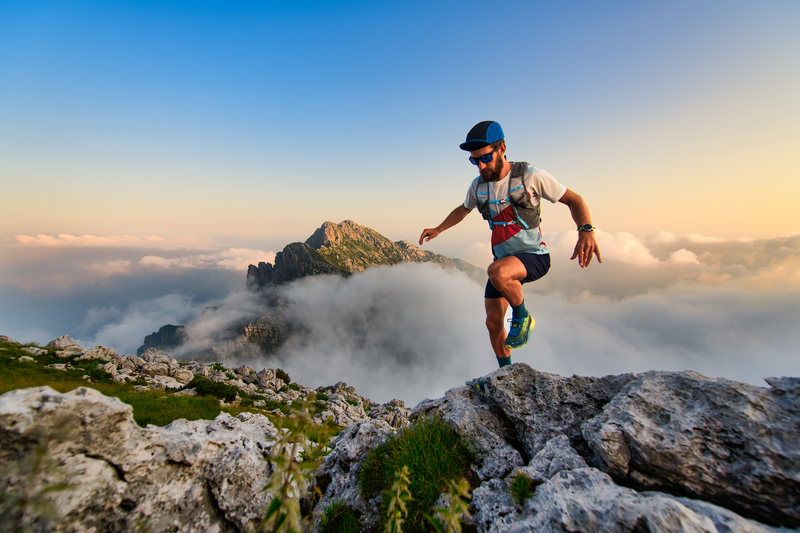
94% of researchers rate our articles as excellent or good
Learn more about the work of our research integrity team to safeguard the quality of each article we publish.
Find out more
MINI REVIEW article
Front. Mar. Sci. , 05 March 2019
Sec. Ocean Observation
Volume 6 - 2019 | https://doi.org/10.3389/fmars.2019.00090
This article is part of the Research Topic Oceanobs'19: An Ocean of Opportunity View all 136 articles
Ocean data assimilation is increasingly recognized as crucial for the accuracy of real-time ocean prediction systems and historical re-analyses. The current status of ocean data assimilation in support of the operational demands of analysis, forecasting and reanalysis is reviewed, focusing on methods currently adopted in operational and real-time prediction systems. Significant challenges associated with the most commonly employed approaches are identified and discussed. Overarching issues faced by ocean data assimilation are also addressed, and important future directions in response to scientific advances, evolving and forthcoming ocean observing systems and the needs of stakeholders and downstream applications are discussed.
A cornerstone of all ocean analysis and forecasting efforts is data assimilation (DA; see Carrassi et al., 2018), the rigorous and systematic combination of ocean observations and ocean models that yields an optimal estimate of the ocean state (both physical and biogeochemical conditions). The term optimal implies that of all possible combinations of the observations and model, it is the resulting best estimate that is sought according to some specified criteria. The founding principles of DA are rooted in Bayes’ theorem, the axioms that govern probability (Bayes, 1763), although similar methods arise in the field of control theory (see Talagrand, 2014). In brief, given a priori information about the laws governing the ocean state in the form of a model, an a priori state estimate from that model, and direct, but incomplete, ocean observations, an a posteriori state estimate is computed that weights all available information according to the hypothesized uncertainties in the model and observations. In a Bayesian framework, the optimal state estimate is that which coincides with the maximum a posteriori probability (Wikle and Berliner, 2007).
While the DA problem can be formulated precisely, the solution is challenging for the vast dimension of the ocean state simulated by operational ocean models that represent many complex non-linear processes. To make the problem tractable, it is necessary to make many simplifying assumptions about, for example, the nature of the a priori errors, so that while formally the resulting ocean state estimate is suboptimal, it is nonetheless useful. The most common DA approaches currently employed in operational oceanography are based on either variational or ensemble methods. In the former case, variational calculus is used to identify the ocean state that maximizes the conditional probability of the unknown ocean state given the observations, while for ensemble approaches the evolution of the conditional probability density function is estimated from the observations and an ensemble of plausible ocean states. Both approaches are accompanied by a litany of challenges and shortcomings which are briefly reviewed next.
