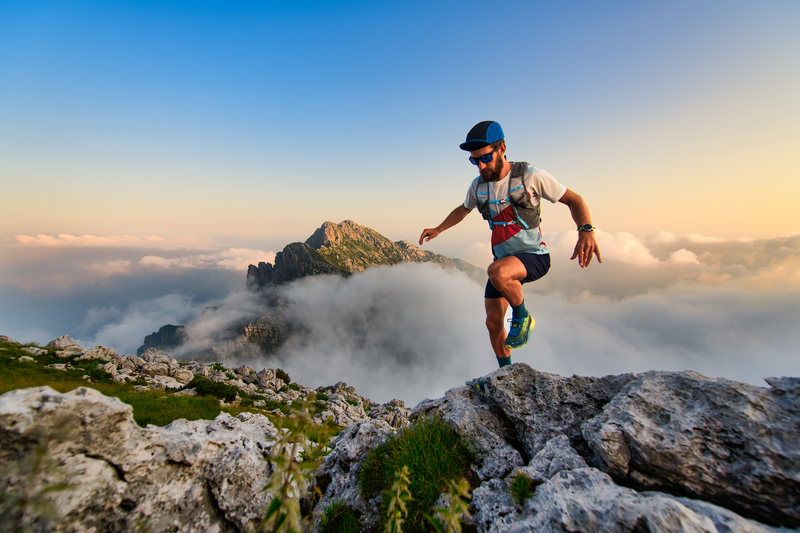
94% of researchers rate our articles as excellent or good
Learn more about the work of our research integrity team to safeguard the quality of each article we publish.
Find out more
ORIGINAL RESEARCH article
Front. Mar. Sci. , 10 September 2018
Sec. Marine Ecosystem Ecology
Volume 5 - 2018 | https://doi.org/10.3389/fmars.2018.00320
This article is part of the Research Topic Mixotrophy in Protists: from Model Systems to Mathematical Models View all 12 articles
Results from a dynamic mathematical model are presented simulating the growth of the harmful algal bloom (HAB) mixotrophic dinoflagellate Karlodinium veneficum and its algal prey, Rhodomonas salina. The model describes carbon-nitrogen-phosphorus-based interactions within the mixotroph, interlinking autotrophic and phagotrophic nutrition. The model was tuned to experimental data from these species grown under autotrophic conditions and in mixed batch cultures in which nitrogen:phosphorus stoichiometry (input molar N:P of 4, 16, and 32) of both predator and prey varied. A good fit was attained to all experimentally derived carbon biomass data. The potential effects of temperature and nutrient changes on promoting growth of prey and thus K. veneficum bloom formation were explored using this simulation platform. The simulated biomass of K. veneficum was highest when they were functioning as mixotrophs and when they consumed prey under elevated N:P conditions. The scenarios under low N:P responded differently, with simulations showing larger deviation between mixotrophic and autotrophic growth, depending on temperature. When inorganic nutrients were in balanced proportions, lower biomass of the mixotroph was attained at all temperatures in the simulations, suggesting that natural systems might be more resilient against Karlodinium HAB development in warming conditions if nutrients were available in balanced proportions. These simulations underscore the need for models of HAB dynamics to include consideration of prey; modeling HAB as autotrophs is insufficient. The simulations also imply that warmer, wetter springs that may bring more N with lower N:P, such as predicted under climate change scenarios for Chesapeake Bay, may be more conducive to development of these HABs. Prey availability may also increase with temperature due to differential growth temperature responses of K. veneficum and its prey.
In conjunction with the growing recognition that harmful algal blooms (HABs) are promoted by increasing nutrient loads to marine and freshwaters (e.g., Anderson et al., 2002; Glibert et al., 2005; Heisler et al., 2008; Glibert and Burford, 2017), there is also an enhanced appreciation for the importance of mixotrophy in the nutrition of many HAB taxa (Jeong et al., 2005a,b; Burkholder et al., 2008; Flynn et al., 2013; Stoecker et al., 2017). The complexities of understanding the dynamics of HABs dominated by mixotrophs compound the already difficult study of system regulation by simple autotrophic physiology (Flynn, 2009; Mitra and Flynn, 2010; Ghyoot et al., 2017; Flynn and McGillicuddy, 2018; Glibert et al., 2018). For instance, some mixotroph species are primarily autotrophic but ingest prey under light limitation or conditions of nutrient limitation or imbalance, while some others appear to be primarily heterotrophic but photosynthesize under certain conditions by retaining chloroplasts from their prey (Stoecker, 1998). The challenge in understanding HAB dynamics where mixotrophy is an important nutritional mode is thus further complicated by the need to not only understand the ecophysiology of the HAB species, but also that of its prey species. Additionally, mixotrophy may also be related to toxin production in some HABs (e.g., Blossom et al., 2012).
Mixotrophy in protists is not a simple additive process of autotrophy plus phagotrophy, but rather a complex integration of physiological interactions (Flynn and Mitra, 2009; Mitra and Flynn, 2010). Only a few physiological experiments (Lundgren et al., 2016; Lin et al., 2017)–and even fewer model constructs–consider the feedback function of rates of change during mixotrophic feeding, the nutritional status of both predator and prey, and linkages to nutrient physiological interactions (Mitra and Flynn, 2006; Flynn, 2010). At present, predictive capabilities that include the role of mixotrophy in bloom formation are just beginning to be developed (Flynn, 2005, 2010; Glibert et al., 2010; Mitra et al., 2014, 2016; Flynn and McGillicuddy, 2018; Flynn et al., 2018).
There is also a growing appreciation that changes in climate may expand the potential niches for some harmful or toxic algal blooms (Hallegraeff, 2010; Fu et al., 2012; Wells et al., 2015; Glibert and Burkholder, 2018). The most direct effects of climate change are those associated with rising temperatures. The frequency and severity of blooms may be exacerbated due to temperature-driven competitive advantages for HAB species over non-HAB species (Hallegraeff, 2010) and other HAB-favorable conditions may expand, such as increased stratification or altered precipitation patterns that affect the timing of freshwater and associated nutrient delivery (e.g., Heisler et al., 2008; Moore et al., 2015; Glibert and Burkholder, 2018). The mixotrophic chrysophyte Ochromonas sp., for example, has been found to become more heterotrophic with increased temperature (Wilken et al., 2013). However, other climate changes events such as ocean acidification with (de)eutrophication may also affect the consortium of organisms that co-occur with the HAB species, and which can be food sources for these mixotrophs (e.g., Fu et al., 2012; Flynn et al., 2015; Wells et al., 2015; Glibert et al., 2018). There is evidence that ingestion, growth rates and cell volume of the heterotrophic dinoflagellate Oxyrrhis marina respond differently to temperature-prey interactions, indicating complex and non-linear predator-prey dynamics with increasing temperatures (Montagnes et al., 2003; Kimmance et al., 2006). Many important questions related to the interactive effects of temperature changes and mixotrophy on HAB dynamics clearly remain.
