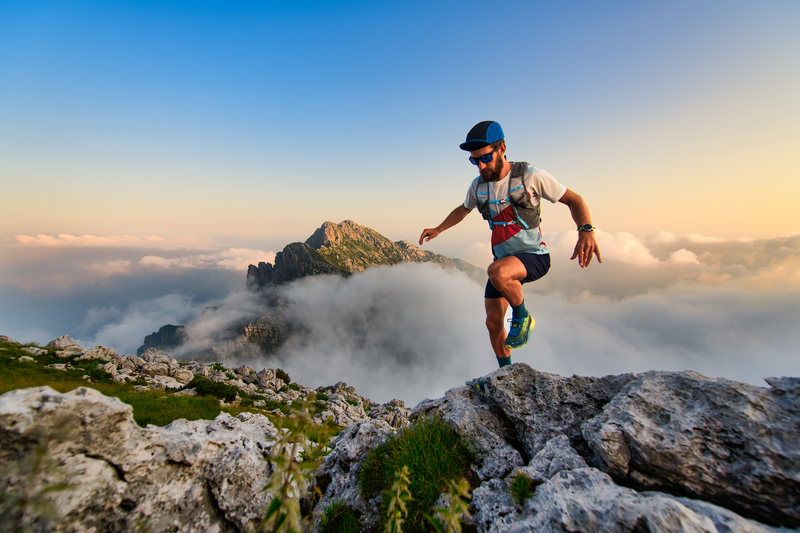
94% of researchers rate our articles as excellent or good
Learn more about the work of our research integrity team to safeguard the quality of each article we publish.
Find out more
REVIEW article
Front. Mar. Sci. , 12 September 2017
Sec. Global Change and the Future Ocean
Volume 4 - 2017 | https://doi.org/10.3389/fmars.2017.00289
This article is part of the Research Topic Seasonal to decadal prediction of marine ecosystems: opportunities, approaches and applications View all 11 articles
Recent years have seen a rapid expansion in the ability of earth system models to describe and predict the physical state of the ocean. Skilful forecasts ranging from seasonal (3 months) to decadal (5–10 years) time scales are now a reality. With the advance of these forecasts of ocean physics, the first generation of marine ecological forecasts has started to emerge. Such forecasts are potentially of great value in the management of living marine resources and for all of those who are dependent on the ocean for both nutrition and their livelihood; however, this is still a field in its infancy. We review the state of the art in this emerging field and identify the lessons that can be learnt and carried forward from these pioneering efforts. The majority of this first wave of products are forecasts of spatial distributions, possibly reflecting the inherent suitability of this response variable to the task of forecasting. Promising developments are also seen in forecasting fish-stock recruitment where, despite well-recognized challenges in understanding and predicting this response, new process knowledge and model approaches that could form a basis for forecasting are becoming available. Forecasts of phenology and coral-bleaching events are also being applied to monitoring and industry decisions. Moving marine ecological forecasting forward will require striking a balance between what is feasible and what is useful. We propose here a set of criteria to quickly identify “low-hanging fruit” that can potentially be predicted; however, ensuring the usefulness of forecast products also requires close collaboration with actively engaged end-users. Realizing the full potential of marine ecological forecasting will require bridging the gaps between marine ecology and climatology on the one-hand, and between science and end-users on the other. Nevertheless, the successes seen thus far and the potential to develop further products suggest that the field of marine ecological forecasting can be expected to flourish in the coming years.
Rapid recent advances in observing and modeling the earth system have driven a quiet revolution in our ability to forecast the evolution of our planet's weather and climate (Bauer et al., 2015). Some of the most impressive results have been seen in the oceanic domain (Meehl et al., 2014), where the slow dynamics and long memory of the ocean readily lend themselves to forecast timescales dramatically longer than in the atmosphere. Skilful forecasts on the annual and even decadal scale of sea surface temperature (SST) and upper ocean heat and salt content (Smith et al., 2007; Keenlyside et al., 2008; Doblas-Reyes et al., 2011; Corti et al., 2012; Matei et al., 2012b; van Oldenborgh et al., 2012), the Atlantic Meridional Overturning Circulation (e.g., Matei et al., 2012a), net primary productivity (e.g., Séférian et al., 2014), the north Atlantic sub-polar gyre (e.g., Wouters et al., 2013), and the uptake of CO2 from the atmosphere (e.g., Li et al., 2016) have all been demonstrated thus far, and this list is expected to continue to grow.
This newfound predictive skill of the ocean represents a tremendous opportunity for society as a whole, and particularly for the parts of it that are most closely linked to the ocean. The high variability of living marine resources is widely recognized as arising in part from interactions with the physical environment (e.g., temperature, salinity, currents) (Drinkwater et al., 2010; Hollowed et al., 2013; Petitgas et al., 2013). This variability manifests itself not just in terms of productivity (e.g., yield of fisheries) but also in terms of spatial distribution (e.g., shifts of fish into new jurisdictions) and the timing of key events (e.g., migrations, spawning), and even propagates further into the human systems dependent on the ocean (e.g., fishing practices, community revenue, and employment associated with commercial and recreational fisheries). In principle, the predictability of the physical system could allow much of this variability to be foreseen: such predictions could be used to both adapt to and mitigate the worst impacts of variability for individual stakeholders and society alike, and to optimize monitoring, exploitation, and management of these resources (Hobday et al., 2016; Tommasi et al., 2017a).
Unfortunately, generating such forecasts of biological systems is not entirely straightforward. Most climate models only produce forecasts of physical variables, such as temperature, salinity, and ocean currents, rather than the variables of direct interest to marine resource management and ecosystem applications. An intermediate “translation” step is therefore usually required, where biological models convert forecasts of the physical environment to forecasts of the biological environment. However, the biological knowledge required to make this linkage is often either poor, or entirely absent; fisheries scientists, for example, have been trying to generate environmentally-driven predictions of fish stock recruitment for close to a century now with little success (e.g., Myers, 1998). In fact, just 15 out of 1,250 fish stocks globally (<2%) incorporate any form of environmental information in the generation of their tactical advice and management (Skern-Mauritzen et al., 2016). Nevertheless, the first generation of forecast products for applications to marine living resources is now appearing, starting in Australia nearly a decade ago (Hobday et al., 2011; Eveson et al., 2015) and more recently in North America (Table 1).
Table 1. Overview of operational nowcast and forecast ecological products for application in the management of living marine resources found by the authors.
Here we take stock of the progress made thus far in applied forecasting of marine ecological quantities, with a view toward the next steps. We focus our review away from the predictability of the physical components of the system, for which the reader is referred to numerous reviews already covering the topic (Meehl et al., 2014; Bauer et al., 2015; Stock et al., 2015) and thus do not comment on the improvements needed for the physical side of marine prediction models. We also restrict our focus to applications of relevance to the management and exploitation of living marine resources and therefore exclude human-health related forecasts (e.g., of harmful algal blooms (e.g., NOAA, 2016), outbreaks of Vibrio sp.) and marine pathogen outbreaks (e.g., Constantin de Magny et al., 2009; Maynard et al., 2016). Instead, we focus here on examples of forecast products relating to living marine resources, covering their productivity, spatial distribution and phenology and associated human systems in turn. The strengths, weaknesses, lessons learned, and future prospects of each of these types of forecasts are examined. We then synthesize these experiences into a set of recommendations to facilitate the field's future advancement, including the identification of research priorities. Through this review, we lay out a roadmap for the future development of this new and promising field.
As a starting point for this review, we have collated and summarized currently available forecast products relating to living marine resources (Table 1, Figure 1). We collected forecast products that the authors were familiar with, and complemented this with a brief literature search: while this is by no means an exhaustive list, we believe that it nevertheless covers the majority of products available today. We restricted the results to examples of products that are currently being produced and updated regularly and that are publicly available. Long-term biological responses to a changing climate were excluded as they are projections rather the predictions: instead the focus was on nowcast-to-decadal scale forecasts. We also only focus on products where environmental or ecological factors outside the system of interest drive the forecast, thereby excluding persistence forecasts and projections based on population dynamics (as is common in e.g., fisheries management). Similarly, we restrict the focus to situations where there are specific ecological forecasts made. As a result, some examples of environmentally-informed fisheries management in which the management might be informed based on relationships between a physical variable and a biological response (eg., Skern-Mauritzen et al., 2016), but where these relationships are not (yet) used in forecasting stock dynamics, are excluded.
Figure 1. Map of known examples of marine ecological nowcast and forecast products listed in Table 1. Details of these products are given in this table for the corresponding number. Note that the Coral Reef Watch forecast product (number 9, blue circle) covers the globe between 45°N and 45°S (dotted blue box).
The results provide several clear insights into the current state of forecasting of living marine resources. Firstly, there are distinct spatial patterns in the distribution of these forecast products, with the majority occurring in North America (Figure 1). Models also tended to be almost exclusively empirical (correlative) in nature: a notable exception are the forecasts of the intensity and spatial distribution of coral bleaching (forecast product 9), which have a strong mechanistic underpinning. Variables predicted could otherwise be grouped into predictions of productivity, spatial distribution or phenology: of these, forecasts of spatial distributions were clearly the most common. We examine these products in more detail below.
Changes in the productivity of fisheries result from changes in all processes that affect current and future exploitable biomass and fishery yields (e.g., growth, survival, and reproduction). The relative importance of these factors is strongly linked to the life-history and fishery of the stock. For long-lived and late-maturing species that have fisheries targeting many age-groups (e.g., temperate-boreal gadoids and flatfishes), changes in growth and mortality of fish currently in the water are critical: in these cases young fish are recruited to the fishery at age 2 or later, and their impact on yield therefore occurs 1–2+ generations into the future (Basson, 1999; Brander, 2003; Planque et al., 2003; Tommasi et al., 2017a), while recruitment forecasts do not become useful until 5–10+ years into the future (Basson, 1999; Brander, 2003; Planque et al., 2003). Forecasting changes in growth and natural mortality 1–5 years ahead would be of more immediate benefit to the management of such species. For shorter-lived species, and those captured at young ages (e.g., sardine, anchovy, sprat, sandeel, and capelin), however, the need for predictability is reversed: fisheries for these stocks often depend on a very small number of age groups (e.g., 1–2) and large inter-annual variations in year-class strength are more important for the future fishery yields than changes in growth and mortality.