Ocean data assimilation is a mainstay at many operational and academic centers, at both global (e.g., Martin et al., 2015) and regional scales (e.g., Edwards et al., 2015). While the tremendous ongoing efforts of many groups are recognized and acknowledged, individual systems will not be discussed per se. The focus instead will be on the general state of the field and ongoing challenges, with a view to the future in the Section “The Future of Ocean DA”.
At present, there are two general approaches to ocean DA that serve the needs of different communities. The first approach closely parallels the procedures employed in numerical weather prediction (NWP; see Kalnay, 2003) in which ocean state estimates are computed sequentially through time, and the resulting estimates updated when sufficient new observations become available. Similar methods are also used for producing ocean re-analyses (see Storto et al., unpublished, this issue). However, the continual restarting of the model and ingestion of data means that, in general, the conservation laws of the system may not be continuously respected. This can present a challenge when using the resulting ocean analyses to compute budgets, unless the contribution from the analysis increments is also explicitly included in budget calculations (e.g., Valdivieso et al., 2015; Storto et al., 2017). Thus, an alternative approach can be used in which ocean data are continuously assimilated over a very long (i.e., multi-decade) time-window during which all conservation properties of the ocean are implicitly respected during the model integrations. This latter approach is advantageous for studying the ocean state on climate timescales, although it too presents its own set of challenges (see Heimbach et al., 2019, this issue). That said, the primary focus of this article will be sequential DA methods for operational and real-time applications and historical re-analyses.
As previously noted, two flavors of sequential ocean DA are commonly used at most operational centers, namely variational methods or ensemble approaches. The advantages and shortcomings of each approach will be considered separately followed by a discussion of some overarching challenges common to both.
Variational (Var) DA can be employed as either 3-dimensional Var (3D-Var) or 4-dimensional Var (4D-Var). During 3D-Var, all data collected within a short time window (∼ days) are assimilated as though collected at a single time. The most recent model forecast during the time window is interpolated to the observation locations close to the observation time, and the observation minus forecast differences (known as the innovations) are used as prior information [a procedure referred to as the first-guess at appropriate time (FGAT)]. A variational approach (called 3D-Var FGAT) is used to identify the optimal ocean state assuming that the innovations are valid at one time. In principle, 3D-Var FGAT is relatively straightforward and computationally affordable since only the non-linear forecast model is involved. During 4D-Var, the actual observation times are respected, and measurement information is implicitly interpolated in space and time (over the observation time window) by the governing model equations. Formally the non-linear problem is solved via a sequence of linear approximations involving a linearized version of the forecast model [usually a simplified form of the tangent-linear model (TLM)] and its adjoint (Courtier et al., 1994). As a result, 4D-Var is considerably more demanding than 3D-Var, not only because of the additional computational expense, but also because the TLM and its adjoint must be developed and maintained. However, the TLM and adjoint model have considerable practical utility beyond DA (e.g., Moore et al., 2004).
The first-guess for Var is also commonly referred to as the background, and an estimate of the errors in the background is required. A common underlying assumption of Var is that all errors have zero mean (i.e., are unbiased) and are described by Gaussian probability distributions, in which case they are completely characterized by the error covariance matrix. Furthermore, it is assumed that errors in the background and observations are uncorrelated. For some state variables, such as biogeochemical tracer concentrations, the errors are fundamentally non-Gaussian but can be transformed into new variables that are approximately Gaussian (e.g., Simon and Bertino, 2009; Fletcher, 2010).
The error covariance matrices for the background are traditionally denoted as P (Ide et al., 1997). By necessity, the corrections that the observations make to the first-guess/background must lie in the space spanned by P and, as such, P has been the subject of much research because of the central role it plays in DA. In basic Var DA, P is prescribed at the start of each data assimilation cycle meaning that it is generally only weakly dependent on the background. In some 3D-Var systems, though, flow-dependence is introduced by way of a tensor (Weaver and Courtier, 2001) that spreads innovations along rather than across background field contours, which is particularly desirable in frontal regions where cross- and along-front correlations typically have very different scales. On the other hand, during 4D-Var the TLM and adjoint model implicitly introduce a flow-dependence in the error covariance via the time evolution of the background.
