- 1National Research Institute of Fisheries Science, Japan Fisheries Research and Education Agency, Yokohama, Japan
- 2Atmosphere and Ocean Research Institute, University of Tokyo, Kashiwa, Japan
- 3City of Mombetsu, Mombetsu, Japan
- 4Graduate School of Fisheries Sciences, Hokkaido University, Hakodate, Japan
- 5Hokkaido National Fisheries Research Institute, Japan Fisheries Research and Education Agency, Kushiro, Japan
Monitoring zooplankton communities is important to understand dynamics in marine ecosystems. However, it is difficult to identify cryptic species and immature stages of zooplankton using morphological classification, which is time-consuming and requires high skill levels. Here, we conducted a metagenetic analysis of the 18S region in 101 zooplankton samples collected weekly throughout 2014 and 2015 at the Okhotsk Tower in Mombetsu, Hokkaido, Japan, and compared the results of this analysis with those provided by morphological analysis. The metagenetic analysis detected 561 molecular taxonomic units (MOTUs), whereas the morphological analysis detected 201 taxonomic groups. Zooplankton communities were dominated by copepods throughout the sampling period; however, non-copepod taxa, which comprised high proportions of both MOTUs (mean 51.1%) and sequence reads (mean 19.1%), were also important. Cryptic diversity detected by the metagenetic analysis was primarily driven by Copepoda and by the larvae of benthic taxa such as Bivalvia, Gastropoda, and Polychaeta. Community structure and diversity varied between periods of warm and cold water, indicating strong correlations with water temperature and thus seasonality. Furthermore, metagenetic analysis revealed detailed seasonal changes in dominant taxa, including larval stages of metazoans with high taxonomic resolutions; these included commercially important organisms such as Japanese scallops. The metagenetic analysis revealed that changes in both water mass and bentho-pelagic interactions sustain ecosystems rich in zooplankton diversity in this area. Metagenetic analysis provides novel insight into zooplankton diversity, and generates massive sequence data that may be used in future research; thus, it is considered an effective tool for monitoring zooplankton communities.
Introduction
Marine zooplankton comprise an abundant and diverse group including ~7,000 described species in 15 phyla (Bucklin et al., 2010). Zooplankton contribute substantially to production in higher trophic levels, including in various taxa important to the fishing industry, and zooplankton communities respond sensitively to environmental and climate changes (Hays et al., 2005). For example, negative impacts of global warming on zooplankton abundance have already been reported in the California Current (Roemmich and McGowan, 1995), and changes in zooplankton community structure are predicted as water temperatures increase in the future (Richardson, 2008). Changes in the distribution of ecologically important copepod species have been observed in large-scale spatial and temporal surveys of zooplankton, which in turn affect the recruitment of Atlantic cod in the North Atlantic (Beaugrand et al., 2003). Such changes in distribution are difficult to predict, as zooplankton distribution patterns are species-specific, and different species have different environmental preferences. To mitigate this difficulty, and to track changes in marine ecosystems in response to rapid climate and environmental changes, techniques must be developed that facilitate the monitoring of zooplankton communities at high taxonomic resolutions.
Zooplankton classification is traditionally carried out using morphological observations of individual specimens, a process that is time-consuming and requires great expertise. Furthermore, such methods are imprecise in identifying immature stages and cryptic species, as revealed by the frequency with which undescribed and cryptic species are observed using molecular techniques (Bucklin et al., 2010). Among molecular-based methods, the metagenetic approach, a promising molecular technique using high-throughput sequencing, is particularly useful in revealing community structure based on massive sequence data recovered from bulk environmental samples. Although metagenetic techniques are still being developed in zooplankton, comparisons with morphological techniques already suggest that metagenetic techniques can detect cryptic diversity in zooplankton communities with high taxonomic coverage and at high resolution (Lindeque et al., 2013; Hirai et al., 2015a; Mohrbeck et al., 2015; Harvey et al., 2017). To date, metagenetic techniques have been used to examine zooplankton communities over large spatial scales, and have accurately detected shifts in community compositions corresponding with changes in environmental conditions (Pearman et al., 2014; de Vargas et al., 2015; Hirai and Tsuda, 2015; Chain et al., 2016). Metagenetic techniques have also been applied to revealing zooplankton community structure and diversity at different sampling depths (Pearman and Irigoien, 2015; Sommer et al., 2017) and in different seasons (Casas et al., 2017), as well as to dietary analysis of zooplanktivorous fish (Albaina et al., 2016). As the utility of metagenetic techniques has been demonstrated for various marine organisms (Aylagas et al., 2016; Danovaro et al., 2016), and as the method is not dependent on subtle morphological characters or the skill of taxonomists, it is expected to become a widespread tool in the rapid and effective monitoring of zooplankton communities and diversity (reviewed in Bucklin et al., 2016).
The northeastern coast of Hokkaido, Japan, provides nursery grounds for juvenile pink and chum salmon, and opens to the Okhotsk Sea (Asami et al., 2007; Nagata et al., 2007), an area undergoing rapid environmental change as sea ice melts, possibly as a result of air temperatures which have risen over the past 50 years at a rate higher than that of the global average (Ohshima, 2008; Radchenko et al., 2010). An environmental monitoring program has been carried out since the 1990s at the Okhotsk Tower, Mombetsu, where zooplankton communities have been investigated using morphological classifications (brief monthly reports of zooplankton communities are available with images of dominant taxa at http://mombetsu.jp/soshiki/kankou/2013-0902-1336-155.html). Water in the region is dominated by the Soya Warm Current during the summer and is replaced by surface water from the Okhotsk Sea during the winter (Aota, 1975, 1985). Drift ice covers the sea surface during the winter, and a peak in chlorophyll a concentration is observed in the spring after the sea ice retreats (Hamasaki et al., 1998; Taguchi et al., 2000). These seasonal environmental changes correspond with shifts of dominant zooplankton taxa (Hamaoka et al., 2010). Given such high seasonality, the northeastern coastal waters off Mombetsu provide ideal conditions for investigating the responses of zooplankton communities to environmental changes. Recently, the metagenetic method was used to identify eukaryotic plankton communities in water samples taken from the region (Nagai et al., 2015) and to identify metazoan zooplankton communities collected by plankton nets (Hirai et al., 2015b). However, only limited numbers of zooplankton samples were analyzed in these previous studies, and the seasonality of zooplankton communities was not fully evaluated.
In this study, we analyzed zooplankton communities collected at the Okhotsk Tower from 2014 to 2015 using a metagenetic analysis of the 18S V9 region, and compared the results of this analysis with those generated by morphological analysis. Furthermore, we used the metagenetic analysis to detect cryptic zooplankton diversity, to investigate seasonal changes in community structure in relation to environmental changes, and thus to identify key factors influencing zooplankton communities in the region. Finally, we discuss the utility of metagenetic analysis as a tool for monitoring zooplankton communities in the coastal waters of northeastern Hokkaido.
Materials and Methods
Sample Collection
Zooplankton samples (n = 101) were collected at the Okhotsk Tower, 1 km off the coast of Mombetsu in northeastern Hokkaido, Japan (44°20.2′N, 143°22.9′E), weekly throughout 2014 and 2015. Samples were collected during the daytime using Kitahara nets with a mesh size of 100 μm in a vertical tow from the bottom (~10 m deep) to the surface. Following collection, samples were preserved at −20°C for metagenetic analysis. Samples used in morphological classification (n = 95) were collected using the same method and were preserved in 5% buffered formalin. Vertical profiles of water temperature and salinity were measured using a RINKO-Profiler ASTD102 (JFE Advantech Co., Ltd.) on most days during the sampling period. Because zooplankton samples were collected from the bottom to the surface, mean water temperature and salinity throughout the water column were also measured. Mean values were used because they sufficiently represent seasonal changes in water temperature and salinity, which were the focus of our study. On zooplankton sampling days, surface water was collected using a bucket to measure concentrations of chlorophyll a (chl a), nitrate, and phosphate, according to methods described by Kasai et al. (2017).