Recruitment forecasting, however, has been, and still is, a major challenge in fisheries oceanography and management (Houde, 2008; Hare, 2014). Numerous published relationships between recruitment and environmental variables have broken down when updated with new data (Myers, 1998), and few such relationships are used in operational fisheries management (Skern-Mauritzen et al., 2016). However, links between recruitment and the environment are nevertheless well-recognized: for example, environmental variability has been shown to be more influential in the recruitment of many fish stocks than spawner biomass (Szuwalski et al., 2015), and explains more variation in productivity than either density-dependent or random processes (Vert-pre et al., 2013). Advances in recruitment process modeling also demonstrate the sensitivity of recruitment to oceanographic variability, including how environmental conditions affect larval food resources and mortality rates (Daewel et al., 2015).
In addition, there are (at least) two broad patterns in recruitment-environment relationships that could provide potential avenues for future forecasting attempts. First, some of the published relationships re-evaluated by Myers (1998) continued to be valid with updated data: those cases tended to be stocks located near limits of species ranges where recruitment processes for those stocks might be particularly sensitive to environmental variations (Myers, 1998). Subsequent updates of previously published relationships for stocks near range limits have also remained valid (Lindegren et al., 2010; Margonski et al., 2010; MacKenzie et al., 2012). Second, biogeographic patterns in the recruitment-environment (temperature) relationship for stocks throughout a species range indicate increases in recruitment for stocks located in cold regions and decreases for stocks in warm regions when temperatures rise (Brander, 2000; Mueter, 2002; MacKenzie and Köster, 2004; Mantzouni and Mackenzie, 2010). Both of these patterns demonstrate some persistent environmental impacts on recruitment and suggest that there may be forecast potential for recruitment in some stocks. Moreover, as recruitment process knowledge increases, this potential is likely to increase in the future.
The future sustainability of all fisheries depends on successful recruitment: clearly, a prolonged decline or downward shift in production of recruits will eventually erode the basis for a fishery and require fishery management actions to prevent local collapse or extinction. Multiple fishery management decisions and strategies such as short-term yield forecasts, fishery, and biomass reference points, recovery plans, and long-term climate change impacts on yields, therefore depend on assumptions (expectations) of future recruitment. Several stocks recognize this fact in their management, and while they do not forecast recruitment directly, exploitation is nevertheless adjusted according to the state of the environment: see (Skern-Mauritzen et al., 2016) for an overview of these stocks. The management of such stocks is therefore potentially amenable to forecast information: for example, the harvest guideline for Pacific sardine, already influenced by sea surface temperature (SST), has been shown to be more effective when informed by short-term recruitment forecasts driven by seasonal SST predictions (Tommasi et al., 2017b). Improvements in the reliability and timing of forecasts of recruitment could therefore have major impacts on different aspects of fishery management across the diverse spectrum of time scales and life-histories (Tommasi et al., 2017a).
While recruitment forecasts would be a key component in forecasting productivity changes, forecasted changes in growth or mortality rates would also be beneficial for fishery management. Changes in biological parameters depend on changes in the biota, not only the abiotic properties of the oceans. For example variations in growth, condition, fecundity, and recruitment can all be influenced by availability of prey and predators, how the prey and predators overlap in time and space (Neuenfeldt and Beyer, 2006) and the relative size distributions of the prey and predators (Golet et al., 2015). New operational forecast models that link the spatial distributions of interacting species (competitors, prey-predators) and use these distributions to forecast feeding, growth and mortality rates could thereby generate forecasts of productivity changes. Indeed, existing multispecies assessment models (e.g., ICES, 2017) contain all of these elements and could therefore be extended into the future to predict productivity changes. Such a step would represent a major scientific advance toward developing and implementing integrated ecosystem-based approaches to management.
In summary, developing productivity forecasts requires identification of the relevant scales and life-histories where predictive skill is needed and available. For example, a zooplankton abundance or a mean temperature averaged over a specific depth range in a specific place and time may be more relevant for larval fish mortality or juvenile growth rates than averaged over some other (e.g., larger) scale. Acquiring the scale-relevant knowledge will require process-oriented field, experimental, and modeling studies. However, recognizing and exploiting the (rare) situations where predictive skill is needed and available and linking them to fishery management systems may lead to valuable new marine ecological forecast products.
Within fisheries management, questions around the productivity (and thus quotas and sustainability) of a fish stock typically gather substantial attention and energy, both from the scientific community and the general public. However, the distribution of the resource in both time and space ultimately sets the framework within which fisheries operate and is thus a second question of critical importance to both fishers and managers.
The first wave of marine ecological forecast products to become fully operationalised has been strongly biased toward prediction of spatial distributions. Seasonal forecasts of the spatial distribution of southern bluefin tuna in the Great Australian Bight (Eveson et al., 2015) have been used to support the strategic planning of fisheries in this region for nearly a decade (Hobday et al., 2016) and were amongst the very first such forecast products to become operational. Distribution forecasts are also actively used in this region in a dynamic management context to close areas with the aim of avoiding by-catch (Hobday et al., 2011). In the United States, nowcasts of sea turtle distributions in the Pacific Ocean north of the Hawaiian Islands were amongst the first dynamic spatial management applications (Howell et al., 2008), and forecasts of the spatial distribution of sardines and blue whales in the California Current ecosystem have recently followed (Kaplan et al., 2016; Hazen et al., in press).
The relative success of spatial distribution forecasts most likely reflects a number of features that make this response variable well-suited to prediction. Spatial distribution studies typically have access to a relative wealth of observational data from scientific surveys, fisheries and other sources (e.g., Eveson et al., 2015 used tagging data) that allow relationships between the environment and the organism to be well-characterized. In contrast, recruitment studies are limited to one realization per year, with a typical time series being <30 years (Ricard et al., 2012). Furthermore, many living marine resources actively respond to environmental variability by shifting their distribution, either to avoid unfavorable conditions (e.g., temperatures that are too warm/cold) or to seek out optimal conditions (e.g., to reproduce or feed). In many cases, these constraints have a physiological basis (e.g., thermal or salinity tolerances) making for particularly robust and mechanistically-rooted links between the environment and the distribution. Finally, at least some, although not all, of the variables by which organisms sense and modulate their spatial distributions, such as temperature and salinity, are also the variables that are predicted directly by forecast systems, simplifying the “translation” from physics to biology tremendously.
Nevertheless, the ability to forecast spatial distributions is also subject to several important limitations. While we observe and are interested in distribution (where the organisms actually are), current forecasting systems are centered on the idea of habitat or ecological niche (where they could potentially be). However, these concepts are not interchangeable, and many processes (often outside of the modeling framework) govern the subset of potential habitats that are utilized and thereby yield the distribution (Dormann, 2007; Araújo and Peterson, 2012; Urban et al., 2016). For example, the dynamics of movement and migration can be important or even dominating: inter-annual shifts in the distribution of herring in the NE Atlantic of up to several thousand kilometers have been shown to be driven by the peculiarities of schooling dynamics (Huse et al., 2010). Similarly, two regions of space that are environmentally comparable may both offer suitable habitat, but one may be inaccessible due to the presence of environmental or physical barriers or extreme distances (Briscoe et al., 2017). Life-history dynamics are also critical to consider (Petitgas et al., 2013), as distributions at a given time point are strongly determined by both what has gone before and the need to close the life-cycle. Furthermore, not all of the variables that are potentially important in shaping distributions are currently measured or forecasted: the distributions of relevant predators, prey, and competitors are also critical (Elith and Leathwick, 2009). Correlative niche models using definitions of habitat based on the variables that we can observe and forecast are therefore inherently weakened by their narrow view of the processes shaping distribution (Warren, 2012, 2013; McInerny and Etienne, 2013).
Future research can help improve upon these early products. An important step in this direction is moving toward more mechanistic representations of distribution by increasing the biological realism of the models (Urban et al., 2016) e.g., explicitly incorporating movement and life-cycle with the limitations imposed by habitat. Suitable frameworks for this type of work already exist within the marine community (Lehodey et al., 2008; Ito et al., 2013) and operational applications are now being developed (Gehlen et al., 2015). Such a transition from empirical toward more mechanistic models would parallel the development pathway seen in terrestrial distribution modeling (Guisan and Zimmermann, 2000; Pearson et al., 2014).
Realizing the full potential of spatial distribution forecasts, will require close collaboration between developers and end-users. Spatial forecasts are already being used for dynamic spatial management (Hobday et al., 2011) based on close interaction with managers and fishers (Hobday et al., 2016). The use of forecast information to design monitoring programmes is also a particularly obvious application, as there are few barriers between the forecast developers and the scientists performing the surveys. However, the most important application will likely continue to be in direct collaborations with the fishing and shipping industries to forecast the distribution of both target and non-target/protected species. In these cases, where forecast users have an economic incentive to increase their effectiveness, a high degree of flexibility to use such information, and in some cases a legal imperative, it is not unreasonable to expect substantial growth in the number, and variety of spatial forecast products in the near future.
Physical forcing of ecosystem dynamics, particularly in high latitude environments, varies seasonally, and animal populations have adapted their phenology (i.e., the timing of life history events) to follow peaks in the seasonal cycles of physical drivers (e.g., temperature) and prey abundance. Variability in phenology affects the reproductive success of marine species (Hjort, 1914; Cushing, 1990; Platt et al., 2003; Durant et al., 2007) and influences their availability to fisheries.