The mixotrophic dinoflagellate Karlodinium veneficum (formerly Gymnodinium galatheanum and K. micrum) is a common toxigenic species that can produce a suite of unique polyketide compounds, karlotoxins (Van Dolah, 2000; Kempton et al., 2002). This species is a constitutive mixotroph (Mitra et al., 2016), possessing the ability to make its own chloroplasts, and is capable of forming blooms of up to 107-108 cells L-1 (e.g., Adolf et al., 2008) that have been associated with fish and shellfish mortality, both in natural waters and aquaculture farms worldwide (Braarud, 1957; Nielsen, 1993; Glibert and Terlizzi, 1999; Deeds et al., 2002; Stoecker et al., 2008; Zhou et al., 2011). Blooms of K. veneficum appear to be increasing in size and frequency of occurrence in estuaries such as Chesapeake Bay, MD, United States (Li et al., 2015) and elsewhere worldwide (Place et al., 2012 and references therein; Dai et al., 2013; Adolf et al., 2015). This mixotroph is also a species for which there are considerable physiological data related to mixotrophy (e.g., Li et al., 1999, 2000; Place et al., 2012; Lin et al., 2017). Given its prevalence and potential threats to natural resources around the world, improved forecasting and predictive ability of this HAB taxon would be an aid to managers.
Here, applying both previously published and newly acquired experimental data, a model was developed to improve our understanding of the interactions of the growth of this mixotroph and its common prey, Rhodomonas salina, under varying nutrient and temperature conditions. The resultant simulations were used to address the hypothesis that growth of this mixotroph may increase due to the combination of increased nutrient concentrations, altered nutrient ratios, and raised temperature, combinations of conditions that may be expected under future climate conditions in eutrophic estuaries. While such responses may seem intuitive, the extent to which changes in nutrient (in form and proportion) together with temperature alter growth of the mixotroph and its prey are not well resolved and therefore simulations enable such interactions to be explored for multiple temperature and nutrient conditions. Results from using such models may help inform nutrient management plans under future climate conditions.
A mechanistic model was developed based on the framework of an existing variable stoichiometric, photo-acclimative mixotrophy model, namely the “perfect beast” construct of Flynn and Mitra (2009). This describes carbon-nitrogen-phosphorus (C-N-P)-based interactions within a mixotroph cell, and builds upon the variable stoichiometric zooplankton model of Mitra (2006) and the phytoplankton model of Flynn (2001). To parameterize the model, previously published data as well as new data on temperature responses of both the mixotroph and prey were applied (Figure 1).
FIGURE 1. Schematic diagram to illustrate the steps taken to determine the constants required for development of the “perfect beast” model (Flynn and Mitra, 2009) of mixotrophy of Karlodinium veneficum and its application under varying nutrient conditions and increasing temperature.
In brief, the “perfect beast” model has eight state variables (Figure 2) describing C, N, and P and chlorophyll (Chl) associated with the core mixotroph (m) biomass (mC, NC, PC, and ChlC) and also the same constituents associated with the contents of the food (F) vacuole (namely, FC, FNC, FPC, and FChlC) after the m have fed on algal prey. The amount of material associated with the F vacuole is relative to the core mC biomass. Thus, the total C associated with the m is mC⋅(1+FC) with the unit of gC L-1. Here the m model was configured to be consistent with the status of K. veneficum as a constitutive m, with its own photoacclimative description of Chl:C. Prey were described using the variable stoichiometric photoacclimative phytoplankton model of Flynn (2001), as deployed in Flynn and Mitra (2009). The full model accounts for predator stoichiometry, prey stoichiometry (i.e., F quality) and their feedback interactions (Figure 2). The model operates using ordinary differential equations (ODEs) using an Euler integration routine with a timestep of 0.0039 d (5.625 min). Equations are provided in the associated Supplementary Table S1; these are given in a linear form to aid reconstruction in the modeling platform of choice.
FIGURE 2. Schematic of the structure of the “perfect beast” model, showing major flows in and out of state variables (solid arrows and boxes) from the external parameters (NO3-, PO43-, and Light), and the major feedback processes (dashed arrows). Autotrophic growth uses inorganic nutrients and light via the photosystems of the mixotroph (phototrophy; white part). A proportion of activity leading to growth is required to support synthesis of those photosystems. Predation brings algal prey into the food vacuole within the confines of the mixotroph cell (heterotrophy; gray part). Interactions between phototrophic and heterotrophic nutrition (Int1) influence the growth of the mixotrophy (Flynn and Mitra, 2009). The state variables (yellow boxes) that describe carbon (C), nitrogen (N), phosphorus (P), and chlorophyll (Chl) associated with core mixotroph biomass are mC (C-biomass of the mixotroph), ChlC (chlorophyll C quota), NC (cellular NC quota), and PC (cellular PC quota), while the same constituents (green boxes) associated with the content of food vacuole are FC (food vacuole C content relative to mC), FChlC (food vacuole Chl content relative to mC), FNC (food vacuole N content relative to mC), and FPC (food vacuole P content relative to mC).
The model was built, and simulations run, using the Powersim Constructor platform, with tuning (calibration) to experimental data performed using the evolutionary algorithm supported by Powersim Solver v2 (Isdalstø, Norway). This algorithm maximizes the likelihood of resolving a global, rather than a local, minimum, and produces the fit closest to the presented data (Haefner, 2005; Flynn, 2018). Most of the constants within the model are not tuned (Tables 1, 2); they are used to modulate physiological feedback processes and the model is not sensitive to their precise value (see source papers for further details).
TABLE 1. Autotrophic state constants that were calculated and gained from tuning against changes in experimental monoculture cultures of Rhodomonas salina and Karlodinium veneficum.
TABLE 2. Constants obtained from tuning the “perfect beast” model of Flynn and Mitra (2009) against experimentally derived changes in carbon biomass in mixed cultures of Karlodinium veneficum (mixotroph) with Rhodomonas salina (prey) when each was grown separately in different N:P condition (low NP = 4, Redfield = 16, and high N:P = 32 on a molar basis) and also combined in a total of 9 combinations.
To configure the full model describing both the mixotroph and its prey, the constants that constrain the autotrophic physiology of predator and prey were first determined from experimental data (Figure 1). Once rates of photosynthesis and inorganic nutrient uptake for these species were calculated for varying nutrients and temperatures, the parameters that control mixotrophic performance of the predator were then ascertained (again through reference to experimental data), for conditions in which predator and prey were both grown under varying nutrient stoichiometry. Finally, the tuned model was run to simulate (predict) growth of K. veneficum and its prey under variable N:P and temperature conditions.
