- 1Australian Institute of Marine Science, Indian Ocean Marine Research Centre, University of Western Australia, Crawley, WA, Australia
- 2Instituto de Física Interdisciplinar y Sistemas Complejos (CSIC-UIB), Palma de Mallorca, Spain
- 3IOMRC and The UWA Oceans Institute, School of Biological Sciences, University of Western Australia, Crawley, WA, Australia
- 4Biological and Environmental Sciences and Engineering, Red Sea Research Center, King Abdullah University of Science and Technology, Thuwal, Saudi Arabia
The rise of the internet coupled with technological innovations such as smartphones have generated massive volumes of geo-referenced data (big data) on human mobility. This has allowed the number of studies of human mobility to rapidly overtake those of animal movement. Today, telemetry studies of animals are also approaching big data status. Here, we review recent advances in studies of human mobility and identify the opportunities they present for advancing our understanding of animal movement. We describe key analytical techniques, potential bottlenecks and a roadmap for progress toward a synthesis of movement patterns of wild animals.
Introduction
The movements of wild animals have always fascinated humans. Animal migrations have been important milestones for human society, from the arrival or departure of migratory birds signaling changes in seasons, to hunter-gatherers following the movement of herds across steppes and savannahs and fishermen following salmon runs and the progress of fish stocks between feeding and breeding grounds. Animal migrations have also been an integral part of the development of human culture, as evidenced by pictures drawn thousands of years ago on cave walls. Today, the study of the ecology of non-human animal (hereafter, animal) movement is a well-established field of science (Nathan, 2008) encompassing a coherent research community with dedicated publication outlets (e.g., Movement Ecology, http://link.springer.com/journal/40462 and Animal Biotelemetry https://link.springer.com/journal/40317) and symposia largely dedicated to animal movement (https://www.bio-logging.net/Symposium/).
Despite our long-standing interest, description of the movement patterns of some animals, particularly birds, and aquatic species such as marine mammals and fishes have presented many challenges, largely because these animals live in environments where humans cannot easily follow their path. Today, these issues are being overcome through the development of sophisticated telemetry technologies that allow researchers to remotely locate and track animals. Over the last 30 years, such telemetry studies have generated insights into the otherwise invisible lives of the animals that occupy the skies, the forests and the open oceans well-beyond our sight.
Given the effort that has been expended on describing the movement patterns of animals for nearly two centuries (Figure 1), it is somewhat ironic that humans have become the subject of tracking studies only very recently (Brockmann et al., 2006; Eagle and Pentland, 2006; Gonzalez et al., 2008) (Figure 1). Human tracking studies have been enabled by the growth of the internet coupled with technological innovations such as smartphones and wearables (e.g., smart watches and fitness trackers) that have generated immense and readily accessible geo-referenced data on human mobility and data on human activities such as heart rate monitoring, sports, and sleep tracking (de Arriba-Pérez et al., 2016). These large datasets, amounting in volume to “big data,” are now being analyzed to describe patterns of human movement (Simini et al., 2012, 2013), features (e.g., sleep, stress, and activities) (de Arriba-Pérez et al., 2016) and interactions (Simini et al., 2012, 2013; Meekan et al., 2017) with a degree of detail, immediacy and precision that was never before possible for any animal species (Meekan et al., 2017). Moreover, such studies have characterized the movement patterns of humans at global scales for the first time (e.g., Brockmann et al., 2006; Gonzalez et al., 2008).
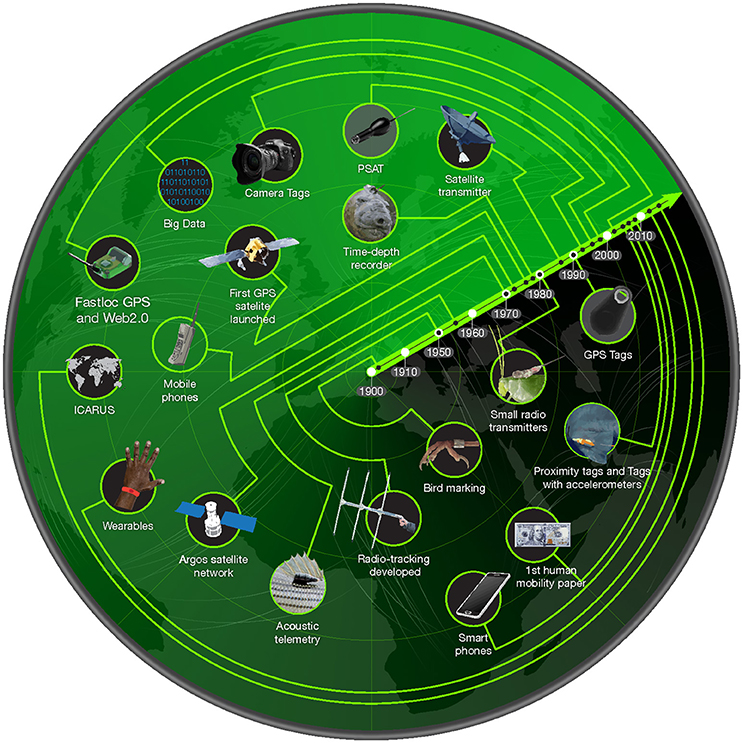
Figure 1. Timeline of Technological Advances in Animal Movement and Human Mobility. The timeline shows the technological advances from animal movement and human mobility research since 1900 to present. Pop-up satellite archival tags (PSATs) are data loggers with a means to transmit the collected data via satellite developed for gill-breathing animals that spend little time at the surface. The International Cooperation for Animal Research Using Space Initiative (ICARUS) is a new animal tracking antenna on the International Space Station that would allow smaller tags to send data back through the low-orbit satellite. The dollar bill represents the first published paper on human mobility, which tracked records of dollar bills across the United States as a proxy for human movement (Brockmann et al., 2006). GPS = global positioning system. Reprinted from Trends in Ecology and Evolution (see Meekan et al., 2017), with permission from Elsevier.
The rapid uptake of telemetry for the study of wild animals means that big data approaches to understand movement can now be extended beyond human subjects. Collaborative research initiatives such as the Tagging of Pacific Pelagics (TOPP) program (http://www.gtopp.org/), and online repositories such as the Ocean Tracking Network (OTN) (http://oceantrackingnetwork.org/), Movebank (Wikelski and Kays, 2010), ZoaTrack (Dwyer et al., 2015) and Birdlife International (http://www.birdlife.org/) (see Campbell et al., 2016 for a full list of repositories), collectively document the movements of tens to hundreds of thousands of wild animals across diverse taxa spanning all continents and biomes (Hussey et al., 2015; Kays et al., 2015). Such datasets now offer the opportunity to transfer big data analytical approaches developed in the field of human mobility to animal movement ecology. As has been pointed out for the field of ecology in general (Hampton et al., 2013), big data analyses have the ability to promote significant advances in our understanding of animal movement ecology, including insights challenging the limits of current theoretical frameworks, by searching for and describing universal patterns, collective behaviors and emergent properties in both terrestrial and marine ecosystems.
Here, we show how developments in human mobility research underpinned by big data analysis (Blondel et al., 2015) can be used to catalyze progress and derive new insights into the global movement patterns of animals. A key requirement for this task will be the creation of global, open access databases of animal tracking. These will not only improve our understanding of the movement ecology of animals, but also provide the opportunity to engage researchers from the broader scientific community, including physicists, mathematicians, computer, and visualization scientists and those interested in complex systems.
Technology as a Driver for the Development of Tracking Animals and Humans
The emergence of the modern study of animal movement can be traced to the development of ring banding in the 1900's (Bairlein, 2001) and radio-transmitter telemetry in the 1950's (LeMunyan et al., 1959) (Figure 1). At the first iteration of the latter technique, radio signals emitted by transmitters deployed on animals were detected by receivers carried by researchers or mounted on platforms so that tagged animals could be located by triangulation of the signals from multiple receivers. Tracking programs were thus limited by the range of the receivers (25–35 km; line of sight). The launch of the ARGOS (Advanced Research and Global observation satellite) satellite network in the late 1970s overcame this problem, as receivers were placed in earth-orbiting satellites and by the 1980's, animals were tracked with satellite transmitters for the first time (Schweinsburg and Lee, 1982) (Figure 1). In subsequent years, satellite telemetry has progressed rapidly with miniaturization of electronics, improved battery capacity and the integration of the Global Positioning System (GPS), allowing position estimates with much lower error (Dujon et al., 2014) and faster acquisition of satellite data. Today these satellite tags have been attached to a range of terrestrial and marine animals and have catalyzed discovery (Hussey et al., 2015; Kays et al., 2015). Although understanding where animals go has been the main focus of telemetry studies, advanced tags now incorporate sensors that report information on behavior, physiological status (See Brown et al., 2013) and environmental conditions experienced by an animal during its movements (See Biuw et al., 2007). At the same time, telemetry techniques have also been developed to track aquatic organisms such as fish that do not return to the surface to breathe. Because radio signals are rapidly attenuated by water, part of this technology has focused on the use of sonar-emitting tags that are deployed either externally or internally on animals. Signals from the tags are detected and recorded at receiver stations that are now spread in networks through parts of the world's oceans (see http://oceantrackingnetwork.org/; Cooke et al., 2011; Hoenner et al., 2018).
