- Institute of Marine Sciences, Middle East Technical University, Mersin, Turkey
Black Sea anchovy (Engraulis encrasicolus) undertake extensive overwintering migrations every fall from nursery grounds to warmer overwintering areas located on the south-eastern coast of the Black Sea. During migration and particularly upon arrival at the Anatolian coast, they support an important fishery and valuable source of income for the regional community. Black Sea anchovy have undergone significant stock fluctuations partly related to climatic conditions; for example, migrating anchovy schools arrived late or failed to arrive at the Anatolian coast when fall temperatures increased. It is therefore of importance to understand the conditions required for successful overwintering migration and explore different migration routes. This study invokes a Lagrangian modeling approach applied to satellite derived circulation and temperature data as a first attempt to model anchovy migration dynamics in the Black Sea. This modeling approach takes the influence of the physical environment into account, while the quality of overwintering grounds, adaptive, schooling, and homing behavior is neglected. The model is used to investigate the possible influence of interannual and seasonal variability of temperature and surface currents, as well as the influence of migration behavior on the success of anchovy overwintering migration for both the Black Sea and Azov Sea anchovy. The results of the present work show the possibility that overwintering anchovy fished along the Turkish Eastern Anatolian coast may not exclusively originate from the northwestern shelf, but mainly from the eastern Black Sea basin. Migration pathways are identified for both Black Sea and Azov Sea anchovy, which are of importance for the national fisheries efforts of riparian countries. The modeling results are in agreement with general patterns of anchovy migration given in the literature indicating that the physical environment may be a major factor in shaping general migration patterns. Simulation results are used to hypothesize about alternatives to previously determined migration routes and provide potential reasons that explain the inability of the Bulgarian anchovy fishery to recover. Results show that the intensity and timing of autumnal cooling, coupled with current strength, can be of significant importance in determining annual and seasonal variability of migration success. Considering the need for fisheries management to account for the variability in fishable overwintering anchovy stocks a modeling approach as developed in the current study may provide such a tool.
Introduction
The key to estimating the available species in an ecosystem requires an understanding of their geographical distribution (Harte, 2002). Movement can affect fish populations through changes in population density, modifying interspecific interactions or through genetic reorganizations (Turchin and Omland, 1999). In fisheries assessment and management, the patterns of fish movement can be used to explain stock fluctuations (Pelletier and Parma, 1994). The way that marine organisms disperse depends on the species, species behavior, and currents (Marinone et al., 2008). Considering the long-distance migration that anchovy in the Black Sea undergo every year, studying movement of populations can provide valuable insights for fisheries science (Goodwin et al., 2006). Therefore, simulation of movement of individual particles in a dynamic environment is an important tool for investigating ecological processes in the marine environment (Heath and Gallego, 1998; Miller et al., 1998; Hare et al., 1999).
Models are very useful tools for understanding and simulating fish movement under changing environmental conditions (Goodwin et al., 2006). However, modeling the movement of fish is challenging as the mechanisms that cause the movement are often not well-known (Watkins and Rose, 2013) and data is scarce (Haas et al., 2004; Roth et al., 2008). Modeling studies have regularly reported close relationships between small pelagics abundance and distribution patterns and sea surface temperature distributions, i.e., in herring in the Barents Sea (Gjøsæter, 1998; Gjøsæter et al., 1998; Huse et al., 2010), capelin in the Barents Sea (Dommasnes and Røttingen, 1984; Ozhigin and Luka, 1985), sardine in the Pacific (west) coast of the USA and Canada and the seas around Japan (Tameishi, 1996; Huse and Ellingsen, 2008; Zwolinski et al., 2011, 2012) and mackerel in the Norwegian Sea (Iversen, 2002). Modeling results also point out that, changes in the physical environment fish are exposed to may eventually alter migration pathways (Wang et al., 2013).
Anchovy migration in the Black Sea is mainly driven by ambient temperature (Chashchin and Akselev, 1990; Panov and Chashchin, 1990; Panov and Spiridonova, 1998; Berdnikov et al., 1999; Shulman, 2002; Shulman et al., 2008). With the approach of cold temperatures, anchovy adults and juveniles aggregate to form dense schools and start wintering migration toward warmer waters located in the southern Black Sea. The upper temperature thresholds for Black Sea anchovy to start forming schools and begin migration vary. Furthermore, estimates of the temperature threshold at which anchovy start migrating differ ranging from 10.5–13.5°C for juveniles and 11.5–15.0°C for adults (Shulman et al., 2008), minimum of 12°C (Chashchin and Akselev, 1990) or 14°C (Panov and Spiridonova, 1998), or between 12 and 14°C (Panov and Chashchin, 1990). Chashchin et al. (2015) suggest adult Black Sea anchovy start to form migrating schools when the water temperature drops below 16–18°C. It has also been found that the adult anchovy first start migration, and later when the temperature drops further, the juveniles start migration (Gucu et al., 2017). In addition, an internal stimulus driving migration is thought to be the body fat content (Shulman, 2002).
The strong variability in anchovy catches in the Black Sea indicates strong spatial and temporal variability in anchovy biomass. As anchovy is a fast-growing and short-lived species, the influence of environmental drivers may regulate survival rate of the early life stages (Guraslan et al., 2014; Gucu et al., 2016). Of these factors, eutrophication, climate variability (Oguz et al., 2008a,b) and the invasion of an alien ctenophore (Mnemiopsis leidyi) have been stated to be of crucial influence.
Two anchovy subspecies are found in the Black Sea, the Black Sea anchovy (Engraulis encrasicolus ponticus) and Azov anchovy (E. encrasicolus maeticus) (Nikolsky, 2013). Azov Sea anchovy spawning grounds are located in the Azov Sea (Figure 1; Chashchin et al., 2015). Black Sea anchovy eggs and larvae have been found in much of the Black Sea, but based on extensive annual surveys of the Soviet Union from the 1970's to early 1990's of the entire northern part of the Black Sea it was concluded that the main spawning ground of Black Sea anchoy is located on the northwestern shelf, rather than the northeastern regions (Ivanov and Beverton, 1985; Chashchin, 1996; Lisovenko and Andrianov, 1996; Chashchin et al., 2015). Unfortunately, this data set does not continue past the solution of the Soviet Union. Later, international studies covering the entire Black Sea in the 1991 and 1992 spawning seasons revealed that in 1992 most anchovy eggs were found in the southern Black Sea, with highest egg densities (1,167 ind m−2) found in the Samsun region and highest larvae densities in the southwestern Black Sea (up to 55 ind. m−2) (Niermann et al., 1994). This pattern of egg and larvae distribution was further confirmed by later surveys along the southern Black Sea coast in 1993 and 1996 (Kideys et al., 1999). Results from a recent ichthyoplankton survey in the southern Black Sea in 2013 revealed egg densities (6–3,051 ind. m−2) and larvae densities (3–359 ind. m−2) to be considerably higher than in previous studies (Gucu et al., 2016). Unfortunately, these surveys were not conducted continuously and over longer time scales, however they suggest anchovy spawning is not restricted to the northwestern shelf but occurs over much of the Black Sea with more or less success.
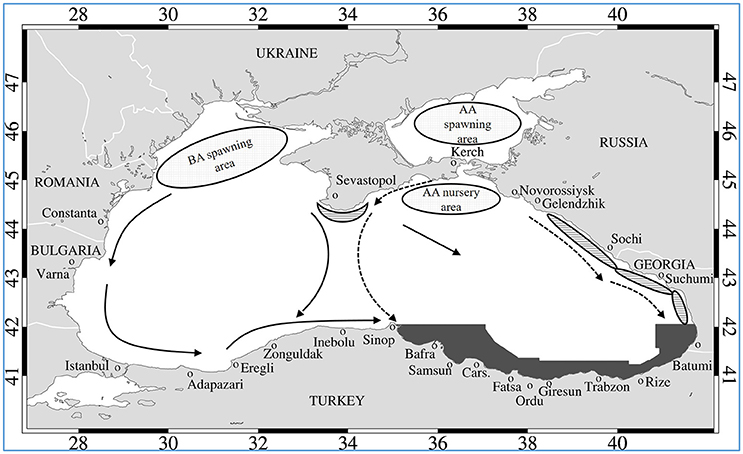
Figure 1. Conceptual figure of Azov anchovy (AA) and Black Sea anchovy (BA) spawning and foraging regions, fall migration paths (indicated by arrows) and overwintering grounds (hatched ovals along Russian and Georgia coasts, as well as the Crimean Peninsula–AA anchovy and gray shaded area–BS anchovy). Redrawn combining concepts of Ivanov and Beverton (1985) and Chashchin (1996).
Adult anchovy densities along the entire southern coast during fall and winter have been determined to be variable during the years 2011–2014, measured as between 3 and 900 Nasc m2 nmi2 (Nautical Acoustic Scattering Coefficient) with the highest overwintering densities of 244–949 Nasc m2 nmi2 in the southwestern coastal waters in fall 2014 and hotspots of high anchovy densities far offshore waters of the southeastern coast (Gucu et al., 2017). Gucu et al. (2016) hypothesize that there is a southern stock of anchovy that reproduces in the area and is non-migrating.
The overwintering grounds of Black Sea anchovy are located along the southeastern Black Sea coast (Figure 1) as recorded by Chashchin (1996) and reconfirmed with recent, multi-year acoustic and landing surveys (Gucu et al., 2017). To reach these overwintering grounds, anchovy must migrate long distances across the Black Sea from the northern shelf spawning and nursery grounds. Black Sea anchovy overwintering migration takes place after the onset of autumnal cooling either at the beginning of October (Ivanov and Beverton, 1985; Lisovenko and Andrianov, 1996; Shulman, 2002) or by mid-November (Chashchin, 1996). Migrating Black Sea anchovy leaving the spawning grounds in the northwestern shelf have been defined to move south along the Romanian and Bulgarian coasts following the rim current eastward along the southwestern Black Sea coast (Ivanov and Beverton, 1985; Chashchin, 1996) and/or move toward the west coast of Crimea and then southwards to the Anatolian coast midway between the Eastern and Western Basin (Figure 1).
Likewise, Azov anchovy leave their spawning and nursery grounds in the Sea of Azov and migrate to the Black Sea overwintering grounds (Chashchin, 1996). They follow the Crimean coast migrating across its central basin midway between western and eastern gyres or migrate along the Caucasian coast (Figure 1) to overwinter there or they may rarely migrate along the Georgian coast even reaching the Turkish border (Chashchin, 1996 and references therein). Azov anchovy are mainly fished by the Ukrainian, Russian and Georgian fishing fleets, while Black Sea anchovy are fished almost exclusively by the Turkish fishing fleet.
This paper aims to explore the influence of climatic variability in the form of temperature variations and changes in geostrophic surface currents on anchovy distribution in the Black Sea, particularly looking at how this variability affects the migration pathways and success of anchovy moving toward the south-eastern coast where they are fished in the late fall and winter. This is achieved by including adult anchovy swimming behavior and the processes of decision-making into an existing Lagrangian Individual Based Model (Fach, 2014) to investigate possible anchovy overwintering migration routes from different nursery grounds to the overwintering grounds located along the south-eastern coast of the Black Sea in three climatically divergent years (2001–2003). This study represents a first attempt at modeling anchovy migration behavior in the Black Sea and the model results agree well with available data on migration pathways and anchovy distribution during the overwinter season from the literature, but should be validated with detailed survey data on anchovy distribution during migration when it becomes available.
The goal of this research is to understand the different factors influencing successful anchovy migration as well as to determine from which regions in the Black Sea anchovy can successfully migrate. The information gained on the variability of migration success between years and seasons due to the intensity and timing of cooling in fall, as well as the strength of currents, is of significant importance when establishing any fisheries management practices. Fisheries management needs to account for changes in the physical environment when targeting fish stocks (Hofmann and Powell, 1998; Xu et al., 2013; Constable et al., 2014; Checkley et al., 2017).