Choosing appropriate forms for P and representing them efficiently in a DA system remains one of the most significant and fundamental challenges for variational ocean DA. For example, estimating the actual level of uncertainty of the first-guess is very difficult, and choosing a P that accurately reflects the inhomogeneity and anisotropic nature of the errors across the broad range of space- and time-scales that characterize the ocean is challenging, although innovative methods for multi-scale DA are being explored (e.g., Li et al., 2015; Mirouze et al., 2016). Another critical aspect in the specification of P is in the transfer of information from the observed variables to other variables, such as spreading information from sea level observations onto the sub-surface. This can be achieved using physically based parameterizations (e.g., Weaver et al., 2005) or using covariance information derived from long model simulations. However, building a database of errors from which P can be computed is non-trivial, and usual approaches rely on anomalies with respect to means, ensemble anomalies, or lagged forecast differences.
While experience at some NWP centers has demonstrated superior performance of 4D-Var relative to 3D-Var (e.g., Lorenc and Jardak, 2018) the cost-versus-benefit of the two approaches is still an open question for the ocean. Traditional 4D-Var methods are iterative sequential algorithms and are not readily parallelizable in time on modern computer architectures since each iteration depends on the previous iterations. Ensemble methods, discussed next, are free of this limitation, although parallel approaches to 4D-Var are being developed (e.g., D’Amore et al., 2014; Fisher et al., 2016).
The sequential DA problem can also be formally solved in the form of the Kálmán-Bucy Filter (KF). However, the large dimension of the system prohibits use of the KF as originally formulated because of the need to evolve the error covariance matrix P in time. A practical solution to this problem is to use an ensemble approach (akin to a Monte Carlo method) to approximate P. The literature abounds with many flavors of ensemble-based KFs (EnKF; Houtekamer and Zhang, 2016) in which a standard feature is an ensemble of non-linear model solutions that reflect the distribution of the errors in the first-guess ocean state resulting from uncertainties in the model inputs and physics. Ensemble generation is by no means a trivial undertaking, however, and care must be exercised when creating an ensemble. Since each ensemble member will typically require a run of the forecast model, the size of the resulting ensemble will usually be much smaller than the dimension of the system. As such, covariance localization and covariance inflation are essential ingredients of any practical EnKF.
Covariance localization is a procedure employed to eliminate spurious covariances arising from the limited size of the ensemble. The method involves applying a point-wise weighting (“localization”) function to the ensemble-derived P which can be a costly procedure. Furthermore, localization can degrade the dynamical consistency of the computed analyses (e.g., Cummings, 2005; Oke et al., 2007), and subsequent forecast. Nevertheless, localization can be useful when accounting for the wide range of circulation scales by using scale-dependent localization functions (e.g., Buehner and Shlyaeva, 2015). The limited size of the ensemble can also lead to an underestimate of the true covariance P, and sophisticated methods for inflating the covariance have been developed to address this problem (e.g., Anderson, 2009).
For some applications, the computational cost of an EnKF can be prohibitive, so less-optimal and more practical approaches, such as ensemble optimal interpolation (EnOI; that uses a time-invariant ensemble to estimate P), are sometimes used (Oke et al., 2002; Evensen, 2003; Sakov and Sandery, 2015). Other simplified EnKF approaches include using the leading eigenvectors of P (e.g., Brasseur and Verron, 2006; Lellouche et al., 2013).
While there is still debate about the minimum required ensemble size for ocean applications, the EnKF is attractive because, like 3D-Var, only the non-linear forecast model is needed, and the ensemble generation is highly parallelizable, which is an additional appeal for operational applications. The ensemble also provides information about uncertainty in the forecasts which can be useful for downstream applications.
Real-time data streams are obviously a critical component of any operational ocean DA system, and include satellite remote sensing observations in the form of sea surface temperature, sea surface height, sea surface salinity, and ocean color. Additional remotely sensed observations of surface currents from coastal high-frequency radars are another important source of data in regional systems. Critical subsurface hydrographic information is provided by profiling Argo floats, permanent mooring arrays (e.g., TAO/TRITON, PIRATA, and RAMA), and CTD and XBT measurements from research vessels, ships of opportunity and tagged marine mammals. Observations from autonomous vehicles and ocean gliders have also become an important data source in recent years. However, regardless of the data stream, quality control is also a critical component of DA.