Telemetry was first used in the marine environment, due to the fact that the ocean is a boundary to human observation of marine animals (Boyd et al., 2004). For example the first time-depth recorders were attached to Weddell seals (Kooyman, 1965) and innovations continue to come from the marine biology field such as the multi-sensor “daily diary” tag (Wilson et al., 2008), and other technological developments such as the CTD-SRDL tag which samples oceanographic variables experienced by tagged animals at the same time as monitoring their movements (Fedak, 2004).
In contrast to the long history of animal movement, one of the first studies of individual human mobility occurred as late as 2006, and tracked the movement patterns of 100 MIT students based on the locations of the cell towers from which their mobile phone calls were made (Eagle and Pentland, 2006). The first continent-wide study of human mobility was published in the same year (Brockmann et al., 2006) and used a crowd-sourcing approach, with individuals voluntarily reporting the location of marked $1 bills across the United States (see wheresgeorge.com). However, the appearance of smartphones (portable technologies with geolocation capability) is the milestone that allowed researchers to study human mobility at truly global scales and in unprecedented detail. Integrated GPS tracking in smartphones together with data provided by geolocated internet posts via text or photographs via twitter and the Flickr photo sharing platform, public transportation cards and credit cards are now providing direct and high-resolution data on human locations, trajectories, opinions, and interactions, allowing researchers to develop and validate models of human mobility across different spatial scales (e.g., city to country, Simini et al., 2012). The importance of social interactions to human individuals has created our willingness to carry our own tags (e.g., smartphones), pay for the associated costs, and document our activity through social networks, underpinning the rapid data expansion on human mobility. The numbers of internet-connected electronic devices currently in use, such as smartphones and tablets, are estimated to be in the range of 8–10 billion, i.e., more than the entire human population of the planet (Cisco, 2015). Thus, despite the relatively short history of research on human mobility compared to animal movement, studies of human mobility have recently begun to match or outstrip the number of publications in the field of animal movement today (Figure 2). They also allow, for the first time, tracking the majority of the individuals of a single very abundant species—humans.
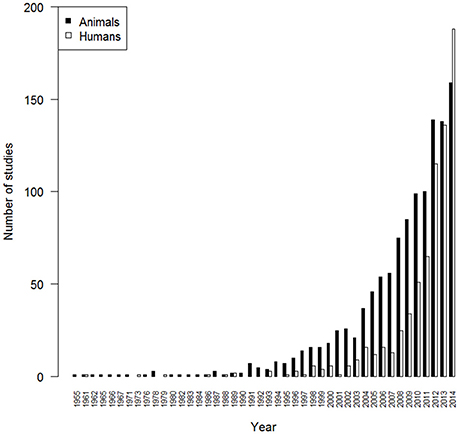
Figure 2. Comparison of output from animal movement and human mobility research. Annual publication output in wild animal movement studies (black), and human mobility research (white). Data from ISI® Web of Knowledge accessed June 1, 2015 using—“animal tracking” OR “animal movement” OR “animal telemetry,” and—“human tracking” OR “human mobility,” as search terms from 1945 to 2014. Search results from human mobility research were vetted for studies related to the biomechanics of human mobility and these were deleted.
Since human dispersal was able to be tracked at the level of individuals (Brockmann et al., 2006; Eagle and Pentland, 2006) it has been found that our trajectories are similar to those of animals such as albatrosses (Viswanathan et al., 1996), monkeys (Ramos-Fernández et al., 2004), and a range of marine predators (Sims et al., 2008). All of these studies showed that trajectories are approximated by a Levy flight—a random walk for which step size follows a power-law distribution (y = x−k) with the exponent k < 2. While the existence of Levy flights in animal and human movement data remains controversial (Reynolds, 2008; Edwards, 2011; Petrovskii et al., 2011; Gautestad and Mysterud, 2013; Pyke, 2015) our main point is simply that, as dispersing agents, both humans or animals, travel only short distances most of the time, but occasionally travel very long distances. Other similarities include the collective movement of pedestrians showing synchronization (Helbing and Molnár, 1995; Vicsek et al., 1995) similar to flocks of birds (Cavagna et al., 2015), herds of ungulates and schools of fish (Toner and Tu, 1998; Vicsek and Zafeiris, 2012). Given that we are now attaining situations where descriptions of both human and animal movements are data-rich and we have recognized the potential similarities between their form and underlying motivations (Meekan et al., 2017), it is an appropriate time to examine, test and apply techniques used to analyse large-scale (e.g., regional to global scale) data on human mobility to animal movement.
In the last decade alone, more than 700,000 ARGOS-linked satellite transmitters have been deployed on animals (Figure 3). These devices are the main platform currently in use by animal movement ecologists (at least in marine systems for mammals, birds, and reptiles; Hussey et al., 2015) and also transmits the data to calculate GPS fixes for tags that are equipped with GPS technology. Although this represents only a tiny fraction of the number of internet-connected electronic devices that are used to track humans (i.e., currently greater than the number of humans on the planet), the outputs of satellite tag deployments on animals are now also approaching big data status (Figure 3). Given that routine satellite tracking has a 30-year history and that these totals do not include non-Argos linked devices [e.g., radio and acoustic telemetry (the main technology for teleost fishes), and biologging devices], we conservatively estimate that there have been more than one million deployments of satellite-linked tags on animals since the beginning of this field of research and these deployments have global coverage (Kays et al., 2015). However, only a small fraction of these data reside in online databases and even less reside in databases that are publically accessible. For example in a review of the literature from 2000 to 2012 in Australasia alone, animal telemetry devices (including all types) were deployed on 12,656 animals and only 9% of these had their data stored in a discoverable and accessible manner (Campbell et al., 2007).
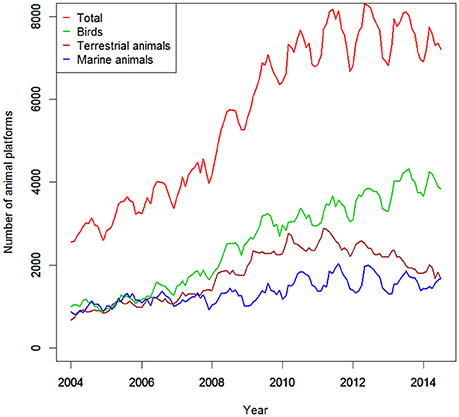
Figure 3. Number of ARGOS satellite transmitters deployed. Time series of the number of ARGOS satellite transmitters deployed from 2004 to 2014 (data provided by CLS ARGOS).
How can Movement Ecology Benefit from Big Data?
A major challenge for animal movement ecology is the cost of tracking devices. Depending on the number of sensors fitted to the satellite-linked tag, most cost somewhere in the range of USD $1,000–$10,000, with satellite communication time an additional impost. High instrument costs generally result in low numbers of tagged animals, with sample sizes further reduced by tag loss and the failure of some tags to report position data (Hays et al., 2007). Combined with the logistics involved in capturing target organisms, it is perhaps not surprising that many researchers are reluctant to share data. However, one of the consequences of low sample sizes is that tracking datasets generally feature a large amount of variation in movements among species, individuals, sexes, sites, and seasons, which further complicates the identification of general patterns. Pooling of datasets among studies through the formation of synthesis groups and open access repositories could provide a means for researchers to escape the constraint of small sample sizes and move analyses from local to larger spatial and temporal scales and from the specific to the generic in their application to the field. Progress in the study of human mobility shows that this would generate four key opportunities for utilizing big data approaches which we outline below; (1) identification of emergent properties in animal movement, (2) analysis of networks of animal movement and behavior, (3) development of machine learning algorithms to understand and characterize patterns from “big” animal movement data and (4) advanced visualization techniques for complex datasets of movement. We do not attempt to review all the methodological developments in each of the human mobility and animal movement fields, but instead focus on these four specific areas that we believe can provide valuable new insight into animal movement.
Identification of Emergent Patterns in Animal Movement
The simultaneous analysis of multiple human trajectories has revealed emergent patterns of human organization at multiple scales, ranging from communities to societies in both decentralized and centralized (complex, stratified) forms. Initially, patterns of human mobility were explained using a gravity model (Stouffer, 1940; Zipf, 1946) where the flux of people between two locations was considered proportional to the importance of the source (starting point) and destination in terms of population size, gross domestic product, etc., and decayed with the distance between locations. This concept has since been superseded by more elaborate radiation models, which describe the flows of people between different locations, but in addition to the importance of source and destination, also consider the importance of the pathway used to travel between the locations. One of the advantages of this latter approach is that it contains no adjustable parameters. Instead, it does make the basic assumption that people move in order to access resources. In the case of humans, the most obvious “resource” is employment (Simini et al., 2012, 2013). Population size has been used as a measure of the “quality” of resources since areas with larger populations (e.g., cities) tend to offer greater opportunities for employment. A benefit of such models is that they can predict commuting and transport patterns, even in areas where such data are not routinely collected, as they rely only on population densities (Simini et al., 2012).