An Overview of the Black Sea Anchovy Fishery Characteristics
In recent years, the Black Sea fishery was dominated mainly by small pelagics, such as anchovy and sprat (STECF, 2015). Applying a Common Fisheries Policy (CFP) to the Black Sea is a challenging endeavor due to the fact that of the six riparian countries, only two are members of the EU (Romania, Bulgaria) with large legal, economic, political, and institutional differences across the basin and diverse fisheries interests and capacities. Despite attempts to improve fisheries management, there is a lack of effective regional cooperation by the riparian countries to date and stocks remain overexploited in the Black Sea (Goulding et al., 2014).
In Turkey, the Black Sea fisheries play an important role both in supplying the increasing protein demand of the growing population and by contributing to the gross domestic product through local employment. In 2013, the Black Sea catch corresponded to 62% of the total catch from Turkish seas (including the Sea of Marmara, the Aegean and the Eastern Mediterranean) with 15,000 fishermen corresponding to 45% of the total employment in marine fisheries (TUIK, 2013). The most important fishery in the Black Sea is anchovy and in 2013 with the relatively low catch of 154 ktons amounted to 58% of the total water resources catch within the Turkish Black Sea EEZ contributing ~249 million Turkish Liras (TRY) to the national income.
Anchovy catches vary greatly from year to year (Figure 2). Following over-exploitation of large pelagic fishes, dolphins, and demersals at the end of the 1960s, anchovy became the most abundant and commercially important target species, followed by sprat (Sprattus sprattus), the second in abundance (Daskalov, 2003). The total Black Sea anchovy catches in the 1970's oscillated at around 250 ktons escalating in the 1980's to as high as 560 ktons (Figure 2). This high anchovy catch regime persisted for a decade due to the extensive bottom-up food supply rendered by the eutrophic ecosystem conditions, and ended with the abrupt collapse of the anchovy fisheries in 1989–1990 (86 ktons). After the collapse, the Turkish anchovy fisheries recovered within a few years and since the early 1990's fluctuated between the range of 120 and 370 ktons while the fishing effort remained much the same (Oguz et al., 2012; STECF, 2015). Interestingly, other anchovy fisheries were not able to recover from the crash in the late 1980's (Figures 2A,B).
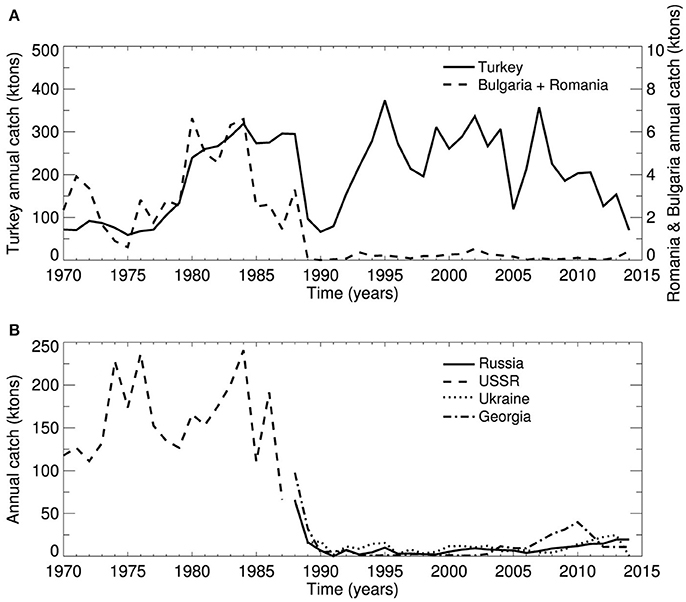
Figure 2. Total annual anchovy landings (ktons) of present and former Black Sea riparian countries from 1970 to 2014 (FAO, 2014). (A) Turkey and Bulgaria-Romania combined and (B) Russia, former USSR, Ukraine and Georgia.
The Turkish anchovy fishery is based on fishing schools of overwintering anchovy along the Turkish coast during winter time with purse seiners. The fishing season officially begins on 1st September until mid-April after which industrial fishing is banned for 4.5 months. However, this time period exceeds the actual availability of anchovy in the area which usually peaks between November and December, and therefore is not thought to impact the strong variability of catches. Fishing is restricted to night time only in an effort to control harvest and light fishing is not permitted, however there is no Total Allowable Catch (TAC) in place. In an effort to reduce fishing pressure, the licensing of new fishing boats has been halted since 2015 and a periodic, voluntary fishing vessel decommissioning program has been applied since 2012 (Gucu et al., 2017). High operational costs of the fishing fleet lead to fishermen targeting only large schools of anchovy that enable them to land large amounts of fish in one operation (Gucu et al., 2017) rendering targeting scattered anchovies non-profitable. Georgia's fishing fleet is much smaller (Castilla-Espino et al., 2014) and Georgian fishery enterprises hire a limited number of Turkish purse seiners each year. The fact that anchovy aggregate to form dense schools when overwintering makes them specifically vulnerable to exploitation and potential overexploitation (Auckland and Reid, 1998; Petitgas et al., 2001).
Methods
In this study, surface geostrophic currents of the Black Sea were calculated from satellite derived Sea Level Anomaly (SLA) data and the mean topography of the Sea. Using these geostrophic currents, a Lagrangian individual based model of anchovy transport was used to simulate anchovy advection across the Black Sea including swimming behavior.
Satellite Data
Black Sea surface circulation fields were calculated using the AVISO+ (Archiving, Validation, and Interpretation of Satellite Oceanographic data) Sea Level Anomalies (SLA) and geostrophic velocity anomalies regional product for the Black Sea. They are level 4 delayed time (DT) daily multi-mission sea surface heights anomalies data on a regular 1/8° × 1/8° grid created by a multi-satellite altimetric ground segment named SSALTO/DUACS (Segment Sol ALTimétrie et Orbitographie) system operating under Centre National d'Etudes Spatiales (CNES) and made available for use via AVISO+ catalog (http://www.aviso.altimetry.fr/en/data/products/sea-surface-height-products/regional/msla-black-sea.html). This daily AVISO data was then interpolated spatially onto a 1/16° × /10° (7 × 8 km) grid and the mean sea surface height (SSH) provided by Korotaev et al. (2003) was added to these fields to compute the absolute dynamic topography (ADT) of the Black Sea. The geostrophic surface currents were computed from these fields (Figure 3). Velocities calculated as >0.5 cm/s were assigned to 0.5 cm/s in order to reduce erroneous satellite data at the boundaries. However, it should be noted that this process smooths out offshore jets that are known to extend 100 km from the shelf break and reach velocities of about 70 cm/s (Ivanov et al., 1985 as cited in Oguz et al., 1994).
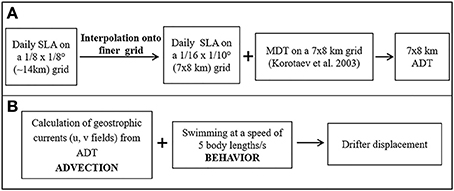
Figure 3. Schematic diagram of data processing and drifter (anchovy) displacement calculation performed in this study: (A) Daily Sea Level Anomaly (SLA) fields provided by AVISO+ were interpolated spatially onto a 7 × 8 km grid. The mean dynamic topography (MDT) compiled by Korotaev et al. (2003) was added to the SLA fields to obtain Absolute Dynamic Topography (ADT) fields from which geostrophic currents were computed. (B) Anchovy swimming behavior added to advection by currents results in net drifter displacement.
Using surface currents in this model is a valid assumption, since anchovy are known to occupy the warm, upper mixed layer and avoid low temperatures of the Cold Intermediate Layer below (Niermann et al., 1994; Kideys et al., 2000; Satilmis et al., 2003). Chashchin et al. (2015) suggested that factors such as wind direction, sea surface temperature distribution and currents are the most critical factors for Black Sea anchovy migration. The Black Sea circulation is highly variable and dominated by mesoscale eddies, meanders and filaments (Oguz et al., 1994; Oguz and Besiktepe, 1999; Korotaev et al. 2003). Incorporating this variability into our modeling approach by using SSH satellite-derived currents covers most of the effect of winds, salinity, eddy formation and frontal features that are of important features of the Black Sea circulation.
To account for temperature in the surface layer, sea surface temperature data obtained from Gruppo di Oceanografia da Satellite (GOS) was compiled and used in the model. This daily temperature product for the Black Sea was created using Advanced Very High Resolution Radiometer (AVHRR) data optimally interpolated onto a 1/16° × 1/16° grid (http://gosweb.artov.isac.cnr.it/). This data was then interpolated to the same 1/16° × 1/10° (7 × 8 km) model grid resolution as the AVISO data and during transport simulations the ambient sea surface temperature was extracted from this data set.
Fish Movement in the Model
In this study, drifters released in the surface current field resemble adult anchovy schools and a single drifter in the Lagrangian Particle Tracking algorithm follows the Eulerian time integration:
where the particle location is denoted by X at a certain time t and the velocity at location X is represented by V.
Compared to the previous particle tracking study of Black Sea anchovy by Fach (2014), the present study not only considers advection of anchovy but also the swimming behavior of anchovy, such as migration, is included. This is achieved by extending Equation 1 with a behavioral movement term:
where displacement by advection (Va) and behavioral swimming (Vb) both influence the velocity of a particle at location X and time t. When total velocity (V) acting on the particle is the sum of both behavioral and advective velocities, the integration of equation 2 gives:
Here the time step (dt) is equal to tn+1−tn where n is the index for time chosen as one minute in this study. Forward Eulerian method was used to integrate equation 2 (Parada et al., 2003; Guizien et al., 2006; Lett et al., 2007) and applied as:
One major benefit of using this method is that it required only the position (Xn) and one velocity field at the current time step V(X, tn) to estimate the new position Xn+1 of the drifter. Another advantage of this scheme was its lower CPU requirements compared to the fourth order Runge Kutta scheme.
The main disadvantage of choosing this first order differencing scheme is reduced accuracy which may eventually lead to divergence from real drifter trajectories (Bennett and Clites, 1987). To counteract such errors, the time step should be minimal. Here, the choice of a 60 s time step was small enough to ensure accuracy comparable to a second-order accurate scheme (Fach, 2014).
Parameterization of Anchovy Behavior
Anchovy are known to be sensitive to temperature, it being a key trigger for the onset of migration (Chashchin and Akselev, 1990; Panov and Chashchin, 1990; Panov and Spiridonova, 1998; Berdnikov et al., 1999; Shulman, 2002; Shulman et al., 2008) and are likely to follow temperature gradients to move toward warmer water in overwintering grounds (Chashchin, 1996). Temperature plays a critical role because of the special climatic feature of the Black Sea, where an exceptionally large zonal west-east temperature gradient exists with fall-winter temperatures in the southeastern region being ~4–5°C higher than the northwestern region (Buongiorno Nardelli et al., 2010; Capet et al., 2012). Anchovy can swim at high speeds of up to five body-lengths/s (bl/s) for long periods of time, as observed in Peruvian anchovy (Peraltilla and Bertrand, 2014), and similar swimming speeds have also been observed in the Black Sea anchovy (A.C. Gucu, pers. comm.). Hence, simulations including anchovy moving along temperature gradients with swimming speeds of 5 bl/s were performed. The mean length of individual adult anchovy in the school is thereby assumed to be 10 cm (Bilgin et al., 2016). In this individual based modeling approach, fish feeding and metabolism are not included, as Black Sea overwintering migration is considered to be a non-feeding migration (Shulman, 2002; Shulman et al., 2008).
