent drawbacks that are typical of high throughput sequencing studies, such as PCR chimeras and sequencing errors (Caron et al., 2012). Ongoing research has focused on fixing such issues to provide a more accurate taxonomic description. Primers have been continuously modified and multiple hyper-variable regions can be simultaneously sequenced to reduce primer mismatch (Parada et al., 2016; Lin et al., 2017). Metagenomics has also been employed to preclude PCR-based bias (Eloe-Fadrosh et al., 2016).
Besides inherent technical issues, another source of bias to quantifying community composition with 18S gene sequences stems from variable copy numbers of ribosomal genes (Countway et al., 2005; Caron et al., 2012). Read counts of 18S genes are commonly used to estimate proportions of protists in amplicon sequencing analyses. However, the relative abundance of 18S gene copies in eukaryotic plankton collected from environmental samplescan be attributed both to variation in the relative abundance of different organisms, and to variation in genomic 18S copy number among those organisms (Zhu et al., 2005; Godhe et al., 2008). Phylogeny-based approaches have been developed to estimate ribosomal gene copy numbers for prokaryotes (Kembel et al., 2012; Angly et al., 2014), however, accuracy of such estimation can be compromised for protists due to the limited number of genomes that have been sequenced. Zhu et al. (2005) have developed a quantitative PCR-based approach to estimate 18S gene copy number for picoeukaryotes by normalizing total copies of 18S gene in the sample with cell abundance, but the results are highly dependent on DNA extraction efficiency, primer specificity and cell enumeration, and can be impractical for uncultured but prevalent phytoplankton species. Estimating 18S gene copy number remains an arduous task.
Due to the large number of sequences produced through high-throughput sequencing, a computational method that determines gene sequencing coverage has been recently developed and has become a promising method to estimate gene copy number variations (Zhao et al., 2013). It has been successfully applied to bacterial communities (Perisin et al., 2016), however, the application of such an approach on protist communities has yet to be performed. By normalizing sequencing coverage of 18S genes with that from single copy genes, we quantified 18S gene copy numbers of selected marine eukaryotic phytoplankton species whose draft assemblies are available in NCBI’s GenBank database. Thus far our results provide 18S gene copy number estimates for multiple representative species from four common phytoplankton classes and found large interspecies and strain-level 18S rRNA gene copy number variations across the different phytoplankton species.
Materials and Methods
Bioinformatics Pipeline
Draft/closed genome assemblies and raw sequences for Emiliania huxleyi, Ostreococcus tauri, Phaeodactylum tricornutum, Symbiodinium kawagutii, Symbiodinium minutum, Thalassiosira oceanica, and Trebouxia sp. were obtained from the NCBI Short Read Archive (see Supplementary Table 1 for NCBI accession numbers, Supplementary Figure 1). Raw sequences were trimmed with Trimmomatic v0.36 (Bolger et al., 2014). Bases with average quality scores below 20 with a sliding window of 4 bases were trimmed. Trimmed reads were quality checked with FastQC (Andrews, 2010). Bowtie2 v2.3.4.1 was used to map reads back to genome assemblies, and per-base sequencing depth was computed with samtools v1.8 depth command (Li et al., 2009; Langmead and Salzberg, 2012).
Identification of Single Copy Genes
Benchmarking Universal Single-Copy Orthologs (BUSCO) was used to assess genome assembly and annotation completeness. BUSCO has a set of phylogeny-specific single copy orthologs (Waterhouse et al., 2018). The protist dataset was phylogenetically close to eukaryotic phytoplankton and was thus selected as an initial set of reference single copy genes. A set of 83 eukaryotic single copy core genes described by Delmont (2018) was used as the reference single copy genes in this study (Supplementary Table 2). The reference single copy genes showed consistent read depth following a Poisson distribution with overdispersion (see example in Supplementary Figure 2), which further validated categorization as single copy genes in the examined phytoplankton genomes (Brynildsrud et al., 2015).
GC% Correction and 18S Gene Copy Number Estimation
GC% in sequences can affect sequencing depth and consequently lead to a biased copy number estimation (Yoon et al., 2009). Therefore, a linear model was fit for GC% and average per-base sequencing depth for single copy genes. If a significant correlation was detected (R2 > 0.1, slope p < 0.05), read-depth of single copy genes and 18S genes was corrected with the model parameters (Perisin et al., 2016) (see example in Supplementary Figure 3). The 18S rDNA V4 region is the most commonly used hypervariable region for amplicon sequencing due to its high resolution and accuracy for phylogenetic placement and was therefore selected to estimate 18S gene copy number (Dunthorn et al., 2012, 2014). The ratio of 18S gene read depth in the V4 region to the median of single copy gene read depth was then used to estimate 18S gene copy number.