Several examples of phenological forecasts now exist. For instance, the availability of lobsters to the Maine fishery varies between years following temperature-driven changes in their molting and inshore migration phenology (Mills et al., 2013). In 2012, during a marine heatwave, temperatures warmed 3 weeks earlier than normal, and lobster landings subsequently also increased sharply 3 weeks early, leading to a large influx of lobster, and a subsequent drop in price and economic challenges for the fishers (Mills et al., 2013). This event motivated the development of a forecast for the timing of the lobster fishery, which has been provided to the industry since 2015 to improve their operational planning and climate-readiness (Mills et al., in review). Timing forecasts of anadromous fish migration are also available (Anderson and Beer, 2009; Burke et al., 2013; Pacific Fishery Management Council, 2016). Other types of forecasts can also have a strong temporal element without being explicit forecasts of phenology, e.g., forecasts of seasonally-dependent high mortality conditions such as disease outbreaks (Maynard et al., 2016) or coral-bleaching risk (Liu et al., in review).
A successfully adopted ecological forecast is one that clearly addresses stakeholders' needs (Hobday et al., 2016). Phenological processes are non-linear, and subtle changes in climate drivers can lead to marked changes in the timing of management- and industry-relevant biological events. Since stakeholders are aware of and affected by the timing of certain ecological events at short time scales, phenological forecasts may be more easily adopted as compared to other types of ecological forecasts. On the other hand, because phenological events are discrete and non-linear, forecast errors are more evident. Binning the forecast into longer temporal bins (e.g., weekly instead of daily) and using probabilistic predictions can help reduce this problem (Mills et al., in review).
A forecast also needs to be skilful in order to be considered successful (Murphy, 1993). Seasonal forecasts of SST have skill in some regions at the coastal scales relevant to stakeholders' needs (Stock et al., 2015), but seasonal prediction skill of other phenologically-relevant physical variables (e.g., onset of upwelling) has not been adequately assessed (Tommasi et al., 2017a). Furthermore, biogeochemical forecasts (e.g., nutrients, chlorophyll, primary production) are still experimental but show some potential (Séférian et al., 2014; Li et al., 2016; Siedlecki et al., 2016). Thus, phenological forecasts that depend on temperature-driven physiological mechanisms (e.g., Liu et al., in review) may more quickly be operationalized than those requiring predictions of prey conditions.
Another challenge in the development of phenological forecasts is the limited availability of long time series of high-temporal-resolution data needed to quantify phenological changes. Integration of phenological forecasts into fisheries opening/closure decisions, survey planning, coastal management, or industry operations will require maintenance and expansion of observing systems for continuous-high resolution climate and biological data. Ensuring spatial constancy is also critical in this context, to allow spatial and temporal shifts to be separated from each other (de Keyzer et al., 2017). In addition, an improved process understanding of the drivers of phenological changes, assessment of physical prediction skill with a phenology focus (i.e., timing of specific events), and development of seasonal biogeochemical forecast capabilities will all represent valuable advances.
While our survey of existing marine ecological forecast products revealed examples of forecasts of species distribution, productivity, and phenology, it did not reveal any examples of forecasts of the human part of the system. However, without an understanding of the human responses to changes in a system, unanticipated consequences can occur, such as shifts in fishing effort following declaration of marine protected areas (e.g., Ward et al., 2001; Abbott and Haynie, 2012), concentration of fishing effort in particular areas (e.g., Parnell et al., 2010), changes in illegal behavior (Österblom and Bodin, 2012), and quota shifts (Emery et al., 2014). Here we examine the potential for developing such forecasts.
Unfortunately, even defining the human component is challenging, which partly explains why exploration of future human behaviors and responses has received considerably less attention than biological responses. The human part of the marine system may include direct participants in marine activities (e.g., fishers, tourists), downstream participants (e.g., fish processors), resource managers, and policy makers. The human system may also include the economic market (e.g., Mullon et al., 2017), or non-consumptive actors (Sanchirico et al., 2013; e.g., Tracey et al., 2013). Defining the relevant human sub-system is the first challenge.
Including humans is seen as an important challenge when modeling marine systems (e.g., Fulton, 2010), and this will increase further in importance when forecasting ecosystem changes. Managers of marine systems may be interested in a range of issues that involve humans, including trade-offs in ecosystem-based fisheries management (EBFM) as a result of environmental change, planning for infrastructure (e.g., in locations where fish are projected to move, fisheries and the necessary infrastructure will need to adjust), and minimizing unanticipated consequences (e.g., that can result from ignoring the people). Social and economic sustainability are now seen as central goals alongside ecological sustainability, and forecasts may provide valuable new insights into how ecosystem changes and management actions will differentially affect multiple objectives (Fulton et al., 2014; Jennings et al., 2016).
In constructing models of human systems, there are several advantages compared to the “biological” part of the system. Humans can talk and be observed (e.g., via observer and vessel monitoring systems), they are relatively easy to sample and census, and extensive historical data are often available (e.g., price, employment, catch, effort) to condition and test models. At times control groups of vessels or fleets are available that allow controlled studies to be performed (e.g., Essington, 2010; Abbott et al., 2015). On the negative side, humans can be deliberately or accidently unreliable or strategic in describing their behavior. They may have preferences which are not revealed until a situation actually occurs, particularly for problems that result from a combination of physics (ocean change), biology (dynamic species responses), and humans (behavioral responses). As a result, there are considerable challenges in building mathematical descriptions of human behavior, and gathering information on people in a systematic way such that it can be included in marine models (Fulton, 2010). For example, in a study of the eastern Bering Sea ecosystem and the response of extensive commercial fishery and subsistence harvests, Haynie and Huntington (2016) found the influence of ecosystem conditions on the outcomes of human activities was weaker than anticipated. They attributed this loose coupling to the ability of fishers and hunters to adjust to variable conditions, and the role of social systems, the market economy, and management in moderating the direct effects of changes in the ecosystem.
Development of predictive models of human behavior has thus been considered challenging, with marine examples to date consisting mostly of models that characterize fleet behavior (e.g., Eales and Wilen, 1986; Michael et al., 2017) or that assess outcomes of conservation or resource management decisions (Fulton et al., 2015). These modeling tools allow a range of options to be explored, and support evaluation of alternative interventions under differing conditions. Model results can be presented at multiple spatial and temporal scales, and relative to ecological, economic, and social objectives. Results can also reveal potential “surprises”, such as bottlenecks in human responses (Fulton et al., 2015). Predicting how fleets will respond to changes in management such as catch shares is particularly challenging, as the observed behavior is a response to environmental, market, and management conditions and changes (e.g., Abbott et al., 2015; Reimer et al., 2017).
While marine ecological forecasting is clearly still a field in its infancy, it is also extremely diverse in the range of issues considered. Although each of the response variables and associated forecast products described has their own strengths and weaknesses, there are also clear trends and commonalities between them. Here we synthesize these lessons with the aim of highlighting the way forward on a broad scale.
Firstly, it is important to remember that it may not be necessary to invoke the complex machinery of a fully-coupled climate model to produce useful forecasts. Many physical, biological and social systems have inherent lags that can be exploited to produce useful forecasts based on observed (rather than forecasted) environmental conditions, an approach used by the Gulf of Maine lobster and Pacific salmon return forecasts (Table 1). Similarly, the influence of environmental factors on year-class strength of a fish stock typically occurs very early in life (Hjort, 1914; Houde, 2008) but in many cases it can take several years before these individuals become important for the fishery. Fraser River salmon forecasts and Gulf of Maine lobster timing have both used this approach to give appreciable forecast horizons without the need for a climate model.
Similarly, the long-term memory of the ocean and its slow dynamics can also be exploited. Statistical forecasts of the physical environment can be produced by assuming persistence of either absolute values, of anomalies or of a trend (e.g., climate warming) into the future. For example, in the North Sea, anomaly persistence forecasts of SST readily give appreciable skill at a one-year lead time (Stock et al., 2015). This approach is implicit in several of the examples noted previously, where exploitation of the fishery is adjusted to the local environmental regime e.g., Pacific sardine management. While such forecasts may not have the elegance or technical bravado of applying a fully coupled climate model, the important question from an end-user's perspective is the presence (or absence) of useful skill, rather than how the forecast is generated.
Where such simple approaches are insufficient and fully coupled climate models are required, a good place to start is by focusing on the predictability of major modes of variability in the ocean, and their biological consequences. Much of the skill of seasonal and, in part, multi-year forecasts at management-relevant local scales arises from the ability of the forecast systems to capture the evolution of predictable basin-scale patterns (e.g., associated with the El Niño-Southern Oscillation, ENSO) and their regional imprints (Goddard et al., 2001; Stock et al., 2015). Thus, some of these types of events may be more predictable than average conditions. For example, in the California Current, skill of seasonal SST predictions is higher during El Niño events (Jacox et al., 2016). This is beneficial for fisheries managers and industry stakeholders, as El Niño events can have dramatic impacts on the California Current ecosystem (Chavez et al., 2002; Jacox et al., 2016). Similarly, there is evidence that the strong and abrupt contraction of the North Atlantic sub-polar gyre in the mid-1990s could have been foreseen with a sub-decadal lead time (Yeager et al., 2012; Wouters et al., 2013; Msadek et al., 2014). These oceanographic changes also have had well-documented effects on the ecosystems of the North Atlantic (Hátún et al., 2009a,b). In both cases, a valuable first step may be to build simple conditional ecological forecast systems (so-called “if-then” forecasts or “forecasts by analogy”) whereby a list of ecosystem responses seen in prior analogous events can be produced: a forecast need not be quantitative in nature to be valuable.