Anchovy behavior in the form of movement following temperature gradients was incorporated into the Lagrangian model following the parameterization of Xu et al. (2013) where anchovy pursue a food gradient. Parameterizing fish movement following gradients is a valid method applied in many different studies, such as Huse et al. (2004) for capelin and cod, Tu et al. (2012) for the spawning migration of Japanese anchovy and Wang et al. (2013) for Japanese anchovy in the Yellow Sea. To implement this approach, the daily distance an adult anchovy can swim was calculated and the temperature distribution within the radius of this distance surrounding the drifter location checked for the warmest temperature. The model anchovy swim in that direction and the displacement of the drifter is obtained by adding the behavioral movement vector to that caused by the advection by currents starting from the drifter's initial position. Selecting the same initial position for both behavioral movement and advection is a reasonable assumption supported by an earlier sensitivity study done by Xu et al. (2013).
Internal fat storage accumulated during the pre-wintering season is suggested to be preparing anchovy for overwintering migration (Shulman, 2002; Shulman et al., 2008). Therefore, we assume for the model purpose that only those anchovy that accumulate enough reserves are able to start migration. With the onset of cooling, Black Sea and Azov Sea anchovy stop feeding, aggregate in large schools and then begin migration (Chashchin et al., 2015). In this study, the actual schooling behavior is not incorporated in the model. We assume that each drifter that is released represents an already aggregated school of anchovy with the necessary fat levels enabling them to perform the long-distance overwintering migration. Since this study focuses on modeling the overwintering migration of adult anchovy schools (from here on they will be referred to as “drifters”) to the overwintering grounds, all drifters reaching the narrow shelf region of the southern Black Sea (≤1,500 m) east of 35°E longitude (Figure 1, gray shaded area) were considered as drifters which achieved a successful migration.
Design of Simulations
To understand the impact of climatic variability on anchovy migration success, several sets of simulations were undertaken. A total of 7,176 Lagrangian drifters resembling anchovy schools were released in the surface circulation field of the Black Sea on October 30th of three different years (2001–2003) and tracked for 2 months. Of these, 1,026 drifters were released in the northwestern shelf (NWS), 179 off Kerch Strait, and the rest in the entire Black Sea. To test different environmental conditions in consecutive years, the years 2001 to 2003 were chosen after the analysis of satellite data spanning two decades, the 1990's and 2000's. The data sets revealed that 2001 was an exceptionally warm year, particularly in summer, with strong stratification mainly because of reduced wind stress in the winter of 2000–2001 (McQuatters-Gollop et al., 2008; Buongiorno Nardelli et al., 2010). The year 2002 was average in terms of temperature distribution and 2003 was remarkably cold when compared to the mean conditions, with significantly higher wind stress (McQuatters-Gollop et al., 2008). Based on this analysis and to compare results with the previous study of anchovy larval dispersal, (Fach, 2014) the years 2001 to 2003 were chosen in order to investigate the upper and lower extremes of environmental variability in the Black Sea, respectively. Incidentally, 2001–2003 also correspond to high anchovy catches of around 300 kton (Figure 2A).
Two sets of simulations in which particle displacement is due to (a) only horizontal advection by surface currents and (b) movement toward the highest temperature with 5 bl/s coupled with advection were run for each of the 3 years (Table 1), enabling the model to assess the impacts of merely physics and of specific behavior on migration success. For model sensitivity analysis, additional simulations using 1, 3 and 6.65 bl/s swimming speeds were undertaken. In the simulations presented here, it is differentiated between Black Sea anchovy, as the dominant species fished by Turkish fisheries assumed to spawn mainly on the NWS of the Black Sea, and Azov Anchovy that spawns in the Azov Sea and enters the Black Sea for overwintering through the Kerch strait.
Anchovy most likely begin overwintering migration depending on the temperatures in October (Chashchin and Akselev, 1990; Panov and Chashchin, 1990; Panov and Spiridonova, 1998; Berdnikov et al., 1999; Shulman, 2002; Shulman et al., 2008) and body fat content (Shulman, 2002; Shulman et al., 2008) but may start migration much earlier than October, as early as mid-September (A. C. Gucu, pers. comm.). Therefore, in a second set of simulations, drifters were released at three different times, September 15th and 30th and October 15th of 2003 to explore seasonal variability. The choice of the year 2003 was made as migration success was the highest of all 3 years and the pathways showed a diverse pattern encompassing a larger geographical area from where drifters could reach the overwintering area compared to the results of the other simulated years.
To ensure a sufficient number of drifters were tracked from different areas of the Black Sea in each simulation for reasons of impartiality, a statistical reliability test was performed. Results obtained by tracking 1,026 drifters released from the NWS were compared with similar simulations that released 7,888 drifters in the same region on October 30th of 2001, 2002, and 2003 with swimming speeds of 1, 3, 5 and 6.65 bl/s in the temperature gradient simulation setup. Results of this analysis showed the % difference in migration success between the simulation results with 7,888 vs. 1,026 drifters to be very low: 0.1, 0.5 and 0% with a swimming speed of 1 bl/s, 0.3, 0.1, and 0.2% at 3 bl/s and 1.4, 0, 0.5% at 5 bl/s in the years 2001, 2002, and 2003, respectively (Table 2). It was found that the results between both simulations do not differ significantly. In addition, the mean distance traveled by drifters was examined with the difference between high and low numbers of drifters between <1 km to 30 km, which is rather small considering the total distances traveled (0.2–3.2%). Hence, tracking small numbers of anchovy in the simulations is a reasonable choice helping reduce CPU requirements and run time.
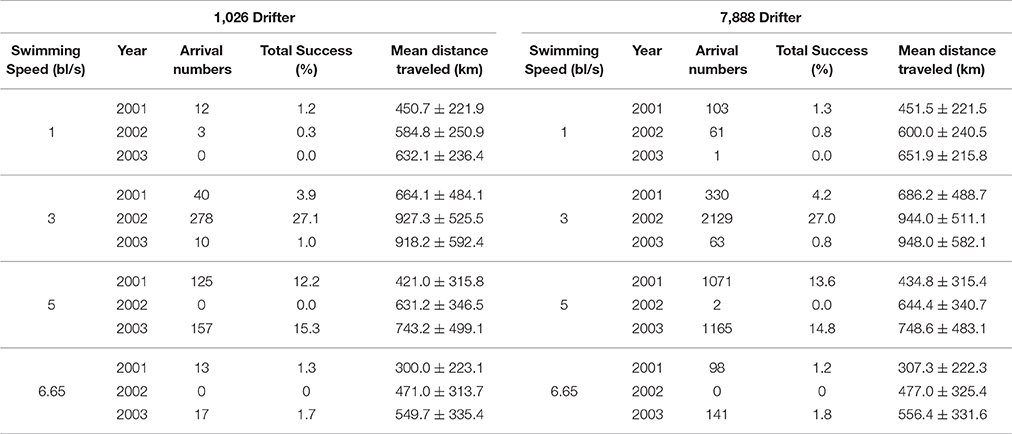
Table 2. The results of the statistical reliability study releasing 1,026 vs. 7,888 drifters from the northwestern Shelf on October 30th in years 2001, 2002, and 2003 in the temperature gradient simulations with 1–6.65 bl/s swimming speed for anchovy of 10 cm size.
Model results are compared to available data on anchovy overwintering migration (Chashchin, 1996; Chashchin et al., 2015) as well as anchovy distribution along the Black Sea coast from recent, multi-year, acoustic and landing surveys (Gucu et al., 2016, 2017). However, the lack of available, long-term anchovy spatial distribution data and anchovy migration observations make it difficult to perform an in-depth model validation.
Results
Environmental Conditions of the Black Sea
The year 2001 was exceptionally warm, with a mild winter followed by a very warm summer compared to other years in the 1990s and 2000s (McQuatters-Gollop et al., 2008; Fach, 2014). High mean SST's of 28°C in the eastern Black Sea were observed in July–August and decreased only slightly in August–September 2001 (24–26.5°C). The year 2002 was average in terms of temperature distribution 2003 was the coldest year in this study with SST's lower throughout most of the year than the mean SST's of the years 1997–2005 (McQuatters-Gollop et al., 2008). Maximum temperatures in July-August were significantly lower than the previous years (23–25.5°C) and August-September SST's were similar to those in 2002 (22–25.5°C), but with colder temperatures moving in from the north (Fach, 2014).
In an effort to explore the environmental conditions observed by migrating anchovy and how they may influence migration behavior and success, a summary of the SST and geostrophic surface currents in combination with SSH during November of all 3 years is presented. November is the most important month for migrating anchovy in this modeling study when direction of movement is shaping the migration routes. Further analysis of September and December is not presented here but can be found in Guraslan (2016).
Analysis of mean SST's over the entire Black Sea indicated distinct differences in the cooling process among the years of interest, in terms of timing (onset) and rates (development over time) that had implications for the migration of anchovy. Mean SST's in 2001 were highest in September (23.7 ± 0.81°C) and October (19.9 ± 0.94°C) with respect to 2002 and 2003 whereas December 2001 (10.0 ± 0.99°C) displayed the lowest SST's among all years. A sharp drop in the monthly mean SST occurred between October and November equivalent to 5.5°, 4.5°, and 5.1°C in the years 2001, 2002, and 2003, respectively. This is of importance as the main external trigger for anchovy to start migration is the cooling (i.e., sudden drop of temperature; Chashchin and Akselev, 1990; Panov and Chashchin, 1990; Shulman et al., 2008). However, not only the onset of cooling but the cooling patterns may also have an effect on the fate of migration as it is assumed that anchovy follow temperature gradients toward warmer regions.
In November 2001, after autumnal cooling commenced, the temperature distribution in the Black Sea showed a cold SST signal expanding over the basin excluding the Batumi Gyre area and southern coastal areas (Figure 4). The inner basin thereby displayed cooler temperatures (around 10°C) than the NWS. The cooling resulted in a northwest-southeast gradient over the basin. Simultaneously, November 2001 was also characterized by mesoscale variability in the flow resulting in the formation of many mesoscale eddies (Figure 4). Most importantly for migrating anchovy, the Sevastopol anticyclone formed on the NWS, an anticyclone occurred southeast of Crimea and a warm jet formed in the southern coast extending from the overwintering area to the inner basin.
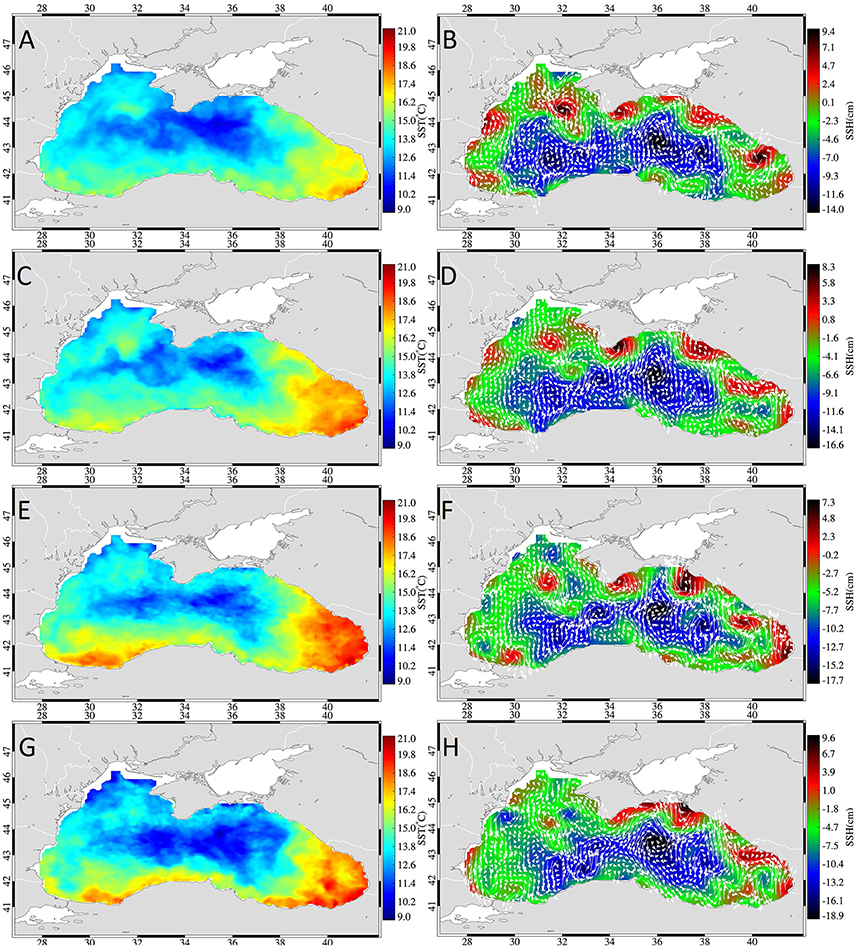
Figure 4. Weekly mean sea surface temperature (left) and sea surface height fields (right) overlaid with geostrophic currents (white arrows) during week 1 (A,B), 2 (C,D), 3 (E,F), and 4 (G,H) of November 2001.