Results and Discussion
Estimates of mean 18S V4-region gene copy numbers range from approximately 2–166 across the seven closed/draft phytoplankton genomes (Figure 1 and Supplementary Table 1). O. tauri, on the lower end, was estimated to have, on average, 3.4 copies of the 18S gene across 13 strains after GC% correction to account for possible sequencing bias. This is similar to the previously reported four copies of the ribosomal gene in strain RCC4221 (Blanc-Mathieu et al., 2014). GC% was shown to have a significant correlation with sequencing depth in O. tauri genome assemblies and could account for the observed difference from the reported four 18S gene copies estimated by Blanc-Mathieu et al. (2014) (Supplementary Figure 3). On the higher end, our results suggest an average of 160 18S gene copies in the dinoflagellate S. kawagutii, which could be attributed to large and repetitive genomes typical of dinoflagellates (Lin, 2011; Wisecaver and Hackett, 2011; Shoguchi et al., 2013; Lin et al., 2015). The notable two orders of magnitude difference can result in highly biased phytoplankton composition characterization, over/under-estimating species with higher/lower 18S gene copy number. For example, a simulated community with equal 18S gene sequence abundances from seven representative species seems to suggest that each species contributes equally to the community. However, upon 18S gene copy number correction, O. tauri and P. tricornutum actually dominate the natural community (Figure 2). In addition, strain level differences in 18S gene copy numbers were also detected, further highlighting the strong variation among eukaryotic phytoplankton, which may be a common characteristic among all protists. Estimates of 18S gene copy numbers in the coccolithophorid, E. huxleyi ranged from 16 to 109 across 14 different strains collected at different sites across the globe (Supplementary Table 1). Strains from English Channel have significantly higher 18S gene copies than those from the neighboring Bergen Sea (Supplementary Figure 4 and