While these simplistic approaches can quickly yield useful forecasts in some situations, the most comprehensive results will ultimately require coupling of biological models to physical forecast systems. In developing such forecast products, it is tempting to focus on a specific response variable or species, driven by the nexus of commercial and academic interests, together with available funding. However, there is no guarantee that such an approach will lead to forecast systems that are both skilful and useful. Instead, we propose that the question should be reversed: rather than asking “how do we predict a particular variable”, it is useful to consider asking “what can we predict?” We propose three concepts that we believe can be valuable in identifying systems and variables that can be predicted skilfully.
Firstly, mechanistic understanding, where available, is clearly favored over empirically derived models when building forecasts (Levins, 1966; Urban et al., 2016). This is not to say that useful forecasts cannot be built upon empirical knowledge: indeed nearly all of the products highlighted here are based on correlations between physical variables in the ocean and biological responses. However, mechanistic knowledge is generally regarded as providing a strong footing for forecasting both biological (Guisan and Zimmermann, 2000; Dickey-Collas et al., 2014) and economic (e.g., Haynie and Pfeiffer, 2012) aspects of marine systems, particularly in cases where extrapolation beyond the range of conditions seen in the training set (e.g., under climate change) is required. Nevertheless, it is important to note that there is often little choice but to employ correlative approaches: while the difficulties of predicting fish-stock recruitment based on empirical relationships with the environment have long been recognized (e.g., Myers, 1998), skilful mechanistic solutions to this problem still appear far off.
Secondly, it is necessary to focus on developing biological forecast products around physical variables that can themselves be predicted. A forecast system based on SST, which can readily be predicted on seasonal and even decadal time scales in many marine ecosystems (Kirtman et al., 2014; Meehl et al., 2014; Stock et al., 2015), is much more likely to yield success than one that requires estimates of food abundance, which at the moment cannot be forecasted skilfully. Similarly, it is important to recognize that the spatial forecast skill of a given variable differs across space (Figure 2) and across time scales (Figure 3). For example, while there is multi-annual SST forecast skill in the North Atlantic (Matei et al., 2012b; Meehl et al., 2014), prediction skill at a multi-annual scale is low over the Pacific Ocean (Figure 3). This contrasts with the seasonal scale, where ENSO provides high seasonal forecast skill to the eastern tropical Pacific Ocean (Figure 2). A full assessment of the predictability of the physical-system at biologically-relevant spatial and temporal scales (Stock et al., 2015) could eliminate areas where forecasts are not possible and is therefore a critical first step in narrowing down the range of skilfully-predictable biological quantities.
Figure 2. Seasonal-scale sea surface temperature (SST) forecast skill. Skill is shown here for the North American Multi-model Ensemble (NMME) as the correlation coefficient (as a percentage) between observed and forecast seasonal anomalies for a 1 month forecast lead time. Correlation coefficients are shown for temperature anomalies averaged over each of four seasons. Top-left: December, January, February (DJF). Top-right: March, April, May (MAM). Bottom-left: June, July, August (JJA). Bottom-right: September, October, November (SON). Modified from (Becker et al., 2014). © 2014 American Meteorological Society. Used with permission.
Figure 3. Decadal-scale sea surface temperature (SST) forecast skill. Skill is shown as the temperature predictability beyond the global warming trend for the ECHAM5/Max Planck Institute Ocean Model (MPI-OM) coupled model with initial conditions provided by the NCEP reanalysis. Correlation coefficients between linearly-detrended observations and forecasts are shown for forecast lead times of (a) 2–5 years and (b) 6–10 years. Only the significant correlations (at the 5% level) are plotted. Modified from (Matei et al., 2012b). © 2012 American Meteorological Society. Used with permission.
Thirdly, researchers should focus on biological responses where there is a high proximity between the biological response and the physical driver, i.e., tight cause-and-effect relationships. For example, a large body of knowledge exists about correlations between large-scale climate indices, such as the North Atlantic Oscillation (NAO) and Atlantic Multidecadal Oscillation (AMO), and biological responses (Ottersen et al., 2001; Stenseth et al., 2003; Alheit et al., 2014; Nye et al., 2014). However, while these indices can potentially be well-predicted by coupled forecast systems (García-Serrano et al., 2012), it seems unlikely that they can be used to build skilful predictive systems of the marine environment due to the large number of steps between the predictor and the response, each of which adds noise to the predictive process. Similarly, biological responses to physical processes that are filtered through multiple processes and trophic levels (e.g., from upwelling to nutrient concentration to primary productivity to secondary productivity to survival of juvenile fish) seem less likely to yield skilful predictions than situations where there is a direct response to the physical driver (e.g., egg mortality due to low salinity, avoidance of waters that are too cold).
However, and most importantly, while these three concepts can help focus on identifying “low-hanging fruit” that it may be possible to predict skilfully, there is no guarantee that these quantities will be useful to end-users. The ultimate value and success of a forecast is determined by whether it is (actively) used by end-users in their decision making process and whether its use results in economic or other benefits (Murphy, 1993). Active engagement with the end-users of the predictions from the very start of the project to co-develop forecast products is therefore key to ensure that their potential value to the end-user is realized (Hobday et al., 2016). While this may necessarily entail a deviation from the approach given above, worsen the forecast skill, or complicate the development process, a forecast product without an end-user is ultimately a waste of effort. Striking the balance between what is feasible and what is useful will therefore be essential to push marine ecological forecasting forward in the future.
While the forecast products highlighted here have successfully shown that forecasting of marine ecological variables is possible, realizing the full potential of this field will require more than just coupling existing biological or socio-economic knowledge to existing climate forecast systems. Developing the next generation of forecast products that moves beyond these initial proof-of-concept examples necessitates further developments in both research and management, as we discuss here.
Perhaps the greatest factor limiting the development of marine ecological forecast products is the gap between the climate modeling and marine science communities. This gap is multidimensional in its nature. Climate modelers and marine scientists, for example, are rarely employed at the same institute and have very different educations and ways of thinking and working. The nature of climate model output is a particularly challenging aspect of this disjoint; while climate scientists routinely work with extremely large data sets on large computing clusters, many analyses and datasets in marine science have historically not required the use of a formal programming language and are stored in a spreadsheet (Berx et al., 2011). Bridging this gap is challenging, and the main linkage between the two disciplines is currently in the form of relatively few individuals that are capable of moving between them. Fortunately, climate-model and climate-data literacy can be learned. The development of training courses to increase the proficiency of both marine biological researchers and students in the use and application of climate-model data should therefore be seen as a simple but high priority action to help bring these two fields closer together. Similarly, the climate data needs in marine science can be better communicated to climate scientists so they can provide output that facilitates the uptake of climate information into marine ecological forecasts. Overall, large projects that bring together researchers across disciplines for sustained periods of time should be encouraged as they facilitate the exchange of expertise in both directions (e.g., Van Pelt et al., 2016).
A second key factor limiting the development and eventual uptake of climate-model forecasts in fisheries and marine science, even for those that are adept with climate-data, is access to forecast data. Getting access to such data for marine scientists typically requires establishing close collaborations with climate modelers directly: on the one hand, this brings new and critical expertise into the project, but on the other hand is not an option available to all. Availability of climate-forecast data on both the seasonal and decadal timescales, including both hindcasts and routine delivery of updated forecasts, is nevertheless critical for the continued development and production of marine ecological forecast products. Fortunately, this problem has been well-recognized for some time now in the climate-modeling community and initiatives aimed at improving access (e.g., the WCRP Grand Challenge on Near Term Climate Predictions) are starting to deliver results. Seasonal scale forecasts are available from the North American Multi-Model Ensemble (NMME: Kirtman et al., 2014) and analogous projects are currently in progress in Europe (e.g., Copernicus C3S). Some decadal forecast products are also openly available e.g., via CMIP5 (IPCC, 2013) and can be used to develop products, although given that most of the models stop around 2005, these cannot be used to produce regularly updated predictions.
From a biological point of view, there is a continuing need to improve the quality of our biological models. Marine science has been limited for many years by its focus on describing, rather than predicting, systems. Expanding our knowledge beyond the empirical toward the mechanistic can be expected to greatly improve the quality of our understanding and our predictive capability (Dickey-Collas et al., 2014; Urban et al., 2016). Incorporating behavior, allowing for adaptive responses, and modeling organisms in terms of their full life-cycle are all key elements that can be expected to be seen in the next generation of models and deliver gains in predictive skill, challenges in parameterising such models notwithstanding (Urban et al., 2016). Similarly, the importance of social science in understanding the marine system is gaining increasing recognition and can be expected to drive important modeling developments in the future as well as to inform the features that make a management system effective (Fulton, 2010; Bundy et al., 2017).
For economics and social sciences, modeling, and data collection are expanding significantly but the integration of these models with biophysical models is nascent. Economic models are both structural and empirical, but even when predictions can be made about how fish populations and market conditions will change, the ability to predict what will occur across large policy changes that alter fishers' incentives is limited (Reimer et al., 2017). However, as more research is conducted and the lessons learned integrated across management systems, better assumptions will be able to be made about the combined impacts of environmental, market, and policy variability and change.
Improvements in applying this knowledge are also needed to take advantage of the potential offered by marine ecological forecasts. Foremost amongst this is the need to further develop frameworks to assess and quantify the value of forecast knowledge. Potential applications of forecast information could be run through a simulation procedure, similar to a Management Strategy Evaluation (MSE) to quantify both the benefits and risks associated with the forecast product. Importantly, the level of forecast skill required of the forecast product to “break-even” (i.e., where the benefits outweigh the risks) can be established within such a framework and used to determine when the procedure should be adopted, modified or potentially rejected. Examples of such analyses can be found in the literature already (e.g., Basson, 1999) and at least one example of the analysis of a forecast system, for the management of the Pacific sardine fishery, has emerged (Tommasi et al., 2017b).