High-Throughput Sequencing
In addition to the 101 environmental zooplankton samples described above, and to verify the accuracy of the metagenetic analysis, two mock community samples for which the community structure was known in advance were also prepared. Total genomic DNA from bulk zooplankton samples was extracted using a DNeasy Blood & Tissue Kit (Qiagen). The concentration of DNA in each sample was measured using a Qubit 3.0 Fluorometer (Thermo Fisher Scientific). The 18S V9 region was amplified using PCR with the eukaryotic universal primers 1389F and 1510R (Amaral-Zettler et al., 2009). Three PCRs were carried out using a KOD Plus version 2 (Toyobo). For the first PCR, a 15 μl reaction volume was created, containing 7 μl distilled water, 1.5 μl 10 × buffer, 1.5 μl dNTPs (2 mM), 0.9 μl MgSO4 (25 mM), 0.9 μl each primer (5 μM), 0.3 μl KOD Plus polymerase, and 2 μl template DNA (1 ng/μl). The first PCR was carried out according to the methods described by Hirai et al. (2017a), with a modification of cycle numbers from 30 to 25. PCR products from the target region were confirmed by electrophoresis using a 2.0% agarose gel. In cases where no clear amplifications were obtained in the first PCR, DNA samples were purified using PowerClean DNA Clean-Up Kit (MO BIO), and the PCR was carried out again. The second and third PCRs, in which adaptors were attached for sequencing in an Illumina MiSeq and dual-index target sequences were used to discriminate samples, respectively, were carried out according to the methods described by Hirai et al. (2017a). The total reaction volume was adjusted to 15 μl containing 2 μl template DNA (1/20 diluted PCR products). The concentration of the final PCR products was measured using a Qubit 3.0 Fluorometer, and each sample was pooled and purified using a QIAquick PCR Purification Kit (Qiagen). Purified PCR products were transferred to Bioengineering Lab. Co., Ltd., where a single sequencing run was carried out using a MiSeq Reagent Kit v2 on an Illumina MiSeq to obtain 2 × 250 bp paired-end sequence reads.
Bioinformatics and Data Analysis
Sequences were filtered for quality and were classified taxonomically according to the methods described by Hirai et al. (2017a). Bioinformatic procedures were carried out using MOTHUR (Schloss et al., 2009). Sequence reads were classified into broad taxonomic groups based on the V9_PR2 reference database (de Vargas et al., 2015) using a naïve Bayesian classifier (Wang et al., 2007). Because this study focused on metazoan zooplankton, only sequence reads identified as “Metazoa” with a threshold >70% were selected for further analysis. Metazoan sequences in the V9_PR2 reference database were aligned using MAFFT (Katoh and Standley, 2013), and were subsequently used as reference sequences for aligning massive sequence reads from the zooplankton samples collected at Okhotsk Tower. Following alignment, preclustering, and the removal of singleton reads, possible chimeras were removed using UCHIME (Edgar et al., 2011), by using both the reference-based mode, the V9_PR2 reference, and the de novo mode. Same numbers of sequence reads were randomly selected based on the minimum number of sequence reads within a single community sample, and massive sequence reads from these samples attributable to Metazoa were clustered into molecular taxonomic units (MOTUs) at a similarity threshold of 97%. In this study, we used MOTUs with an abundance threshold of ≥8 sequence reads. These similarity and abundance threshold values were determined based on the results of the mock community analysis, in order to avoid overestimating diversity by intra-specific sequence variations in 18S RNA genes and erroneous sequence reads produced during PCR and sequencing. MOTUs were grouped based on the results of a naïve Bayesian analysis at a threshold of >70%. Unclassified MOTUs were further investigated using a BLAST search of the NCBI database, and were assigned to taxonomic groups in cases where ≥95% sequence identity was observed. Additionally, a BLAST search was carried out for the 10 most abundant MOTUs in the major taxonomic groups of Bivalvia, Copepoda, Gastropoda, and Polychaeta. These abundant MOTUs were assigned either to the family (Bivalvia, Copepoda, and Polychaeta) or superfamily (Gastropoda) level, in cases where they were ≥99% similar to taxonomic groups in the public database or to higher taxonomic levels when the similarity threshold was ≥95%. Although there are possible methodological biases, including copy numbers of 18S rRNA genes and primer mismatches during PCR, sequence read abundance in metagenetic analyses can be used as a proxy for biomass in zooplankton (Lindeque et al., 2013; Hirai et al., 2015a). Therefore, we used proportions of sequence reads for quantitative analysis in the metagenetic analysis in this study.
Zooplankton diversity was measured using the total number of MOTUs in conjunction with Shannon and Simpson diversity indexes. Correlations between environmental variables and MOTU numbers were determined using Pearson's product-moment correlation coefficient (r). Bray–Curtis similarity was calculated between community samples for multidimensional scaling (MDS) analysis in PRIMER version 7 (Clarke and Gorley, 2015), based on the abundance of sequence reads and the presence/absence of MOTUs in each sample. In the abundance-based analyses, the proportions of sequence reads attributable to each MOTU were logx+1-transformed. The strongest environmental determinants of zooplankton communities were analyzed in a redundancy analysis (RDA) in CANOCO v.5, using the forward selection method (ter Braak and Verdonschot, 1995). Seasonal changes in taxonomic composition were also investigated, based on the presence/absence of specific MOTUs or sequence reads.
Morphological Analysis
Specimens were classified to the lowest-ranking taxon possible using morphological techniques, and the abundance of morphological groups in each sample was counted. These counts, as well as diversity indexes, MDS plots, and taxonomic compositions, were compared with those generated in the metagenetic analysis. Because this study did not collect biomass data; instead, the abundance of each taxonomic group (individuals/m3) was used in the quantitative analyses used to measure diversity indexes and community similarity. Correlations between diversity measured metagenetically and diversity measured morphologically were compared using Pearson's product-moment correlation coefficient (r). In the abundance-based community analysis, similarities between communities were calculated using logx+1-transformed abundance data from each morphological group.
Results
Seasonal Changes in Environmental Conditions and Zooplankton Abundance
Mean water temperatures ranged from −1.6 to 20.2°C in 2014 and from −1.6 to 20.3°C in 2015 (Figure 1). Water temperature peaked from July to September, and temperatures < 0°C were primarily observed from January to March. Salinity levels were low in the winter and high in the summer, although occasional abrupt decreases in salinity were observed throughout both years. Low salinity levels (≤32.5) were observed primarily during November and May. Salinity levels ≥33.6 were primarily detected from July to October. Chl a concentration ranged from 0.1 to 13.3 mg/m3 during the sampling period, and peaked in spring in both 2014 and 2015; however, high chl a concentrations were occasionally observed in summer and autumn, particularly in 2015. Chl a concentrations remained low during winter. Concentrations of nitrate and phosphate were highest during winter, with peaks of 18.3 and 1.5 μM, respectively, and began decreasing as chl a concentrations increased in early in spring (March–April). Concentrations of these nutrients remained low during summer and autumn. Zooplankton abundance ranged from 2.63 × 103 to 4.97 × 104 individuals/m3, peaking in early May after peaks in chl a in both 2014 and 2015, and returning to low levels during the winter.
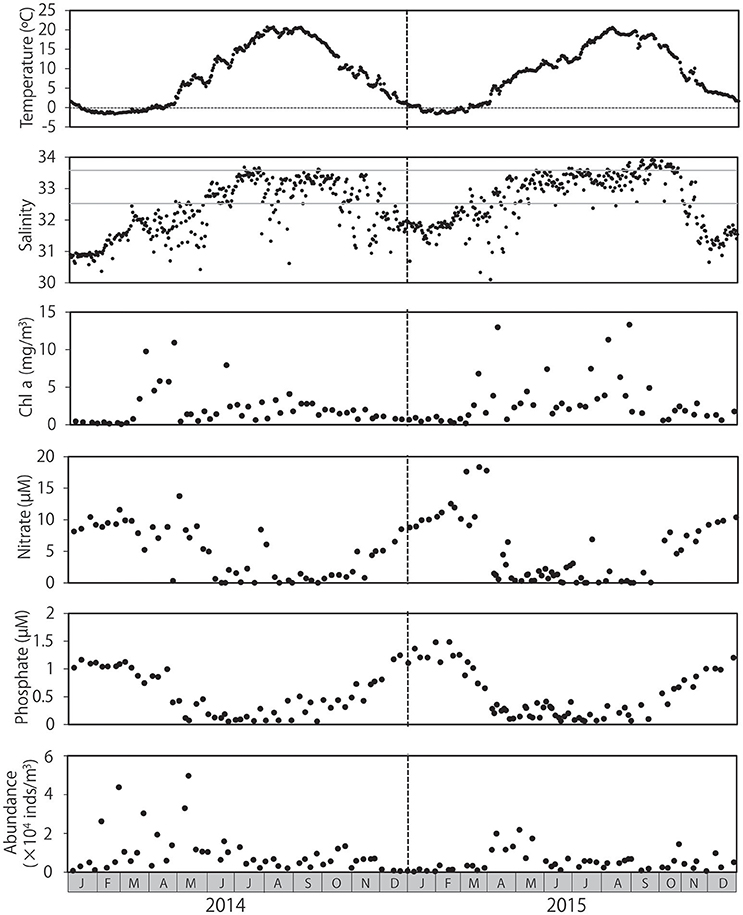
Figure 1. Seasonal changes in environmental conditions and overall zooplankton abundance at Okhotsk Tower, in the coastal waters of northeastern Hokkaido. Water temperature and salinity are provided as means throughout the water column. The concentrations of chlorophyll a, nitrate, and phosphate were measured at the surface. Gray lines in salinity (32.5 and 33.6) indicate the influence of the Okhotsk Sea surface water and Soya Warm Current, respectively.