Animals, like humans move at both small and large scales (i.e., they have daily and seasonal movements). For example, many animals have large separation between their breeding grounds and foraging grounds and the migration between the two is commonly conducted on an annual basis. Movement within the breeding and foraging ground contains smaller daily movements akin to human commuting patterns, such as movement over one to a few days of parents tending young; so called central place foraging, where the young are left at the breeding ground while the parent obtains resources to nourish them. However, we lack similar predictive models for animals, potentially for two main reasons: firstly, most studies have yet to shift from a focus on single trajectories or those of a small set of animals (with some notable exceptions such as Block et al., 2011; Raymond et al., 2015; Sequeira et al., in press) to research that examines the collective movement patterns of a species or entire populations. Secondly, the key resource(s) that drives animal movement patterns are largely hidden from the human observer (Getz and Saltz, 2008). Food is often considered the main driver, but resources can also include refuges or mates for reproduction, issues that are also relevant to human movement. Assuming that food is indeed the main resource driving animal movement, large data sets could allow formulation of a radiation model. As mentioned above, the human population density of a location is taken as a proxy of the quality of resources, so for animals this could be existing proxies of prey density like Chlorophyll-a for example. Step selection functions (Fortin et al., 2005) and resource selection functions (Manly et al., 2007) may also be of relevance here for identifying key resources. These functions have previously been used with telemetry data as the input (Schick et al., 2008) and are useful in animal ecology because they provide insight into the mechanisms behind animal distribution (Cagnacci et al., 2010) by contrasting resources/habitat used against those available. Then as for humans, the radiation model approach with “big” animal data will be capable of predicting fluxes of animals in places where there are no observations, information that would be extremely useful for conservation management.
Sensors for assessing behavior and for documenting the internal and external states of animals now also provide a means for identifying and quantifying the context and drivers of animal movement (see review by Brown et al., 2013). For example, the “daily diary” tag (https://wildlifecomputers.com/our-tags/daily-diary/) has a tri-axial accelerometer, providing high resolution data (32 sec−1) on movement on three axes (pitch, roll, and heading). Where previous work has matched behaviors to accelerometer signals, such information allows movements to be related to specific behaviors since foraging, sleeping, running, etc. produce characteristic patterns of acceleration (see Nielsen et al., 2010). Indeed, progress in inferring patterns of human movements from accelerometer data have advanced to the extent that they can be used to infer specific gestures (Liu et al., 2009) and gait patterns allowing individual recognition (Mantyjarvi et al., 2005). Similarly, the CTD-SRDL tag records location and diving profiles of marine vertebrates. As the animal dives, the tag samples the water column for conductivity, temperature, and depth. Animals carrying these tags effectively become autonomous environmental samplers (Costa et al., 2003) gathering large data sets on both the physical and biological conditions they are experiencing (Fedak, 2004) and their own physiological state along their movement trajectories (Biuw et al., 2007). These types of tags and the contextual data sets that they generate provide the benchmark for studies of animal movement and their use will become more widespread in the future as technology improves and costs decline. Technological advances will likely include an improvement in the amount of data that can be recorded (and potentially transmitted) and miniaiturization, with the latter allowing for a range of smaller animals to become the targets for tagging. For example, the ICARUS initiative (http://icarusinitiative.org/) is working to mount a new animal tracking antenna on the International Space Station that would allow smaller tags (currently 5 g) to send data back through the low-orbit satellite, allowing the tagging of very small birds and even large insects.
Analysis of Networks of Animal Movement and Behavior
On a daily basis, the city is one of the most important scales for the organization of human movement. Geolocalized data from phones shows that most travel occurs between the home and the workplace, with the pathways channeling the flux of people between these locations being a key feature of a city (Louail et al., 2015). Analyses of commuting patterns have shown that, rather than unicentric, cities are polycentric with several coexisting centers (Kloosterman and Musterd, 2001; Roth et al., 2011), information that is highly relevant for urban planning. Given that many animals also commute, for example from breeding and/or refuges to foraging sites, the use of a similar analytical approach including residency analysis coupled with origin-destination matrices (Louail et al., 2015) would allow for the spatial properties of these animal commuting flows to be revealed in a novel manner. Such an approach to the analysis of habitat use by animal populations would complement existing methods such as switching state-space models that infer behavioral state (i.e., migrating vs. foraging) (Jonsen et al., 2013), to allow comparisons of the commuting flows of a species from a range of breeding sites. These comparisons could identify sub-populations at risk where the distance or time of commuting was increasing over time, perhaps as a result of environmental change. The state-space models mentioned above are a common and very useful approach for analyzing animal tracking data. Not only do they infer behavioral state, they estimate the parameters of their distributions and importantly, they also provide uncertainty information around the location estimates as these can be subject to severe errors (Patterson et al., 2008), unlike human tracking data. In addition, they intrinsically account for the inherent autocorrelation present in animal movement data. Despite this not being a common feature of network models, they are being developed in order that they account for memory and thus deal also with autocorrelation indirectly (Salnikov et al., 2016). Although there have been some advances in techniques to allow population level inference from state space and other hierarchical models (Hooten et al., 2016; Jonsen, 2016) they are not widely used for this purpose, potentially because of their additional complexity and computational burden (Hooten et al., 2017). However advances in software such as STAN, C++, Template Model Builder (TMB), and Integrated Nested Laplace Approximation (INLA) could assist here as could use of Cloud computing using platforms like Amazon Web Services and others.
At a larger spatial scale (100s of km), the extent to which human activity patterns correspond to administrative boundaries has been examined by applying algorithms of topological community detection to country-wide telephone call networks (Sobolevsky et al., 2013) and patterns in the circulation of bank notes (Thiemann et al., 2010). These studies typically report poor matches between administrative boundaries and actual geographic boundaries calculated from human mobility data. For humans, the economic and social implications of poorly defined borders and sizes of administrative regions are obvious. But such community detection algorithms also have clear applicability to animal tracking data, particularly in the case of highly mobile, migratory species, because their range is likely to encompass different political jurisdictions, management regimes and potential threats (Dallimer and Strange, 2015). One of the most common aims of the analysis of tracking data of animals is the identification of biologically important areas such as those used for breeding and foraging and migratory corridors, as these areas are often the focus for species conservation and management. Common approaches to this task are home range analysis (e.g., kernel density; Worton, 1989) and spatially explicit time-in-area analyses (Hemson et al., 2005). However, both have difficulty identifying areas that are critical but infrequently occupied, such as migratory corridors. While more advanced methods exist in animal movement ecology to deal with these limitations such as Brownian (Horne et al., 2007) and biased bridges (Benhamou, 2011) these methods are undertaken on individual trajectories and scaling up to population level inference requires a representative sample of individual tracks and secondary analyses (e.g., overlaying home ranges in GIS software, use of random effects for parameters). Community detection algorithms used to represent patterns of human space use may be ideal for this task, as they are not subject to the same limitation and importantly, they can also determine how sub-populations might be connected at larger spatial scales (Rodríguez et al., 2017). Again, the power of these algorithms relies on the use of massive data that examines the movements of hundreds of individuals across ecosystems (e.g., Rodríguez et al., 2017), an approach that is still relatively uncommon in animal studies. At a wider scale, such analyses would aid the development of effective conservation and management across socio-political borders. In human societies, community detection algorithms have also provided insight into processes such as the spread of emergent infectious diseases (Colizza et al., 2006). A combination of human mobility (air travel and daily commuting patterns) and demographic data has enabled the development of models of the worldwide spread of epidemic diseases (Balcan et al., 2009) that have been ground-truthed with empirical data from surveillance and virologic sources (Tizzoni et al., 2012). Similarly, community detection algorithms could provide information for the management of disease outbreaks in animals, particularly for migratory species (e.g., Russell et al., 2005). Indeed, there may be overlap between both human and animal data in situations where animal migrations can enhance the global spread of pathogens (Altizer et al., 2011), for example, zoonotic pathogens such as the Ebola virus in bats (Leroy et al., 2009).