Previously available experimental data were first exploited. These included rates of autotrophic growth of both K. veneficum and R. salina under varied N:P stoichiometry (molar N:P of 4, 16, and 32) in exponential growth phase (Lin et al., 2017; Figure 1); these experimental data are provided in the Supplementary Table S2). In order to establish the variable N:P conditions in those experiments, NO3- concentrations were held constant at ∼1232 μg N L-1, while initial PO43- concentrations were varied. The constants that describe the autotrophic physiology of prey (e.g., half saturation constants for N and P uptake, aKNi, aKP, and of growth, aμmaxphot) and m (e.g., mKNi, and mKP) were obtained from model tuning based on the change in residual NO3- and PO43- concentrations (nutrient kinetics), and Chl and C biomass (see below) during culture growth (Table 1). From a second set of experiments, based on monocultures of K. veneficum and R. salina, growing autotrophically, initial slopes of photosynthesis-irradiance (PI) curves were calculated (Lin and Glibert, unpublished). Additional physiological data (Supplementary Table S1) for parameterizing the autotrophic component of the model were obtained from Flynn and Mitra (2009). The C biomass of K. veneficum was estimated based on a cellular C-volume relationship for dinoflagellates (Menden-Deuer and Lessard, 2000) and a conversion factor of 0.2 pg C μm-3 from volume to C for R. salina was applied (Jakobsen and Hansen, 1997). Cell size of predator and prey were recorded in parallel with the cell densities in the study of Lin et al. (2017); cellular volume (CV) was estimated from these data using the following equation:
where W and L are the width and length of cells.
To parameterize temperature responses, new experimental data were also obtained on rates of autotrophic growth of K. veneficum and R. salina across a temperature gradient (Figure 1). The same strains of K. veneficum and R. salina used in Lin et al. (2017) were inoculated separately into f/2 media (Guillard, 1975) and maintained at 12, 17, 20, 25, 28°C under irradiance of 430 micromol photons m-2 s-1 in a 12 h light:12 h dark cycle in batch cultures. The strains were acclimated for 2 weeks to the experimental conditions, after which growth was monitored over 96 h. Aliquots (2 mL) were collected for cell enumeration at 0, 24, 48, 72, and 96 h from each flask and were preserved in paraformaldehyde (final concentrations of 1% v/v) at 4°C for later cell enumeration. The cells were identified and gated based on size, shapes, and auto-fluorescence using a BD Accurri C6 flow cytometry. Then, cell-specific growth rates of predator and prey were determined separately based on the rates of changes in the slopes of the regression of natural log-transformed cell-densities change over 96 h.
After the mixotroph model was calibrated, and after autotrophic temperature responses of predator and prey were experimentally determined (and assumed to remain the same under mixotrophic conditions), the model was used to simulate 10-day growth responses of the mixotroph and its prey under 3 nutrient conditions (N:P = 4, N:P = 16, and N:P = 32 for both predator and prey) under varying temperatures (Figure 1). Rates of maximum growth of K. veneficum are reported in autotrophic and in mixotrophic nutritional modes.
All statistical analyses were performed with R. The Shapiro–Wilk test was used to verify normality of the experimental data while the Levenes’ test was used to assess the homogeneity of variance. Cell-specific growth rates of K. veneficum and R. salina were compared for statistical differences in slopes of regression of natural log-transformed data under each temperature conditions (ANCOVA test). Two-way analysis of variance was applied to test for the interactive effects between temperature and species. Regressions were considered significant at p < 0.05 with the adjusted r2 value.
The responses to temperature of the mixotroph and its prey differed. Autotrophic growth rates of K. veneficum ranged between 0.06 and 0.29 d-1 and increased with increasing temperature up to 20°C above which growth rates fell sharply (Figure 3). The growth rates of R. salina had a similar range as those in the predator, from 0.06 to 0.26 d-1, but the prey grew significantly faster than its predator at temperatures > 20°C (ANCOVA, p < 0.001; Figure 3). Variations between temperature responses of the maximal growth rates of K. veneficum and R. salina were statistically significant (two-way ANOVA: F-value = 2.88, p = 0.011; Figure 3).
FIGURE 3. Effect of temperature on cell-specific, autotrophic growth rates (μmaxphot) of Karlodinium veneficum and Rhodomonas salina.
Model tuning was undertaken in 2 steps. Half saturation values (i.e., mKNi, mKP, aKNi, and aKP) were calculated based on nutrient depletion to determine transport of nutrients in relation to the cell quotas, and photosynthetic rates were used to determine cell C when cells were in autotrophic growth. Maximum and minimum ratios of N:C and P:C define the nutrient status of the cells (Table 1). These parameters were then applied to mixotrophic growth.
The mixotroph model was successfully calibrated against previously available data on the growth of the mixotroph and its prey as a function of variable nutrient stoichiometry (Figure 4). Between 67 and 97% of the variations in the nine experimental data sets could be explained by the simulations for biomass of the mixotroph, with no significant difference between the observed and predicted data (p < 0.05).
FIGURE 4. Fits of the “perfect beast” model (lines) to experimental data (symbols) for carbon biomass from nine mixed-culture systems. The low-NP Karlodinium veneficum (A–C) Redfield-NP K. veneficum (D–F) and high-NP K. veneficum (G–I) provided with low-NP, Redfield-NP and high-NP prey Rhodomonas salina during mixed-culture experiments, respectively. N, nitrogen; P, phosphorus. R2 coefficients are determined for the predator and prey under varying nutrient conditions.
The parameters that control mixotrophic growth showed variability with the nutritional status (C:N:P) of K. veneficum and its prey (Table 2). The most sensitive parameters were assimilation efficiency (mAEmin) and half saturation constant for ingestion (mKIng) and maximum growth rate of heterotrophic growth (mμmaxhet; Table 2). For example, the minimum assimilation efficiency (mAEmin) of C in the mixotroph from the ingested prey ranged from 0.370 under conditions in which both the mixotroph and prey were grown with nutrients supplied in Redfield conditions (N:P = 16), to 0.840 when the prey was under Redfield growth conditions but K. veneficum was grown under low N:P conditions. Thus, there was significantly higher mAEmin when the mixotroph was initially under low N:P conditions than when it was under Redfield N:P conditions and given the same quality prey. In addition, the half saturation for ingestion (mKIng) for K. veneficum grown under low N:P conditions was significantly lower when they were mixed with the high N:P prey than when K. veneficum in the same nutrient state was given prey grown under Redfield N:P and low N:P conditions (0.204 vs. 0.295 and 0.490, respectively). For those K. veneficum grown under high N:P conditions, their mKIng ranged from 0.010 to 0.309 with the lowest value corresponding to prey grown under low N:P. Maximum growth rate of K. veneficum as a photo-heterotroph (mμmaxhet) was consistently higher than maximum growth rate of K. veneficum as an autotroph (mμmaxphot) by factors of 1.19 (low N:P K. veneficum with Redfield N:P prey) to 2.72 (high N:P K. veneficum with low N:P prey; Table 2).
Using the tuned mixotroph-prey models, and having established individual temperature responses of K. veneficum and R. salina, scenarios were developed to estimate growth of both species under variable stoichiometry and temperature conditions (Figure 1). For K. veneficum, growth as an autotroph and as a mixotroph were compared, and for R. salina, growth with and without the predator were estimated. Three stoichiometric conditions were simulated, holding both species in the same N:P condition for each scenario (N:P = 4, 16, 32).