Zooplankton Diversity across the Sampling Period
A minimum number of 15,939 sequence reads remained within a single community sample after quality-filtering of raw sequence reads. For standardization, 15,939 sequence reads were therefore randomly selected from each of the 101 community samples, and so a total of 1,609,839 reads from the samples were used in the following analyses. After clustering at the 97% similarity threshold and applying the abundance threshold, 561 MOTUs were identified. The number of MOTUs identified across the sampling period was higher than the number of morphological groups identified (201), and the number of taxa attributable to each taxonomic group was generally higher in the metagenetic analysis than in the morphological analysis (Figure 2).
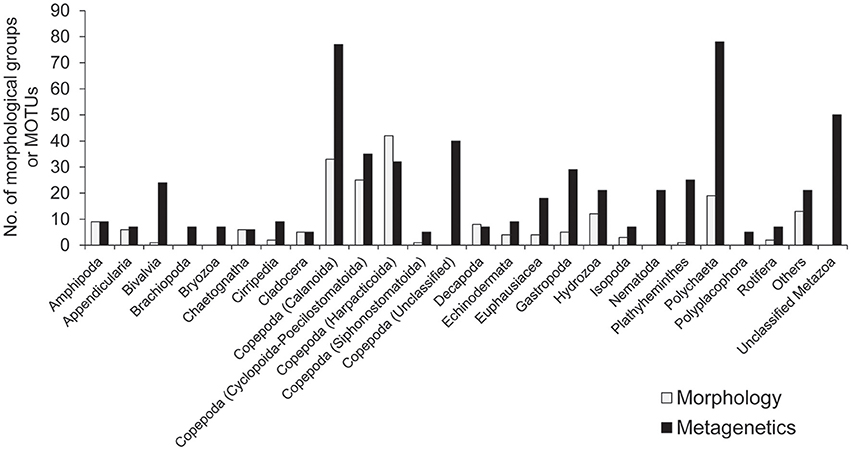
Figure 2. The number of major taxonomic groups identified by morphological and metagenetic analyses. Taxa attributable to Copepoda are further classified to order. Taxonomic groups represented by fewer than five morphological groups and molecular taxonomic units (MOTUs) are grouped under “Others.” Detailed information for all taxonomic groups is shown in Table S1.
Among the taxa identified, the highest diversity was observed in Copepoda, which accounted for 189 MOTUs and 101 morphological groups. Within Copepoda, the majority of diversity was driven by Calanoida (77 MOTUs and 33 morphological groups) and Cyclopoida-Poecilostomatoida (35 MOTUs and 25 morphological groups). Further diversity was contributed by Harpacticoida, the only group in which more morphological groups (42) were detected than MOTUs (32). A further 40 MOTUs attributable to Copepoda could not be classified to lower taxa. In addition to Copepoda, the high diversity detected in the metagenetic analysis was largely driven by meroplankton diversity. Among this group, Polychaeta in Annelida were particularly diverse, contributing 78 MOTUs, although morphological classification identified only 19 groups. The same pattern was observed in various other groups, where the numbers of MOTUs were generally higher (24 in Bivalvia, 9 in Cirripedia, 29 in Gastropoda, and 25 in Platyhelminthes) than the number of morphological groups identified (1 in Bivalvia, 2 in Cirripedia, 5 in Gastropoda, and 1 in Platyhelminthes). In some cases, MOTUs were identified in groups not detected by morphological analysis (7 in Brachiopoda, 7 in Bryozoa, 21 in Nematoda, and 5 in Polyplacophora). Gelatinous plankton attributable to Hydrozoa contributed 21 MOTUs and 12 morphological groups. Fifty MOTUs remained unclassified at any rank lower than Metazoa. Minor taxonomic groups (<5 morphological groups or MOTUs; these comprised a total of 13 morphological groups and 21 MOTUs) are grouped under “Others” in Figure 2, and detailed information about the diversity of all taxonomic groups is shown in Table S1.
Seasonal Changes in Zooplankton Diversity and Community Structure
The number of MOTUs identified per sample ranged from 29 to 133, and was always higher than the number of morphological groups identified, which ranged from 5 to 55 (Figure 3). Seasonal changes in the numbers of MOTUs and morphological groups were correlated (r = 0.64, p < 0.01). Water temperature was positively correlated with the number of MOTUs (r = 0.71, p < 0.01) and the number of morphological groups identified (r = 0.64, p < 0.01), resulting in large numbers of taxa from summer to autumn and low numbers of taxa during the winter. Similar seasonality was detected in the Shannon and Simpson diversity indexes, although the seasonality was not as pronounced in the Simpson index. Significant correlations between the metagenetic and morphological analyses were observed both in the Shannon and Simpson diversity indexes (p < 0.01); however, the correlation was stronger in the Shannon index (r = 0.61) than in the Simpson index (r = 0.32).
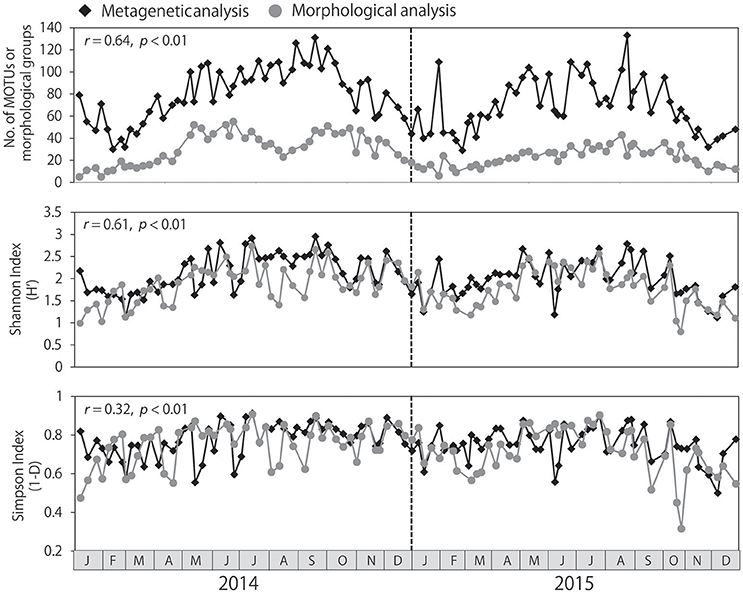
Figure 3. Seasonal changes in zooplankton diversity detected by metagenetic and morphological analyses, including numbers of both molecular taxonomic units (MOTUs) and morphological groups. Shannon and Simpson diversity indexes were calculated based on sequence read numbers in the metagenetic analysis and based on abundance (individuals/m3) in the morphological analysis. Correlations between metagenetic and morphological analyses are represented using Pearson's product-moment correlation coefficients (r).
In addition to seasonal changes in diversity, MDS ordination revealed clear seasonal changes in community structure in both metagenetic and morphological analyses (Figure 4). Water temperature was the strongest environmental factor influencing both abundance-based (sequence reads or numbers of individuals) and non-abundance-based (presence/absence of MOTUs or morphological species) measurements (p < 0.01 for all analyses). Seasonal change was clockwise, characterized by gradual changes in zooplankton communities correlated with increases or decreases in water temperature. The same seasonal pattern was observed in both 2014 and 2015.