In addition to diseases, the propagation of ideas, opinions and innovations can also be followed within societies using data now available via social media. For example, geolocated messages posted on twitter (tweets) have allowed researchers to measure the overall happiness and moods of people (Mitchell et al., 2013) and to characterize the worldwide patterns in linguistic geography (Mocanu et al., 2013). The availability of such datasets has opened up enormous potential for the near real-time study of human behavior and societal trends at very large spatial scales (1,000's of km) (Mocanu et al., 2013). Developments in tagging technology may soon also enable behavioral analyses of animals, including cultural transmission among individuals. For example, understanding the effects of social interactions of animals on movement ecology has been highlighted as a key research question (Hays et al., 2016). Data from proximity tags recording the proximity and duration of encounters between tagged animals and captured via download to networks of radio (see Rutz et al., 2012) or acoustic (Holland et al., 2009; Lidgard et al., 2014) receivers, can be used to map network topologies. Such maps will allow the depiction of social interactions among animals and show how cultural information diffuses and diversifies in societies of wild animals (Rutz et al., 2012). At present, limitations of the technology restrict the use of these tags to animals that live in small groups (10s of individuals) and move over limited ranges (10s of km), but miniaturization of the technology and declining costs mean that this approach will become increasingly popular and could eventually lead to the application of the technology to entire local populations (Krause et al., 2013). In turn, this will allow an understanding of social structure at the level of populations (Krause et al., 2013). Depending on how we define an encounter, or association between individuals, a network approach could be applied to these data to identify associations among and within communities and species, such as predator-prey relationships. This goal is considered a critical next step in animal movement ecology (Hussey et al., 2015; Kays et al., 2015).
Development of Machine Learning Algorithms to Understand and Characterize Patterns from “Big” Animal Movement Data
A benefit of “big data” approaches is the increased opportunity to apply machine learning algorithms to learn from data in order to formulate predictions or classify subjects of analysis. Such methods have already been used widely in the study of animal movement, particularly where the goal has been to categorize a set of data inputs into groups. Some examples include categorizing the different behaviors in diving profiles of aquatic animals from time-depth recorders (Schreer and Testa, 1995; Schreer et al., 2001; Thums et al., 2008) and from accelerometry data (Watanabe et al., 2005; Sakamoto et al., 2009; Nielsen et al., 2010; Nathan et al., 2012). Comparable applications exist in studies of human behavior categorized using data from accelerometers in wearable devices and smartphones (Casale et al., 2011; Kwapisz et al., 2011). However, the power of machine learning approaches increases sharply with the volume of available data, which has grown to be orders-of-magnitude greater for human subjects than for any other animal species.
The methods mentioned above are supervised machine learning techniques where the focus of the analysis is on prediction, based on known properties learned from the training data. Machine learning can also be un-supervised, where the focus is on finding some structure in the input or the discovery of hidden patterns in the data. Hidden Markov models are examples of one class of unsupervised machine learning algorithm commonly used to predict or classify future states from animal and human (e.g., Ashbrook and Starner, 2003) tracking data. For example, in the case of animals, such states include spatial location and a behavioral mode such as migrating or foraging (Patterson et al., 2008). An approach of potential use for animal movement is where kernel density estimation was used to estimate the probability that an individual will be at a given location at a specific time in the future, by using both spatial and temporal information via multiple kernel functions derived from smartphone data (Do et al., 2015). Although machine learning approaches are already used in animal movement studies, they are usually limited by the familiar problem of low sample size. Pooling of data among studies may provide the information required to apply these data-hungry techniques to investigate patterns at population and community scales. Advances will be required to deal with the datasets (see Brown et al., 2013) provided by acceleration and magnetometer sensors now coupled with GPS in animal-borne tags, which provide an opportunity to retrieve valuable insights on the links between animal condition, behavior, and movement and the environment (Goto et al., 2017). This process has already commenced in studies of human mobility where machine-learning algorithms applied to data from mobile devices customizes their services to the needs and circumstances of each individual and across many individuals to yield individualized assessments of condition (Jordan and Mitchell, 2015). A related approach to machine learning, known as the “inverse problem” approach calculates the causal factors from a set of observations. For example, time series data recorded by high resolution GPS loggers attached to seabirds has been used to understand how the environment impacts movement by simultaneously estimating navigational decision making (the animal heading) and the influence of external factors (ocean winds) on their flight patterns (Goto et al., 2017). These approaches applied to big data sets would allow for an understanding of navigational decision making across a range of species and a range of environmental factors such as wind, currents and other oceanographic drivers.
Advanced Visualization Techniques for Complex Datasets of Movement
Visualization of human mobility data is a major challenge because it requires access to appropriate computational tools for displaying the large amounts of location and sensor data and associated attributes of the physical and biological environment. This problem is particularly acute in the study of community networks of human mobility (Giannotti et al., 2011). For example, advanced analytical approaches are yielding a number of supporting tools to visualize and analyze massive datasets of human trajectories in order to classify places as home, work or locations of social activities (Andrienko et al., 2015) and identify hotspots of other activities (Shen et al., 2015). A number of these advanced tools are now freely available and could be used to further our understanding of animal movement patterns. For example, software such as the Wireless Rope has been developed to allow the real-time and dynamic visualization of devices connected by Bluetooth links (Nicolai et al., 2006). In turn, this allows examination of interactions among the subjects carrying these devices. Similarly, MAPMOLTY (MAPping MObility loyaLTY) is a web-based visualization tool that uses human mobility data and a set of points of interest to compute a number of quantitative indicators that show the loyalty of humans to these points and to depict such relationships on a map (de Lira et al., 2014). ImMens also supports interactive visual exploration of large datasets including geographical information (Liu et al., 2013), while GLEAMviz (Van den Broeck et al., 2011) offers an environment to simulate models of spread of disease in combination with integrated mobility patterns of humans. Use of the latter program is not just confined to the study of disease and could be applied to animal movement. All of these visualization tools (see also: http://www.creativebloq.com/design-tools/data-visualization-712402) developed to visualize and analyse human movement data offer ecologists a means to make sense of increasingly large and complex datasets provided both by the sensors contained in modern tracking devices and the growing datasets that have tagged multiple individuals and species across a range of life history stages.
Challenges of the Big Data Approach to Animal Tracking
Although there has been significant progress on the synthesis of some of the larger animal tracking data sets (e.g., TOPP, Block et al., 2011; Sequeira et al., in press), for the most part, these data do not reside in repositories that are open to the general scientific community, even after publication. A lack of easy access complicates and hinders any attempt to search for general patterns in animal movement and document large-scale movement patterns. This major impediment to research is well-recognized (Campbell et al., 2007; Rutz and Hays, 2009; Hays, 2014; Hussey et al., 2015; Kays et al., 2015) and some attempts have been made to rectify it, notably through initiatives such as Movebank (Wikelski and Kays, 2010) (see Campbell et al., 2016 for a full list of repositories), however they do not provide open access to data. In addition, these data facilities are almost invariably focused on one group of animals (in the case of Movebank, birds) and thus represent only a small fraction of existing data. The opportunity to seek and explore general patterns and underlying principles in animal movement is consequently limited to the taxa contained in the database. Clearly, open access to tracking data represents a major bottleneck to the advance of animal movement ecology and to the realization of the full value of the investment in time and money that many thousands of individual researchers and institutions have made in collecting these data over the last decades. Development of a culture to deposit the data in open-access data repositories as a resource for the research community, as is now the case in many fields of biology such as genetics (Mount, 2004), must be a critical priority for all grant agencies, government institutions and commercial entities that invest in this type of research. Some progress is now being made on this issue with journals that are the primary outlets of this type of research insisting that data sets on which results are based also be published online (e.g., Nature), mostly driven by efforts to address the current crisis of reproducibility of results. Although initiatives such as Movebank may shift the field toward an open access model, such a global, one-size-fits-all storage facility may be difficult to manage in the long term and perhaps regional data repositories such as ZoaTrack (Dwyer et al., 2015) may be less challenging to implement (Campbell et al., 2007). In the latter, data become open access after an initial moratorium period. Such an approach has also been successful for the Integrated Marine Observing System (IMOS) Animal Tracking Database (http://imos.org.au/facilities/animaltracking/). Open access datasets need to be coupled with appropriate, standardized metadata providing the important contextual information on the tracking data (Campbell et al., 2016). For example, details of the season, sex and maturity status of the animal tracked and details of the type of tag and its program (e.g., duty cycles, etc.) should be included. Also important is the need to include details on the data processing and provide access to the raw datasets. Such constraints are not trivial and databases will need careful consideration of how to standardize the collection of such data in order to provide the context needed to facilitate big data analyses, without this being onerous to data owners. Campbell et al. (2016) provides details of the data reporting standards that should be implemented for data collected by animal borne telemetry devices. However, such challenges are not unique to ecology and standardized, machine readable meta-data software (e.g., Morpho) might assist here (Hampton et al., 2013). Hampton et al. (2013) provide an informative list of action items for ecologists to ensure their data endure for future big data analyses and Raffaelli et al. (2014) provide lessons to be learnt from previous attempts to analyse and organize big data for ecology. Open access databases combined with the availability of supercomputing resources through cloud computing services such as Amazon Web services and others will make big data analyses more accessible to a larger community of researchers.