In 2002, cooling started on the NWS in the second week of November and spread across the entire western basin, thereby creating a temperature gradient that was located almost in a W-E direction (Figure 5). Later in that month, warmer waters reached the southern and northern coasts of the basin and the Batumi eddy strengthened exhibiting high (~20°C) temperatures. The surface geostrophic currents were characterized by very structured flow with little mesoscale variability, indicating a strong rim current system around a large cyclonic cell with minor formation of mesoscale eddies.
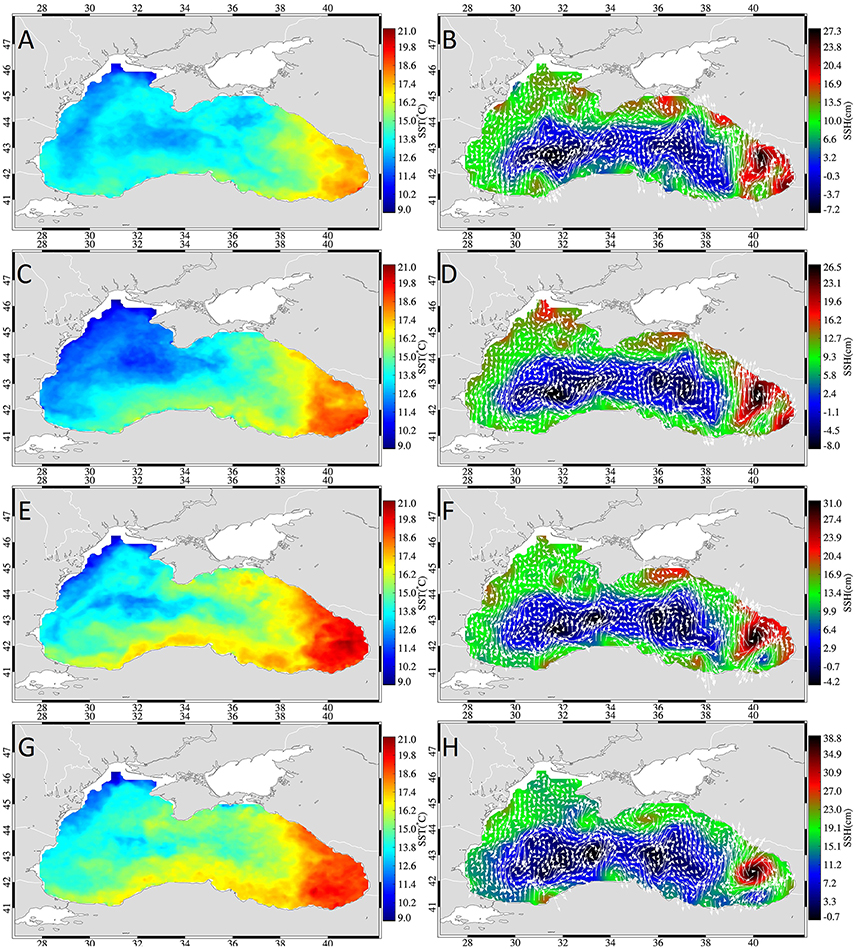
Figure 5. Weekly mean sea surface temperature (left) and sea surface height fields (right) overlaid with geostrophic currents (white arrows) during week 1 (A,B), 2 (C,D), 3 (E,F), and 4 (G,H) of November 2002.
The temperature distribution for 2003 showed cold temperatures on the NWS and off the Sea of Azov that warmed a little in the second week of November and then continued cooling in the western basin, creating a northwest-southeast temperature gradient (Figure 6) similar to 2001. The Batumi gyre was not well-defined and characterized with lower temperatures than in the other 2 years. This year was characterized by high mesoscale variability, especially within the western basin. The occurrence of the Sevastopol eddy at the shelf break zone and the anticyclone southeast of Crimea should be noted. In addition, the Sinop anticyclone generated a jet which extended into the inner basin from the south coast especially in weeks 3 and 4 of November (Figure 6).
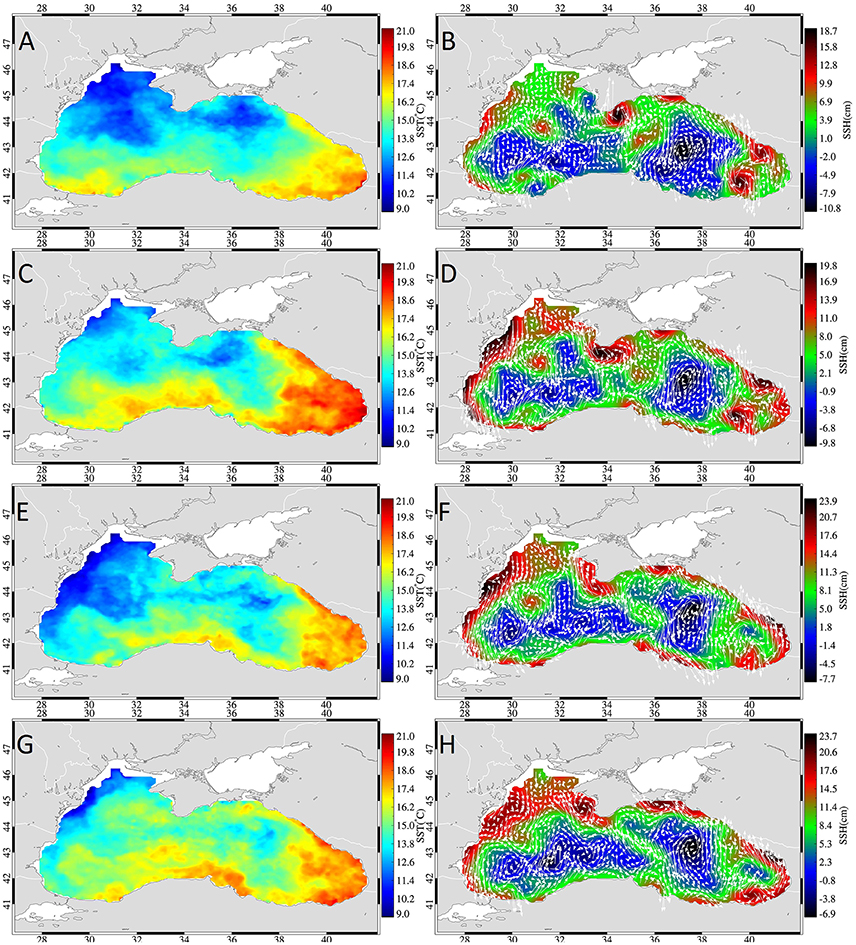
Figure 6. Weekly mean sea surface temperature (left) sea surface height fields (right) overlaid with geostrophic currents (white arrows) during week 1 (A,B), 2 (C,D), 3 (E,F), and 4 (G,H) of November 2003.
Interannual Variability in Overwintering Migration
Black Sea anchovy
When drifters representing Black Sea anchovy were released at the end of October on the NWS and tracked for 2 months in the advection only simulation, the results showed that the transport of anchovy from the NWS to the overwintering grounds is not possible given the variability by currents alone and hence migration fails in years 2001, 2002, and 2003 (Figures 7A–F).
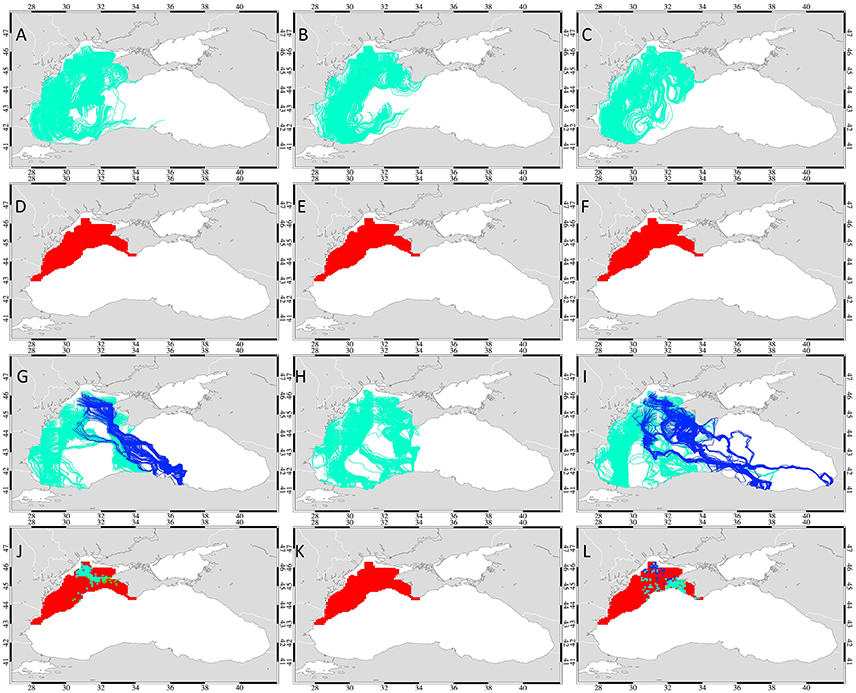
Figure 7. Simulations of interannual variability: The paths (A–C,G–I) and start points (D–F,J–L) of drifters released on the northwestern shelf at the end of October. Drifters were advected by surface geostrophic currents alone (A–F) and followed temperature gradients (G–L) swimming 5 bl/s in 2001, 2002, and 2003 (left, middle, and right column). The paths of drifters reaching the overwintering area in 2 months are marked blue, the ones that do not reach are marked green. The start points of drifters that complete a successful migration in 2, 4, 6, and 8 weeks are color-coded green, cyan, yellow and dark blue, respectively. The start points of the drifters that fail to reach the overwintering grounds are marked red.
However, simulation results with anchovy swimming along temperature gradients showed that successful migration is possible in 2001 and 2003, but fails in 2002 (Figures 7G–I). In 2001, simulated anchovy followed an almost diagonal path from the northern area of the NWS to final destinations near Sinop and Samsun in the western region of the overwintering area. A total of 125 drifters (12.2%) completed successful migration in this period and amongst those, 96 drifters from the northern part of the NWS reached the overwintering area in 4 weeks (Figures 7J–L, Table 3). The successful drifters are seen to follow the northern edge of the Sevastopol anticyclone and then another anticyclone located in the southeastern zone of the Sevastopol anticyclone (Figure 7G). Drifters approach the southern basin following the south-eastward front formed at ~15 nm offshore Sinop region during the second and third weeks of November to reach the Sinop region. Subsequently, some drifters follow the warm Rim Current eastwards and reach the Samsun region in the last week of November. Many drifters from the NWS still fail successful migration in this simulation, because of persistently cold temperatures in the interior basin which guide them to the warmer western coastal waters reaching only the Istanbul and Adapazari regions of the west Anatolian coast.