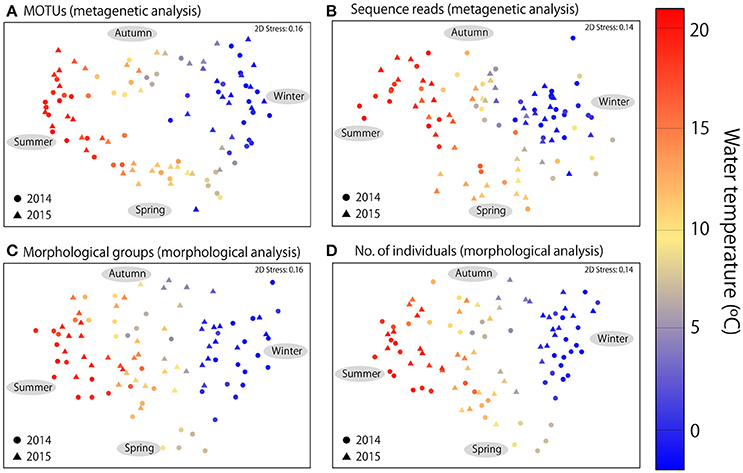
Figure 4. Multidimensional scaling ordination of zooplankton communities. (A) Metagenetic analysis based on presence/absence of MOTUs in samples. (B) Numbers of molecular taxonomic unit (MOTU) sequence reads per sample. (C) Morphological analysis based on presence/absence of morphological groups. (D) Numbers of individuals detected in morphological groups per sample. Proportions of sequence reads and numbers of individuals were logx+1-transformed in abundance-based analyses, and similarities were calculated using the Bray–Curtis similarity index. The color of each sample indicates mean water temperature.
Seasonal Changes in Zooplankton Taxonomic Compositions
Copepod MOTUs contributed substantially to zooplankton diversity in every month throughout the sampling period (ranging from 27.4 to 79.5%; mean 48.9%; Figure 5A). Proportions were particularly high during the winter and low during the summer and autumn. Among copepods, the highest proportion of MOTUs was contributed by Calanoida (average 27.5%), followed by Cyclopoida-Poecilostomatoida (mean 8.9%) and Harpacticoida (mean 7.3%); the mean proportion of unclassified Copepoda was 5.2%. In the morphological analysis, copepods comprised 23.5–100% of samples (mean 58.0%; Figure 6A). Calanoida contributed most to copepod diversity in the morphological analysis (mean 28.5%), followed by Harpacticoida (mean 15.3%) and Cyclopoida-Poecilostomatoida (mean 14.1%).
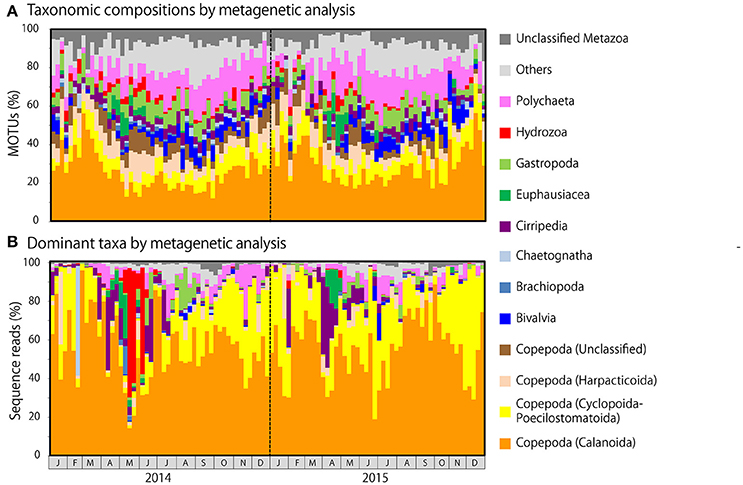
Figure 5. Metagenetic detection of seasonal changes in (A) taxonomic compositions, as measured by the proportion of molecular taxonomic units (MOTUs) per sample attributable to major taxonomic groups, and (B) the identity of dominant taxa, as measured by the proportion of sequence reads per sample attributable to major taxonomic groups. MOTUs and sequence reads attributable to Copepoda are classified to order. Taxonomic groups that did not contribute to ≥10% of all sequences in any sample are grouped under others.
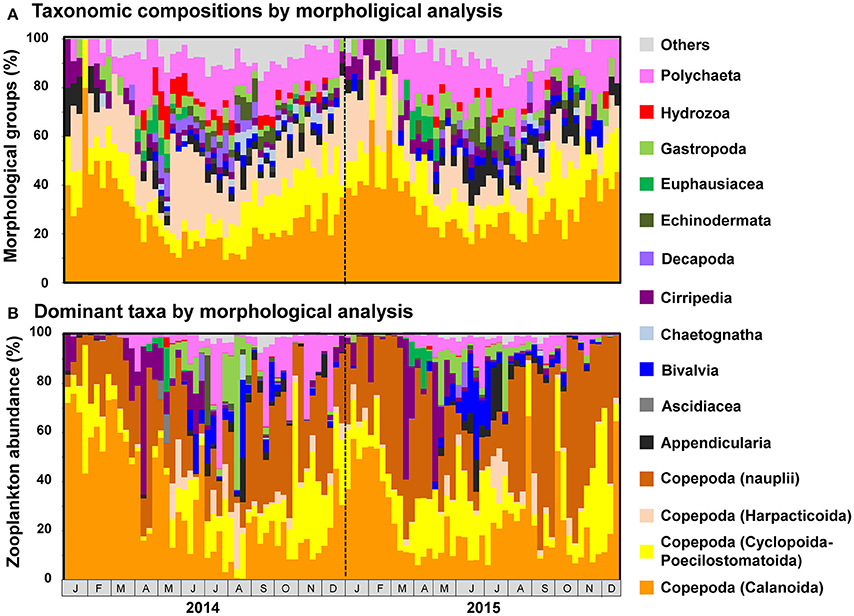
Figure 6. Seasonal changes in (A) taxonomic composition and (B) abundance of major morphological groups, as detected by morphological analysis. Individuals attributable to Copepoda are classified to order. Taxonomic groups that did not contribute to ≥10% of all sequences in any sample are grouped under others.
Among non-copepod taxa, high proportions of MOTUs and morphological groups were attributable to meroplankton, including Bivalvia (MOTU maximum 14.3%, mean 4.7%; morphological maximum 11.1%, mean 2.4%), Cirripedia (MOTU maximum 7.5%, mean 3.8%; morphological maximum 20.0%, mean 3.9%), Gastropoda (MOTU maximum 12.7%, mean 6.0%; morphological maximum 15.4%, mean 4.4%), and Polychaeta (MOTU maximum 25.0%, mean 12.1%; morphological maximum 29.4%, mean 13.4%). Rare taxa were proportionally more common in the metagenetic analysis (mean 12.5%) than in the morphological analysis (mean 5.8%). Unclassified MOTUs comprised 7.0% of taxa in the metagenetic analysis.
Seasonal Changes in Dominant Zooplankton
Copepods were particularly abundant among the sequence reads, accounting for 17.7–99.6% of all reads per month (mean 80.9%; Figure 5B). Among copepods, Calanoida were the most abundant (14.3–96.0%; mean 55.6%), particularly in winter. Cyclopoida-Poecilostomatoida were also common (1.6–69.3%; mean 21.9%), and were sometimes more abundant than Calanoida. Harpacticoida and unclassified Copepoda sequence reads were comparatively uncommon (mean 2.9 and 0.5%, respectively). However, high proportions of Harpacticoida (up to 37.7%) were occasionally observed. Copepods were also abundant in the morphological analysis, accounting for 31.7–100% (mean 77.7%) of individuals (Figure 6B). However, high proportions of Copepoda individuals sampled were unclassified nauplii (mean 30.5%). Among the classified taxa, the most dominant taxa belonged to Calanoida (mean 27.7%), followed by Cyclopoida-Poecilostomatoida (mean 16.6%). Harpacticoida were comparatively uncommon (mean 2.9%).