Supplementary Table 1). In contrast, 18S gene copy number was found to be more consistent for the diatom P. tricornutum strains that were also isolated from a large distribution of locations. It is unclear at this time whether this degree of variation in 18S gene copy number is due to inherent variability within particular phytoplankton groups or a result of the geographical location where the isolates were obtained (Figure 1). Further research is necessary to assess whether there are biogeographical patterns to these strain-level differences. The intraspecies geographic variation in 18S gene copy number adds another level of complexity to characterizing plankton community structure, and suggests that site-specific copy number estimations and corrections may be necessary for further compositional studies.
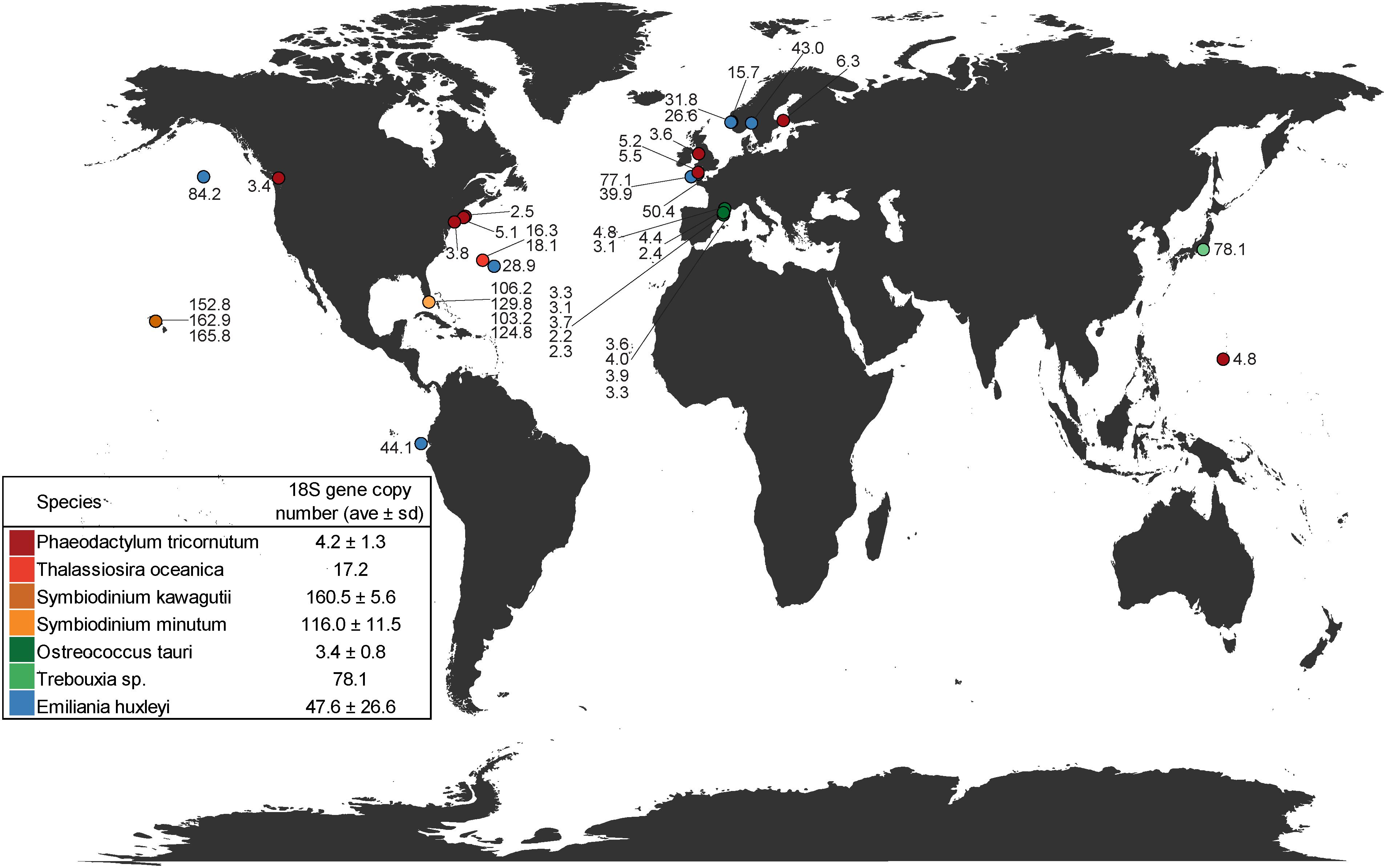
Figure 1. Geographic distribution of 18S gene copy number estimates for representative phytoplankton species with assembled/draft genomes plotted in relation to their isolation location. Symbols with more than one number represent locations in which multiple strains were sequenced. Inset table provides averages ± standard deviation of all strains for each species examined.
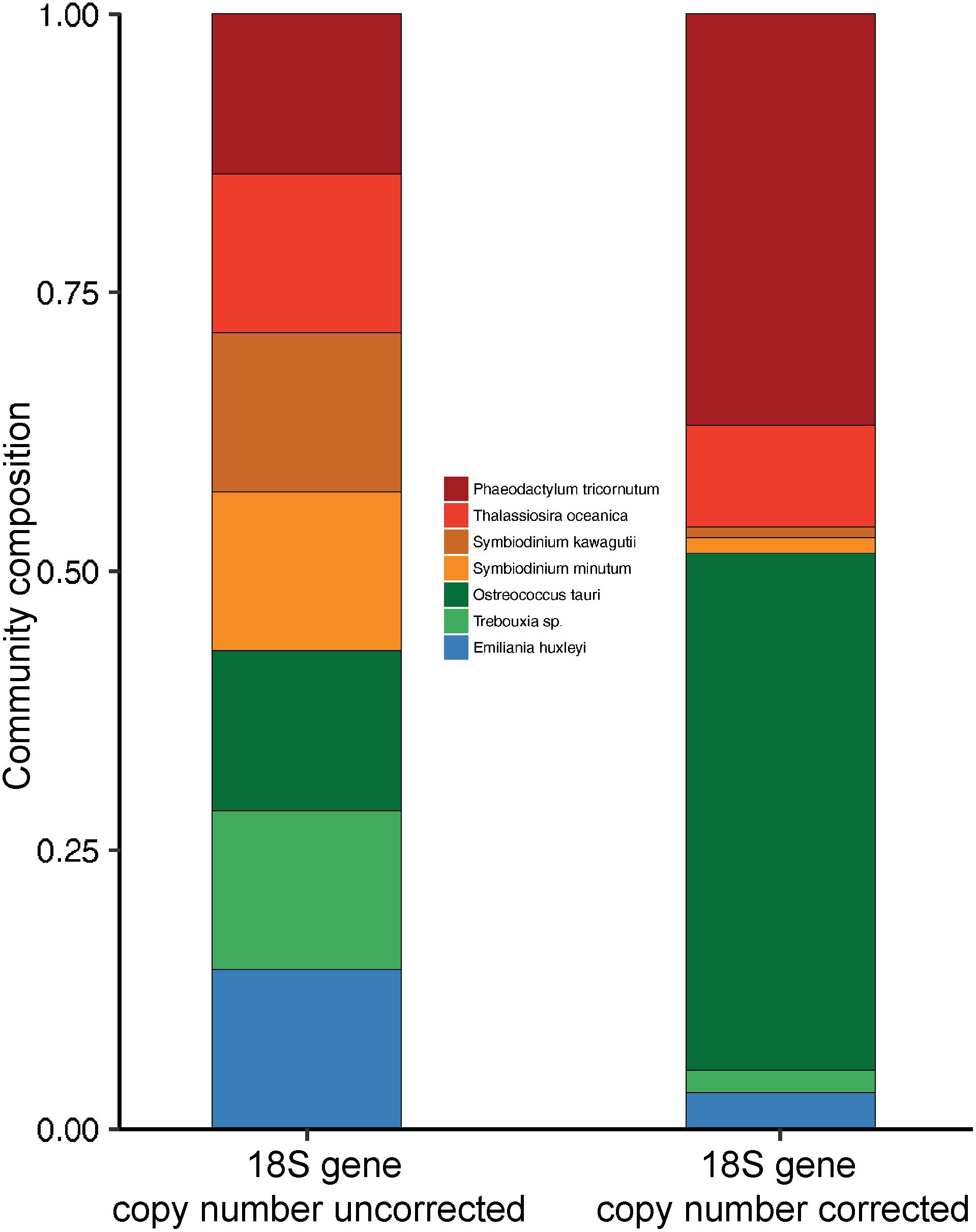
Figure 2. Composition of a simulated phytoplankton community with equal sequence abundance from seven phytoplankton species before and after 18S gene copy number correction. Correction is based on 18S gene copy number estimates from this study.
Tremendous sequencing effort has been devoted to estimate ribosomal copy numbers in bacteria and archaea, but our knowledge on their eukaryotic protist counterparts has benefited much less from the fast-developing sequencing technologies. Copy number variation can exert a strong influence on protist community composition and lead to biased ecological inferences. Recent bioinformatics technologies including metagenome assembly of genomes have the potential to provide new insights to estimating 18S gene copy numbers for multiple species simultaneously