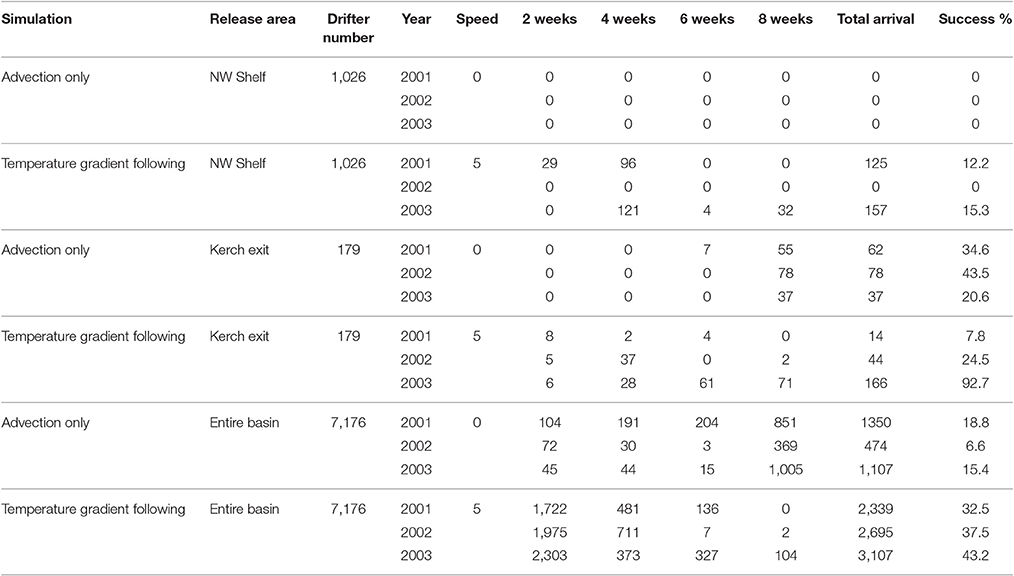
Table 3. Migration success of drifters released on October 30th in years 2001, 2002, and 2003 from the northwestern shelf, Kerch Strait exit region and the entire basin in the advection only and the temperature gradient simulation with 5 bl/s swimming speed.
In 2002, none of the drifters completed successful migration to the overwintering area even with application of swimming speed 5 bl/s (Figure 7H) due to particularly cold temperatures (19.0–21.0°C) in the second week of November which covered the western basin, leaving a slightly warmer region at the southern edge (Figure 5C). Hence, the drifters originating from the Danube and Constansa area followed the warmer Rim Current along the Anatolian coast, reached the closest warm regions between the Bosphorus and Eregli and stayed there. The remaining drifters crossed the basin or followed the eastern edge of the western gyre and also reached the Inebolu region of western Anatolia. In this year, strong flow around the intense western and eastern cyclonic gyres was observed with low mesoscale variability in the surface currents. This facilitated the efficient southward transport of drifters within the western basin, but the regional temperature distribution oriented the migration toward the warm patch off the southwestern coast and therefore no drifters reached the overwintering grounds.
In 2003, temperature gradient following drifters had the highest success rate between all 3 years and a total of 157 (15.3%) drifters reached the overwintering area (Figures 7I,L). Among those, most drifters originated from the areas located at the outer shelf between 31.8 and 33.3°E longitudes on the western side of Sevastopol, completing migration in 4 weeks (Table 3). Those drifters initially moved south along the warmer edge of the cyclonic formation at the Sevastopol coast in the first week of November and reached the western edge of the warmer Crimea eddy (Figure 6). In the second week, they followed the outer edge of the Crimea eddy merging with the central inner basin front carrying warmer waters of the southern basin northwards from the Sinop anticyclone to the Crimea anticyclone. On approaching the front at the northern region of the Sinop eddy, the drifters followed the warm waters of the Rim Current and moved along with it eastward. They finally completed their migration at the Carsamba (Samsun) and Fatsa (Ordu) coasts. In addition, some of the drifters that reached the tip of Crimea were observed to move southeastward, then across the Eastern Gyre and proceed to the southern coast of the eastern basin. Drifters from the northernmost areas of the NWS were able to reach the warmest parts of the overwintering area in 8 weeks. They followed the southern edge of the Sevastopol eddy at the shelf break and within the western gyre followed the front between the cyclonic gyres in the interior basin. These drifters reached the Inebolu region veering eastward at 42°N and approached the warmest regions of the basin located in the southeastern corner of the Black Sea, the Batumi Region, in 8 weeks. The intense cyclonic activity observed within the western basin, the increased Danube fresh water discharge flow and the strong western gyre are the features that caused retention of the remaining drifters in the western basin, leading them toward the nearest warmer regions at the Bulgaria, Bosphorus and Zonguldak—Inebolu coasts.
Azov Anchovy
When drifters representing Azov anchovy were released from the Kerch Strait area in the advection only simulation, 34.6, 43.5, and 20.6% of the drifters completed successful migration in 2001, 2002, and 2003, respectively (Table 3). This indicates that currents in the eastern part of the basin, though variable from year to year, do actively support transport to overwintering grounds. The specific pathways were along the western periphery of the eastern gyre in all years, whereby the pathway moved slightly east from 2001 to 2003 (Figures 8A–C) depending on the location of the Eastern Gyre in that particular year. Figures 8A–C details how the currents on the western periphery of the cyclonic eastern gyre connect the Kerch Strait region to the western region of the overwintering area. The average travel times were 6 to 8 weeks in 2001 and 8 weeks in both 2002 and 2003 (Figures 8D–F, Table 3).
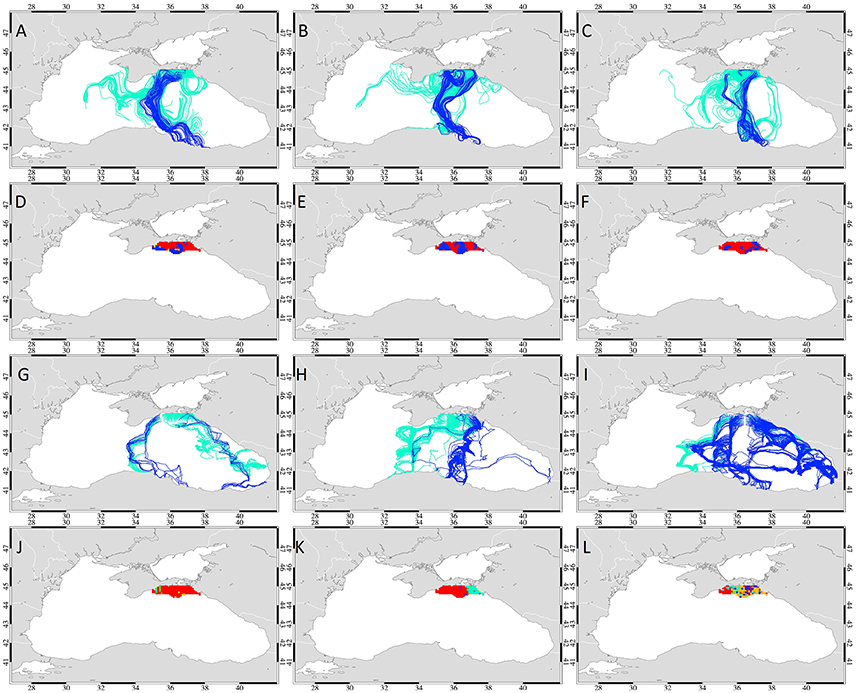
Figure 8. Simulations of interannual variability: The paths (A–C,G–I) and start points (D–F,J–L) of drifters released at Kerch Strait exit area at the end of October. Drifters were advected by surface geostrophic currents alone (A–F) and followed temperature gradients (G–L) swimming 5 bl/s in 2001, 2002, and 2003 (left, middle, and right column). The paths of drifters reaching the overwintering area in 2 months are marked blue, the ones that do not reach are marked green. The start points of drifters that complete a successful migration in 2, 4, 6, and 8 weeks are color-coded green, cyan, yellow and dark blue, respectively. The start points of the drifters that fail to reach the overwintering grounds are marked red.
Contrary to advection only simulation, in the temperature gradient simulation, the pathway orientation, migration success and the location of the source regions of drifters that complete a successful migration from Kerch region to the overwintering area experienced significant interannual variability (Figures 8G–I). Migration success is very low in 2001 (%7.8), low in 2002 (%24.5) but very high in 2003 with a 92.7% success rate (Figures 8J–L, Table 3). This strong variation is caused by the interannual variability in temperature distribution in the eastern basin apparent when analyzing the pathways of successful drifters.
In 2001, two possible pathways were identified: (i) midway between western and eastern gyres from source regions close to Crimea. Migration took only 2 weeks (Figure 8J), (ii) Movement along the east coast of the Black Sea to the Novorossysk region and further by offshore drift toward the Trabzon region and then to the Batumi region in 6 weeks. In 2002 the most successful drifters originated from the eastern sector of the release area and the majority completed migration in 4 weeks (Figure 8K). The successful drifters followed a third (iii) diagonal open ocean path from the Novorossysk region to the western (Bafra—Carsamba) section of the target area. They proceeded to move further east toward the Batumi region at 42°N latitude and approached the warmest areas of the basin at the Turkish—Georgian border in 4 weeks. In 2003, the western and eastern pathways previously identified in 2001 (the year of moderate mesoscale variability) reappeared with connections between them (Figure 8I). More than half of the successful drifters migrated south along the eastern Black Sea coast, moving partially against the flow associated with the rim currents in this region. These drifters took 6–8 weeks to arrive in the overwintering region, while those migrating along the western periphery currents of the eastern gyre appeared in 2 to 4 weeks (Figure 8L).
Entire Basin
Analysis of drifters released throughout the entire Black Sea in the advection only simulation portray the regions from where drifters can be advected to the overwintering area as the mouth of the Kerch Strait, along a longitudinal transect between the Sea of Azov and the Anatolian coast, the central coast of Anatolia in 2001 and in addition the Batumi region in 2003 (Figures 9A–C). Migration took between 4 to 8 weeks and varied significantly between years, with most drifters arriving in 2001 (18.8%), 15% in 2003 and only 6.6% in 2002 after different migration times (Table 3). The low success in 2002 is due to the well-organized cyclonic current flow around the interior basin which reduced retention in this area.
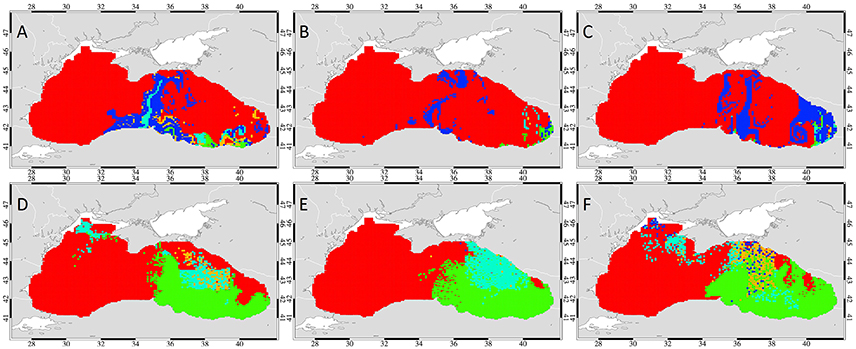
Figure 9. Simulations of interannual variability: The start points of 7176 drifters released at the end of October in the advection only simulation (A–C) and in the temperature gradient simulation (D–F) in 2001, 2002, and 2003 (left, middle, and right column). The start points of drifters that complete successful migration in 2, 4, 6, and 8 weeks are color-coded green, cyan, yellow, and dark blue, respectively. The start points of the drifters that do not reach the overwintering grounds are marked red.
In the temperature gradient simulations (Figures 9D–F), the migration success increased significantly (32.6–43%) and the migration duration decreased (Table 3). In these simulations, the eastern basin was the dominating source area for successful migration in all simulations. In particular, the southern part of the eastern basin is seen to transport drifters in 2 weeks in all years (Table 3). Successful drifters from the northern half of the eastern basin completed migration in 4 to 6 weeks in 2001 and 2002 simulations. However, in 2003, the majority of drifters in the northern half of the eastern basin were faster, completing migration in 2 weeks. It can be seen again, that only in the temperature gradient simulations drifters originating from the NWS were able to arrive at the overwintering area.