The amount of accessible digital data and the ease by which it can be collected on nearly any aspect of human activity has shifted research from a theory and hypothesis-led basis to one that is data-driven. These approaches are not necessarily incompatible (Smalheiser, 2002) and need not represent a threat to the study of animal movement ecology. Big data can be used to formulate new hypotheses that could not be tested in the past due to lack of data or conceptual constraints imposed by dominant paradigms. Importantly, big data analyses could uncover patterns and relationships in the data leading to discoveries of behaviors previously unknown or perhaps even contemplated, a situation likely where many of the animal subjects that we study have sensory systems so divergent from our own and are thus likely to perceive the environment in a manner very different to our experience (e.g., echolocating animals).
Roadmap
We have identified a number of approaches from studies of human mobility that could increase the progress of research on animal movement. In order to attain the same rates of advance achieved by studies of human mobility, it is imperative that data that already exists is gathered in central repositories and made freely available to researchers. Data sharing can be achieved through collaborations of researchers across locations and subject areas, but also by funders (governments, NGOs etc.) and scientific journals insisting that the data supporting publications are uploaded to public-access repositories accompanied by appropriate meta-data. There has been a ground-swell of support for this initiative across the research community interested in animal movement. This will also attract the communities that have catalyzed the development of human mobility studies, notably computer and data scientists and scientists working within the complex-system paradigm, to help propel the field of animal movement forward.
Beyond the insights into patterns of movement of animals that might be gained from a transfer of analytical techniques between the research communities involved with human mobility and animal movement, an integration of human and animal movement will help deliver more effective conservation and management options, as these are critically dependent on interactions between humans and wildlife. This is necessary because patterns of human mobility have a direct relationship to many of the anthropogenic threats faced by animal populations worldwide (Meekan et al., 2017). For example, road kills (Clevenger et al., 2003) and ship-strike (Elvin and Taggart, 2008; Silber et al., 2015) pose major risks to the conservation of many animals throughout the world, and the infrastructure deployed to support human mobility, including train and road lines and cities and harbors, also fragment animal habitats (van Bohemen, 1998). Indeed, to identify possible human-animal interactions and to better inform conservation strategies, data on human presence in the environment also needs to be integrated with animal tracking data. Just as data on human mobility were a fundamental underpinning of developments such as “smart-cities” where electronic developments were used to improve the decision-making process by engaging citizens with democratic activities (Paskaleva, 2009), data on animal movement can potentially aid the construction of “smart-environments” where a better understanding of movement patterns of both humans and wildlife would assist conservation and management planning both interactively and in the long-term (Meekan et al., 2017).
The opportunity to accelerate progress in animal movement ecology based on approaches used in human mobility studies will require the development of supporting infrastructure and communities. Once quality-controlled data are available through the use of open repositories, analysis code developed on open platforms such as R (R Core Team, 2017) and web-based visualization and analytical resources can be written for the estimation of new metrics that describe movement patterns. These developments will catalyze progress by enabling the search for common or analogous mechanisms that describe the ways in which animals move and use their environments, as well as identifying impacts and disruptions to patterns of animal movement by both natural and anthropogenic threats and teleconnections in animal movement at global scales. Most importantly, because animals perceive their environments through sensory systems that are often very different from those of humans, the analysis of big data offers the opportunity to search for and identify patterns within animal movement data sets for which we are effectively “blind,” yet may be critical in organizing the ecology and behavior of these species.
Research on human mobility initially borrowed analytical techniques developed for the study of animal mobility (Viswanathan et al., 1996). This process has now begun to move in a full circle, through the application of new techniques developed for or applied to the analysis and visualization of large data sets of human mobility to animal models. In many ways, the data now available from the latest generations of animal tags are very similar to that recorded by smart devices now being worn by humans. New smartwatches include an accelerometer, thermometer, heart rate monitor, altimeter, barometer, compass, chronograph, cell phone and GPS navigation, an almost identical range of sensors to those deployed on the “daily diary” tags used to reveal animal behaviors such as foraging, resting and migrating. The significance of being able to passively obtain data on the habits of millions of users from these new technologies has already been recognized (Kwapisz et al., 2011). Perhaps the major difference between tags that track animals and the smart devices that now track humans is the fact that animals must be caught and restrained to have a tag fitted, whereas humans wear such devices voluntarily and typically pay for the privilege to do so. The convergence between these technologies emphasizes the possibilities for cross-fertilization and collaboration between the research fields of animal movement and human mobility. Given the concerns about the conservation future of large wild animals, the cross-fertilization and collaboration advocated here are not only necessary to catalyze scientific advances, but ultimately an imperative for effective conservation and survival of many animals, since the principal threats to their existence now are largely anthropogenic.
Author Contributions
CD conceived of the idea. MT, AMMS, CD, and VE obtained the seed funding to bring the group together to draft the manuscript. MT led the writing with contributions from all authors.
Funding
AMMS was supported by an ARC Grant DE170100841 and an IOMRC (UWA, AIMS, CSIRO) fellowship. JF-G and VE were supported by Agencia Estatal de Investigación (AEI, Spain) and Fondo Europeo de Desarrollo Regional (FEDER) through project SPASIMM (FIS2016-80067-P AEI/FEDER, UE), and by research funding from KAUST.
Conflict of Interest Statement
The authors declare that the research was conducted in the absence of any commercial or financial relationships that could be construed as a potential conflict of interest.
The handling Editor declared a past co-authorship with some of the authors.
References
Altizer, S., Bartel, R., and Han, B. A. (2011). Animal migration and infectious disease risk. Science 331, 296–302. doi: 10.1126/science.1194694
Andrienko, N., Andrienko, G., Fuchs, G., and Jankowski, P. (2015). Scalable and privacy-respectful interactive discovery of place semantics from human mobility traces. Inform. Visual. 15, 117–153. doi: 10.1177/1473871615581216
Ashbrook, D., and Starner, T. (2003). Using GPS to learn significant locations and predict movement across multiple users. Pers. Ubiquit. Comput. 7, 275–286. doi: 10.1007/s00779-003-0240-0
Bairlein, F. (2001). Results of bird ringing in the study of migration routes. Ardea 89, 7–19. Available online at: http://ardea.nou.nu/ardea_show_abstract.php?lang=uk&nr=108
Balcan, D., Colizza, V., Gonçalves, B., Hu, H., Ramasco, J. J., and Vespignani, A. (2009). Multiscale mobility networks and the spatial spreading of infectious diseases. Proc. Natl. Acad. Sci. U.S.A. 106, 21484–21489. doi: 10.1073/pnas.0906910106
Benhamou, S. (2011). Dynamic approach to space and habitat use based on biased random bridges. PLoS ONE 6:e14592. doi: 10.1371/journal.pone.0014592
Biuw, M., Boehme, L., Guinet, C., Hindell, M. A., Costa, D., Charrassin, J.-B., et al. (2007). Variations in behaviour and condition of a Southern Ocean top predator in relation to in-situ oceanographic conditions. Proc. Natl. Acad. Sci. U.S.A. 104, 13705–13710. doi: 10.1073/pnas.0701121104
Block, B. A., Jonsen, I. D., Jorgensen, S. J., Winship, A. J., Shaffer, S. A., Bograd, S. J., et al. (2011). Tracking apex marine predator movements in a dynamic ocean. Nature 475, 86–90. doi: 10.1038/nature10082
Blondel, V. D., Decuyper, A., and Krings, G. (2015). A survey of results on mobile phone datasets analysis. EPJ Data Science. 4:10. doi: 10.1140/epjds/s13688-015-0046-0
Boyd, I. L., Kato, A., and Ropert-Coudert, Y. (2004). Bio-logging Science: Sensing beyond the Boundaries. Memoirs of National Institute of Polar Research Special Issue. 58, 1–14.