Finally, the role of stakeholders in the development of these forecast products is often easy to overlook, but is also critical (Hobday et al., 2016). Stakeholder participation is crucial at all phases of developing forecast products, and should be involved all the way from the scoping of the project through its development to its evaluation and into operational delivery (e.g., Liu et al., in review). Support to train stakeholders on how to interpret and use this forecast information is therefore also essential: engaged and informed stakeholders will ultimately both inspire the development of new forecast products that the scientific community cannot foresee and ensure their success. Efforts to increase and support stakeholder engagement are therefore expected to yield large dividends.
This review of the current state of the marine ecological forecast products shows a field that is developing quickly. Within the last 5 years, many products have come online and are now being produced in an operational manner, with many more still under development. A number of these products are quite mature, and have been in operation for close to a decade: it therefore seems safe to say that the field has moved beyond the “proof-of-concept” phase and is now preparing to roll-out the technology on a much larger scale.
Realizing the full potential offered by marine ecological forecasting can, however, seem like a daunting task, currently available forecast products notwithstanding. The underlying complexity of biological systems and the difficultly of observing life in the ocean are particularly challenging obstacles. Nevertheless, it is worth remembering the tremendous progress that has been made in other fields: meteorology, in particular, makes for a particularly inspirational example. Starting from the early numerical experiments of Lorenz in the 1950s, weather forecasting has progressed steadily and surely to the point where reliable 5 and 7 day forecasts are now a reality (Figure 4), a situation that was pure fantasy just a few decades ago (Bauer et al., 2015). These advances have occurred in spite of challenging observational difficulties and the inherent complexity and chaotic nature of weather systems. Marine biological science faces many similar problems today. While it is tempting to despair of the complexity of ecosystems, the lessons from the history of numerical weather prediction teach us that useful products can be developed in the face of complexity.
Figure 4. Development of weather forecast skill since 1981 for 3, 5, 7, and 10 day lead times for the northern (NH) and southern hemispheres (SH). Forecasts are regarded as being useful if they exceed a skill of 60%, while >80% is considered to be of high accuracy. Forecast skill is the correlation between the forecasts and the verifying analysis of the height of the 500-hPa level, expressed as the anomaly with respect to the climatological height. The collapse of the curves in the late 1990s is due to advances in incorporating satellite observations into forecast models. Reprinted by permission from Macmillan Publishers Ltd, Nature (Bauer et al., 2015) copyright 2015.
The pathway followed by marine ecological forecasting, however, will likely be different from the incremental progress of numerical weather prediction (Figure 4). We expect the next few years to exhibit a form of “Cambrian explosion” in the number and variety of such forecast products, as marine researchers become aware of the potential of forecasting and start to populate the “niche.” We expect that this second generation of products will be dominated by direct use of the available model outputs: this is the approach advocated in the section “How to go forward.” However, once the initial “low-hanging fruit” products have come online, progress can be expected to be more incremental in nature, as the joint development of forecast systems between physical modelers and marine scientists starts to address the more challenging problems; indeed, such collaborations are already starting to emerge e.g., the J-SCOPE forecasting system in the California Current (Kaplan et al., 2016; Siedlecki et al., 2016).
The marine ecological forecast products that have emerged thus far are by no means distributed uniformly across the globe, or even across the developed world. While Australia has clearly been the pioneer, with multiple mature operational products, and the United States has also seen rapid development of operational products within the last few years, Europe has yet to see the first such products emerge. Counterintuitively, the waters surrounding Europe have some of the longest forecast horizons in the world (Figure 3), particularly in the North Atlantic sub-polar gyre region where decadal-scale forecasts are a reality (Matei et al., 2012b; Meehl et al., 2014), but also in its shelf seas (Stock et al., 2015). Moreover, many of its seas have a long history of scientific investigation and some of the key hypotheses about fish stock productivity were inspired by variations in European stocks (Hjort, 1914; Paasche et al., 2015). We postulate two potential explanations for this discrepancy. Firstly, while local fisheries in Australia and the USA essentially are governed by one national agency, management of European fisheries needs to balance the interests of many nations at the same time, and therefore may be less flexible and slower to respond to new opportunities. Secondly, both Australia and the USA border the Pacific Ocean and their waters are influenced by strong and regular variability in the form of both ENSO and the Pacific Decadal Oscillation (PDO). Furthermore, many of the first seasonal forecasts of the ocean (for ENSO) were developed in this area more than a decade ago, while the dominate mode of variability in the North Atlantic (the NAO) has until recently been thought to be unpredictable (Scaife et al., 2014; Smith et al., 2016), again giving the Pacific a natural advantage in this respect. Nevertheless, the high predictability of the front doorstep of Europe represents a tremendous potential that we expect to see tapped in the future.
As marine forecasts become more common, we can also expect to see both dramatic failures and unintended consequences emerge. These negative outcomes may result if a forecast fails in a technical sense, but they will also arise if forecasts are not used or interpreted properly. As the technical potential for forecasting in marine systems becomes more tangible, the need is rising for systematic processes to engage end-users in designing forecasts that can effectively support their specific decision-making requirements. In addition, information that is understandable to stakeholders about what the forecast provides, temporal and spatial scales at which it is relevant, its associated levels of uncertainty and its limitations is also critical. In many cases, successful use of a forecast will require that stakeholders beyond the immediate users are capable of interpreting the forecast correctly, so as not to disrupt highly connected systems in which actors may have competing interests, such as fishery governance systems and seafood supply chains. Active engagement with end-users to communicate the limitations and assumptions inherent in these forecasts is therefore critical to minimize the problems created when forecasts inevitably fail or are misused.
In conclusion, we have reviewed and highlighted the lessons learned so far from this first generation of forecast products. While the recommendations made here will not guarantee the successful development of other popular prediction systems in the future, they can nevertheless be used to increase the rate of development, identify “low-hanging fruits” where there is a good chance of developing forecast systems and ensure the relevance of the products to end-users. Following such an approach will, we believe, lead to a rapid blooming of forecast products that can close the gap between the potential and the reality of marine ecological forecasting.
All authors contributed to the initial discussions shaping this manuscript. MRP, AJH, BRM, and DT lead the drafting of the manuscript. All authors contributed to the revision of the manuscript and approved the final version.
The authors declare that the research was conducted in the absence of any commercial or financial relationships that could be construed as a potential conflict of interest.
This manuscript stems from the discussions held as part of Theme Session I on “Seasonal-to-decadal prediction of marine ecosystems: opportunities, approaches, and applications” held as part of the ICES Annual Science conference in Riga, Latvia, 19–23 September 2016 and co-sponsored by PICES. The authors would like to recognize and thank all those that participated in the theme session for the lively discussions and insightful comments that formed the foundation of this work. The authors would also like to thank two reviewers whose critical and insightful comments helped improve this manuscript at the review stage. The research leading to these results has received funding from the European Union 7th Framework Programme (FP7 2007–2013) under grant agreement number 308299 (NACLIM) and the Horizon 2020 research and innovation programme under grant agreement number 727852 (Blue-Action). BRM was also supported in part by the COFASP ERA-NET partners, which have received funding from the European Union's Seventh Framework Programme for research, technological development and demonstration under grant agreement no. 321553 (GOFORIT project), and by the national funding agency of Denmark (Innovationsfonden). KEM was supported by the U.S. National Aeronautics and Space Administration through grant EP-15-03 to the Maine Space Grant Consortium, of which the Gulf of Maine Research Institute is an affiliate. KOS was supported by the Research Council of Norway grant RETROSPECT, project number 244262. This work was also supported by the German BMBF project RACE (DM, FKZ:03F0729D).