In the low N:P scenario, a significantly higher C biomass of K. veneficum in mixotrophic growth was attained in simulations with increased temperature (≥25°C) compared with that under comparable autotrophic conditions (ANCOVA, p < 0.001; Figures 5A,D). The highest mixotrophic growth rate, 0.30 d-1, was attained at 25°C, which was 1.5-fold higher than the simulation without prey at this temperature. In the Redfield N:P scenarios, there were no significant differences between autotrophic and mixotrophic growth rates of K. veneficum (ANCOVA, p = 0.161) and relatively low overall C biomass of the mixotroph was attained in the 10-day simulation (Figures 5B,E). Differences between mixotrophic and autotrophic growth of K. veneficum under high N:P conditions considered a distinct trend toward statistical significance (ANCOVA, p = 0.072). The growth patterns of K. veneficum in the two nutritional modes were very similar, showing increases in biomass reaching the maximum value of ∼2200 μgC L-1 at 20°C under mixotrophic conditions, but a lower growth rate and corresponding lower C biomass accumulation at the highest temperature (Figures 5C,F).
FIGURE 5. Simulated changes in carbon biomass of Karlodinium veneficum in mixotrophic (A–C) and autotrophic (D–F) growth under low N:P (=4), Redfield N:P (=16) and high N:P (=32) conditions, and variable temperature conditions over 10-day simulations.
The accumulation of C biomass and growth rates of R. salina in the presence and absence of the mixotroph were also estimated under variable nutrient and temperature conditions (Figure 6). In the presence of the mixotroph, prey biomass in all N:P conditions declined, but the patterns of decline varied depending on the nutrient conditions (Figures 6A–C). Under low N:P conditions, prey biomass gradually declined to zero within the 10-day simulation. Under Redfield conditions, prey remained detectable, but low, throughout this period. In the highest N:P simulation, prey biomass declined most quickly, to a near-zero biomass within 4 days. The patterns of the changes in prey biomass without predator were comparable among the three nutrient conditions, but higher biomass values were usually attained in the N-rich conditions at the near-highest temperatures (Figure 6F).
FIGURE 6. Simulated changes in carbon biomass of prey Rhodomonas salina with Karlodinium veneficum as predator (A–C) and without predator (D–F) under low N:P (=4), Redfield N:P (=16) and high N:P (=32) conditions, and variable temperature conditions over 10-day simulations.
Changes in cellular N:P of K. veneficum with time was also explored in the model output in autotrophic and mixotrophic growth to determine the extent to which the mixotroph was using inorganic nutrients under the different stoichiometric and temperature conditions. The cellular elemental ratios of K. veneficum under low N:P and Redfield N:P growth conditions varied considerably in the first 2 days of simulated growth, then converged at a value of ∼7, but those of K. veneficum grown in high N:P conditions converged on a value of ∼5 within 2 days under all temperatures conditions (Figure 7). Thus, in the simulations, under the condition of excess N (high N:P), K. veneficum appeared to become increasingly enriched with internal P through mixotrophy.
FIGURE 7. Simulated changes in cellular N:P ratio of Karlodinium veneficum in mixotrophic (A–C) and autotrophic (D–F) growth under low N:P (=4), Redfield N:P (=16) and high N:P (=32) conditions, and variable temperature conditions over 10-day simulations.
Despite the increasing recognition of the importance of mixotrophy in planktonic communities, especially HABs (Jeong et al., 2005a,b; Burkholder et al., 2008; Flynn et al., 2013; Stoecker et al., 2017), modeling of plankton dynamics that incorporates mixotrophy is in its infancy (but see Thingstad et al., 1996; Stickney et al., 2000; Ward et al., 2011; Våge et al., 2013; Mitra et al., 2014; Berge et al., 2017; Ghyoot et al., 2017). For simplicity, most model approaches have assumed independence between phototrophic and phagotrophic regulations (e.g., Thingstad et al., 1996; Baretta-Bekker et al., 1998; Jost et al., 2004; Våge et al., 2013; Ward and Follows, 2016). The “perfect beast’ model (Flynn and Mitra, 2009) integrates phototrophy vs. phagotrophy with feedback functions to better represent a nearly true of mixotrophic behaviors, especially for predicting rates of ingestion (Mitra and Flynn, 2010). The “perfect beast” is a construct that can be configured to represent different types of constitutive and non-constitutive mixotrophs, and is consistent with our understanding of the different physiologies associated withthese different types of mixotrophs (Mitra et al., 2016). Although the model has been previously configured to represent different generic mixotroph types, and used to explore the implications of different types of mixotrophy in oligotrophic through to eutrophic conditions (Flynn and Mitra, 2009; Mitra and Flynn, 2010; Flynn and Hansen, 2013; Mitra et al., 2014), this is the first time that this, or indeed any, multi-stoichiometric model of protist mixotrophy has been specifically tuned to simulate experimental data of the complexity explored here. In large measure, this reflects the paucity of such data not only for mixotroph activity but also for the prey. Indeed, very few empirical or modeling studies of phytoplankton describe multiple stoichiometries (i.e., C:N:P), despite increasing evidence that in nature such multiple nutrient states are important features structuring ecology and that nutrient stoichiometry is changing in many systems with anthropogenic nutrient loads (e.g., Peñuelas et al., 2012; Sutton et al., 2013; Glibert, 2017).
This study has successfully tuned the “perfect beast” to experimental data sets of the harmful dinoflagellate, K. veneficum and its prey, Rhodomonas sp., under varying nutrient conditions. Temperature growth responses were added to the original ‘perfect beast’ construct, thus allowing scenarios of mixotrophic growth under both varying nutrient and temperature conditions to be explored. The modeled scenarios highlighted several distinct differences in responses of K. veneficum as an autotroph and as a mixotroph in different nutrient and temperature conditions. Both autotrophic and mixotrophic K. veneficum attained much higher biomass in non-Redfieldian nutrient conditions compared to balanced nutrient growth. The modeled results in autotrophic K. veneficum biomass were quite consistent with the experimental data from Lin et al. (2017). Thus, simple stoichiometric relationships for predicting HAB developments need careful reconsideration, particularly in eutrophic systems where the corresponding changes in nutrient ratios and forms as well as prey stoichiometry may ultimately affect cellular functions (such as C:N:P ratio) within the harmful algal mixotrophs (Mitra and Flynn, 2005, 2010). Indeed, it has been increasingly recognized that the benefits of mixotrophy to cells are synergistic, not additive (e.g., Mitra and Flynn, 2010).
In both low N:P and high N:P simulations, K. veneficum appeared to be more mixotrophic and attained higher biomass with increasing temperature compared with growth under Redfield conditions or growth as an autotroph (Figure 5). Under low N:P condition, temperature effects on mixotrophic growth rates of K. veneficum were also significant, with mixotrophic growth rates increasing faster than autotrophic growth rates at the highest temperature (i.e., 25°C; Figure 5A). This growth stimulation may imply a higher demand for C from prey. These growth patterns support the notion that mixotrophy is likely to be greater under nutrient imbalanced conditions, that is, that mixotrophy is not just a mechanism to acquire C, but also a mechanism by which nutrients are acquired (e.g., Glibert and Burkholder, 2011). For example, the assimilation efficiency (mAEmin) in the mixotrophs was the highest for those K. veneficum grown under low N:P conditions and mixed with prey in Redfield N:P conditions, indicating nutrient sources from ingested prey were required (Table 2). On the other hand, the cellular N:P of K. veneficum in simulations under high N:P was low compared to the other nutrient conditions for this mixotroph (Figure 7C). The present simulations agreed with laboratory data: the incidence of K. veneficum feeding are enhanced when ambient nutrient ratios deviated from Redfield ratio with either N and/or P deficiency depending on the cellular status of the mixotrophic dinoflagellate (Lin et al., 2017). These results also suggest that under the warmest temperatures simulated, increased growth rates of prey could contribute to an increased growth of the mixotroph (Figure 6). The growth rates of R. salina were higher than those of K. veneficum > 20°C, and thus prey availability increases faster at these temperatures. This situation may be enhanced in the environments that deviate from balanced nutrient proportions.