Brockmann, D., Hufnagel, L., and Geisel, T. (2006). The scaling laws of human travel. Nature 439, 462–465. doi: 10.1038/nature04292
Brown, D., Kays, R., Wikelski, M., Wilson, R., and Klimley, A. (2013). Observing the unwatchable through acceleration logging of animal behavior. Anim. Biotelemetry 1:20. doi: 10.1186/2050-3385-1-20
Cagnacci, F., Boitani, L., Powell, R. A., and Boyce, M. S. (2010). Animal ecology meets GPS-based radiotelemetry: a perfect storm of opportunities and challenges. Philos. Trans. R. Soc. B Biol. Sci. 365, 2157–2162. doi: 10.1098/rstb.2010.0107
Campbell, H. A., Beyer, H. L., Dennis, T. E., Dwyer, R. G., Forester, J. D., Fukuda, Y., et al. (2007). Finding our way: on the sharing and reuse of animal telemetry data in Australasia. Sci. Tot. Environ. 534, 79–84. doi: 10.1016/j.scitotenv.2015.01.089
Campbell, H. A., Urbano, F., Davidson, S., Dettki, H., and Cagnacci, F. (2016). A plea for standards in reporting data collected by animal-borne electronic devices. Anim. Biotelemetry 4:1. doi: 10.1186/s40317-015-0096-x
Casale, P., Pujol, O., and Radeva, P. (2011). “Human activity recognition from accelerometer data using a wearable device,” in Pattern Recognition and Impage Analysis, eds J. Vitrià, J. Sanches, and M. Hernández (Berlin; Heidelberg: Springer), 289–296. doi: 10.1007/978-3-642-21257-4_36
Cavagna, A., Giardina, I., Grigera, T. S., Jelic, A., Levine, D., Ramaswamy, S., et al. (2015). Silent flocks: constraints on signal propagation across biological groups. Phys. Rev. Lett. 114:218101. doi: 10.1103/PhysRevLett.114.218101
Cisco (2015). Cisco Visual Networking Index: Global Mobile Data Traffic Forecast Update 2014–2019 White Paper.
Clevenger, A., Chruszcz, B., and Gunson, K. E. (2003). Spatial patterns and factors influencing small vertebrate fauna road-kill aggregations. Biol. Conserv. 109, 15–26. doi: 10.1016/S0006-3207(02)00127-1
Colizza, V., Barrat, A., Barthélemy, M., and Vespignani, A. (2006). The modeling of global epidemics: stochastic dynamics and predictability. Bull. Math. Biol. 68, 1893–1921. doi: 10.1007/s11538-006-9077-9
Cooke, S. J., Iverson, S. J., Stokesbury, M. J. W., Hinch, S. G., Fisk, A. T., VanderZwaag, D. L., et al. (2011). Ocean Tracking Network Canada: a network approach to addressing critical issues in fisheries and resource management with implications for ocean governance. Fisheries 36, 583–592. doi: 10.1080/03632415.2011.633464
Costa, D. P., Klinck, J., Hofman, E., Burns, J. M., Fedak, M. A., and Crocker, D. E. (2003). Marine mammals as ocean sensors. Integr. Comp. Biol. 43:920.
Dallimer, M., and Strange, N. (2015). Why socio-political borders and boundaries matter in conservation. Trends Ecol. Evol. 30, 132–139. doi: 10.1016/j.tree.2014.12.004
de Arriba-Pérez, F., Caeiro-Rodríguez, M., and Santos-Gago, J. M. (2016). Collection and processing of data from wrist wearable devices in heterogeneous and multiple-user scenarios. Sensors 16:1538. doi: 10.3390/s16091538
de Lira, V., Rinzivillo, S., Times, V., Renso, C., and Tedesco, P. (2014). “MAPMOLTY: a web tool for discovering place loyalty based on mobile crowdsource data,” in Web Engineering. Lecture Notes in Computer Science, eds S. Casteleyn, G. Rossi, and M. Winckler (Cham: Springer International Publishing), 528–531. doi: 10.1007/978-3-319-08245-5_43
Do, T. M. T., Dousse, O., Miettinen, M., and Gatica-Perez, D. (2015). A probabilistic kernel method for human mobility prediction with smartphones. Pervasive Mob. Comput. 20, 13–28. doi: 10.1016/j.pmcj.2014.09.001
Dujon, A. M., Lindstrom, R. T., and Hays, G. C. (2014). The accuracy of Fastloc-GPS locations and implications for animal tracking. Methods Ecol. Evol. 5, 1162–1169. doi: 10.1111/2041-210X.12286
Dwyer, R. G., Campbell, H. A., Brooking, C., Brimblecombe, W., Hunter, J., Watts, M. E., et al. (2015). An open web-based system for the analysis and sharing and analysis of animal tracking data. Anim. Biotelemetry 3:1. doi: 10.1186/s40317-014-0021-8
Eagle, N., and Pentland, A. (2006). Reality mining: sensing complex social systems. Pers. Ubiquitous Comput. 10, 255–268. doi: 10.1007/s00779-005-0046-3
Edwards, A. M. (2011). Overturning conclusions of Lévy flight movement patterns by fishing boats and foraging animals. Ecology 92, 1247–1257. doi: 10.1890/10-1182.1
Elvin, S. S., and Taggart, C. T. (2008). Right whales and vessels in Canadian waters. Mar. Policy 32, 379–386. doi: 10.1016/j.marpol.2007.08.001
Fedak, M. (2004). Marine animals as platforms for oceanographic sampling: a “win/win” situation for biology and operational oceanography. Mem. Natl. Inst. Polar Res., Spec. Issue 58, 133–147.
Fortin, D., Beyer, H. L., Boyce, M. S., Smith, D. W., Duchesne, T., and Mao, J. S. (2005). Wolves influence elk movements: behavior shapes a trophic cascade in yellowstone national park. Ecology 86, 1320–1330. doi: 10.1890/04-0953
Gautestad, A. O., and Mysterud, A. (2013). The Lévy flight foraging hypothesis: forgetting about memory may lead to false verification of Brownian motion. Mov. Ecol. 1:9. doi: 10.1186/2051-3933-1-9
Getz, W. M., and Saltz, D. (2008). A framework for generating and analyzing movement paths on ecological landscapes. Proc. Natl. Acad. Sci. 105, 19066–19071. doi: 10.1073/pnas.0801732105
Giannotti, F., Nanni, M., Pedreschi, D., Pinelli, F., Renso, C., Rinzivillo, S., et al. (2011). Unveiling the complexity of human mobility by querying and mining massive trajectory data. VLDB J. 20, 695–719. doi: 10.1007/s00778-011-0244-8
Gonzalez, M. C., Hidalgo, C. A., and Barabasi, A.-L. (2008). Understanding individual human mobility patterns. Nature 453, 779–782. doi: 10.1038/nature06958
Goto, Y., Yoda, K., and Sato, K. (2017). Asymmetry hidden in birds' tracks reveals wind, heading, and orientation ability over the ocean. Sci. Adv. 3:e1700097. doi: 10.1126/sciadv.1700097
Hampton, S. E., Strasser, C. A., Tewksbury, J. J., Gram, W. K., Budden, A. E., Batcheller, A. L., et al. (2013). Big data and the future of ecology. Front. Ecol. Environ. 11, 156–162. doi: 10.1890/120103
Hays, G. C. (2014). Charismatic marine mega-fauna: some recent discoveries and future challenges. J. Exp. Mar. Biol. Ecol. 450, 1–5. doi: 10.1016/j.jembe.2013.10.015
Hays, G. C., Bradshaw, C. J. A., James, M. C., Lovell, P., and Sims, D. W. (2007). Why do Argos satellite tags deployed on marine animals stop transmitting? J. Exp. Mar. Biol. Ecol. 349, 52–60. doi: 10.1016/j.jembe.2007.04.016
Hays, G. C., Ferreira, L. C., Sequeira, A. M. M., Meekan, M. G., Duarte, C. M., Bailey, H., et al. (2016). Key questions in marine megafauna movement ecology. Trends Ecol. Evol. 31, 463–475. doi: 10.1016/j.tree.2016.02.015
Helbing, D., and Molnár, P. (1995). Social force model for pedestrian dynamics. Phys. Rev. E 51, 4282–4286. doi: 10.1103/PhysRevE.51.4282
Hemson, G., Johnson, P., South, A., Kenward, R., Ripley, R., and MacDonald, D. (2005). Are kernels the mustard? Data from global positioning system (GPS) collars suggests problems for kernel home-range analyses with least-squares cross-validation. J. Anim. Ecol. 74, 455–463. doi: 10.1111/j.1365-2656.2005.00944.x
Hoenner, X., Huveneers, C., Steckenreuter, A., Simpfendorfer, C., Tattersall, K., Jaine, F., et al. (2018). Australia's continental-scale acoustic tracking database and its automated quality control process. Sci. Data 5:170206. doi: 10.1038/sdata.2017.206
Holland, K. N., Meyer, C. G., and Dagorn, L. C. (2009). Inter-animal telemetry: results from first deployment of acoustic ‘business card’ tags. Endanger. Species Res. 10, 287–293. doi: 10.3354/esr00226
Hooten, M. B., Buderman, F. E., Brost, B. M., Hanks, E. M., and Ivan, J. S. (2016). Hierarchical animal movement models for population-level inference. Environmetrics 27, 322–333. doi: 10.1002/env.2402
Hooten, M. B., Johnson, D. S., McClintock, B. T., and Morales, J. M. (2017). Animal Movement; Statistical Models for Telemetry Data. Boca Raton, FL: CRC Press.