Besides copepods, meroplankton were also comparatively abundant in sequence reads (Figure 5B). Cirripedia abundance peaked in early spring (maximum: 42.0%). Peaks in Bivalvia and Gastropoda abundance of 14.2 and 20.9%, respectively, were also observed, primarily in summer. Polychaeta abundance, which was observed primarily in spring and autumn, averaged 3.9% and peaked at 28.5%. In addition to meroplankton, Hydrozoa occasionally contributed high proportions of sequence reads (maximum 66.9%, during May and June 2014); however, proportions were generally low. High proportions of Euphausiacea were observed in April in both 2014 and 2015 (maximum 22.6%). In the morphological analysis, high proportions of meroplankton were observed coinciding with those detected by metagenetic analysis, although the maximum proportions differed (60.2% in Cirripedia, 32.1% in Bivalvia, 62.4% in Gastropoda, 53.1% in Polychaeta, 17.7% in Euphausiacea, and 4.2% in Hydrozoa; Figure 6B). Unclassified Metazoa and sequences classified as “Others” were not as abundant in the sequence read analysis (mean 2.7% for “Others,” 0.8% for unclassified Metazoa), and similar results were found in the morphological analysis, where the proportion of “Others” was 0.8%. Peaks in “Others” were observed both in sequence read abundance and in morphological abundance during the summer (peaking at 11.3 and 7.4%, respectively).
Seasonal Patterns of MOTU Abundance in Major Taxonomic Groups
The monthly means of sequence reads and abundance showed similar peaks between metagenetic and morphological analyses of major taxa of Bivalvia, Copepoda, Gastropoda, and Polychaeta (Figure 7). In the morphological analysis, the major morphological groups were the larval stages of Bivalvia, Gastropoda, and Polychaeta, as well as Copepoda nauplii, that were not classified to low ranks. However, metagenetic analysis revealed seasonal changes in the abundance of MOTUs classifiable to various taxonomic groups (Figure 7), including shifts in dominant taxa on a monthly basis. In Bivalvia, the dominant MOTUs in August 2014 belonged to Mytilidae and Veneridae, whereas in October 2014 they belonged to Corbiculidae (Figure 7A). The dominant Bivalvia MOTUs in June 2015 and September 2015 were attributable to Mactridae and Mytilidae, respectively. One MOTU classified to Pectinidae (MOTU 109) was relatively abundant in June 2014 and May 2015. The morphological analysis classified adult Copepoda into species, and both MOTUs and morphological species showed seasonal shifts in dominant taxa (Figure 7B). Correspondence between metagenetic and morphological analyses was observed in Oithonidae (MOTU 1; including Oithona similis), Paracalanidae (MOTU 3; including Paracalanus parvus s. l.), Clausocalanidae (MOTU 6; including Pseudocalanus newmani or P. minutus), and Centropodoidea (MOTUs 2, 4, 9, and 11; including the genus Acartia and Eurytemora). There were, on the other hand, major MOTUs that were only detected in the metagenetic analysis, which were attributed to the families Calanidae (MOTU 5), Pontellidae (MOTU 8), and Metridinidae (MOTU 10). Gastropoda MOTUs were classified to superfamily-level, due to the relatively low sequence variations among taxonomic families. However, MOTU-based analysis successfully detected seasonal changes in dominant Gastropoda, compared to morphological analysis (Figure 7C). The dominant MOTUs Cerithioidea (MOTU 37), Buccinoidea (MOTU 38), and Cephalaspidea (MOTU 43) were replaced by Truncatelloidea (MOTU 29), Caenogastropoda (MOTU 41), and Bulloidea (MOTU 51) during May and August. Polychaeta larval stages were partially classified to family-level by morphological analysis, and metagenetic and morphological analyses showed the same peaks of some dominant taxa, including Spionidae and Sabellariidae (Figure 7D). Seasonal occurrences in dominant Polychaeta were clearly shown by the MOTU-based analysis. Although detailed monthly changes in most of major taxonomic groups were different when comparing 2014 and 2015, Polynoidae (MOTU 14) was dominant from March to July in both years. Spionidae (MOTU 19) showed a high proportion of sequence reads in November and December in 2014, and other dominant MOTUs also showed specific peaks of sequence reads, such as Nephtyidae (MOTU 35) peak in July in both 2014 and 2015.
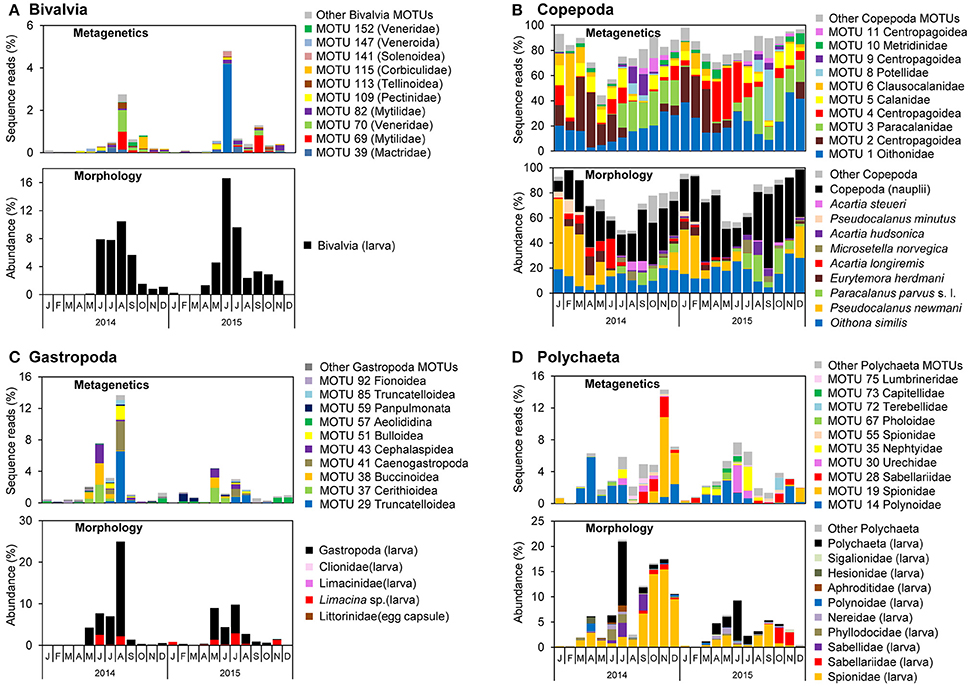
Figure 7. Monthly proportions of sequence reads for major molecular taxonomic units (MOTUs) using metagenetic analysis and abundance using morphological analysis in (A) Bivalvia, (B) Copepoda, (C) Gastropoda, and (D) Polychaeta. Proportions of sequence reads or abundances are calculated per sample and averaged per month.
Discussion
Cryptic Zooplankton Diversity
Metagenetic analysis revealed a higher diversity of zooplankton taxa than those revealed by morphological analysis. The 18S V9 region used in the present study is highly variable; however, species-level data are not sufficiently high-resolution in some taxonomic groups of zooplankton, making accurate classification difficult in certain cases (Wu et al., 2015). Although a high similarity threshold (e.g., 99%) is expected to be used when analyzing metazoan zooplankton (Mohrbeck et al., 2015), we instead used a comparatively low similarity threshold (97%), a technique recommended to prevent overestimations of diversity (Kunin et al., 2010) that is often used in metagenetic analyses of zooplankton using the 18S V9 region (Pearman et al., 2014; Casas et al., 2017). Additionally, we used a strict abundance threshold (eight minimum sequence reads), because rare MOTUs are not always reliable and lead to overestimations of diversity (Bokulich et al., 2013; Hirai et al., 2017b; Leray and Knowlton, 2017; Sommer et al., 2017). Indeed, an even stricter minimum threshold of 16 sequence reads would have identified 429 MOTUs, a number much higher than that detected morphologically. Metagenetic analysis is not free from errors which sometimes lead to the overestimation of MOTU numbers; however, the strict criteria used in the present study nevertheless indicate a richer, often more cryptic diversity than previously recognized based on morphological observations in the study area.
Much of this cryptic diversity was contributed by Copepoda, particularly Calanoida, because molecular techniques are capable of classifying even nauplii and cryptic species (Bucklin et al., 2010). However, a substantial proportion was contributed by meroplankton taxa. Meroplankton taxa are primarily the larvae of benthic organisms, which are difficult to identify morphologically, leading to the increased diversity detected by metagenetic analysis. Although other metagenetic studies targeting the 18S region have reported cryptic diversity among meroplankton (Lindeque et al., 2013; Pearman et al., 2014; Sommer et al., 2017), the contribution of meroplankton to overall diversity was particularly high in the present study. For example, as many Polychaeta MOTUs were detected as calanoid MOTUs. This unexpectedly high diversity in meroplankton may be attributable to shallow water depth in the study area, suggesting that the metagenetic method is especially effective in assessing zooplankton diversity at this location. Metagenetic analysis also detected higher diversity in various other taxonomic groups, including gelatinous organisms such as Hydrozoa, providing further evidence for the existence of cryptic zooplankton diversity in the study area, and suggesting that morphological analysis alone is not sufficient to characterize plankton communities accurately.