Outputs from these simulated scenarios have implications for growth of this HAB in eutrophic conditions in warming environments. In eutrophic estuaries such as Chesapeake Bay, there are large seasonal variations in nutrient loads and in their stoichiometry (e.g., Kemp et al., 2005; Li et al., 2015). A conceptual model was previously developed of summer blooms of K. veneficum in Chesapeake Bay that incorporates the role of prey with a high N:P ratio originating from river inputs and a source inocula of K. veneficum from southern Bay waters with a lower N:P content (Lin et al., 2018). Nutrient inputs through tributaries are greatest during the high-flow period, typically starting through March to May. During this period, prey can accumulate in the tributaries and are typically characterized by high N:P ratios due to disproportionate high N loading (Fisher et al., 1992; Kemp et al., 2005). The peak in summer K. veneficum blooms generally occurs 1–3 months later relative to these inputs, in June through September (Li et al., 2015). In this regard, according to previous laboratory experiments, growth performance of low NP-K. veneficum had a two-fold increase when fed upon prey with N-rich conditions compared to same nutrient conditions of prey (Lin et al., 2017). Thus, the enhanced growth of K. veneficum derived from the oceanic end member of the Bay may be enhanced if they encountered prey originating from the tributaries with different patterns of nutrient loading.
With accelerating climate change, mean temperatures may rise by 2–6°C by the end of the century in all seasons for Chesapeake Bay (Muhling et al., 2018). This may expand the window for K. veneficum growth in several ways. Prey availability may increase due to growth stimulation at higher temperatures. Also, recent Mid-Atlantic climate projections show that warming will likely increase current interannual variability, and that winter/spring increases in precipitation are likely (e.g., Najjar et al., 2010), bringing increased N and high N:P conditions with these flows. These wetter spring conditions, with more nutrients may lead to more N-rich and/or P-deplete prey that may further support the development of these HABs. As mixotrophs may be more temperature sensitive than their autotrophic prey, the increased temperatures could enhance their ingestion capabilities and effectively control the growth of autotrophic prey (e.g., Yang et al., 2016). The modeled biomass of K. veneficum as a mixotroph was found to achieve the highest biomass when they consumed prey under high N:P conditions (Figure 5). Interestingly, the model suggests that while the highest biomass for K. veneficum is attained at 20°C under high N:P, and falls off rapidly above 20°C, under low N:P conditions, highest biomass is attained at 25°C. These differing temperature responses raise important questions that warrant further exploration experimentally.
Using models of mixotrophy, based on food uptake and photosynthesis measurements of K. veneficum and its congener, K. armiger, and assuming constant Redfield ratios, Berge et al. (2017) predicted succession of these species and their relative investments in autotrophy and phagotrophy. Their model suggested that nutrient uptake and high investments in photosynthesis would yield high autotrophic growth rates in spring, but increased phagotrophy in summer. In another recent model, Ghyoot et al. (2017) developed a flexible model in which a distinction was made between constitutive mixotrophs, those that synthesize and maintain their chloroplasts, and non-constitutive mixtrophs, those that acquire chloroplasts. The next important step in mixotroph modeling will be to incorporate variable nutrient stoichiometry in a model of seasonal succession of both constitutive and non-constitutive mixotrophs.
In conclusion, the current study expanded modeling of mixotrophic growth to conditions of variable stoichiometry and temperature. These simulations have highlighted the consideration of particulate prey in modeling HAB dynamics under future warming; it is insufficient to only consider dissolved nutrients. The complexities shown here in consideration of differential impacts of temperature upon the growth of mixotroph predator and prey, parallel those expected under (de)eutrophication scenarios with ocean acidification (Flynn et al., 2015). Such changes in species competitive advantage under multi-stressor environments (light, temperature, pH, nutrients) will require a concerted effort in physiology-modeling research to conceptualize adequately to aid ecosystem management. Even though the current models are based only on bottom-up, nutrient conditions, and are focused on only one typical prey species without modeling the role of the toxic contents of K. veneficum in predation purpose (Sheng et al., 2010), they have provided some insight into the potential trend in HABs under future eutrophication and warming conditions.
C-HL and PG conceived and designed the experiments and analyzed the simulation model outputs. KF and AM developed the model structures. C-HL and KF performed the model tuning. PG contributed the experimental materials, reagents, and tools. CL wrote the paper with editorial contributions from all authors.
C-HL was supported by funding from the Taiwanese Government, the Bay and Rivers Fellowship from the Horn Point Laboratory, and travel support from the Ryan Saba Memorial Fellowship. Additional support for this work was provided by the National Oceanic and Atmospheric Administration National Centers for Coastal Ocean Science Competitive Research program under award no. NA17NOS4780180. This is contribution number 5519 from the University of Maryland Center for Environmental Science.
The authors declare that the research was conducted in the absence of any commercial or financial relationships that could be construed as a potential conflict of interest.
The handling Editor declared a past co-authorship with the authors KF and PG.
This work honors the memory of a friend of C-HL, Ryan Saba.
The Supplementary Material for this article can be found online at: https://www.frontiersin.org/articles/10.3389/fmars.2018.00320/full#supplementary-material
Adolf, J. E., Bachvaroff, T., and Place, A. R. (2008). Can cryptophyte abundance trigger toxic Karlodinium veneficum blooms in eutrophic estuaries? Harmful Algae 8, 119–128. doi: 10.1016/j.hal.2008.08.003
Adolf, J. E., Bachvaroff, T. R., Deeds, J. R., and Place, A. R. (2015). Ichthyotoxic Karlodinium veneficum (Ballantine) J Larsen in the upper Swan river estuary (Western Australia): ecological conditions leading to a fish kill. Harmful Algae 48, 83–93. doi: 10.1016/j.hal.2015.07.006
Anderson, D. M., Glibert, P. M., and Burkholder, J. M. (2002). Harmful algal blooms and eutrophication: nutrient sources, composition, and consequences. Estuaries 25, 704–726. doi: 10.1007/BF02804901
Baretta-Bekker, J. G., Baretta, J. W., Hansen, A. S., and Riemann, B. (1998). An improved model of carbon and nutrient dynamics in the microbial food web in marine enclosures. Aquat. Microb. Ecol. 14, 91–108. doi: 10.3354/ame014091
Berge, T., Chakraborty, S., Hansen, P. J., and Andersen, K. H. (2017). Modeling succession of key resource-harvesting traits of mixotrophic plankton. ISME J. 11, 212–223. doi: 10.1038/ismej.2016.92
Blossom, H. E., Daugbjerg, N., and Hansen, P. J. (2012). Toxic mucus traps: a novel mechanism that mediates prey uptake in the mixotrophic dinoflagellate Alexandrium pseudogonyaulax. Harmful Algae 17, 40–53. doi: 10.1016/j.hal.2012.02.010
Braarud, T. (1957). A red water organism from Walvis Bay (Gymnodinium galatheanum n. sp.). Galathea Deep Sea Exped. 1, 137–138.