A significant challenge for ocean DA is characterization of observation errors described by the observation error covariance matrix, R. In addition to instrument errors, R also formally includes the influence of errors due to interpolation of the model fields to the observation points, as well as errors associated with the inability of the model to represent all of the processes captured by an observation. The latter is probably the most significant contributor to R and is perhaps the least well understood (e.g., Oke and Sakov, 2008). Furthermore, quantifying and accounting for spatial and temporal correlations in satellite observation errors is a challenge, but can have a significant impact on the ocean state estimate (Chabot et al., 2015). Not accounting for such correlations therefore can also significantly limit the capabilities of ocean DA to capitalize fully on the dense observations that are now available from many different platforms.
In modern DA systems, the impact of each observing platform on the analyses and forecasts can be quantified and continuously monitored. This can provide valuable feedback to instrument operators in cases where platform impacts systematically drift from the norm and become outliers. Such quantitative information can also help government agencies lobby for resources to maintain or expand existing high impact observing systems.
While variational and ensemble DA methods present their own particular difficulties, some challenges transcend both approaches. For instance, model error is a significant limiting factor in all DA systems. Sources of model error include numerical approximations due to constraints on grid resolution and the limitations of parameterizations of important physical and biogeochemical processes. While model errors can be formally accounted for during DA (Bennett, 2002), specification of the model error covariance matrix is a major challenge. Surface and lateral boundary condition errors also represent a significant source of error, particularly at open boundaries in regional models.
Another significant obstacle for many ocean DA systems is systematic errors in the form of bias, which violates the fundamental assumption that underpins current approaches to DA. Sources of bias include model error and boundary condition error. While bias-correction techniques are currently employed at some centers in an attempt to minimize the impact of model error (e.g., Balmaseda et al., 2007), ultimately the root-cause of systematic error must be identified and eliminated. Observation bias is also an issue (particularly in satellite observations due to instrument differences), and care must be taken to account for bias either before or during the DA process (e.g., Lea et al., 2008; While and Martin, unpublished). As coupled Earth system modeling becomes the new norm, the need for DA methods that can simultaneously estimate the systematic model bias and the time-evolving model state will need to be addressed. In uncoupled systems, such model biases have traditionally been attributed to errors in atmospheric forcing. However, such a one-sided view will not be possible in Earth system models where the forecast must satisfy the initial constraints and model equations of all components.
More often than not, DA upsets the dynamical balances in the model, and the ensuing readjustment of the system can introduce unrealistic and intermittent levels of wave energy. This long-standing and ubiquitous problem is referred to as “initialization shock” and has received much attention in NWP where its effect on a forecast can be calamitous if left unchecked. In ocean DA it has received much less attention, although many centers apply ad-hoc techniques [such as Incremental Analysis Updates (IAU)] to mitigate the problem. However, the ensuing ocean wave activity resulting from initialization shocks can be especially pernicious for some applications, such as biogeochemical modeling (e.g., Raghukumar et al., 2015; Waters et al., 2017).
There are many exciting new directions and future opportunities for discovery in ocean DA. Perhaps the most immediate development borrowed from NWP is the merger of ensemble and variational methods that draws on the strengths of both approaches. Specifically, the static estimate of P used in Var and the flow-dependent estimate of P from an ensemble are combined to form a “hybrid” P that is employed in a DA system (e.g., Lorenc et al., 2015). In this way, the dynamical interpolation properties of the adjoint and the flow-dependent covariance information from the ensemble are simultaneously exploited. Experience in NWP suggests that the performance of hybrid approaches can improve the performance of an analysis-forecast system (Lorenc and Jardak, 2018), and efforts are underway to develop similar procedures for global (e.g., Penny et al., 2015; Frolov et al., 2016; Storto et al., 2018) and regional (e.g., Oddo et al., 2016) ocean prediction systems.
DA analysis and re-analysis products at higher horizontal and vertical resolution will continue to be a priority and a challenge. As such, the need for regional DA systems will likely increase, either stand-alone or embedded in global or other regional models. Therefore, the development of DA capabilities in nested models is an important and emerging research area.
While DA in coupled Earth system models is a high priority (see Penny et al., unpublished, this issue), DA in coupled sub-component models is also of considerable interest. For example, DA in ocean-sea-ice models (e.g., Buehner et al., 2017), physical-biogeochemical ocean models (Fennel et al., unpublished) and acoustic-physical models (Lermusiaux and Chiu, 2002) targets pressing environmental and operational concerns.