Abbott, J. K., and Haynie, A. C. (2012). What are we protecting? Fisher behavior and the unintended consequences of spatial closures as a fishery management tool. Ecol. Appl. 22, 762–777. doi: 10.1890/11-1319.1
Abbott, J. K., Haynie, A. C., and Reimer, M. N. (2015). Hidden flexibility: institutions, incentives, and the margins of selectivity in fishing. Land Econ. 91, 169–195. doi: 10.3368/le.91.1.169
Alheit, J., Licandro, P., Coombs, S., Garcia, A., Giráldez, A., Santamaría, M. T. G., et al. (2014). Atlantic Multidecadal Oscillation (AMO) modulates dynamics of small pelagic fishes and ecosystem regime shifts in the eastern North and Central Atlantic. J. Mar. Syst. 131, 21–35. doi: 10.1016/j.jmarsys.2013.11.002
Anderson, J. J., and Beer, W. N. (2009). Oceanic, riverine, and genetic influences on spring chinook salmon migration timing. Ecol. Appl. 19, 1989–2003. doi: 10.1890/08-0477.1
Araújo, M. B., and Peterson, A. T. (2012). Uses and misuses of bioclimatic envelope modeling. Ecology 93, 1527–1539. doi: 10.1890/11-1930.1
Basson, M. (1999). The importance of environmental factors in the design of management procedures. ICES J. Mar. Sci. 56, 933–942. doi: 10.1006/jmsc.1999.0541
Bauer, P., Thorpe, A., and Brunet, G. (2015). The quiet revolution of numerical weather prediction. Nature 525, 47–55. doi: 10.1038/nature14956
Becker, E., den Dool, H., van Zhang, Q., Van den Dool, H., and Zhang, Q. (2014). Predictability and forecast skill in NMME. J. Clim. 27, 5891–5906. doi: 10.1175/JCLI-D-13-00597.1
Berx, B., Dickey-Collas, M., Skogen, M., De Roeck, Y.-H., Klein, H., Barciela, R., et al. (2011). Does operational oceanography address the needs of fisheries and applied environmental scientists? Oceanography 24, 166–171. doi: 10.5670/oceanog.2011.14
Brander, K. M. (2000). Effects of environmental variability on growth and recruitment in cod (Gadus morhua) using a comparative approach. Oceanol. Acta 23, 485–496. doi: 10.1016/S0399-1784(00)00133-X
Brander, K. M. (2003). What kinds of fish stock predictions do we need and what kinds of information will help us to make better predictions? Sci. Mar. 67, 21–33. doi: 10.3989/scimar.2003.67s121
Briscoe, D. K., Hobday, A. J., Carlisle, A., Scales, K., Eveson, J. P., Arrizabalaga, H., et al. (2017). Ecological bridges and barriers in pelagic ecosystems. Deep Sea Res. II, Top. Stud. Oceanogr. 140, 182–192. doi: 10.1016/j.dsr2.2016.11.004
Bundy, A., Chuenpagdee, R., Boldt, J. L., de Fatima Borges, M., Camara, M. L., Coll, M., et al. (2017). Strong fisheries management and governance positively impact ecosystem status. Fish Fish. 18, 412–439. doi: 10.1111/faf.12184
Burke, B. J., Peterson, W. T., Beckman, B. R., Morgan, C., Daly, E. A., and Litz, M. (2013). Multivariate models of adult pacific salmon returns. PLoS ONE 8:e54134. doi: 10.1371/journal.pone.0054134
Chavez, F., Pennington, J., Castro, C., Ryan, J., Michisaki, R., Schlining, B., et al. (2002). Biological and chemical consequences of the 1997–1998 El Niño in central California waters. Prog. Oceanogr. 54, 205–232. doi: 10.1016/S0079-6611(02)00050-2
Constantin de Magny, G., Long, W., Brown, C. W., Hood, R. R., Huq, A., Murtugudde, R., et al. (2009). Predicting the distribution of Vibrio spp. in the Chesapeake Bay: a vibrio cholerae Case Study. EcoHealth 6, 378–389. doi: 10.1007/s10393-009-0273-6
Corti, S., Weisheimer, A., Palmer, T. N., Doblas-Reyes, F. J., and Magnusson, L. (2012). Reliability of decadal predictions. Geophys. Res. Lett. 39:L21712. doi: 10.1029/2012GL053354
Cushing, D. H. (1990). Plankton production and year-class strength in fish populations: an update of the match/mismatch hypothesis. Adv. Mar. Biol. 26, 249–293. doi: 10.1016/S0065-2881(08)60202-3
Daewel, U., Schrum, C., and Gupta, A. (2015). The predictive potential of early life stage individual-based models (IBMs): an example for Atlantic cod Gadus morhua in the North Sea. Mar. Ecol. Prog. Ser. 534, 199–219. doi: 10.3354/meps11367
de Keyzer, C. W., Rafferty, N. E., Inouye, D. W., and Thomson, J. D. (2017). Confounding effects of spatial variation on shifts in phenology. Glob. Chang. Biol. 23, 1783–1791. doi: 10.1111/gcb.13472
Dickey-Collas, M., Payne, M. R., Trenkel, V. M., and Nash, R. D. M. (2014). Hazard warning: model misuse ahead. ICES J. Mar. Sci. 71, 2300–2306. doi: 10.1093/icesjms/fst215
Doblas-Reyes, F. J., Balmaseda, M. A., Weisheimer, A., and Palmer, T. N. (2011). Decadal climate prediction with the European centre for medium-range weather forecasts coupled forecast system: impact of ocean observations. J. Geophys. Res. 116:D19111. doi: 10.1029/2010JD015394
Dormann, C. F. (2007). Promising the future? Global change projections of species distributions. Basic Appl. Ecol. 8, 387–397. doi: 10.1016/j.baae.2006.11.001
Drinkwater, K. F., Beaugrand, G., Kaeriyama, M., Kim, S., Ottersen, G., Perry, R. I., et al. (2010). On the processes linking climate to ecosystem changes. J. Mar. Syst. 79, 374–388. doi: 10.1016/j.jmarsys.2008.12.014
Durant, J. J. M., Hjermann, D. D. Ø., Res, C., Ottersen, G., and Stenseth, N. C. (2007). Climate and the match or mismatch between predator requirements and resource availability. Clim. Res. 33, 271–283. doi: 10.3354/cr033271
Eales, J., and Wilen, J. E. (1986). An examination of fishing location choice in the pink shrimp fishery. Mar. Res. Econ. 2, 331–351. doi: 10.1086/mre.2.4.42628909
Elith, J., and Leathwick, J. R. (2009). Species distribution models: ecological explanation and prediction across space and time. Annu. Rev. Ecol. Evol. Syst. 40, 677–697. doi: 10.1146/annurev.ecolsys.110308.120159
Emery, T. J., Hartmann, K., Green, B. S., Gardner, C., and Tisdell, J. (2014). Fishing for revenue: how leasing quota can be hazardous to your health. ICES J. Mar. Sci. 71, 1854–1865. doi: 10.1093/icesjms/fsu019
Essington, T. E. (2010). Ecological indicators display reduced variation in North American catch share fisheries. Proc. Natl. Acad. Sci. U.S.A. 107, 754–759. doi: 10.1073/pnas.0907252107
Eveson, J. P., Hobday, A. J., Hartog, J. R., Spillman, C. M., and Rough, K. M. (2015). Seasonal forecasting of tuna habitat in the great Australian bight. Fish. Res. 170, 39–49. doi: 10.1016/j.fishres.2015.05.008
Fulton, E. A., Smith, A. D. M., Smith, D. C., and Johnson, P. (2014). An integrated approach is needed for ecosystem based fisheries management: insights from ecosystem-level management strategy evaluation. PLoS ONE 9:e84242. doi: 10.1371/journal.pone.0084242
Fulton, E. A., Bax, N. J., Bustamante, R. H., Dambacher, J. M., Dichmont, C., Dunstan, P. K., et al. (2015). Modelling marine protected areas: insights and hurdles. Philos. Trans. R. Soc. B 370:20140278. doi: 10.1098/rstb.2014.0278
Fulton, E. A. (2010). Approaches to end-to-end ecosystem models. J. Mar. Syst. 81, 171–183. doi: 10.1016/j.jmarsys.2009.12.012
García-Serrano, J., Doblas-Reyes, F. J., and Coelho, C. A. S. (2012). Understanding Atlantic multi-decadal variability prediction skill. Geophys. Res. Lett. 39, 1–6. doi: 10.1029/2012GL053283
Gehlen, M., Barciela, R., Bertino, L., Brasseur, P., Butenschön, M., Chai, F., et al. (2015). Building the capacity for forecasting marine biogeochemistry and ecosystems: recent advances and future developments. J. Oper. Oceanogr. 8, s168–s187. doi: 10.1080/1755876X.2015.1022350
Goddard, L., Mason, S. J., Zebiak, S. E., Ropelewski, C. F., Basher, R., and Cane, M. A. (2001). Current approaches to seasonal to interannual climate predictions. Int. J. Climatol. 21, 1111–1152. doi: 10.1002/joc.636
Golet, W. J., Record, N. R., Lehuta, S., Lutcavage, M., Galuardi, B., Cooper, A. B., et al. (2015). The paradox of the pelagics: why bluefin tuna can go hungry in a sea of plenty. Mar. Ecol. Prog. Ser. 527, 181–192. doi: 10.3354/meps11260
Guisan, A., and Zimmermann, N. E. (2000). Predictive habitat distribution models in ecology. Ecol. Modell. 135, 147–186. doi: 10.1016/S0304-3800(00)00354-9
Hare, J. A. (2014). The future of fisheries oceanography lies in the pursuit of multiple hypotheses. ICES J. Mar. Sci. 71, 2343–2356. doi: 10.1093/icesjms/fsu018
Hátún, H., Payne, M. R., and Jacobsen, J. A. (2009a). The North Atlantic subpolar gyre regulates the spawning distribution of blue whiting (Micromesistius poutassou). Can. J. Fish. Aquat. Sci. 66, 759–770. doi: 10.1139/F09-037
Hátún, H., Payne, M. R., Beaugrand, G., Reid, P. C., Sandø, A. B., Drange, H., et al. (2009b). Large bio-geographical shifts in the north-eastern Atlantic Ocean: from the subpolar gyre, via plankton, to blue whiting and pilot whales. Prog. Oceanogr. 80, 149–162. doi: 10.1016/j.pocean.2009.03.001
Haynie, A. C., and Huntington, H. P. (2016). Strong connections, loose coupling: the influence of the Bering Sea ecosystem on commercial fisheries and subsistence harvests in Alaska. Ecol. Soc. 21, art6. doi: 10.5751/ES-08729-210406
Haynie, A. C., and Pfeiffer, L. (2012). Why economics matters for understanding the effects of climate change on fisheries. ICES J. Mar. Sci. 69, 1160–1167. doi: 10.1093/icesjms/fss021
Hazen, E. L., Palacios, D. M., Forney, K. A., Howell, E. A., Becker, E., Hoover, A. L., et al. (in press). WhaleWatch: a dynamic management tool for predicting blue whale density in the California Current. J. Appl. Ecol. doi: 10.1111/1365-2664.12820
Hjort, J. (1914). Fluctuations in the Great Fisheries of Northern Europe Viewed in Light of Biological Research. Rapports et Procés-Verbaux des Réunions de Conseil International pour l'Exploration de la Mer, 20, 1–228.