Burkholder, J. M., Glibert, P. M., and Skelton, H. M. (2008). Mixotrophy, a major mode of nutrition for harmful algal species in eutrophic waters. Harmful Algae 8, 77–93. doi: 10.1016/j.hal.2008.08.010
Dai, X., Lu, D., Guan, W., Wang, H., He, P., Xia, P., et al. (2013). Newly recorded Karlodinium veneficum dinoflagellate blooms in stratified water of the East China Sea. Deep Sea Res. II 101, 237–243. doi: 10.1016/j.dsr2.2013.01.015
Deeds, J. R., Terlizzi, D. E., Adolf, J. E., Stoecker, D. K., and Place, A. R. (2002). Toxic activity from cultures of Karlodinium micrum ( = Gyrodinium galatheanum)(Dinophyceae)—a dinoflagellate associated with fish mortalities in an estuarine aquaculture facility. Harmful Algae 1, 169–189. doi: 10.1016/S1568-9883(02)00027-6
Fisher, T. R., Peele, E. R., Ammerman, J. W., and Harding Jr, L. W. (1992). Nutrient limitation of phytoplankton in Chesapeake Bay. Mar. Ecol. Prog. Ser. 82, 51–63. doi: 10.3354/meps082051
Flynn, K. J., Mitra, A., Glibert, P. M., and Burkholder, J. M. (2018). “Mixotrophy in HABs: by whom, on whom, when, why and what next,” in Global Ecology and Oceanography of Harmful Algal Blooms, eds P. M. Glibert, E. Berdalet, M. A. Burford, G. C. Pitcher, and M. Zhou (Cham: Springer Press), 113–132.
Flynn, K. J. (2001). A mechanistic model for describing dynamic multi-nutrient, light, temperature interactions in phytoplankton. J. Plankt. Res. 23, 977–997. doi: 10.1093/plankt/23.9.977
Flynn, K. J. (2005). Castles built on sand: dysfunctionality in plankton models and the inadequacy of dialogue between biologists and modellers. J. Plankt. Res. 27, 1205–1210. doi: 10.1093/plankt/fbi099
Flynn, K. J. (2009). Going for the slow burn: why should possession of a low maximum growth rate be advantageous for microalgae? Plant Ecol. Divers. 2, 179–189. doi: 10.1080/17550870903207268
Flynn, K. J. (2010). Do external resource ratios matter?: implications for modelling eutrophication events and controlling harmful algal blooms. J. Mar. Syst. 83, 170–180. doi: 10.1016/j.jmarsys.2010.04.007
Flynn, K. J. (2018). Dynamic Ecology - An Introduction to the Art of Simulating Trophic Dynamics. Swansea: Swansea University.
Flynn, K. J., Clark, D. R., Mitra, A., Fabian, H., Hansen, P. J., Glibert, P. M., et al. (2015). Ocean acidification with (de)eutrophication will alter future phytoplankton growth and succession. Proc. R. Soc. Lond. B Biol. Sci. 282:20142604. doi: 10.1098/rspb.2014.2604
Flynn, K. J., and Hansen, P. J. (2013). Cutting the canopy to defeat the “selfish gene”; conflicting selection pressures for the integration of phototrophy in mixotrophic protists. Protist 164, 811–823. doi: 10.1016/j.protis.2013.09.002
Flynn, K. J. and McGillicuddy, D. J. Jr. (2018). “Modeling marine harmful algal blooms; current status and future prospects,” in Harmful Algal Blooms: A Compendium Desk Reference, eds. S. E. Shumway, J.-A.M. Burkholder, and S. Morton (New York, NY: Wiley Science Publishers).
Flynn, K. J., and Mitra, A. (2009). Building the “perfect beast”: modelling mixotrophic plankton. J. Plankt. Res. 31, 965–992. doi: 10.1093/plankt/fbp044
Flynn, K. J., Stoecker, D. K., Mitra, A., Raven, J. A., Glibert, P. M., Hansen, P. J., et al. (2013). Misuse of the phytoplankton–zooplankton dichotomy: the need to assign organisms as mixotrophs within plankton functional types. J. Plankt. Res. 35, 3–11. doi: 10.1093/plankt/fbs062
Fu, F. X., Tatters, A. O., and Hutchins, D. A. (2012). Global change and the future of harmful algal blooms in the ocean. Mar. Ecol. Prog. Ser. 470, 207–233. doi: 10.3354/meps10047
Ghyoot, C., Flynn, K. J., Mitra, A., Lancelot, C., and Gypens, N. (2017). Modeling plankton mixotrophy: a mechanistic model consistent with the Shuter-type biochemical approach. Front. Ecol. Evol. 5:78. doi: 10.3389/fevo.2017.00078
Glibert, P. M. (2017). Eutrophication, harmful algae and biodiversity—challenging paradigms in a world of complex nutrient changes. Marine Poll. Bull. 124, 591–606. doi: 10.1016/j.marpolbul.2017.04.027
Glibert, P. M., Allen, J. I., Bouwman, A. F., Brown, C. W., Flynn, K. J., Lewitus, A. J., et al. (2010). Modeling of HABs and eutrophication: status, advances, challenges. J. Mar. Syst. 83, 262–275. doi: 10.1016/j.jmarsys.2010.05.004
Glibert, P. M., and Burford, M. A. (2017). Globally changing nutrient loads and harmful algal blooms: recent advances, new paradigms, and continuing challenges. Oceanography 30, 58–69. doi: 10.5670/oceanog.2017.110
Glibert, P. M., and Burkholder, J. M. (2011). Harmful algal blooms and eutrophication: “strategies” for nutrient uptake and growth outside the Redfield comfort zone. Chin. J. Oceanogr. Limnol. 29, 724–738. doi: 10.1007/s00343-011-0502-z
Glibert, P. M., and Burkholder, J. M. (2018). “Causes of harmful algal blooms,” in Harmful Algal Blooms: A Compendium Desk Reference, eds S. Shumway, J. M. Burkholder, and S. L. Morton (West Sussex: Wiley Press),1–21.
Glibert, P. M., Heil, C. A., Wilkerson, F. P., and Dugdale, R. C. (2018). “Nutrients and harmful algal blooms: dynamic kinetics and flexible nutrition,” in Global Ecology and Oceanography of Harmful Algal Blooms, eds P. M. Glibert, E. Berdalet, M. A. Burford, G. C. Pitcher, and M. Zhou (Cham: Springer Press), 93–112.