and will dramatically increase the number of estimates for protists (Delmont et al., 2018). With slight modifications, the bioinformatic pipeline implemented in this study can be applied to environmental sequences to provide an estimate of 18S gene copy numbers for dominant species. Assembled contigs that can be accurately taxonomically and functionally annotated as single copy genes, along with 18S genes, will generate an 18S gene copy number estimate. We propose that this pipeline be applied to metagenomic samples obtained from each location in which amplicon sequencing based compositional analyses are routinely performed.
Our findings emphasize the need to incorporate 18S gene copy number variation in protist compositional studies and provides a promising means to measure them in eukaryotic plankton, although further research is warranted due to complex genomes and polyploidy, especially in Alveolates. We anticipate that continuing sequencing efforts with consistent sequencing platforms and guaranteed sequencing depth along with emerging bioinformatics tools will add more perspectives to 18S gene copy number estimates and correction and will result in more accurate representation of eukaryotic community structure.
Author Contributions
WG designed the research and analyzed the data. WG and AM wrote the manuscript.
Funding
This research was supported by NASA OBB 2016 80NSSC17 K0552.
Conflict of Interest Statement
The authors declare that the research was conducted in the absence of any commercial or financial relationships that could be construed as a potential conflict of interest.
Acknowledgments
We thank S. Gifford for valuable and constructive comments.
Supplementary Material
The Supplementary Material for this article can be found online at: https://www.frontiersin.org/articles/10.3389/fmars.2019.00219/full#supplementary-material
References
Andrews, S. (2010). FastQC: A Quality Control Tool for High Throughput Sequence Data. Available at: http://www.bioinformatics.babraham.ac.uk/projects/fastqc/ (accessed April 19, 2018).