Hobday, A. J., Hartog, J. R., Spillman, C. M., Alves, O., and Hilborn, R. (2011). Seasonal forecasting of tuna habitat for dynamic spatial management. Can. J. Fish. Aquat. Sci. 68, 898–911. doi: 10.1139/f2011-031
Hobday, A. J., Spillman, C. M., Paige Eveson, J., and Hartog, J. R. (2016). Seasonal forecasting for decision support in marine fisheries and aquaculture. Fish. Oceanogr. 25, 45–56. doi: 10.1111/fog.12083
Hollowed, A. B., Barange, M., Beamish, R. J., Brander, K. M., Cochrane, K., Drinkwater, K. F., et al. (2013). Projected impacts of climate change on marine fish and fisheries. ICES J. Mar. Sci. 70, 1023–1037. doi: 10.1093/icesjms/fst081
Houde, E. D. (2008). Emerging from Hjort's shadow. J. Northwest Atlant. Fish. Sci. 41, 53–70. doi: 10.2960/J.v41.m634
Howell, E., Kobayashi, D., Parker, D., Balazs, G., and Polovina, A. (2008). TurtleWatch: a tool to aid in the bycatch reduction of loggerhead turtles Caretta caretta in the Hawaii-based pelagic longline fishery. Endanger. Species Res. 5, 267–278. doi: 10.3354/esr00096
Howell, E. A., Hoover, A., Benson, S. R., Bailey, H., Polovina, J. J., Seminoff, J. A., et al. (2015). Enhancing the turtlewatch product for leatherback sea turtles, a dynamic habitat model for ecosystem-based management. Fish. Oceanogr. 24, 57–68. doi: 10.1111/fog.12092
Huse, G., Fernö, A., and Holst, J. (2010). Establishment of new wintering areas in herring co-occurs with peaks in the ‘first time/repeat spawner’ ratio. Mar. Ecol. Prog. Ser. 409, 189–198. doi: 10.3354/meps08620
ICES (2017). Report of the Working Group on Multispecies Assessment Methods (WGSAM). ICES CM 2016/SSGEPI:21, Reykjavik.
IPCC (2013). Climate Change 2013: The Physical Science Basis. Contribution of Working Group I to the Fifth Assessment Report of the Intergovernmental Panel on Climate Change. Cambridge University Press, Cambridge; NY, NY, 1535.
Ito, S. I., Okunishi, T., Kishi, M. J., and Wang, M. (2013). Modelling ecological responses of Pacific saury (Cololabis saira) to future climate change and its uncertainty. ICES J. Mar. Sci. 70, 980–990. doi: 10.1093/icesjms/fst089
Jacox, M. G., Hazen, E. L., Zaba, K. D., Rudnick, D. L., Edwards, C. A., Moore, A. M., et al. (2016). Impacts of the 2015-2016 El Niño on the California current system: early assessment and comparison to past events. Geophys. Res. Lett. 43, 7072–7080. doi: 10.1002/2016GL069716
Jennings, S., Stentiford, G. D., Leocadio, A. M., Jeffery, K. R., Metcalfe, J. D., Katsiadaki, I., et al. (2016). Aquatic food security: insights into challenges and solutions from an analysis of interactions between fisheries, aquaculture, food safety, human health, fish and human welfare, economy and environment. Fish Fish. 17, 893–938. doi: 10.1111/faf.12152
Kaplan, I. C., Williams, G. D., Bond, N. A., Hermann, A. J., and Siedlecki, S. A. (2016). Cloudy with a chance of sardines: forecasting sardine distributions using regional climate models. Fish. Oceanogr. 25, 15–27. doi: 10.1111/fog.12131
Keenlyside, N. S., Latif, M., Jungclaus, J., Kornblueh, L., and Roeckner, E. (2008). Advancing decadal-scale climate prediction in the North Atlantic sector. Nature 453, 84–88. doi: 10.1038/nature06921
Kirtman, B. P., Min, D., Infanti, J. M., Kinter, J. L., Paolino, D. A., Zhang, Q., et al. (2014). The North American multimodel ensemble: phase-1 seasonal-to-interannual prediction; Phase-2 toward developing intraseasonal prediction. Bull. Am. Meteorol. Soc. 95, 585–601. doi: 10.1175/BAMS-D-12-00050.1
Lehodey, P., Senina, I., and Murtugudde, R. (2008). A spatial ecosystem and populations dynamics model (SEAPODYM)—Modeling of tuna and tuna-like populations. Prog. Oceanogr. 78, 304–318. doi: 10.1016/j.pocean.2008.06.004
Li, H., Ilyina, T., Müller, W. A., and Sienz, F. (2016). Decadal predictions of the North Atlantic CO2 uptake. Nat. Commun. 7:11076. doi: 10.1038/ncomms11076
Lindegren, M., Möllmann, C., Nielsen, A., Brander, K. M., Mackenzie, B. R., and Stenseth, N. C. (2010). Ecological forecasting under climate change: the case of Baltic cod. Proc. Biol. Sci. 277, 2121–2130. doi: 10.1098/rspb.2010.0353
MacKenzie, B. R., and Köster, F. W. (2004). Fish production and climate: sprat in the Baltic Sea. Ecology 85, 784–794. doi: 10.1890/02-0780
MacKenzie, B. R., Meier, H. E. M., Lindegren, M., Neuenfeldt, S., Eero, M., Blenckner, T., et al. (2012). Impact of climate change on fish population dynamics in the Baltic sea: a dynamical downscaling investigation. Ambio 41, 626–636. doi: 10.1007/s13280-012-0325-y
Mantzouni, I., and Mackenzie, B. R. (2010). Productivity responses of a widespread marine piscivore, Gadus morhua, to oceanic thermal extremes and trends. Proc. Biol. Sci. 277, 1867–1874. doi: 10.1098/rspb.2009.1906
Margonski, P., Hansson, S., Tomczak, M. T., and Grzebielec, R. (2010). Climate influence on Baltic cod, sprat, and herring stock–recruitment relationships. Prog. Oceanogr. 87, 277–288. doi: 10.1016/j.pocean.2010.08.003
Matei, D., Baehr, J., Jungclaus, J. H., Haak, H., Muller, W. A., and Marotzke, J. (2012a). Multiyear prediction of monthly mean atlantic meridional overturning circulation at 26.5 N. Science 335, 76–79. doi: 10.1126/science.1210299
Matei, D., Pohlmann, H., Jungclaus, J., Müller, W., Haak, H., and Marotzke, J. (2012b). Two Tales of initializing decadal climate prediction experiments with the ECHAM5/MPI-OM model. J. Clim. 25, 8502–8523. doi: 10.1175/JCLI-D-11-00633.1
Maynard, J., van Hooidonk, R., Harvell, C. D., Eakin, C. M., Liu, G., Willis, B. L., et al. (2016). Improving marine disease surveillance through sea temperature monitoring, outlooks and projections. Philos. Trans. R. Soc. B 371:20150208. doi: 10.1098/rstb.2015.0208
McInerny, G. J., and Etienne, R. S. (2013). “Niche” or “distribution” modelling? A response to Warren. Trends Ecol. Evol. 28, 191–192. doi: 10.1016/j.tree.2013.01.007
Meehl, G. A., Goddard, L., Boer, G., Burgman, R., Branstator, G., Cassou, C., et al. (2014). Decadal climate prediction an update from the trenches. Bull. Am. Meteorol. Soc. 95, 243–267. doi: 10.1175/BAMS-D-12-00241.1
Michael, P. E., Wilcox, C., Tuck, G. N., Hobday, A. J., and Strutton, P. G. (2017). Japanese and Taiwanese pelagic longline fleet dynamics and the impacts of climate change in the southern Indian Ocean. Deep Sea Res. 140, 242–250. doi: 10.1016/j.dsr2.2016.12.003
Mills, K., Pershing, A., Brown, C., Chen, Y., Chiang, F.-S., Holland, D., et al. (2013). Fisheries management in a changing climate: lessons from the 2012 ocean heat wave in the Northwest Atlantic. Oceanography 26, 191–195. doi: 10.5670/oceanog.2013.27
Msadek, R., Delworth, T. L., Rosati, A., Anderson, W., Vecchi, G., Chang, Y.-S., et al. (2014). Predicting a decadal shift in north atlantic climate variability using the GFDL forecast system. J. Clim. 27, 6472–6496. doi: 10.1175/JCLI-D-13-00476.1
Mueter, F. (2002). Opposite effects of ocean temperature on survival rates of 120 stocks of Pacific salmon (Oncorhynchus spp.) in northern and southern areas. Can. J. Fish. Aquat. Sci. 463, 456–463. doi: 10.1139/f02-020
Mullon, C., Guillotreau, P., Galbraith, E. D., Fortilus, J., Chaboud, C., Bopp, L., et al. (2017). Exploring future scenarios for the global supply chain of tuna. Deep Sea Res. 140, 251–267. doi: 10.1016/j.dsr2.2016.08.004
Murphy, A. H. (1993). What Is a Good Forecast? An essay on the nature of goodness in weather forecasting. Weather Forecast. 8, 281–293. doi: 10.1175/1520-0434(1993)008<0281:WIAGFA>2.0.CO;2
Myers, R. A. (1998). When do environment–recruitment correlations work? Rev. Fish Biol. Fish 8, 285–305. doi: 10.1023/A:1008828730759
Neuenfeldt, S., and Beyer, J. E. (2006). Environmentally driven predator-prey overlaps determine the aggregate diet of the cod Gadus morhua in the Baltic Sea. Mar. Ecol. Prog. Ser. 310, 151–163. doi: 10.3354/meps310151
NOAA (2016). HAB Forecasts. Avaliable online at: https://coastalscience.noaa.gov/research/habs/forecasting.
Nye, J. A., Baker, M. R., Bell, R., Kenny, A., Kilbourne, K. H., Friedland, K. D., et al. (2014). Ecosystem effects of the Atlantic multidecadal oscillation. J. Mar. Syst. 133, 103–116. doi: 10.1016/j.jmarsys.2013.02.006
Österblom, H., and Bodin, Ö. (2012). Global cooperation among diverse organizations to reduce illegal fishing in the southern ocean. Conserv. Biol. 26, 638–648. doi: 10.1111/j.1523-1739.2012.01850.x
Ottersen, G., Planque, B., Belgrano, A., Post, E., Reid, P., and Stenseth, N. C. (2001). Ecological effects of the North Atlantic oscillation. Oecologia 128, 1–14. doi: 10.1007/s004420100655
Paasche, Ø., Österblom, H., Neuenfeldt, S., Bonsdorff, E., Brander, K. M., Conley, D. J., et al. (2015). Connecting the seas of norden. Nat. Clim. Change 5, 89–92. doi: 10.1038/nclimate2471
Pacific Fishery Management Council (2016). Preseason Report I: Stock Abundance Analysis and Environmental Assessment Part 1 for 2016 Ocean Salmon Fishery Regulations. Pacific Fishery Management Council, Portland, OR.