Seasonal Changes in Zooplankton Diversity
The seasonal changes in temperature, salinity, chl a, and nutrient content measured in the present study conform with patterns measured from 1996 to 2014 in the study area (Kasai et al., 2017), and changes in zooplankton abundance followed patterns reported previously (Hamaoka et al., 2010). Although we observed abrupt changes in environmental variables, such as declines in salinity and increases in chl a, possibly caused by increased rainfall, influx of inland water, or turbulence, such changes have been reported as characteristic of study sites located in coastal regions (Hamasaki et al., 1998; Kasai et al., 2017). Thus, zooplankton samples used in this study were collected under typical environmental conditions and can be considered representative of common seasonal changes of zooplankton communities in the area.
The substantial variations in environmental conditions typical of the study area drove seasonal changes in zooplankton diversity. Patterns of presence and absence in different taxa differed clearly in winter and summer in both metagenetic and morphological analyses in correlation with water temperature. This result conforms to those reported in a previous morphological study that showed seasonal variability in species distribution was driven by differing water masses (Hamaoka et al., 2010). Because high zooplankton diversity, such as in copepods, is primarily observed in warm-water masses (Rombouts et al., 2009; Tittensor et al., 2010), water exchanges during summer and autumn may have generated the high diversity detected in the present study. Additionally, proportionally more non-copepod taxa, particularly among meroplankton, were detected by metagenetic analysis than by morphological analysis throughout the year, suggesting that meroplankton contribute substantially more to diversity than previously recognized, particularly from summer to autumn. Thus, metagenetic analysis revealed clear seasonality in zooplankton diversity at high taxonomic resolution, and environmental correlations suggest that these changes in diversity were driven by water mass exchanges and interactions between planktonic and benthic communities.
Seasonal Changes in Dominant Taxa
Although there are methodological limitations (e.g., PCR bias, gene copy numbers), sequence reads provide semi-quantitative information for zooplankton biomass (Lindeque et al., 2013; Hirai et al., 2015a; Albaina et al., 2016; Clarke et al., 2017), which is not directly comparable with the number of individuals in morphological analysis. We nevertheless observed similar seasonal patterns in diversity indexes, abundance-based community structures, and taxonomic compositions between the metagenetic and morphological analyses. These results support the utility of abundance-based analysis of metagenetic data in detecting dominant taxa. The relatively low correlation in Simpson index and some discrepancies in dominant taxa we measured may have been caused by the use of abundance rather than biomass data in the morphological analysis. Furthermore, fluctuations in diversity patterns, including significant dips in diversity, particularly in the Simpson index, may have been caused by occasional changes in environmental variables, or by the presence of large-bodied taxa (e.g., high proportions of Chaetognatha and Hydrozoa in 2014).
The metagenetic analysis provided detailed taxonomic data using sequence reads, whereas morphological classification was often unsuccessful. Our MOTU-level analysis of Bivalvia, Gastropoda, and Polychaeta revealed seasonal and annual changes in the occurrence and dominance of certain taxa that were not detected in the morphological analysis, due to the difficulty of identifying meroplankton. Additionally, copepod nauplii were particularly abundant in the morphological analysis; however, most sequence reads of copepods were successfully classified into detailed taxonomic groups throughout the sampling period in the metagenetic analysis. Although the majority of sequence reads were attributable to copepods, non-copepod taxa made non-trivial contributions, particularly from summer to autumn. Indeed, high diversity index values were observed in warm periods, indicating the co-existence of multiple species from various groups, which may promote zooplankton productivity in the study area. The proportional contribution of meroplankton to sequence read counts was greater than that detected in a metagenetic study in Tosa Bay, off Japan, (Hirai et al., 2017a) or in reefs in the Red Sea (Pearman et al., 2014). Furthermore, stable isotope analyses in the coastal waters of northeastern Hokkaido indicated an interaction between planktonic and benthic organisms (Hiwatari et al., 2011). As observed for zooplankton diversity, environmental factors such as water temperature, driven by water mass exchange, and a strong bentho-pelagic interaction in coastal waters, acted together to influence seasonal changes in dominant taxa.
The Metagenetic Approach as a Tool for Monitoring Zooplankton Communities
Metagenetic approaches provide a sensitive method for monitoring zooplankton diversity, as demonstrated in studies of zooplankton communities in the English Channel (Lindeque et al., 2013), tropical and subtropical Pacific (Hirai and Tsuda, 2015; Hirai et al., 2015a), station ALOHA (Sommer et al., 2017), Red Sea (Pearman et al., 2014; Pearman and Irigoien, 2015; Casas et al., 2017), Monterey Bay (Harvey et al., 2017), Canadian coastal areas (Chain et al., 2016), and Storm Bay (Clarke et al., 2017). Given the alignment of results generated from metagenetic and morphological methods, we conclude that metagenetic analysis was successfully used to monitor changes in zooplankton diversity and communities in the study area, and that this in turn corresponded with environmental changes, particularly water temperature. Metagenetic analysis yielded higher taxonomic coverage and resolution, and the strong bentho-pelagic interactions and importance of meroplankton in this study meant that the metagenetic technique was particularly effective in evaluating zooplankton diversity in the study area. Furthermore, because the MOTU-level analysis of Bivalvia detected a Pectinidae MOTU, possibly derived from Japanese scallop (Mizuhopecten yessoensis), we also posit that the technique is useful in monitoring the dynamics of specific taxa, including commercially important species in the study area. In a previous study, immunostaining was used to detect M. yessoensis larvae in the study area (Katakura et al., 2013, 2015); however, metagenetic analysis functions as a taxonomically comprehensive method of classification that is not restricted to a specific species. Conversely, metagenetic analysis provides only semi-quantitative data (e.g., proportions of sequence reads), and thus, does not provide detailed information concerning the size, number, or developmental stage of individuals detected (Lindeque et al., 2013). Thus, a combined analysis, using both morphological and metagenetic techniques, is the optimal approach for monitoring zooplankton communities.
Relatively large numbers of sequences have been registered in 18S rRNA gene (Quast et al., 2013; de Vargas et al., 2015); however, we encountered many unclassified metazoan MOTUs. To further develop metagenetic analysis techniques, we recommend additional efforts to accumulate even more zooplankton sequences than exist in current databases. Furthermore, we used the 18S V9 region because of its capacity to identify various metazoan zooplankton; however, marker choice affects the results of the metagenetic analysis. For example, although mitochondrial genes such as COI have high species-level resolution, primer mismatches remain common, particularly in highly variable regions of genes (Deagle et al., 2014; Clarke et al., 2017). Thus, molecular markers should be chosen according to the purpose of each study.
The coastal waters of the Okhotsk Sea provide both a productive fishery ground and an important locality for monitoring marine ecosystems (Radchenko et al., 2010). Because climate and environmental changes are proceeding rapidly, it is important to understand existing zooplankton community structures when predicting future changes. However, the present study generated results over a span of only 2 years; accordingly, additional longer-term zooplankton monitoring efforts are recommended to further characterize marine ecosystems in the region and to identify the effects of long-term climate changes on these ecosystems. Nevertheless, because the data generated in this study do not depend on subtle morphological differences or on taxonomic expertise, they will provide a reliable and straightforward dataset for implementation in future studies using different spatial and temporal spans. The present study showed that metagenetic analysis successfully detected cryptic diversity in the study area, with implications for detailed taxonomic information concerning dominant zooplankton taxa. Thus, we consider metagenetic analysis is an especially useful tool for monitoring metazoan zooplankton in the coastal waters of the Okhotsk Sea, northeastern Hokkaido.
Data Accessibility
Raw Illumina MiSeq data are available from the DDBJ Sequence Read Archive under accession number DRA005786.
Author Contributions
JH, SK, and SN: contributed to research design; SK: carried out field sampling; HK: contributed to the measurement of environmental variables; JH: contributed to laboratory work, data analysis, and writing the paper; all authors read and approved the final manuscript.