Angly, F. E., Dennis, P. G., Skarshewski, A., Vanwonterghem, I., Hugenholtz, P., and Tyson, G. W. (2014). CopyRighter: a rapid tool for improving the accuracy of microbial community profiles through lineage-specific gene copy number correction. Microbiome 2:11. doi: 10.1186/2049-2618-2-11
Blanc-Mathieu, R., Verhelst, B., Derelle, E., Rombauts, S., Bouget, F.-Y., Carré, I., et al. (2014). An improved genome of the model marine alga Ostreococcus tauri unfolds by assessing Illumina de novo assemblies. BMC Genomics 15:1103. doi: 10.1186/1471-2164-15-1103
Bolger, A. M., Lohse, M., and Usadel, B. (2014). Trimmomatic: a flexible trimmer for Illumina sequence data. Bioinformatics 30, 2114–2120. doi: 10.1093/bioinformatics/btu170
Brynildsrud, O., Snipen, L.-G., and Bohlin, J. (2015). CNOGpro: detection and quantification of CNVs in prokaryotic whole-genome sequencing data. Bioinformatics 31, 1708–1715. doi: 10.1093/bioinformatics/btv070
Caron, D. A., Countway, P. D., Jones, A. C., Kim, D. Y., and Schnetzer, A. (2012). Marine protistan diversity. Annu. Rev. Mar. Sci. 4, 467–493. doi: 10.1146/annurev-marine-120709-142802
Countway, P. D., Gast, R. J., Savai, P., and Caron, D. A. (2005). Protistan diversity estimates based on 18S rDNA from seawater incubations in the Western North Atlantic. J. Eukaryot. Microbiol. 52, 95–106. doi: 10.1111/j.1550-7408.2005.05202006.x
de Vargas, C., Audic, S., Henry, N., Decelle, J., Mahé, F., Logares, R., et al. (2015). Ocean plankton. Eukaryotic plankton diversity in the sunlit ocean. Science 348:1261605. doi: 10.1126/science.1261605
Delmont, T. (2018). Assessing the Completion of Eukaryotic Bins With anvi’o. Meren Lab. Available at: http://merenlab.org/2018/05/05/eukaryotic-single-copy-core-genes/ (accessed July 24, 2018).
Delmont, T. O., Quince, C., Shaiber, A., Esen,Ö. C., Lee, S. T., Rappé, M. S., et al. (2018). Nitrogen-fixing populations of Planctomycetes and Proteobacteria are abundant in surface ocean metagenomes. Nat. Microbiol. 3, 804–813. doi: 10.1038/s41564-018-0176-9
Dunthorn, M., Klier, J., Bunge, J., and Stoeck, T. (2012). Comparing the hyper-variable V4 and V9 regions of the small subunit rDNA for assessment of ciliate environmental diversity. J. Eukaryot. Microbiol. 59, 185–187. doi: 10.1111/j.1550-7408.2011.00602.x
Dunthorn, M., Otto, J., Berger, S. A., Stamatakis, A., Mahé, F., Romac, S., et al. (2014). Placing environmental next-generation sequencing amplicons from microbial eukaryotes into a phylogenetic context. Mol. Biol. Evol. 31, 993–1009. doi: 10.1093/molbev/msu055
Eloe-Fadrosh, E. A., Ivanova, N. N., Woyke, T., and Kyrpides, N. C. (2016). Metagenomics uncovers gaps in amplicon-based detection of microbial diversity. Nat. Microbiol. 1:15032. doi: 10.1038/nmicrobiol.2015.32
Godhe, A., Asplund, M. E., Harnstrom, K., Saravanan, V., Tyagi, A., and Karunasagar, I. (2008). Quantification of diatom and dinoflagellate biomasses in coastal marine seawater samples by real-time PCR. Appl. Environ. Microbiol. 74, 7174–7182. doi: 10.1128/AEM.01298-08
Kembel, S. W., Wu, M., Eisen, J. A., and Green, J. L. (2012). Incorporating 16S gene copy number information improves estimates of microbial diversity and abundance. PLoS Comput. Biol. 8:e1002743. doi: 10.1371/journal.pcbi.1002743
Langmead, B., and Salzberg, S. L. (2012). Fast gapped-read alignment with Bowtie 2. Nat. Methods 9, 357–359. doi: 10.1038/nmeth.1923
Li, H., Handsaker, B., Wysoker, A., Fennell, T., Ruan, J., Homer, N., et al. (2009). The sequence alignment/map format and SAMtools. Bioinformatics 25, 2078–2079. doi: 10.1093/bioinformatics/btp352