Various community DA resources are being developed, such as the Data Assimilation Research Testbed (DART; Anderson et al., 2009), the Object-Oriented Prediction System (OOPS), the Joint Effort for Data assimilation Integration (JEDI), EnKF-C (Sakov, 2014), and the Parallel Data Assimilation Framework (PDAF; Nerger and Hiller, 2013). These are likely to play a more significant role in the development of existing and new ocean DA capabilities. Since the development of DA systems requires a considerable investment of time and resources, community resources such as these will be vital for streamlining the process.
The development of DA methods that do not rely on the assumption of unbiased, Gaussian distributed errors (such as particle filters, van Leeuwen et al., 2015) is also actively being pursued to deal, for example, with the typically non-Gaussian distribution of biogeochemical variables in coupled physical-biological systems.
Responding to new and emerging observing platforms is also a crucial ongoing endeavor in ocean DA. For example, the planned launch in 2021 of the Surface Water and Ocean Topography (SWOT) mission promises to deliver an unprecedented level of detail about the ocean topography, and ocean DA systems must be ready to make efficient use of this new data stream (e.g., Carrier et al., 2016). SWOT will also usher in DA at the ocean sub-mesoscale (∼0.1 to ∼10 km), and considerably enhance the utility of high-resolution satellite radiometers and rapid sampling in situ probes deployed on ocean gliders and AUVs. Satellite-derived surface salinity also lends support to other observations currently assimilated by most operational systems (e.g., Toyoda et al., 2015; Martin et al., 2019), and there is a need for concomitant physical and biogeochemical measurements in support of coupled physical-biogeochemical DA to ensure consistency between the different fields. Finally, DA-based tools for designing adaptive sampling arrays are also emerging, heralding a new era of model-informed ocean observing systems.
AM and MM wrote the majority of this article. All authors contributed to the text.
SF acknowledges support from the Office of Naval Research award number N0001412WX20323.
The authors declare that the research was conducted in the absence of any commercial or financial relationships that could be construed as a potential conflict of interest.
Anderson, J. (2009). Spatially and temporally varying adaptive covariance inflation for ensemble filters. Tellus 61A, 72–83. doi: 10.1111/j.1600-0870.2008.00361.x
Anderson, J., Hoar, T. J., Raeder, K., Liu, H., Collins, N., Torn, R., et al. (2009). The data assimilation research test bed: a community facility. Bull. Am. Meteorol. Soc. 90, 1283–1296. doi: 10.1175/2009BAMS2618.1
Balmaseda, M. A., Dee, D., Vidard, A., and Anderson, D. L. T. (2007). A multivariate treatment of bias for sequential data assimilation: application to the tropical oceans. Q. J. R. Meteorol. Soc. 133, 167–179. doi: 10.1002/qj.12
Bayes, T. (1763). An essay towards solving a problem in the doctrine of chances. Philos. Trans. R. Soc. 53, 370–418.
Bennett, A. F. (2002). Inverse Modeling of the Ocean and Atmosphere. Cambridge: Cambridge University Press, 234. doi: 10.1017/CBO9780511535895
Brasseur, P., and Verron, J. (2006). The SEEK filter method for data assimilation in oceanography: a synthesis. Ocean Dyn. 56, 650–661. doi: 10.1007/s10236-006-0080-3
Buehner, M., Bertino, L., Caya, A., Heimbach, P., and Smith, G. (2017). “Sea ice data assimilation,” in Sea Ice Analysis and Forecasting: Towards an Increased Reliance on Automated Prediction Systems, eds T. Carrieres, M. Buehner, J.-F. Lemieux, and L. T. Pedersen (Cambridge: Cambridge University Press), 109–143.