In 2001, the source areas supplying anchovy to the overwintering area were located within the open sea regions of the eastern basin (Figure 9D). The warmer coastal anticyclonic eddies were retention areas for drifters at the beginning of November when the sites of very high temperatures retreated to the narrow area at the southeastern boundary of the Black Sea. In 2002, drifters in the northern and southern regions of the eastern basin migrated in 2 and 4 weeks. The W-E SST gradient during November 2002 contributed to successful migration of drifters from the eastern basin. Moreover, low mesoscale variability during this time, stronger flow associated with the rim current and around the cyclonic western and eastern gyre in this period established fast transport from north to south between the gyres in November 2002. At the beginning of November 2003, the intense cooling that started in the second half of October resulted in decline of the highest temperature regions toward the narrow coastal area in the Batumi region. But in the second half of November, the region of high SST again extended further westwards (38°E) into the eastern inner basin, enabling successful migration of the majority of drifters in only 2 weeks.
Seasonal Variability of Overwintering Migration
The seasonal variability in migration success and pathways was explored by releasing drifters from both the NWS and Kerch Strait regions on three different dates (September 15, 30 and October 15) 2003, the year in which the highest migration success was observed in the temperature gradient simulations.
Similar to the above results, drifters from the NWS representing Black Sea anchovy were unable to reach the overwintering area in the advection only simulation for any of the release dates (Table 4), indicating that active swimming behavior is a prerequisite for successful arrival at the overwintering areas. In the temperature gradient simulations a migration success rate of 13.3% for drifters starting in mid-September (Figures 10A,D, Table 4) was observed, close to the 15% success rate of the reference simulation (initiated on October 30). Successful drifters moved toward the warmer Crimean Peninsula region and approached the warmer southern regions in October by following the warm water margin between the southern edge of Crimea eddy and the small anticyclone at the south. At the end of September, warm temperatures had reached the southeastern coast of Crimea whilst cool SST's approached in October from the northwest with the high SST's retreating toward the south coast. Warm water areas in the southeastern basin then began to retreat toward the eastern coastal areas at the end of October.
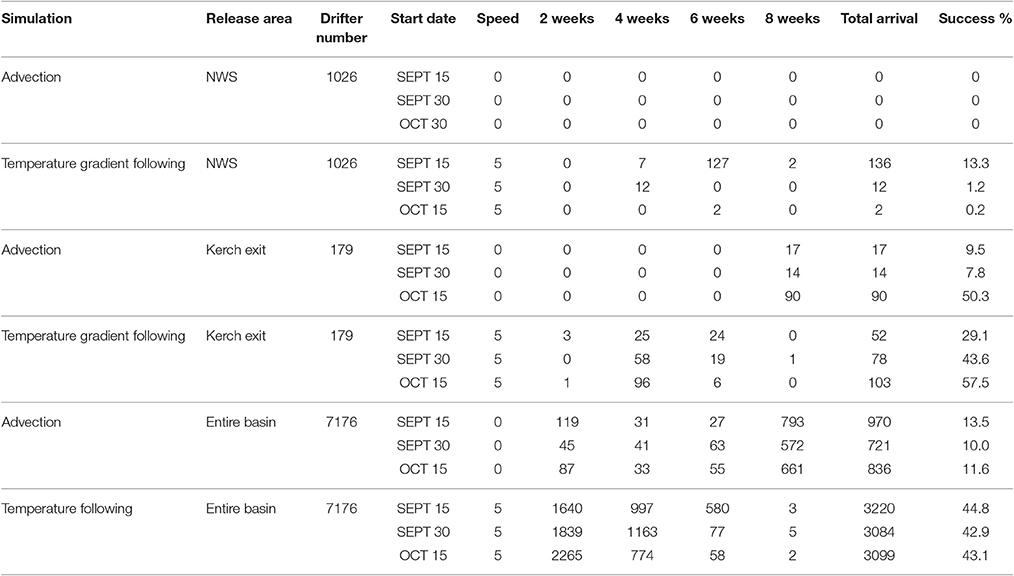
Table 4. Migration success of drifters released from the northwestern shelf and the Kerch Strait exit region on September 15th, 30th and October 15th 2003 in the advection only and the temperature gradient simulations with 5 bl/s swimming speed.
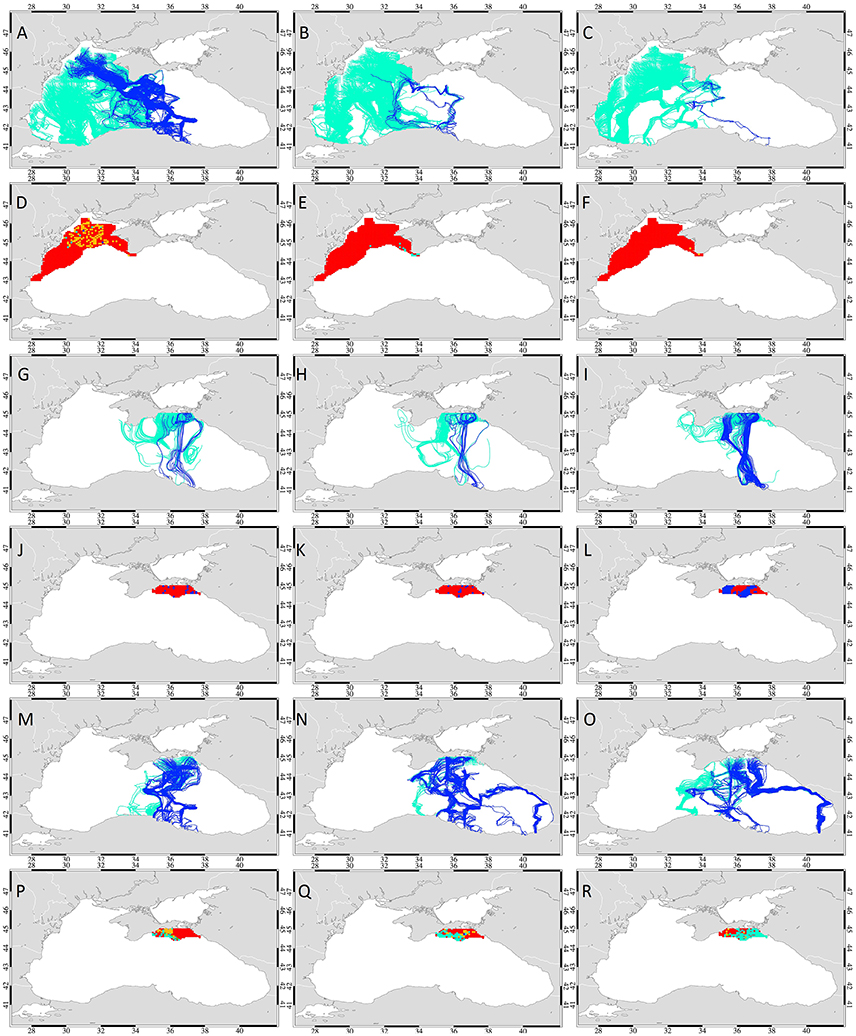
Figure 10. Simulations of seasonal variability: The paths (A–C) and start points (D–F) of drifters released on the northwestern shelf in temperature gradient simulations. Further, the paths of drifters (G–I) and start points (J–L) released at Kerch Strait exit area in the advection only simulations and the paths (M–O) and start points (P–R) of drifters released at Kerch Strait exit area in the temperature gradient simulations. Release times were September 15th, 30th and October 15th in 2003 (left, middle, and right column). The paths of drifters reaching the overwintering area in 2 months are marked blue, the ones that do not reach are in green. The start points of drifters that complete a successful migration in 2, 4, 6, and 8 weeks are color-coded green, cyan, yellow, and dark blue, respectively. The start points of the drifters that do not reach the overwintering grounds are marked red.
However, the drifters that started migration at the end of September (Figures 10B,E) or mid-October (Figures 10C,F) revealed a very low migration success of 0.2–21.2% (Table 4). This was mainly due to the intense cooling in the second half of October (weeks 3 and 4) that produced a strong northwest-southeast temperature gradient, remnants of which remained until the first week of November (Figure 6A). Drifters released then therefore did not move toward Crimea and then further south, but headed toward the warmer SST regions in the southeastern part of the western basin which was then the only accessible warm area in the western basin. Concurrently, the warm temperatures at the southern coast further retreated toward the Anatolian coastline and drifters arrived either at the central (Zonguldak—Inebolu) or western (Istanbul) coasts of Anatolia outside the overwintering area. Only very few drifters from the tip of the Crimean Peninsula completed successful migration then. Therefore, for drifters originating from the NWS in 2003, it was advantageous to approach the warmer southern basin prior to the start of cooling in October arriving at the SST hotspots as the cooling progressed.
When the drifters representing Azov anchovy were released from the Kerch region in mid and late September and mid-October in 2003 with only advection by currents, successful drifters followed a direct southward path completing migration in 8 weeks (Figures 10G–L, Table 4). Migration success was 7.8–9.5% for drifters discharged in mid/late September, less than half the success rate of the reference simulation (start date October 30). However, drifters released in mid-October displayed a 5-fold increased success rate with 50% reaching the overwintering area, 30% higher than the results of the reference simulation.
The inclusion of temperature gradient following behavior in the seasonal variability analysis of drifters released from Kerch exit region revealed a series of pathways for successful drifters due to the variability of SST distribution (Figures 10M–R). Migration time decreased to 6 and 4 weeks (Figures 10P–R, Table 4). Although decreased success rate occurred for those released mid- (29.1%) and late-September (43.6%), elevated migration success was found in the simulation starting mid-October with over half of the drifters (57.5%) completing migration to the overwintering area. However, that was still 35.2% less than the reference simulation (92.7%). This comparatively low success rate was again due to the cooling event at the end of October which caused the high SST regions in the southern basin to withdraw toward the Anatolian coastline and those in the south-eastern basin to retreat further. Drifters released on September 15th followed a single pathway (Figure 10M) moving southwards to the eastern inner basin through and around the Crimean eddy that carries warm waters northwards around its western edge. Drifters completed migration in 4 weeks following warm SST's extending from the Anatolian coast to the inner basin arriving at the western part of the overwintering area between Sinop and Samsun.
Following discharge on September 30th, the majority of successful drifters completed migration in 4 weeks originating from the southwestern shelf region at the exit of Kerch Strait (Figure 10Q). These drifters initially accumulated in the Crimean eddy region where they approached the inner basin. With the onset of cold SSTs approaching from the northwestern basin, a retreat of warm SSTs to the coasts of Rize and Batumi occurred thereby some drifters followed this gradient moving eastward toward the Caucasus coast (Sochi—Sukhumi) region and continued southwards from Sukhumi to Rize coast (by-passing the Batumi coast) to reach the eastern region Trabzon—Rize, of the overwintering area. Other drifters moved toward the Sinop—Fatsa region.
Successful drifters released on October 15, 2003 either moved eastward toward Sochi, following a southward route along the coast to the overwintering area or alternatively directly migrated south arriving in the Sinop region (Figures 10M,O). In addition, a few drifters moved westward along 43°N latitude to the western basin deviating southeastward toward the overwintering area to arrive at the Samsun—Carsamba region. This pattern was also previously observed by Chashchin et al. (2015), stating that in winter, a larger portion of the Azov anchovy stock occupy the northeastern basin, while the rest of the stock form dense aggregations in response to the northern wind observed in November and migrate along the east coast to warmer areas, overwintering along the Georgian coast in cold years.