Horne, J. S., Garton, E. O., Krone, S. M., and Lewis, J. S. (2007). Analyzing animal movements using Brownian bridges. Ecology 88, 2354–2363. doi: 10.1890/06-0957.1
Hussey, N. E., Kessel, S. T., Aarestrup, K., Cooke, S. J., Cowley, P. D., Fisk, A. T., et al. (2015). Aquatic animal telemetry: a panoramic window into the underwater world. Science 348:1255642. doi: 10.1126/science.1255642
Jonsen, I. (2016). Joint estimation over multiple individuals improves behavioural state inference from animal movement data. Sci. Rep. 6:20625. doi: 10.1038/srep20625
Jonsen, I. D., Basson, M., Bestley, S., Bravington, M. V., Patterson, T. A., Pedersen, M. W., et al. (2013). State-space models for bio-loggers: a methodological road map. Deep Sea Res. II 88–89, 34–46. doi: 10.1016/j.dsr2.2012.07.008
Jordan, M. I., and Mitchell, T. M. (2015). Machine learning: trends, perspectives, and prospects. Science 349, 255–260. doi: 10.1126/science.aaa8415
Kays, R., Crofoot, M. C., Jetz, W., and Wikelski, M. (2015). Terrestrial animal tracking as an eye on life and planet. Science 348:aaa2478. doi: 10.1126/science.aaa2478
Kloosterman, R. C., and Musterd, S. (2001). The polycentric urban region: towards a research agenda. Urban Stud. 38, 623–633. doi: 10.1080/00420980120035259
Kooyman, G. L. (1965). Techniques used in measuring diving capacities of Weddell Seals. Polar Rec. 12, 391–394. doi: 10.1017/S003224740005484X
Krause, J., Krause, S., Arlinghaus, R., Psorakis, I., Roberts, S., and Rutz, C. (2013). Reality mining of animal social systems. Trends Ecol. Evol. 28, 541–551. doi: 10.1016/j.tree.2013.06.002
Kwapisz, J. R., Weiss, G. M., and Moore, S. A. (2011). Activity recognition using cell phone accelerometers. SIGKDD Explor. Newsl. 12, 74–82. doi: 10.1145/1964897.1964918
LeMunyan, C. D., White, W., Nyberg, E., and Christian, J. J. (1959). Design of a miniature radio transmitter for use in animal studies. J. Wildl. Manage. 23, 107–110. doi: 10.2307/3797755
Leroy, E. M., Epelboin, A., Mondonge, V., Pourrut, X., Gonzalez, J.-P., Muyembe-Tamfum, J.-J., et al. (2009). Human Ebola outbreak resulting from direct exposure to fruit bats in Luebo, Democratic Republic of Congo, 2007. Vector-Borne Zoon. Dis. 9, 723–728. doi: 10.1089/vbz.2008.0167
Lidgard, D. C., Bowen, W. D., Jonsen, I. D., and Iverson, S. J. (2014). Predator-borne acoustic transceivers and GPS tracking reveal spatiotemporal patterns of encounters with acoustically tagged fish in the open ocean. Mar. Ecol. Prog. Ser. 501, 157–168. doi: 10.3354/meps10670
Liu, J., Zhong, L., Wickramasuriya, J., and Vasudevan, V. (2009). uWave: accelerometer-based personalized gesture recognition and its applications. Pervasive Mob. Comput. 5, 657–675. doi: 10.1016/j.pmcj.2009.07.007
Liu, Z., Jiang, B., and Heer, J. (2013). imMens: real-time visual querying of big data. Computer Graph. Forum 32, 421–430. doi: 10.1111/cgf.12129
Louail, T., Lenormand, M., Picornell, M., García Cant,ú, O., Herranz, R., Frias-Martinez, E., et al. (2015). Uncovering the spatial structure of mobility networks. Nat. Commun. 6:6007. doi: 10.1038/ncomms7007
Manly, B. F. L., McDonald, L., Thomas, D. L., McDonald, T. L., and Erickson, W. (2007). Resource Selection by Animals: Statistical Design and Analysis for Field Studies. Dordrecht: Springer science and business media.
Mantyjarvi, J., Lindholm, M., Vildjiounaite, E., Makela, S. M., and Ailisto, H. A. (eds.). (2005). “Identifying users of portable devices from gait pattern with accelerometers,” in Proceedings (ICASSP ‘05) IEEE International Conference on Acoustics, Speech, and Signal Processing (Philadelphia, PA). doi: 10.1109/ICASSP.2005.1415569
Meekan, M. G., Duarte, C. M., Fernández-Gracia, J., Thums, M., Sequeira, A. M. M., Harcourt, R., et al. (2017). The ecology of human mobility. Trends Ecol. Evol. 32, 198–210. doi: 10.1016/j.tree.2016.12.006
Mitchell, L., Frank, M. R., Harris, K. D., Dodds, P. S., and Danforth, C. M. (2013). The geography of happiness: connecting twitter sentiment and expression, demographics, and objective characteristics of place. PLoS ONE 8:e64417. doi: 10.1371/journal.pone.0064417
Mocanu, D., Baronchelli, A., Perra, N., Gonçalves, B., Zhang, Q., and Vespignani, A. (2013). The twitter of babel: mapping world languages through microblogging platforms. PLoS ONE 8:e61981. doi: 10.1371/journal.pone.0061981
Mount, D. W. (2004). Bioinformatics: Sequence and Genome Analysis. Cold Spring Harbour, NY: Cold Spring Harbour lab Press.
Nathan, R. (2008). An emerging movement ecology paradigm. Proc. Natl. Acad. Sci. U.S.A. 105, 19050–19051. doi: 10.1073/pnas.0808918105
Nathan, R., Spiegel, O., Fortmann-Roe, S., Harel, R., Wikelski, M., and Getz, W. M. (2012). Using tri-axial acceleration data to identify behavioral modes of free-ranging animals: general concepts and tools illustrated for griffon vultures. J. Exp. Biol. 215, 986–996. doi: 10.1242/jeb.058602
Nicolai, T., Yoneki, E., Behrens, N., and Kenn, H. (2006). “Exploring social context with the wireless rope,” in On the Move to Meaningful Internet Systems 2006: OTM 2006 Workshops: OTM Confederated International Workshops and Posters, AWeSOMe, CAMS, COMINF, IS, KSinBIT, MIOS-CIAO, MONET, OnToContent, ORM, PerSys, OTM Academy Doctoral Consortium, RDDS, SWWS, and SeBGIS 2006, Montpellier, eds R. Meersman, Z. Tari, and P. Herrero (Berlin; Heidelberg: Springer), 874–883.
Nielsen, L. R., Pedersen, A. R., Herskin, M. S., and Munksgaard, L. (2010). Quantifying walking and standing behaviour of dairy cows using a moving average based on output from an accelerometer. Appl. Anim. Behav. Sci. 127, 12–19. doi: 10.1016/j.applanim.2010.08.004
Paskaleva, K. A. (2009). Enabling the smart city: the progress of city e-governance in Europe. Int. J. Innov. Reg. Dev. 1, 405–422. doi: 10.1504/IJIRD.2009.02273
Patterson, T. A., Thomas, L., Wilcox, C., Ovaskainen, O., and Matthiopoulos, J. (2008). State-space models of individual animal movement. Trends Ecol. Evol. 23, 87–94. doi: 10.1016/j.tree.2007.10.009
Petrovskii, S., Mashanova, A., and Jansen, V. A. (2011). Variation in individual walking behavior creates the impression of a Lévy flight. Proc. Natl. Acad. Sci. U.S.A. 108, 8704–8707. doi: 10.1073/pnas.1015208108
Pyke, G. H. (2015). Understanding movements of organisms: it's time to abandon the Lévy foraging hypothesis. Methods Ecol. Evol. 6, 1–16. doi: 10.1111/2041-210X.12298
R Core Team (2017). R: A Language and Environment for Statistical Computing. Vienna: R Foundation for Statistical Computing.