Buehner, M., and Shlyaeva, A. (2015). Scale-dependent background-error covariance localisation. Tellus A 67:28027. doi: 10.3402/tellusa.v67.28027
Carrassi, A., Bocquet, M., Bertino, L., and Evensen, G. (2018). Data assimilation in the geosciences: an overview of methods, issues, and perspectives. Wiley Interdiscip. Rev. Clim. Change 9:e535. doi: 10.1002/wcc.535
Carrier, M. J., Ngodock, H. E., Smith, S. R., Souopgui, I., and Bartels, B. (2016). Examining the potential impact of SWOT observations in an ocean analysis–forecasting system. Mon. Weather Rev. 144, 3767–3782. doi: 10.1175/MWR-D-15-0361.1
Chabot, V., Nodet, M., Papadakis, N., and Vidard, A. (2015). Accounting for observation errors in image data assimilation. Tellus A 67, 4117–4119. doi: 10.3402/tellusa.v67.23629
Courtier, P., Thépaut, J.-N., and Hollingsworth, A. (1994). A strategy for operational implementation of 4D-Var using an incremental approach. Q. J. R. Meteorol. Soc. 120, 1367–1388. doi: 10.1002/qj.49712051912
Cummings, J. A. (2005). Operational multivariate ocean data assimilation. Q. J. R. Meteorol. Soc. 131, 3583–3604. doi: 10.1256/qj.05.105
D’Amore, L., Arcucci, R., Carracciulo, L., and Murli, A. (2014). A scalable approach for variational data assimilation. J. Sci. Comput. 61, 239–257. doi: 10.1007/s10915-014-9824-2
Edwards, C. A., Moore, A. M., Hoteit, I., and Cornuelle, B. D. (2015). Regional ocean data assimilation. Ann. Rev. Mar. Sci. 7, 6.1–6.22. doi: 10.1146/annurev-marine-010814-015821
Evensen, G. (2003). The ensemble Kalman filter: theoretical formulation and practical implementation. Ocean Dyn. 53, 343–367. doi: 10.1007/s10236-003-0036-9
Fisher, M., Gratton, S., Gürol, S., Trémolet, Y., and Vasseur, X. (2016). Low rank updates in preconditioning the saddle point systems arising from data assimilation problems. Optim. Methods Softw. 33, 45–69. doi: 10.1080/10556788.2016.1264398
Fletcher, S. J. (2010). Mixed gaussian-lognormal four-dimensional data assimilation. Tellus A 62, 266–287. doi: 10.1111/j.1600-0870.2010.00439.x
Frolov, S., Bishop, C. H., Holt, T. R., Cummings, J. A., and Kuhl, D. D. (2016). Facilitating strongly-coupled ocean-atmosphere data assimilation with an interface solver. Mon. Weather Rev. 144, 3–20. doi: 10.1175/MWR-D-15-0041.1
Heimbach, P., Fukumori, I., Hill, C. N., Ponte, R. M., Stammer, D., Campin, J. M., et al. (2019). Putting it all together: enhancing the global ocean and climate observing systems with complete self-consistent ocean state estimates. Front. Mar. Sci. 19:55.
Houtekamer, P. L., and Zhang, F. (2016). Review of the ensemble Kalman filter for atmospheric data assimilation. Mon. Weather Rev. 144, 4489–4525. doi: 10.1175/MWR-D-15-0440.1
Ide, K., Courtier, P., Ghil, M., and Lorenc, A. C. (1997). Unified notation for data assimilation: operational, sequential and variational. J. Meteorol. Soc. Japan 75, 181–189. doi: 10.2151/jmsj1965.75.1B_181
Kalnay, E. (2003). Atmospheric Modeling, Data Assimilation and Predictability. Cambridge: Cambridge University Press, 341.
Lea, D. J., Drecourt, J.-P., Haines, K., and Martin, M. J. (2008). Ocean altimeter assimilation with observational and model bias correction. Q. J. R. Meteoro. Soc. 134, 1761–1774. doi: 10.1002/qj.320
Lellouche, J.-M., Le Galloudec, O., Drevillon, M., Regnier, C., Greiner, E., Garric, G., et al. (2013). Evaluation of global monitoring and forecasting systems at Mercator Ocean. Ocean Sci. 9, 57–81. doi: 10.5194/os-9-57-2013
Lermusiaux, P. F. J., and Chiu, C.-S. (2002). “Four-dimensional data assimilation for coupled physical-acoustical fields,” in Acoustic Variability, eds N. G. Pace and F. B. Jensen (Dordrecht: Kluwer Academic Press),417–424.