Glibert, P. M., Seitzinger, S., Heil, C. A., Burkholder, J. M., Parrow, M. W., Codispoti, L. A., et al. (2005). The role of eutrophication in the global proliferation of harmful algal blooms. Oceanography 18, 198–209. doi: 10.5670/oceanog.2005.54
Glibert, P. M., and Terlizzi, D. E. (1999). Cooccurrence of elevated urea levels and dinoflagellate blooms in temperate estuarine aquaculture ponds. Appl. Environ. Microbiol. 65, 5594–5596.
Guillard, R. R. (1975). “Culture of phytoplankton for feeding marine invertebrates,” in Culture of Marine Invertebrate Animals, eds W. L. Smith and M. H. Chanley (New York, NY: Plenum Press), 29–60.
Haefner, J. W. (2005). Modeling Biological Systems: Principles and Applications. Berlin: Springer Science & Business Media.
Hallegraeff, G. M. (2010). Ocean climate change, phytoplankton community responses, and harmful algal blooms: a formidable predictive challenge1. J. Phycol. 46, 220–235. doi: 10.1111/j.1529-8817.2010.00815.x
Heisler, J., Glibert, P. M., Burkholder, J. M., Anderson, D. M., Cochlan, W., Dennison, W. C., et al. (2008). Eutrophication and harmful algal blooms: a scientific consensus. Harmful Algae 8, 3–13. doi: 10.1016/j.hal.2008.08.006
Jakobsen, H. H., and Hansen, P. J. (1997). Prey size selection, grazing and growth response of the small heterotrophic dinoflagellate Gymnodinium sp. and the ciliate Balanion comatum—a comparative study. Mar. Ecol. Prog. Ser. 158, 75–86. doi: 10.3354/meps158075
Jeong, H. J., Park, J. Y., Nho, J. H., Park, M. O., Ha, J. H., Seong, K. A., et al. (2005a). Feeding by red-tide dinoflagellates on the cyanobacterium Synechococcus. Aquat. Microb. Ecol. 41, 131–143. doi: 10.3354/ame041131
Jeong, H. J., Yoo, Y. D., Park, J. Y., Song, J. Y., Kim, S. T., Lee, S. H., et al. (2005b). Feeding by phototrophic red-tide dinoflagellates: five species newly revealed and six species previously known to be mixotrophic. Aquat. Microb. Ecol. 40, 133–150. doi: 10.3354/ame040133
Jost, C., Lawrence, C. A., Campolongo, F., Van de Bund, W., Hill, S., and DeAngelis, D. L. (2004). The effects of mixotrophy on the stability and dynamics of a simple planktonic food web model. Theor. Popul. Biol. 66, 37–51. doi: 10.1016/j.tpb.2004.02.001
Kemp, W., Boynton, W., Adolf, J., Boesch, D., Boicourt, W., Brush, G., et al. (2005). Eutrophication of Chesapeake Bay: historical trends and ecological interactions. Mar. Ecol. Prog. Ser. 303, 1–29. doi: 10.3354/meps303001
Kempton, J. W., Lewitus, A. J., Deeds, J. R., Law, J. M., and Place, A. R. (2002). Toxicity of Karlodinium micrum (Dinophyceae) associated with a fish kill in a South Carolina brackish retention pond. Harmful Algae 1, 233–241. doi: 10.1016/S1568-9883(02)00015-X
Kimmance, S. A., Atkinson, D., and Montagnes, D. J. S. (2006). Do temperature–food interactions matter? Responses of production and its components in the model heterotrophic flagellate Oxyrrhis marina. Aquat. Microb. Ecol. 42, 63–73. doi: 10.3354/ame042063
Li, A., Stoecker, D. K., and Adolf, J. E. (1999). Feeding, pigmentation, photosynthesis and growth of the mixotrophic dinoflagellate Gyrodinium galatheanum. Aquat. Microb. Ecol. 19, 163–176. doi: 10.3354/ame019163
Li, A., Stoecker, D. K., and Coats, D. W. (2000). Mixotrophy in Gyrodinium galatheanum (Dinophyceae): grazing responses to light intensity and inorganic nutrients. J. Phycol. 36, 33–45. doi: 10.1046/j.1529-8817.2000.98076.x
Li, J., Glibert, P. M., and Gao, Y. (2015). Temporal and spatial changes in Chesapeake Bay water quality and relationships to Prorocentrum minimum, Karlodinium veneficum, and CyanoHAB events, 1991–2008. Harmful Algae 42, 1–14. doi: 10.1016/j.hal.2014.11.003
Lin, C.-H., Accoroni, S., and Glibert, P. M. (2017). Karlodinium veneficum feeding responses and effects on larvae of the eastern oyster Crassostrea virginica under variable nitrogen: phosphorus stoichiometry. Aquat. Microb. Ecol. 79, 101–114. doi: 10.3354/ame01823
Lin, C.-H., Lyubchich, V., and Glibert, P. M. (2018). Time series models of decadal trends in the harmful algal species Karlodinium veneficum in Chesapeake Bay. Harmful Algae 73, 110–118. doi: 10.1016/j.hal.2018.02.002
Lundgren, V. M., Glibert, P. M., Granéli, E., Vidyarathna, N. K., Fiori, E., Ou, L., et al. (2016). Metabolic and physiological changes in Prymnesium parvum when grown under, and grazing on prey of, variable nitrogen: phosphorus stoichiometry. Harmful Algae 55, 1–12. doi: 10.1016/j.hal.2016.01.002
Menden-Deuer, S., and Lessard, E. J. (2000). Carbon to volume relationships for dinoflagellates, diatoms, and other protist plankton. Limnol. Oceanogr. 45, 569–579. doi: 10.4319/lo.2000.45.3.0569
Mitra, A. (2006). A multi-nutrient model for the description of stoichiometric modulation of predation in micro-and mesozooplankton. J. Plankt. Res. 28, 597–611. doi: 10.1093/plankt/fbi144
Mitra, A., and Flynn, K. J. (2005). Predator–prey interactions: is ‘ecological stoichiometry’sufficient when good food goes bad? J. Plankt. Res. 27, 393–399. doi: 10.1093/plankt/fbi022
Mitra, A., and Flynn, K. J. (2006). Accounting for variation in prey selectivity by zooplankton. Ecol. Model. 199, 82–92. doi: 10.1016/j.ecolmodel.2006.06.013
Mitra, A., and Flynn, K. J. (2010). Modelling mixotrophy in harmful algal blooms: more or less the sum of the parts? J. Mar. Syst. 83, 158–169. doi: 10.1016/j.jmarsys.2010.04.006
Mitra, A., Flynn, K. J., Burkholder, J., Berge, T., Calbet, A., Raven, J. A., et al. (2014). The role of mixotrophic protists in the biological carbon pump. Biogeosciences 10, 13535–13562. doi: 10.5194/bg-11-995-2014
Mitra, A., Flynn, K. J., Tillmann, U., Raven, J. A., Caron, D., Stoecker, D. K., et al. (2016). Defining planktonic protist functional groups on mechanisms for energy and nutrient acquisition: incorporation of diverse mixotrophic strategies. Protist 167, 106–120. doi: 10.1016/j.protis.2016.01.003
Montagnes, D. J. S., Kimmance, S. A., and Atkinson, D. (2003). Using Q10: can growth rates increase linearly with temperature? Aquat. Microb. Ecol. 32, 307–313. doi: 10.3354/ame032307