Funding
This study was supported by a research project grant from the Japan Fisheries Research and Education Agency (FRA) and a grant from the Japan Society for the Promotion of Science (grant number 247024).
Conflict of Interest Statement
The authors declare that the research was conducted in the absence of any commercial or financial relationships that could be construed as a potential conflict of interest.
Acknowledgments
We thank Hitomi Yoshida and Katsushi Murai (Garinko & Okhotsk Tower Co., Ltd.) for assisting with sampling using the plankton net and for carrying out CTD observation around the Okhotsk Tower. We also thank Masaki Iwabuchi (Econixe Co., Ltd.) for carrying out the morphological classifications of zooplankton sampled in our study.
Supplementary Material
The Supplementary Material for this article can be found online at: https://www.frontiersin.org/articles/10.3389/fmars.2017.00379/full#supplementary-material
References
Albaina, A., Aguirre, M., Abad, D., Santos, M., and Estonba, A. (2016). 18S rRNA V9 metabarcoding for diet characterization: a critical evaluation with two sympatric zooplanktivorous fish species. Ecol. Evol. 6, 1809–1824. doi: 10.1002/ece3.1986
Amaral-Zettler, L. A., McCliment, E. A., Ducklow, H. W., and Huse, S. M. (2009). A method for studying protistan diversity using massively parallel sequencing of V9 hypervariable regions of small-subunit ribosomal RNA genes. PLoS ONE 4:e6372. doi: 10.1371/journal.pone.0006372
Aota, M. (1985). “Water masses in the coastal waters of Okhotsk Sea,” in Coastal Oceanography of Japanese Islands, ed The Oceanographic Society of Japan (Tokyo: Tokai University Press), 13–19.
Asami, H., Shimada, H., Sawada, M., Sato, H., Miyakoshi, Y., Ando, D., et al. (2007). Influence of physical parameters on zooplankton variability during early ocean life of juvenile chum salmon in the coastal waters of eastern Hokkaido, Okhotsk Sea. N. Pac. Anadr. Fish Comm. Bull. 4, 211–221.
Aylagas, E., Borja, Á., Irigoien, X., and Rodríguez-Ezpeleta, N. (2016). Benchmarking DNA metabarcoding for biodiversity-based monitoring and assessment. Front. Mar. Sci. 3:96. doi: 10.3389/fmars.2016.00096
Beaugrand, G., Brander, K. M., Lindley, J. A., Souissi, S., and Reid, P. C. (2003). Plankton effect on cod recruitment in the North Sea. Nature 426, 661–664. doi: 10.1038/nature02164
Bokulich, N. A., Subramanian, S., Faith, J. J., Gevers, D., Gordon, J. I., Knight, R., et al. (2013). Quality-filtering vastly improves diversity estimates from illumina amplicon sequencing. Nat. Methods 10, 57–59. doi: 10.1038/nmeth.2276
Bucklin, A., Lindeque, P. K., Rodriguez-Ezpeleta, N., Albaina, A., and Lehtiniemi, M. (2016). Metabarcoding of marine zooplankton: prospects, progress and pitfalls. J. Plankton Res. 38, 393–400. doi: 10.1093/plankt/fbw023
Bucklin, A., Nishida, S., Schnack-Schiel, S., Wiebe, P. H., Lindsay, D., Machida, R. J., et al. (2010). “A census of zooplankton of the global ocean,” in Life in the World's Oceans: Diversity, Distribution and Abundance, ed A. D. McIntyre (Oxford: Blackwell Publishing Ltd.), 247–265.
Casas, L., Pearman, J. K., and Irigoien, X. (2017). Metabarcoding reveals seasonal and temperature-dependent succession of zooplankton communities in the Red Sea. Front. Mar. Sci. 4:241. doi: 10.3389/fmars.2017.00241
Chain, F. J. J., Brown, E. A., MacIsaac, H. J., and Cristescu, M. E. (2016). Metabarcoding reveals strong spatial structure and temporal turnover of zooplankton communities among marine and freshwater ports. Divers. Distrib. 22, 493–504. doi: 10.1111/ddi.12427
Clarke, L. J., Beard, J. M., Swadling, K. M., and Deagle, B. E. (2017). Effect of marker choice and thermal cycling protocol on zooplankton DNA metabarcoding studies. Ecol. Evol. 7, 873–883. doi: 10.1002/ece3.2667
Danovaro, R., Carugati, L., Berzano, M., Cahill, A. E., Carvalho, S., Chenuil, A., et al. (2016). Implementing and innovating marine monitoring approaches for assessing marine environmental status. Front. Mar. Sci. 3:213. doi: 10.3389/fmars.2016.00213
Deagle, B. E., Jarman, S. N., Coissac, E., Pompanon, F., and Taberlet, P. (2014). DNA metabarcoding and the cytochrome c oxidase subunit I marker: not a perfect match. Biol. Lett. 10:e20140562. doi: 10.1098/rsbl.2014.0562
de Vargas, C., Audic, S., Henry, N., Decelle, J., Mahé, F., Logares, R., et al. (2015). Eukaryotic plankton diversity in the sunlit ocean. Science 34:1261605. doi: 10.1126/science.1261605
Edgar, R. C., Haas, B. J., Clemente, J. C., Quince, C., and Knight, R. (2011). UCHIME improves sensitivity and speed of chimera detection. Bioinformatics 27, 2194–2200. doi: 10.1093/bioinformatics/btr381
Hamaoka, S., Katakura, S., Nagata, R., and Hirakawa, K. (2010). “Seasonal variability in zooplankton community, with special reference to copepods in the inshore waters,” in Proceedings 25th International Symosium on Okhotsk Sea & Sea Ice, Vol. 25 (Mombetsu), 169–172.
Hamasaki, K., Ikeda, M., Ishikawa, M., Shirasawa, K., and Taguchi, S. (1998). Seasonal variability of size-fractionated chlorophyll a in Monbetsu Harbor, Hokkaido, northern Japan. Plankton Biol. Ecol. 45, 151–158.
Harvey, J. B. J., Johnson, S. B., Fisher, J. L., Peterson, W. T., and Vrijenhoek, R. C. (2017). Comparison of morphological and next generation DNA sequencing methods for assessing zooplankton assemblages. J. Exp. Mar. Biol. Ecol. 487, 113–126. doi: 10.1016/j.jembe.2016.12.002
Hays, G. C., Richardson, A. J., and Robinson, C. (2005). Climate change and marine plankton. Trends Ecol. Evol. 20, 337–344. doi: 10.1016/j.tree.2005.03.004
Hirai, J., Hidaka, K., Nagai, S., and Ichikawa, T. (2017a). Molecular-based diet analysis of the early post-larvae of Japanese sardine Sardinops melanostictus and Pacific round herring Etrumeus teres. Mar. Ecol. Prog. Ser. 564, 99–113. doi: 10.3354/meps12008
Hirai, J., Kuriyama, M., Ichikawa, T., Hidaka, K., and Tsuda, A. (2015a). A metagenetic approach for revealing community structure of marine planktonic copepods. Mol. Ecol. Resour. 15, 68–80. doi: 10.1111/1755-0998.12294
Hirai, J., Nagai, S., and Hidaka, K. (2017b). Evaluation of metagenetic community analysis of planktonic copepods using illumina MiSeq: comparisons with morphological classification and metagenetic analysis using Roche 454. PLoS ONE 12:e0181452. doi: 10.1371/journal.pone.0181452
Hirai, J., and Tsuda, A. (2015). Metagenetic community analysis of epipelagic planktonic copepods in the tropical and subtropical Pacific. Mar. Ecol. Prog. Ser. 534, 65–78. doi: 10.3354/meps11404
Hirai, J., Yasuike, M., Fujiwara, A., Nakamura, Y., Hamaoka, S., Katakura, S., et al. (2015b). Effects of plankton net characteristics on metagenetic community analysis of metazoan zooplankton in a coastal marine ecosystem. J. Exp. Mar. Biol. Ecol. 469, 36–43. doi: 10.1016/j.jembe.2015.04.011
Hiwatari, T., Koshikawa, H., Nagata, R., Suda, Y., Hamaoka, S., and Kohata, K. (2011). Trophic relationships in early spring along the Okhotsk coast of Hokkaido, Japan, as traced by stable carbon and nitrogen isotopes. Plank. Benth. Res. 6, 56–67. doi: 10.3800/pbr.6.56
Kasai, H., Nagata, R., Murai, K., Katakura, S., Tateyama, K., and Hamaoka, S. (2017). Seasonal change in oceanographic environments and the influence of interannual variation in the timing of sea-ice retreat on chlorophyll a concentration in the coastal water of Northeastern Hokkaido along the Okhotsk Sea. Bull. Coast. Oceanogr. 54, 181–192.