Raffaelli, D., Bullock, J., Cinderby, S., Durance, I., Emmett, B., Harris, J., et al. (2014). Big data and ecosystem research programmes. Adv. Ecol. Res. 51, 41–77. doi: 10.1016/B978-0-08-099970-8.00004-X
Ramos-Fernández, G., Mateos, J. L., Miramontes, O., Cocho, G., Larralde, H., and Ayala-Orozco, B. (2004). Lévy walk patterns in the foraging movements of spider monkeys (Ateles geoffroyi). Behav. Ecol. Sociobiol. 55, 223–230. doi: 10.1007/s00265-003-0700-6
Raymond, B., Lea, M.-A., Patterson, T., Andrews-Goff, V., Sharples, R., Charrassin, J.-B., et al. (2015). Important marine habitat off east Antarctica revealed by two decades of multi-species predator tracking. Ecography 38, 121–129. doi: 10.1111/ecog.01021
Reynolds, A. (2008). How many animals really do the lévy walk? Comment. Ecology 89, 2347–2351. doi: 10.1890/07-1688.1
Rodríguez, J. P., Fernández-Gracia, J., Thums, M., Hindell, M. A., Sequeira, A. M., Meekan, M. G., et al. (2017). Big data analyses reveal patterns and drivers of the movements of southern elephant seals. Sci. Rep. 7:112. doi: 10.1038/s41598-017-00165-0
Roth, C., Kang, S. M., Batty, M., and Barthelemy, M. (2011). Structure of urban movements: polycentric activity and entangled hierarchical flows. PLoS ONE 6:e15923. doi: 10.1371/journal.pone.0015923
Russell, C. A., Smith, D. L., Childs, J. E., and Real, L. A. (2005). Predictive spatial dynamics and strategic planning for raccoon rabies emergence in Ohio. PLoS Biol. 3:e88. doi: 10.1371/journal.pbio.0030088
Rutz, C., and Hays, G. C. (2009). New frontiers in biologging science. Biol. Lett. 5, 289–292. doi: 10.1098/rsbl.2009.0089
Rutz, C., Burns, Z. T., James, R., Ismar, S. M., Burt, J., Otis, B., et al. (2012). Automated mapping of social networks in wild birds. Curr. Biol. 22, R669–R671. doi: 10.1016/j.cub.2012.06.037
Sakamoto, K. Q., Sato, K., Ishizuka, M., Watanuki, Y., Takahashi, A., Daunt, F., et al. (2009). Can ethograms be automatically generated using body acceleration data from free-ranging birds? PLoS ONE. 4:e5379. doi: 10.1371/journal.pone.0005379
Salnikov, V., Schaub, M. T., and Lambiotte, R. (2016). Using higher-order Markov models to reveal flow-based communities in networks. Sci. Rep. 6:23194. doi: 10.1038/srep23194
Schick, R. S., Loarie, S. R., Colchero, F., Best, B. D., Boustany, A., Conde, D. A., et al. (2008). Understanding movement data and movement processes: current and emerging directions. Ecol. Lett. 11, 1338–1350. doi: 10.1111/j.1461-0248.2008.01249.x
Schreer, J. F., and Testa, J. W. (1995). Statistical classification of diving behavior. Mar. Mamm. Sci. 11, 85–93. doi: 10.1111/j.1748-7692.1995.tb00277.x
Schreer, J. F., Kovacs, K. M., and Hines, R. J. O. (2001). Comparative diving patterns of pinnipeds and seabirds. Ecol. Monogr. 71, 137–162. doi: 10.1890/0012-9615(2001)071[0137:CDPOPA]2.0.CO;2
Schweinsburg, R. E., and Lee, L. J. (1982). Movement of four satellite-monitored polar bears in Lancaster Sound, Northwest Territories. Arctic 35, 504–511. doi: 10.14430/arctic2357
Sequeira, A., Rodríguez, J., Eguíluz, V., Harcourt, R., Hindell, M., Sims, D., et al. (in press). Convergence of marine megafauna movement patterns in coastal open oceans. Proc. Nat. Acad. Sci. doi: 10.20350/digitalCSIC/8525
Shen, Y., Zhao, L., and Fan, J. (2015). Analysis and visualization for hot spot based route recommendation using short-dated Taxi GPS traces. Information 6:134. doi: 10.3390/info6020134
Silber, G. K., Adams, J. D., Asaro, M. J., Cole, T. V., Moore, K. S., Ward-Geiger, L. I., et al. (2015). The right whale mandatory ship reporting system: a retrospective. PeerJ. 3:e866. doi: 10.7717/peerj.866
Simini, F., Gonzalez, M. C., Maritan, A., and Barabasi, A.-L. (2012). A universal model for mobility and migration patterns. Nature 484, 96–100. doi: 10.1038/nature10856
Simini, F., Maritan, A., and Néda, Z. (2013). Human mobility in a continuum approach. PLoS ONE 8:e60069. doi: 10.1371/journal.pone.0060069
Sims, D. W., Southall, E. J., Humphries, N. E., Hays, G. C., Bradshaw, C. J. A., Pitchford, J. W., et al. (2008). Scaling laws of marine predator search behaviour. Nature 451, 1098–1102. doi: 10.1038/nature06518
Smalheiser, N. R. (2002). Informatics and hypothesis-driven research. EMBO Rep. 3:702. doi: 10.1093/embo-reports/kvf164
Sobolevsky, S., Szell, M., Campari, R., Couronne, T., Smoreda, Z., and Ratti, C. (2013). Delineating geographical regions with networks of human interactions in an extensive set of countries. PLoS ONE 8:e81707. doi: 10.1371/journal.pone.0081707
Stouffer, S. A. (1940). A theory relating to mobility and distance. Am. Sociol. Rev. 5, 845–867. doi: 10.2307/2084520
Thiemann, C., Theis, F., Grady, D., Brune, R., and Brockmann, D. (2010). The structure of borders in a small world. PLoS ONE 5:e15422. doi: 10.1371/journal.pone.0015422
Thums, M., Bradshaw, C. J., and Hindell, M. A. (2008). A validated approach for supervised dive classification in diving vertebrates. J. Exp. Mar. Biol. Ecol. 363, 75–83. doi: 10.1016/j.jembe.2008.06.024
Tizzoni, M., Bajardi, P., Poletto, C., Ramasco, J., Balcan, D., Goncalves, B., et al. (2012). Real-time numerical forecast of global epidemic spreading: case study of 2009 A/H1N1pdm. BMC Med. 10:165. doi: 10.1186/1741-7015-10-165
Toner, J., and Tu, Y. (1998). Flocks, herds, and schools: a quantitative theory of flocking. Phys. Rev. E 58, 4828–4858. doi: 10.1103/PhysRevE.58.4828
van Bohemen, H. D. (1998). Habitat fragmentation, infrastructure and ecological engineering. Ecol. Eng. 11, 199–207.
Van den Broeck, W., Gioannini, C., Goncalves, B., Quaggiotto, M., Colizza, V., and Vespignani, A. (2011). The GLEaMviz computational tool, a publicly available software to explore realistic epidemic spreading scenarios at the global scale. BMC Infect. Dis. 11:37. doi: 10.1186/1471-2334-11-37
Vicsek, T., and Zafeiris, A. (2012). Collective motion. Phys. Rep. 517, 71–140. doi: 10.1016/j.physrep.2012.03.004
Vicsek, T., Czirók, A., Ben-Jacob, E., Cohen, I., and Shochet, O. (1995). Novel type of phase transition in a system of self-driven particles. Phys. Rev. Lett. 75, 1226–1229. doi: 10.1103/PhysRevLett.75.1226
Viswanathan, G. M., Afanasyev, V., Buldyrev, S. V., Murphy, E. J., Prince, P. A., and Stanley, H. E. (1996). Levy flight search patterns of wandering albatrosses. Nature 381, 413–415. doi: 10.1038/381413a0
Watanabe, S., Izawa, M., Kato, A., Ropert-Coudert, Y., and Naito, Y. (2005). A new technique for monitoring the detailed behaviour of terrestrial animals: a case study with the domestic cat. Appl. Anim. Behav. Sci. 94, 117–131. doi: 10.1016/j.applanim.2005.01.010
Wikelski, M., and Kays, R. (2010). Movebank: Archive, Analysis and Sharing of Animal Movement Data. World Wide Web electronic publication. Avilable online at: https://www.movebank.org/
Wilson, R. P., Shepard, E. L. C., and Liebsch, N. (2008). Prying into the intimate details of animal lives: use of a daily diary on animals. Endanger. Species Res. 4, 123–137. doi: 10.3354/esr00064
Worton, B. J. (1989). Kernel methods for estimating the utilization distribution in home-range studies. Ecology 70, 164–168. doi: 10.2307/1938423
Keywords: big data, telemetry, smartphone, wearables, biologging, data synthesis
Citation: Thums M, Fernández-Gracia J, Sequeira AMM, Eguíluz VM, Duarte CM and Meekan MG (2018) How Big Data Fast Tracked Human Mobility Research and the Lessons for Animal Movement Ecology. Front. Mar. Sci. 5:21. doi: 10.3389/fmars.2018.00021
Received: 08 September 2017; Accepted: 17 January 2018;
Published: 13 February 2018.
Edited by:
Alastair Martin Mitri Baylis, South Atlantic Environmental Research Institute, Falkland IslandsReviewed by:
Theoni Photopoulou, Nelson Mandela Metropolitan University, South AfricaHamish Campbell, Charles Darwin University, Australia
Copyright © 2018 Thums, Fernández-Gracia, Sequeira, Eguíluz, Duarte and Meekan. This is an open-access article distributed under the terms of the Creative Commons Attribution License (CC BY). The use, distribution or reproduction in other forums is permitted, provided the original author(s) and the copyright owner are credited and that the original publication in this journal is cited, in accordance with accepted academic practice. No use, distribution or reproduction is permitted which does not comply with these terms.
*Correspondence: Michele Thums, m.thums@aims.gov.au