Model Sensitivity
To analyze the sensitivity of model results to the body length of 10 cm specified in this study, a sensitivity analysis of how their migration success from the NWS is affected by body size was undertaken. The average length of migrating adult anchovy is known to be between 8.3 cm (if 0 age class is assumed to start migration) and 13.3 cm (Bilgin et al., 2016). The model simulations presented in the reliability test (Table 2) where a total of 1026 drifters were released over the North-western shelf on October 30th in 2001, 2002, and 2003 therefore cover the entire range of speeds that these different sized anchovies may be able to swim. Migration success of the smallest size (8.3 cm) therefore may vary between 0 and 1% when assuming 1 bl/s swimming speed, as it is unable to swim to the overwintering grounds as fast as the 10 cm anchovy (Table 2, 0–1.2% success). In addition, when traveling at 5 bl/s this anchovy class travels about 41.5 cm/s, which is in between the success of 10 cm anchovy at the speeds of 3 and 5 bl/s (30–50 cm/s, 0–27.1% success). The largest anchovies may reach 13.3 cm in length and hence their swimming ability surpasses the 10 cm anchovy. Despite the logical assumption that this makes the larger anchovy likely to be more successful than the 10 cm anchovy at 1 bl/s and at 5 bl/s, simulations showed that moving at 66.5 cm/s instead of 50 cm/s actually severely decreased migration success to 0–1.7%, comparable with the 1 bl/s simulation for the 10 cm anchovy (Table 2). This is because of the cooling event in November starting on the NWS in each year of the simulations whereby the ability to swim fast led drifters toward higher temperatures in the southwestern Black Sea coast, which were then isolated from the warmer waters in the southeastern Black Sea and hence missed the opportunity to migrate toward the warmest waters located in the southwestern Black Sea coast/Batumi region.
It can be concluded that the model is sensitive to the size of anchovy migrating as it translates effectively into migration speed. A smaller sized anchovy may be slightly less successful reaching overwintering grounds from the NWS, while a larger animal can possibly reach the grounds faster. However, as mentioned above, it is important to note that swimming speed alone does not determine migration success rate in the model but largely the temperature distribution in the Black Sea, as well as the prevailing currents at the time of migration. In 2001, the success rate was 1.2, 3.9, 12.2, and 1.3 % with 1, 3, 5, and 6.65 body-lengths per second swimming speed (Table 2) respectively and similarly, in 2003, the success rate was 0, 1, 15.3, and 1.7 % with speeds of 1, 3, 5, and 6.65 bl/s, respectively. During these 2 years, which at the same time are also characterized by moderate (2001) and high (2003) mesoscale variability in currents, the migration success increased with accelerating swimming speeds. Especially, increasing swimming speed from 3 to 5 bl/s improves the success rate by 8.3 (2001) and 14.3% (2003). The key to successful migration here was the timing and the extent of the northwest-southeast temperature gradient developing in the Black Sea during October due to cooling on the NWS (Figures 4, 6). However, in 2002, the year with lowest observed variability, the application of 1 and 5 bl/s swimming speeds reveal 0.3 and 0 % migration success, respectively, whereas 3 bl/s resulted in the highest migration success of 27.1% among all simulations in which the drifters were released from the NWS. At the same time, the mean distance traveled increased by 213.4, 342.5, and 286.1 km for all simulations using 3 bl/s. In 2002 the entire western basin cooled significantly (Figures 5A,C) and a strong west-east temperature gradient formed, rendering warm regions in the western basin unreachable by anchovy, no matter how high the swimming speed (Table 2). Therefore, while migration success in this model is sensitive to swimming speed which may also be dependent on body length, temperature distribution in the Black Sea as well as current flow can override this influence.
Discussion
Source of Anchovy Reaching Overwintering Region
In this study, model simulations were undertaken to elucidate the impact of environmental factors, such as sea surface temperature distribution and geostrophic surface flow on the overwintering migration of anchovy in the Black Sea. Simulations including anchovy behavior in the form of swimming along temperature gradients clearly showed that even with speeds of 5 bl/s, anchovy migration success rates for all 3 years studied were only between 0 and 15.3%, indicating that the spawning and nursery areas on the NWS are not likely to play a major role in supplying anchovy to overwintering grounds in this modeling approach within the time frame of the present study. Calculations for all three years of simulations revealed only 4.3% of anchovy originated from the NWS, 2.8% originating from a small area at the very north of the NWS and 1.5% from a larger area to the south. Simulations showed that 95.7% of anchovy arriving at the overwintering area originated from elsewhere in the Black Sea, more specifically from the eastern Black Sea (75%), the northern area of the eastern Black Sea including the Kerch Strait region (16%), and east of Crimea (4.7%) (Figure 11A). These findings suggest that anchovy overwintering at the eastern Anatolian coast originate mainly from the eastern Black Sea. These regions lie outside the traditionally accepted main spawning and nursery ground in the Black Sea (Figure 1), however spawning in the northeastern Black Sea is well-documented (Ivanov and Beverton, 1985; Chashchin, 1996; Chashchin et al., 2015). We hypothesize that if anchovy from the NWS cannot migrate successfully to the overwintering area in great numbers, but aggregations of migrating anchovy continue to arrive and are fished annually, this may mean that anchovy in the eastern Black Sea spawn successful enough to be able to sustain this overwintering population. This is also supported by studies indicating anchovy spawn successfully over much of the southern Black Sea (Einarsson and Gürtürk, 1960; Niermann et al., 1994; Kideys et al., 1999; Gucu et al., 2016).
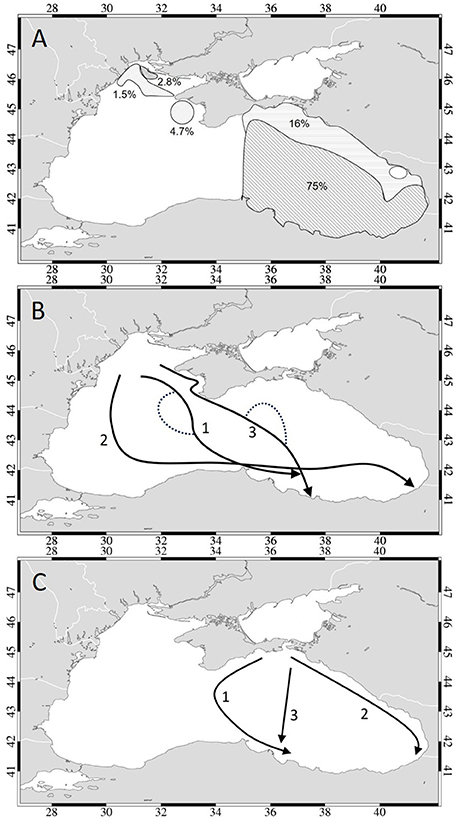
Figure 11. Conceptual figure of (A) the regions from where anchovy can successfully reach the overwintering area when swimming along temperature gradients indicating the success rate of migration (%) from each region, showing the migration pathways identified for (B) Black Sea anchovy released from the northwestern shelf and (C) Azov anchovy released from the Kerch exit region.
Anchovy Migration Pathways
For those anchovy schools that migrated successfully from the NWS, three different pathways were identified (Figure 11B): (1) movement from Crimea to the southern Black Sea coast midway between the western and eastern cyclonic gyres (in 2001 and 2003) and (2) through the western gyre (in 2003). An additional pathway (3) was identified within the eastern basin in which anchovy first traveled in the Crimea eddy, reaching the southern waters of the eastern basin by migrating across the eastern gyre (in 2003). Variations of these pathways appeared due to the yearly variability in gyres and eddies (Figure 11B, dashed lines). Except for the direct transport pathway between the eastern and western gyre, the identified pathways were seen to change on a yearly basis and were more complex than the migration routes suggested earlier by Ivanov and Beverton (1985) and Chashchin (1996) (see Figure 1).
It is important to note that migration along the Bulgarian/Romanian coasts did not appear in any of the temperature gradient simulations for different years and start dates, in contrast to Ivanov and Beverton (1985) and Chashchin et al. (2015) who state that the most important fall migration route is the one following the western coast of the Black Sea. Anchovy landing data (Figure 2) shows that the anchovy fisheries in Bulgaria and Romania collapsed alongside other anchovy fisheries in the Black Sea in 1989/90 (FAO, 2014; STECF, 2015). Whilst the Turkish anchovy fishery recovered within a few years to reach pre-collapse stock levels, so far there are no reported recovery signs for the Bulgarian/Romanian anchovy fisheries. It has been acknowledged the lack of recovery of the Bulgarian/Romanian fisheries may be in part due to reduced fishing efforts by those countries after becoming EU member states in 2007 and the subsequent application of EU fishing regulations (STECF, 2015; Gucu et al., 2017). However, the complete lack of recovery prior to 2007 has also been hypothesized to be partly due to either a change in spawning areas or changes in migration routes (STECF, 2015; Gucu et al., 2017).
The results of this study indicate that the environmental conditions of temperature and surface circulation during the study period (2001–2003) caused anchovy not to migrate along the Bulgarian-Romanian coasts at all when following temperature gradients, providing the temperature distribution in the Black Sea during autumnal cooling as possible explanation for why this route may be chosen less by migrating anchovy. It may even be possible that a shift in temperature distributions or currents in response to cooling events prevents recovery of this fishery since it caused anchovy to migrate to overwintering regions via open ocean pathways. However, to verify this hypothesis an investigation of long-term satellite data is needed. Furthermore, in warm years, anchovy overwintering along the Crimean coast or other northern areas on the shelf has been reported (Chashchin, 1996; Bingel and Gücü, 2010; Chashchin et al., 2015). Gucu et al. (2017) speculate that apart from inter-specific population dynamics, the actual reason for the “collapse” of the anchovy fishery (in 1989/90, 2007, and 2014) might be that by overwintering outside the known grounds and spending the winter further north in the Black Sea the anchovy was unavailable to the fishery in the southern basin. This points to the urgent need to clarify the prevailing physical conditions during the specific time of migration to be able to manage such fluctuations as has also been suggested by Chashchin (1996).
Anchovy originating off the Kerch Strait, assumed to be representing Azov anchovy in this study, were able to be transported to the overwintering area by currents alone following paths midway between the eastern and western gyre, which agrees well with previous studies (Fach, 2014; Ozturk et al., 2017). From temperature gradient simulations, three major pathways of Azov anchovy were identified (Figure 11C): (1) parallel to the eastern Crimean coast and then following the Eastern Gyre reaching the western region of the overwintering area (in 2001 and 2003), (2) diagonally from the eastern zone of the Kerch exit area to the western section of the overwintering area (across the Eastern Gyre) (in 2002), and a third path (3) parallel to the east coast against the flow of the rim current (that carries warm waters of the south northwards) (in 2001 and 2003). However, depending on the proximity of the Rim Current to the east coast, in 2001 the pathway is shifted offshore whereas in 2003 the pathway runs close to the shore and reaches the Georgian coast. These findings are in agreement with observations of Chashchin et al. (2015) who state that in cold years anchovy aggregations move along the Caucasian coast and approach the Turkish—Georgian border. In addition, migration toward the Crimean Peninsula is observed although it is thought to be less frequent and as a consequence more anchovy may be found at the Caucasus coast than the Crimean (Chashchin et al., 2015). However, our modeling results suggest that this pathway is dependent on the occurrence of a strong temperature gradient at the Kerch Strait region and the extension of warm water along the east coast and hence did not occur very frequently. Instead, southward migration in the inner basin between the two cyclonic gyres (pathway 1, Figure 11C) was observed in both 2001 and 2003, as well as in the seasonal simulations. However, the suggested migration toward the tip of the Crimean Peninsula by anchovy released from the Kerch Strait region was observed in most simulations of this study within the time frame considered, though was not marked as successful migration in the context of this study.