Lin, S. (2011). Genomic understanding of dinoflagellates. Res. Microbiol. 162, 551–569. doi: 10.1016/J.RESMIC.2011.04.006
Lin, S., Cheng, S., Song, B., Zhong, X., Lin, X., Li, W., et al. (2015). The symbiodinium kawagutii genome illuminates dinoflagellate gene expression and coral symbiosis. Science 350, 691–694. doi: 10.1126/science.aad0408
Lin, Y., Cassar, N., Marchetti, A., Moreno, C., Ducklow, H., and Li, Z. (2017). Specific eukaryotic plankton are good predictors of net community production in the Western Antarctic Peninsula. Sci. Rep. 7:14845. doi: 10.1038/s41598-017-14109-1
Parada, A. E., Needham, D. M., and Fuhrman, J. A. (2016). Every base matters: assessing small subunit rRNA primers for marine microbiomes with mock communities, time series and global field samples. Environ. Microbiol. 18, 1403–1414. doi: 10.1111/1462-2920.13023
Perisin, M., Vetter, M., Gilbert, J. A., and Bergelson, J. (2016). 16Stimator: statistical estimation of ribosomal gene copy numbers from draft genome assemblies. ISME J. 10, 1020–1024. doi: 10.1038/ismej.2015.161
Shoguchi, E., Shinzato, C., Kawashima, T., Gyoja, F., Mungpakdee, S., Koyanagi, R., et al. (2013). Draft assembly of the symbiodinium minutum nuclear genome reveals dinoflagellate gene structure. Curr. Biol. 23, 1399–1408. doi: 10.1016/J.CUB.2013.05.062
Sogin, M. L., Morrison, H. G., Huber, J. A., Mark Welch, D., Huse, S. M., Neal, P. R., et al. (2006). Microbial diversity in the deep sea and the underexplored "rare biosphere". Proc. Natl. Acad. Sci. U.S.A. 103, 12115–12120. doi: 10.1073/pnas.0605127103
Waterhouse, R. M., Seppey, M., Simão, F. A., Manni, M., Ioannidis, P., Klioutchnikov, G., et al. (2018). BUSCO applications from quality assessments to gene prediction and phylogenomics. Mol. Biol. Evol. 35, 543–548. doi: 10.1093/molbev/msx319
Wisecaver, J. H., and Hackett, J. D. (2011). Dinoflagellate genome evolution. Annu. Rev. Microbiol. 65, 369–387. doi: 10.1146/annurev-micro-090110-102841
Yoon, S., Xuan, Z., Makarov, V., Ye, K., and Sebat, J. (2009). Sensitive and accurate detection of copy number variants using read depth of coverage. Genome Res. 19, 1586–1592. doi: 10.1101/gr.092981.109
Zhao, M., Wang, Q., Wang, Q., Jia, P., and Zhao, Z. (2013). Computational tools for copy number variation (CNV) detection using next-generation sequencing data: features and perspectives. BMC Bioinformatics 14(Suppl. 11):S1. doi: 10.1186/1471-2105-14-S11-S1
Keywords: 18S rRNA gene, amplicon sequencing, plankton community composition, bioinformatics, microbial ecology
Citation: Gong W and Marchetti A (2019) Estimation of 18S Gene Copy Number in Marine Eukaryotic Plankton Using a Next-Generation Sequencing Approach. Front. Mar. Sci. 6:219. doi: 10.3389/fmars.2019.00219
Received: 11 February 2019; Accepted: 08 April 2019;
Published: 26 April 2019.
Edited by:
Sandie M. Degnan, The University of Queensland, AustraliaReviewed by:
Chih-Ching Chung, National Taiwan Ocean University, TaiwanDagmar Hajkova Leary, United States Naval Research Laboratory, United States
Copyright © 2019 Gong and Marchetti. This is an open-access article distributed under the terms of the Creative Commons Attribution License (CC BY). The use, distribution or reproduction in other forums is permitted, provided the original author(s) and the copyright owner(s) are credited and that the original publication in this journal is cited, in accordance with accepted academic practice. No use, distribution or reproduction is permitted which does not comply with these terms.
*Correspondence: Adrian Marchetti, amarchetti@unc.edu