Li, Z., McWilliams, J. C., Ide, K., and Farrara, J. D. (2015). A multiscale variational data assimilation scheme: formulation and illustration. Mon. Weather Rev. 143, 3804–3822. doi: 10.1175/MWR-D-14-00384.1
Lorenc, A. C., Bowler, N. E., Clayton, A. M., Pring, S. R., and Fairbairn, D. (2015). Comparison of hybrid-4DEnVar and hybrid-4DVar data assimilation methods for global NWP. Mon. Weather Rev. 143, 212–229. doi: 10.1175/MWR-D-14-00195.1
Lorenc, A. C., and Jardak, M. (2018). A comparison of hybrid variational data assimilation methods for global NWP. Q. J. R. Meteorol. Soc. 144, 2748–2760. doi: 10.1002/qj.3401
Martin, M. J., Balmaseda, M., Bertino, L., Brasseur, P., Brassington, G., Cummings, J., et al. (2015). Status and future of data assimilation in operational oceanography. J. Operat. Oceanogr. 8(Supp. 1), s28–s48. doi: 10.1080/1755876X.2015.1022055
Martin, M. J., King, R. R., While, J., and Aguiar, A. (2019). Assimilating satellite sea-surface salinity data from SMOS, aquarius and SMAP into a global ocean forecasting system. Q. J. R. Meteorol. Soc. 1–22. doi: 10.1002/qj.3461
Mirouze, I., Blockley, E. W., Lea, D. J., Martin, M. J., and Bell, M. J. (2016). A multiple length scale correlation operator for ocean data assimilation. Tellus A 68, 1–13. doi: 10.3402/tellusa.v68.29744
Moore, A. M., Arango, H. G., Di Lorenzo, E., Cornuelle, B. D., Miller, A. J., and Neilson, D. J. (2004). A comprehensive ocean prediction and analysis system based on the tangent linear and adjoint of a regional ocean model. Ocean Model. 7, 227–258. doi: 10.1016/j.ocemod.2003.11.001
Nerger, L., and Hiller, W. (2013). software for ensemble-based data assimilation systems - implementation strategies and scalability. Comput. Geosci. 55, 110–118. doi: 10.1016/j.cageo.2012.03.026
Oddo, P., Storto, A., Dobricic, S., Russo, A., Lewis, C., Onken, R., et al. (2016). A hybrid variational-ensemble data assimilation scheme with systematic error correction for limited-area ocean models. Ocean Sci. 12, 1137–1153. doi: 10.5194/os-12-1137-2016
Oke, P. R., Allen, J. S., Miller, R. N., Egbert, G. D., and Kosro, P. M. (2002). Assimilation of surface velocity data into a primitive equation coastal ocean model. J. Geophys. Res. 107:3122. doi: 10.1029/2000JC000511
Oke, P. R., and Sakov, P. (2008). Representation error of oceanic observations for data assimilation. J. Atmospher. Ocean. Technol. 25, 1004–1017. doi: 10.1175/2007JTECHO558.1
Oke, P. R., Sakov, P., and Corney, S. P. (2007). Impacts of localisation in the EnKF and EnOI: experiments with a small model. Ocean Dyn. 57, 32–45. doi: 10.1007/s10236-006-0088-8
Penny, S. G., Behringer, D. W., Carton, J. A., and Kalnay, E. (2015). A hybrid global ocean data assimilation system at NCEP. Mon. Weather Rev. 143, 4660–4677. doi: 10.1063/1.5001819
Raghukumar, K., Edwards, C. A., Goebel, N. L., Broquet, G., Veneziani, M., Moore, A. M., et al. (2015). Impact of assimilating physical oceanographic data on modeled ecosystem dynamics in the California Current System. Prog. Oceanogr. 138, 546–558. doi: 10.1016/j.pocean.2015.01.004
Sakov, P. (2014). EnKF-C user guide. arXiv [Preprint]. arXiv:1410.1233
Sakov, P., and Sandery, P. A. (2015). Comparison of EnOI and EnKF regional ocean reanalysis systems. Ocean Model. 89, 45–60. doi: 10.1016/j.ocemod.2015.02.003
Simon, E., and Bertino, L. (2009). Application of the Gaussian anamorphosis to assimilation in a 3-D coupled physical-ecosystem model of the North Atlantic with the EnKF: a twin experiment. Ocean Model. 4, 495–510.