Katakura, S., Hamaoka, S., Konashi, K., Mizuno, K., and Shimizu, Y. (2013). “The occurrence pattern of Japanese scallop larvae Mizuhopecten yessoensis by using an immunostaining method in water around the Okhotsk Tower, Mombetsu, in 2011,” in Proceedings 28th International. Symposium on Okhotsk Sea & Sea Ice, Vol. 28 (Mombetsu), 38–41.
Katakura, S., Hamaoka, S., Miyagawa, T., Kawasaki, T., and Shimizu, Y. (2015). “Occurrence and abundance Japanese scallop larvae in water around Okhotsk Tower in 2011–2014,” in Proceedings 30th International Symposium on Okhotsk Sea & Sea Ice, Vol. 30 (Mombetsu), 35–38.
Katoh, K., and Standley, D. M. (2013). MAFFT multiple sequence alignment software version 7: improvements in performance and usability. Mol. Biol. Evol. 30, 772–780. doi: 10.1093/molbev/mst010
Kunin, V., Engelbrektson, A., Ochman, H., and Hugenholtz, P. (2010). Wrinkles in the rare biosphere: pyrosequencing errors can lead to artificial inflation of diversity estimates. Environ. Microbiol. 12, 118–123. doi: 10.1111/j.1462-2920.2009.02051.x
Leray, M., and Knowlton, N. (2017). Random sampling causes the low reproducibility of rare eukaryotic OTUs in illumina COI metabarcoding. PeerJ 5:e3006. doi: 10.7717/peerj.3006
Lindeque, P. K., Parry, H. E., Harmer, R. A., Somerfield, P. J., and Atkinson, A. (2013). Next generation sequencing reveals the hidden diversity of zooplankton assemblages. PLoS ONE 8:e81327. doi: 10.1371/journal.pone.0081327
Mohrbeck, I., Raupach, M. J., Arbizu, P. M., Knebelsberger, T., and Laakmann, S. (2015). High-throughput sequencing—the key to rapid biodiversity assessment of marine Metazoa? PLoS ONE 10:e0140342. doi: 10.1371/journal.pone.0140342
Nagai, S., Urushibara, S., Yasuike, M., Nakamura, Y., Fujiwara, A., Takano, Y., et al. (2015). “Plankton metagenomics in Okhotsk Sea,” in Proceedings 30th International Symposium on Okhotsk Sea & Sea Ice (Mombetsu), 30, 31–34.
Nagata, M., Miyakoshi, Y., Fujiwara, M., Ando, D., Asami, H., Kaneko, T., et al. (2007). Spatial distribution and feeding interactions between chum and pink salmon juveniles in the coastal water of the Okhotsk Sea in the eastern Hokkaido. N. Pac. Anadr. Fish Comm. Tech. Rep. 7, 88–91.
Ohshima, K. I. (2008). Changes in the sea of Okhotsk due to lobal warming – weakening pump function to the North Pacific. PICES Sci. Rep. 36, 16–20.
Pearman, J. K., El-Sherbiny, M., Lanzén, A., Al-Aidaroos, A. M., and Irigoien, X. (2014). Zooplankton diversity across three Red Sea reefs using pyrosequencing. Front. Mar. Sci. 1:27. doi: 10.3389/fmars.2014.00027
Pearman, J. K., and Irigoien, X. (2015). Assessment of zooplankton community composition along a depth profile in the central Red Sea. PLoS ONE 10:e0133487. doi: 10.1371/journal.pone.0133487
Quast, C., Pruesse, E., Yilmaz, P., Gerken, J., Schweer, T., Yarza, P., et al. (2013). The SILVA ribosomal RNA gene database project: improved data processing and web-based tools. Nucleic Acids Res. 41, D590–D596. doi: 10.1093/nar/gks1219
Radchenko, V. I., Dulepova, E. P., Figurkin, A. L., Katugin, O. N., Ohshima, K., Nishioka, J., et al. (2010). “Status and trends of the Sea of Okhotsk region, 2003–2008,” in Marine Ecosystems of the North Pacific Ocean, 2003–2008, PICES Special Publication 4, eds S. M. McKinnell and M. J. Dagg (Sidney: North Pacific Marine Science Organization), 268–299.
Richardson, A. R. (2008). In hot water: zooplankton and climate change. ICES J. Mar. Sci. 65, 279–295. doi: 10.1093/icesjms/fsn028
Roemmich, D., and McGowan, J. (1995). Climatic warming and the decline of zooplankton in the California current. Science 267, 1324–1326. doi: 10.1126/science.267.5202.1324
Rombouts, I., Beaugrand, G., Ibanez, F., Gasparini, S., Chiba, S., and Legendre, L. (2009). Global latitudinal variations in marine copepod diversity and environmental factors. Proc. R. Soc. Lond. B 276, 3053–3062. doi: 10.1098/rspb.2009.0742
Schloss, P. D., Westcott, S. L., Ryabin, T., Hall, J. R., Hartmann, M., Hollister, E. B., et al. (2009). Introducing MOTHUR: open-source, platform-independent, community-supported software for describing and comparing microbial communities. Appl. Environ. Microbiol. 75, 7537–7541. doi: 10.1128/AEM.01541-09
Sommer, S. A., Van Woudenberg, L., Lenz, P. H., Cepeda, G., and Goetze, E. (2017). Vertical gradients in species richness and community composition across the twilight zone in the North Pacific Subtropical Gyre. Mol. Ecol. 26, 6136–6156. doi: 10.1111/mec.14286
Taguchi, S., Satoh, F., Hamaoka, S., Ikeda, M., Ishikawa, M., and Shirasawa, K. (2000). Effect of ice algal community on the increase of chlorophyll a concentration during spring in coastal water of the Sea of Okhotsk. Polar Biosci. 13, 1–14.
ter Braak, C. F., and Verdonschot, P. M. (1995). Canonical correspondence analysis and related multivariate methods in aquatic ecology. Aquat. Sci. 57, 255–289. doi: 10.1007/BF00877430
Tittensor, D. P., Mora, C., Jetz, W., Lotze, H. K., Ricard, D., Berghe, E. V., et al. (2010). Global patterns and predictors of marine biodiversity across taxa. Nature 466, 1098–1101. doi: 10.1038/nature09329
Wang, Q., Garrity, G. M., Tiedje, J. M., and Cole, J. R. (2007). Naïve Bayesian classifier for rapid assignment of rRNA sequences into the new bacterial taxonomy. Appl. Environ. Microbiol. 73, 5261–5267. doi: 10.1128/AEM.00062-07
Keywords: zooplankton, metagenetics, diversity, coastal waters, Okhotsk Sea
Citation: Hirai J, Katakura S, Kasai H and Nagai S (2017) Cryptic Zooplankton Diversity Revealed by a Metagenetic Approach to Monitoring Metazoan Communities in the Coastal Waters of the Okhotsk Sea, Northeastern Hokkaido. Front. Mar. Sci. 4:379. doi: 10.3389/fmars.2017.00379
Received: 09 August 2017; Accepted: 10 November 2017;
Published: 11 December 2017.
Edited by:
Pei-Yuan Qian, Hong Kong University of Science and Technology, Hong KongReviewed by:
Yong Wang, Sanya Institute of Deep-Sea Science and Engineering (CAS), ChinaGáspár Jékely, University of Exeter, United Kingdom
Copyright © 2017 Hirai, Katakura, Kasai and Nagai. This is an open-access article distributed under the terms of the Creative Commons Attribution License (CC BY). The use, distribution or reproduction in other forums is permitted, provided the original author(s) or licensor are credited and that the original publication in this journal is cited, in accordance with accepted academic practice. No use, distribution or reproduction is permitted which does not comply with these terms.
*Correspondence: Junya Hirai, aGlyYWlAYW9yaS51LXRva3lvLmFjLmpw