Moore, S. K., Johnstone, J. A., Banas, N. S., and Salathe, E. P. Jr. (2015). Present-day and future climate pathways affecting Alexandrium blooms in Puget Sound, WA, USA. Harmful Algae 48, 1–11. doi: 10.1016/j.hal.2015.06.008
Muhling, B. A., Gaitán, C. F., Stock, C. A., Saba, V. S., Tommasi, D., and Dixon, K. W. (2018). Potential salinity and temperature futures for the Chesapeake Bay using a statistical downscaling spatial disaggregation framework. Estuar. Coast. 41, 349–372. doi: 10.1007/s12237-017-0280-8
Najjar, R. G., Pyke, C. R., Adams, M. B., Breitburg, D., Hershner, C., Kemp, M., et al. (2010). Potential climate-change impacts on the Chesapeake Bay. Estuar. Coast. Shelf. Sci. 86, 1–20. doi: 10.1016/j.ecss.2009.09.026
Nielsen, M. V. (1993). Toxic effect of the marine dinoflagellate Gymnodinium galatheanum on juvenile cod Gadus morhua. Mar. Ecol. Prog. Ser. 93, 273–277. doi: 10.3354/meps095273
Peñuelas, J., Sardans, J., Rivas-ubach, A., and Janssens, I. A. (2012). The human-induced imbalance between C, N and P in Earth’s life system. Glob. Change Biol. 18, 3–6. doi: 10.1111/j.1365-2486.2011.02568.x
Place, A. R., Bowers, H. A., Bachvaroff, T. R., Adolf, J. E., Deeds, J. R., and Sheng, J. (2012). Karlodinium veneficum —the little dinoflagellate with a big bite. Harmful Algae 14, 179–195. doi: 10.1016/j.hal.2011.10.021
Sheng, J., Malkiel, E., Katz, J., Adolf, J. E., and Place, A. R. (2010). A dinoflagellate exploits toxins to immobilize prey prior to ingestion. Proc. Natl. Acad. Sci. U.S.A. 107, 2082–2087. doi: 10.1073/pnas.0912254107
Stickney, H. L., Hood, R. R., and Stoecker, D. K. (2000). The impact of mixotrophy on planktonic marine ecosystems. Ecol. Model. 125, 203–230. doi: 10.1146/annurev-marine-010816-060617
Stoecker, D. K. (1998). Conceptual models of mixotrophy in planktonic protists and some ecological and evolutionary implications. Eur. J. Protistol. 34, 281–290. doi: 10.1016/S0932-4739(98)80055-2
Stoecker, D. K., Adolf, J. E., Place, A. R., Glibert, P. M., and Meritt, D. W. (2008). Effects of the dinoflagellates Karlodinium veneficum and Prorocentrum minimum on early life history stages of the eastern oyster (Crassostrea virginica). Mar. Biol. 154, 81–90. doi: 10.1007/s00227-007-0901-z
Stoecker, D. K., Hansen, P. J., Caron, D. A., and Mitra, A. (2017). Mixotrophy in the marine plankton. Annu. Rev. Mar. Sci. 9, 311–335. doi: 10.1146/annurev-marine-010816-060617
Sutton, M. A., Bleeker, A., Howard, C. M., Erisman, J. W., Abrol, Y. P., Bekunda, M., et al. (2013). Our Nutrient World. The Challenge to Produce More Food and Energy with Less Pollution. Edinburgh: Centre for Ecology & Hydrology.
Thingstad, T. F., Havskum, H., Garde, K., and Riemann, B. (1996). On the strategy of “eating your competitor”: a mathematical analysis of algal mixotrophy. Ecology 77, 2108–2118. doi: 10.2307/2265705
Våge, S., Castellani, M., Giske, J., and Thingstad, T. F. (2013). Successful strategies in size structured mixotrophic food webs. Aquat. Ecol. 47, 329–347. doi: 10.1007/s10452-013-9447-y
Van Dolah, F. M. (2000). Marine algal toxins: origins, health effects, and their increased occurrence. Environ. Health Perspect. 108, 133–141.
Ward, B. A., Dutkiewicz, S., Barton, A. D., and Follows, M. J. (2011). Biophysical aspects of resource acquisition and competition in algal mixotrophs. Am. Nat. 178, 98–112. doi: 10.1086/660284
Ward, B. A., and Follows, M. J. (2016). Marine mixotrophy increases trophic transfer efficiency, mean organism size, and vertical carbon flux. Proc. Natl. Acad. Sci. U.S.A. 113, 2958–2963. doi: 10.1073/pnas.1517118113
Wells, M. L., Trainer, V. L., Smayda, T. J., Karlson, B. S. O., Trick, C. G., Kudela, R. M., et al. (2015). Harmful algal blooms and climate change: learning from the past and present to forecast the future. Harmful Algae 49, 68–93. doi: 10.1016/j.hal.2015.07.009
Wilken, S., Huisman, J., Naus-Wiezer, S., and Donk, E. (2013). Mixotrophic organisms become more heterotrophic with rising temperature. Ecol. Lett. 16, 225–233. doi: 10.1111/ele.12033
Yang, Z., Zhang, L., Zhu, X., Wang, J., and Montagnes, D. J. S. (2016). An evidence-based framework for predicting the impact of differing autotroph-heterotroph thermal sensitivities on consumer–prey dynamics. ISME J. 10, 1767–1778. doi: 10.1038/ismej.2015.225
Keywords: mixotrophy, Karlodinium veneficum, Rhodomonas salina, harmful algal blooms, mathematical model, stoichiometry, temperature responses
Citation: Lin C-H, Flynn KJ, Mitra A and Glibert PM (2018) Simulating Effects of Variable Stoichiometry and Temperature on Mixotrophy in the Harmful Dinoflagellate Karlodinium veneficum. Front. Mar. Sci. 5:320. doi: 10.3389/fmars.2018.00320
Received: 28 March 2018; Accepted: 22 August 2018;
Published: 10 September 2018.
Edited by:
Matthew D. Johnson, Woods Hole Oceanographic Institution, United StatesReviewed by:
Lasse Tor Nielsen, Technical University of Denmark, DenmarkCopyright © 2018 Lin, Flynn, Mitra and Glibert. This is an open-access article distributed under the terms of the Creative Commons Attribution License (CC BY). The use, distribution or reproduction in other forums is permitted, provided the original author(s) and the copyright owner(s) are credited and that the original publication in this journal is cited, in accordance with accepted academic practice. No use, distribution or reproduction is permitted which does not comply with these terms.
*Correspondence: Kevin J. Flynn, ay5qLmZseW5uQHN3YW5zZWEuYWMudWs=
Disclaimer: All claims expressed in this article are solely those of the authors and do not necessarily represent those of their affiliated organizations, or those of the publisher, the editors and the reviewers. Any product that may be evaluated in this article or claim that may be made by its manufacturer is not guaranteed or endorsed by the publisher.
Research integrity at Frontiers
Learn more about the work of our research integrity team to safeguard the quality of each article we publish.