Storto, A., Oddo, P., Cipollone, A., Mirouze, I., and Lemieux, B. (2018). Extending an oceanographic variational scheme to allow for affordable hybrid and four-dimensional data assimilation. Ocean Model. 128, 67–86. doi: 10.1016/j.ocemod.2018.06.005
Storto, A., Yang, C., and Masina, S. (2017). Constraining the global ocean heat content through assimilation of CERES-derived TOA energy imbalance estimates. Geophys. Res. Lett. 44, 10520–10529. doi: 10.1002/2017GL075396
Talagrand, O. (2014). “4D-VAR: four-dimensional variational assimilation,” in Advanced Data Assimilation for Geosciences, Chapter 1, 3-30, eds É Blayo, M. Bocquet, E. Cosme, and L. F. Cugliandodo (Oxford: Oxford University Press), 584.
Toyoda, T., Fujii, Y., Kuragano, T., Matthews, J. P., Abe, H., Ebuchi, N., et al. (2015). Improvements to a global ocean data assimilation system through the incorporation of aquarius surface salinity data. Q. J. R Meteorol. Soc. 141, 2750–2759. doi: 10.1002/qj.2561
Valdivieso, M., Haines, K., Balmaseda, M., Chang, Y. S., Drevillon, M., Ferry, N., et al. (2015). An assessment of air–sea heat fluxes from ocean and coupled reanalyses. Clim. Dyn. 49, 983–1008. doi: 10.1007/s00382-015-2843-3
van Leeuwen, P.-J., Cheng, Y., and Reich, S. (2015). Nonlinear Data Assimilation. Berlin: Springer, 117. doi: 10.1007/978-3-319-18347-3
Waters, J., Bell, M. J., Martin, M. J., and Lea, D. J. (2017). Reducing ocean model imbalances in the equatorial region caused by data assimilation. Q. J. R. Meteorol. Soc. 143, 195–208. doi: 10.1002/qj.2912
Weaver, A. T., and Courtier, P. (2001). Correlation modelling on the sphere using a generalized diffusion equation. Q. J. R. Meteorol. Soc. 127, 1815–1846. doi: 10.1002/qj.49712757518
Weaver, A. T., Deltel, C., Machu, R., Ricci, S., and Daget, N. (2005). A multivariate balance operator for variational ocean data assimilation. Q. J. R. Meteorol. Soc. 131, 3605–3625. doi: 10.1256/qj.05.119
Keywords: data assimilation, calculus of variations, Kálmán filters, ensembles, modeling
Citation: Moore AM, Martin MJ, Akella S, Arango HG, Balmaseda M, Bertino L, Ciavatta S, Cornuelle B, Cummings J, Frolov S, Lermusiaux P, Oddo P, Oke PR, Storto A, Teruzzi A, Vidard A and Weaver AT (2019) Synthesis of Ocean Observations Using Data Assimilation for Operational, Real-Time and Reanalysis Systems: A More Complete Picture of the State of the Ocean. Front. Mar. Sci. 6:90. doi: 10.3389/fmars.2019.00090
Received: 08 October 2018; Accepted: 18 February 2019;
Published: 05 March 2019.
Edited by:
Laura Lorenzoni, University of South Florida, United StatesReviewed by:
Fabien Roquet, University of Gothenburg, SwedenCopyright © 2019 Moore, Martin, Akella, Arango, Balmaseda, Bertino, Ciavatta, Cornuelle, Cummings, Frolov, Lermusiaux, Oddo, Oke, Storto, Teruzzi, Vidard and Weaver. This is an open-access article distributed under the terms of the Creative Commons Attribution License (CC BY). The use, distribution or reproduction in other forums is permitted, provided the original author(s) and the copyright owner(s) are credited and that the original publication in this journal is cited, in accordance with accepted academic practice. No use, distribution or reproduction is permitted which does not comply with these terms.
*Correspondence: Andrew M. Moore, YW1tb29yZUB1Y3NjLmVkdQ==
Disclaimer: All claims expressed in this article are solely those of the authors and do not necessarily represent those of their affiliated organizations, or those of the publisher, the editors and the reviewers. Any product that may be evaluated in this article or claim that may be made by its manufacturer is not guaranteed or endorsed by the publisher.
Research integrity at Frontiers
Learn more about the work of our research integrity team to safeguard the quality of each article we publish.