Parnell, P. E., Dayton, P. K., Fisher, R. A., Loarie, C. C., and Darrow, R. D. (2010). Spatial patterns of fishing effort off San Diego: implications for zonal management and ecosystem function. Ecol. Appl. 20, 2203–2222. doi: 10.1890/09-1543.1
Pearson, R. G., Stanton, J. C., Shoemaker, K. T., Aiello-Lammens, M. E., Ersts, P. J., Horning, N., et al. (2014). Life history and spatial traits predict extinction risk due to climate change. Nat. Clim. Change 4, 217–221. doi: 10.1038/nclimate2113
Petitgas, P., Rijnsdorp, A. D., Dickey-Collas, M., Engelhard, G. H., Peck, M. A., Pinnegar, J. K., et al. (2013). Impacts of climate change on the complex life cycles of fish. Fish. Oceanogr. 22, 121–139. doi: 10.1111/fog.12010
Planque, B., Fox, C. J., Saunders, M. A., and Rockett, P. (2003). On the prediction of short term changes in the prediction of recruitment in North Sea cod (Gadus morhua) using statistical temperature forecasts. Sci. Mar. 67(Suppl. 1), 211–218. doi: 10.3989/scimar.2003.67s1211
Platt, T., Fuentes-Yaco, C., and Frank, K. T. (2003). Marine ecology: spring algal bloom and larval fish survival. Nature 423, 398–399. doi: 10.1038/423398b
Reimer, M. N., Abbott, J. K., and Haynie, A. C. (2017). Empirical models of fisheries production: conflating technology with incentives? Mar. Res. Econ. 32, 169–190. doi: 10.1086/690677
Ricard, D., Minto, C., Jensen, O. P., and Baum, J. K. (2012). Examining the knowledge base and status of commercially exploited marine species with the RAM legacy stock assessment database. Fish Fish. 13, 380–398. doi: 10.1111/j.1467-2979.2011.00435.x
Sanchirico, J. N., Lew, D. K., Haynie, A. C., Kling, D. M., and Layton, D. F. (2013). Conservation values in marine ecosystem-based management. Mar. Policy 38, 523–530. doi: 10.1016/j.marpol.2012.08.008
Scaife, A. A., Arribas, E., Brookshawa, R. T., Dunstone, N., Eade, R., Fereday, C. K., et al. (2014). Skillful long range prediction of European and North American winters. Geophys. Res. Lett. 5, 2514–2519. doi: 10.1002/2014GL059637
Séférian, R., Bopp, L., Gehlen, M., Swingedouw, D., Mignot, J., Guilyardi, E., et al. (2014). Multiyear predictability of tropical marine productivity. Proc. Natl. Acad. Sci. U.S.A. 111, 11646–11651. doi: 10.1073/pnas.1315855111
Siedlecki, S. A., Kaplan, I. C., Hermann, A. J., Nguyen, T. T., Bond, N. A., Newton, J. A., et al. (2016). Experiments with seasonal forecasts of ocean conditions for the Northern region of the California Current upwelling system. Sci. Rep. 6:27203. doi: 10.1038/srep27203
Skern-Mauritzen, M., Ottersen, G., Handegard, N. O., Huse, G., Dingsør, G. E., Stenseth, N. C., et al. (2016). Ecosystem processes are rarely included in tactical fisheries management. Fish Fish. 17, 165–175. doi: 10.1111/faf.12111
Smith, D. M., Cusack, S., Colman, A. W., Folland, C. K., Harris, G. R., and Murphy, J. M. (2007). Improved surface temperature prediction for the coming decade from a global climate model. Science 317, 796–799. doi: 10.1126/science.1139540
Smith, D. M., Scaife, A. A., Eade, R., and Knight, J. R. (2016). Seasonal to decadal prediction of the winter North Atlantic Oscillation: emerging capability and future prospects. Q. J. R. Meteorol. Soc. 142, 611–617. doi: 10.1002/qj.2479
Stenseth, N. C., Ottersen, G., Hurrell, J. W., Mysterud, A., Lima, M., Chan, K.-S., et al. (2003). Review article. Studying climate effects on ecology through the use of climate indices: the North Atlantic Oscillation, El Nino Southern Oscillation and beyond. Proc. R. Soc. B 270, 2087–2096. doi: 10.1098/rspb.2003.2415
Stock, C. A., Pegion, K., Vecchi, G. A., Alexander, M. A., Tommasi, D., Bond, N. A., et al. (2015). Seasonal sea surface temperature anomaly prediction for coastal ecosystems. Prog. Oceanogr. 137, 219–236. doi: 10.1016/j.pocean.2015.06.007
Szuwalski, C. S., Vert-Pre, K. A., Punt, A. E., Branch, T. A., and Hilborn, R. (2015). Examining common assumptions about recruitment: a meta-analysis of recruitment dynamics for worldwide marine fisheries. Fish Fish. 16, 633–648. doi: 10.1111/faf.12083
Tommasi, D., Stock, C. A., Hobday, A. J., Methot, R., Kaplan, I. C., Eveson, J. P., et al. (2017a). Managing living marine resources in a dynamic environment: the role of seasonal to decadal climate forecasts. Prog. Oceanogr. 152, 15–49. doi: 10.1016/j.pocean.2016.12.011
Tommasi, D., Stock, C. A., Pegion, K., Vecchi, G. A., Methot, R. D., Alexander, M. A., et al. (2017b). Improved management of small pelagic fisheries through seasonal climate prediction. Ecol. Appl. 27, 378–388. doi: 10.1002/eap.1458
Tracey, S., Buxton, C., Gardner, C., Green, B., Hartmann, K., Haward, M., et al. (2013). Super trawler scuppered in australian fisheries management reform. Fisheries 38, 345–350. doi: 10.1080/03632415.2013.813486
Urban, M. C., Bocedi, G., Hendry, A. P., Mihoub, J.-B., Peer, G., Singer, A., et al. (2016). Improving the forecast for biodiversity under climate change. Science 353:aad8466. doi: 10.1126/science.aad8466
van Oldenborgh, G. J., Doblas-Reyes, F. J., Wouters, B., and Hazeleger, W. (2012). Decadal prediction skill in a multi-model ensemble. Clim. Dyn. 38, 1263–1280. doi: 10.1007/s00382-012-1313-4
Van Pelt, T. I., Napp, J. M., Ashjian, C. J., Harvey, H. R., Lomas, M. W., Sigler, M. F., et al. (2016). An introduction and overview of the Bering Sea Project: volume, IV. Deep Sea Res. 134, 3–12. doi: 10.1016/j.dsr2.2016.09.002
Vert-pre, K. A., Amoroso, R. O., Jensen, O. P., and Hilborn, R. (2013). Frequency and intensity of productivity regime shifts in marine fish stocks. Proc. Natl. Acad. Sci. U.S.A. 110, 1779–1784. doi: 10.1073/pnas.1214879110
Ward, T. J., Heinemann, D., and Evans, N. (2001). The Role of Marine Reserves as Fisheries Management Tools: a Review of Concepts, Evidence and International Experience. Canberra, ACT.
Warren, D. L. (2012). In defense of 'niche modeling. Trends Ecol. Evol. 27, 497–500. doi: 10.1016/j.tree.2012.03.010
Warren, D. L. (2013). ‘Niche modeling’: that uncomfortable sensation means it's working. A reply to McInerny and Etienne. Trends Ecol. Evol. 28, 193–194. doi: 10.1016/j.tree.2013.02.003
Wouters, B., Hazeleger, W., Drijfhout, S., van Oldenborgh, G. J. J., and Guemas, V. (2013). Multiyear predictability of the North Atlantic subpolar gyre. Geophys. Res. Lett. 40, 3080–3084. doi: 10.1002/grl.50585
Keywords: ecological forecasting, seasonal-to-decadal-forecasting, spatial distribution, recruitment, Phenology, marine ecological forecasting, ecological prediction
Citation: Payne MR, Hobday AJ, MacKenzie BR, Tommasi D, Dempsey DP, Fässler SMM, Haynie AC, Ji R, Liu G, Lynch PD, Matei D, Miesner AK, Mills KE, Strand KO and Villarino E (2017) Lessons from the First Generation of Marine Ecological Forecast Products. Front. Mar. Sci. 4:289. doi: 10.3389/fmars.2017.00289
Received: 17 February 2017; Accepted: 25 August 2017;
Published: 12 September 2017.
Edited by:
Corinna Schrum, Helmholtz Zentrum Geesthacht, GermanyReviewed by:
Johanna Baehr, University of Hamburg, GermanyCopyright © 2017 Payne, Hobday, MacKenzie, Tommasi, Dempsey, Fässler, Haynie, Ji, Liu, Lynch, Matei, Miesner, Mills, Strand and Villarino. This is an open-access article distributed under the terms of the Creative Commons Attribution License (CC BY). The use, distribution or reproduction in other forums is permitted, provided the original author(s) or licensor are credited and that the original publication in this journal is cited, in accordance with accepted academic practice. No use, distribution or reproduction is permitted which does not comply with these terms.
*Correspondence: Mark R. Payne, bXBheUBhcXVhLmR0dS5kaw==
Disclaimer: All claims expressed in this article are solely those of the authors and do not necessarily represent those of their affiliated organizations, or those of the publisher, the editors and the reviewers. Any product that may be evaluated in this article or claim that may be made by its manufacturer is not guaranteed or endorsed by the publisher.
Research integrity at Frontiers
Learn more about the work of our research integrity team to safeguard the quality of each article we publish.