Importance of Cooling Events
In the simulations of movement following temperature gradients, the average travel time of anchovy schools starting migration from the NWS was found to be 2–4 weeks in 2001 but about 4–8 weeks in 2003. In both years, the SST gradient followed a northwest-southeast pattern that helps successful migration to the overwintering area, whereas in 2002 a more west-east SST gradient resulted in failure of migration. That was due to the short cooling period that took place in the second week of November 2002, when cold temperatures approached from the west and prevailed over the entire western basin (excepting the west coast of Anatolia). This incident ultimately decreased the anchovy's opportunity to find warm areas in the southwestern basin. Additionally, the presence of the Sevastopol eddy at the shelf break in 2001 and 2003 facilitated transport of drifters to the overwintering area by increasing thermal gradients in this frontal region. In 2003, the occurrence of unstable jets and filaments along the southern coast extending into the inner basin further increased successful migration.
It should be noted that in the simulations, anchovy migration was facilitated mainly by currents associated with mesoscale eddies along the coastal regions such as the Sevastopol eddy, the Crimean eddy and the anticyclonic eddy southeast of the Crimean eddy, Sinop eddy, Kizilirmak eddy, and Batumi eddy, as opposed to strong currents associated with the rim current. Often an increase in biological activity along such fronts is called the “edge-effect” and most fish have been found to accumulate at fronts (Roberts, 1980; Kleckner and McCleave, 1988; Castillo et al., 1996; Reese et al., 2011). Fish accumulations along fronts are driven by two factors, optimum temperature conditions and increased prey availability at the front (Owen, 1981; Largier, 1993).
The simulation results suggest that the most important factors shaping migration pathways, and their success, are the intensity and timing of cooling events more than the intensity of currents around eddies and the rim current because of their contribution to the formation of temperature gradients across the Black Sea which determine the pathways chosen by the anchovy. The analysis showed that the onset of migration long before intense cooling in October/November may increase migration success from the NWS region. When anchovy start migration later, direct pathways to the southeastern overwintering grounds may be inaccessible hence migration success decreases significantly. Cold winters caused by successive cold, dry wind outbreaks blowing from the northern sector of Euro-Asia associated with strong positive modes of NAO (Polonsky et al., 2007; Valchev et al., 2012) and EA-WR (Kazmin et al., 2010) lead to cold air temperatures and sea-water temperatures of below 6°C on the shelf (Mihailov et al., 2016).
The timing of the onset of cooling together with its distribution across the Black Sea may therefore be one of the mechanisms that can explain the large fluctuations in anchovy landings in addition to the effects of overfishing, such as those observed in 2005 and 2014 (Figure 2A) when catches were below 120 ktons, close to the dramatic decline in catch levels of the 1989/1990 period. In 2005, Chashchin et al. (2015) reported accumulations of Black Sea anchovy south of Crimea during the relatively warm winter, which could help explain low catches in the south. Corresponding movement toward south Crimea is observed in all model simulations and when no warm water leads the anchovy south-ward, the model anchovy similarly stay in this region. Regular observation of this migration pathway supports the hypothesis that stock fluctuations may partially be caused by shifts in environmental conditions and consequently possible shifts in overwintering areas.
The ongoing warming trend in the oceanic environment will inevitably impact species distribution and migratory pathways that are dependent on temperature as an external stimulus. A shift in species distribution has already been observed for some fish species in the world's oceans (Dulvy et al., 2000; Genner et al., 2004; Perry et al., 2005; Nye et al., 2009; Punzón and Villamor, 2009; Simpson et al., 2011; Sunday et al., 2015). For Black Sea and Azov Sea anchovy this may mean two things: (1) a shift in spawning grounds to previously unsuitable regions or (2) a delay in the start of overwintering migration or even a halt of migration if the intensity and/or duration of cooling events do not decrease the temperature to a level sufficiently low to commence migration. In this case, it is likely that school formation would cease and anchovy remain dispersed in spawning/nursery areas. This would result in a shift in fishing grounds and fishing effort as dispersed anchovy becomes no longer a profitable target for exploitation by industrial fisheries. Gucu et al. (2016) already suggest the existence of local, non-migrating anchovy populations in the southern Black Sea basin, an area that was previously thought less suitable for spawning. However, the response of fish populations to continued warming is rather complex and non-linear (Guraslan et al., 2014), as anchovy metabolism, spawning and recruitment success are temperature dependent.
It should be noted that the model applied in this study has uncertainties associated with it, such as those resulting from a fixed swimming speed of 5bl/s during the entire migration. Anchovy swimming speeds may vary depending on conditions during migration. Or anchovy may swim on average 3 bl/s for extended periods of time (Gucu et al., 2017), however the model results discussed above do not change significantly in simulations considering this lower swimming speed (Guraslan, 2016). In addition, simulations were run for 2 months, overlooking longer migration times. While this is a valid assumption, as it has been shown that Black Sea anchovy migration times lie well within that time frame (Gucu et al., 2017), variability in migration times is likely. In addition, lack of data on the cues that influence migration is a big factor creating uncertainties in fish movement models (Haas et al., 2004; Roth et al., 2008) which is especially true for Black Sea anchovy migration. Watkins and Rose (2013), suggest in their study evaluating different animal movement models that gradient following behavior, as implemented in the current study, produced the best fish movement analysis in all tested environments, also confirmed by Politikos et al. (2015). This approach has also been proven successful in other studies (e.g., Railsback et al., 1999; Xu et al., 2013). However, Watkins and Rose (2013) also note that the assumptions that fish are able to sense environmental conditions in the neighboring model cells are sometimes questionable. To reduce uncertainties in the modeling approach, it is of importance to validate the model in greater detail than possible within the current study, including detailed density distributions of anchovy during different seasons. However, at this time the modeling community is limited by the lack of available data. One future development may be to include genetic algorithms in an effort to adaptively optimize parameters known to influence migration using artificial evolution (Strand et al., 2002; Utne and Huse, 2012; Watkins and Rose, 2013).
Conclusion
In this study, we demonstrate that the Lagrangian modeling approach including fish behavior applied to satellite derived circulation and temperature data can be used to explore the migration of complex organisms such as fish in the marine environment. The modeled anchovy migration pathways are in agreement with general patterns of anchovy migration given in the literature indicating that the physical environment may be a major factor in shaping general migration patterns. Results of model simulations suggest that most anchovy reaching the overwintering area along the eastern Anatolian coast may originate from the eastern Black Sea and not from the northwestern shelf, which has been traditionally assumed to be the main source of overwintering anchovy in the southeastern shelf area. Migration simulation results are used to hypothesize that there may be alternative migration routes to those traditionally accepted, that are caused by environmental variability in the Black Sea, such as the timing and progression of autumnal cooling together with current strength. Such alternative routes can help explain the low catch of the Bulgarian and Romanian fisheries.
The present work shows in detail, how physical processes such as the timing of fall cooling and the intensity of currents and mesoscale eddies can play a critical role in modifying the highly variable small pelagic fish migratory pathways, their migration success and their availability to fisheries given the interannual and seasonal variability. Physical dynamics may be an important factor explaining the strong interannual variability of anchovy catches. For ecosystem-based marine resource management strategies, it is therefore of great importance to understand the variability in parameters of the physical environment and related uncertainties. This study also demonstrates that modeling is a valuable tool in understanding the processes of fish migration, which is difficult to observe with conventional methods. With this tool, complex processes of environmental variability and the impact on migration success of anchovy can be explored to help predict the timing and success of migration for different years which is of crucial importance to fisheries management.
Currently, the modeling approach of this study includes the influence of the physical environment, while the quality of overwintering grounds, adaptive, schooling and homing behavior is neglected. A limitation of this approach is that model results could not be validated against detailed, long-term anchovy survey data for lack of availability. Efforts to develop coupled hydrodynamic-ecosystem models of the Black Sea environment are underway (e.g., Cannaby et al., 2015) as are models that include food-web interactions to derive fisheries management advice (Akoglu et al., 2015; Salihoglu et al., in review). Application of the fish movement model presented here with a fully coupled hydrodynamic-ecosystem model, may be better able to address not only overwintering but also spawning migration behavior exhibited by anchovy in the Black Sea. However, the modeling community is limited by dependable observations. Reliable data on anchovy distribution for model validation as well as data on cues that may influence migration and selection of preferable habitat is crucial in order to reduce the uncertainty of this modeling approach and of future fish movement models to be developed to propose management schemes.
Author Contributions
BF and TO conceived and designed the research, BF and CG designed the model. CG implemented and analyzed the model and led the writing of the paper. All authors contributed significantly to the writing of the paper.
Funding
This study was partially supported by The Scientific and Technological Research Council of Turkey (TUBITAK) 2211-C graduate scholarship program awarded to CG, the EU 7th Framework project CoCoNET (contract no. 287844), as well as partial funding by KAMAG-110G124 project funded by The Scientific and Technological Research Council of Turkey (TUBITAK). The authors acknowledge support by DEKOSIM (BAP-08-11-DPT2012K120880) funded by the Turkish Ministry of Development.
Conflict of Interest Statement
The authors declare that the research was conducted in the absence of any commercial or financial relationships that could be construed as a potential conflict of interest.
Acknowledgments
The authors would like to thank Prof. A. C. Gucu for fruitful discussions and guidance during this study. The authors gratefully acknowledge Alison Kideys for English language editing the manuscript.
References
Akoglu, E., Libralato, S., Salihoglu, B., Oguz, T., and Solidoro, C. (2015). EwE-F 1.0: an implementation of Ecopath with Ecosim in Fortran 95/2003 for coupling. Geosci. Model Dev. 8, 1511–1537. doi: 10.5194/gmdd-8-1511-2015
Auckland, R., and Reid, D. G. (1998). The Impact of Changing Stock Size on the Aggregative Behaviour of North Sea Herring, ICES Document CM 1998/J: 2, 19.
Bennett, J. R., and Clites, A. H. (1987). Accuracy of trajectory calculation in a finite-difference circulation model. J. Comput. Phys. 68, 272–282. doi: 10.1016/0021-9991(87)90058-1
Berdnikov, S. V., Selyutin, V. V., Vasilchenko, V. V., and Caddy, J. F. (1999). Trophodynamic model of the Black and Azov Sea pelagic ecosystem: consequences of the comb jelly, Mnemiopsis leydei, invasion. Fish Res. 42, 261–289. doi: 10.1016/S0165-7836(99)00049-1
Bilgin, S., Sumer, C., Bektas, S., Satilmis, H. H., and Bircan, R. (2016). Evaluation of anchovy (Engraulis encrasicolus) population dynamics studies (1985-2015) in terms of fisheries management in the Black Sea. Ege J. Fish. Aquat. Sci. 33, 169–182. doi: 10.12714/egejfas.2016.33.2.12
Bingel, F., and Gücü, A. C. (2010). “Black Sea anchovy and stock assessment,” in Proceedings of the Sustainable Fisheries Workshop, National Anchovy Workshop, (Trabzon), 38–57.
Buongiorno Nardelli, B., Colella, S., Santoleri, R., Guarracino, M., and Kholod, A. (2010). A re-analysis of Black Sea surface temperature. J. Mar. Syst. 79, 50–64. doi: 10.1016/j.jmarsys.2009.07.001
Cannaby, H., Fach, B. A., Arkin, S. S., and Salihoglu, B. (2015). Climatic controls on biophysical interactions in the Black Sea under present day conditions and a potential future (A1B) climate scenario. J. Mar. Syst. 141, 149–166. doi: 10.1016/j.jmarsys.2014.08.005
Capet, A., Barth, A., Beckers, J. M., and Marilaure, G. (2012). Interannual variability of Black Sea's hydrodynamics and connection to atmospheric patterns. Deep Sea Res. II 77–80, 128–142. doi: 10.1016/j.dsr2.2012.04.010
Castilla-Espino, D., García-del-Hoyo, J. J., Metreveli, M., and Bilashvili, K. (2014). Fishing capacity of the southeastern Black Sea anchovy fishery. J. Mar. Syst. 135, 160–169. doi: 10.1016/j.jmarsys.2013.04.013
Castillo, J., Barbieri, M. A., and Gonzalez, A. (1996). Relationships between sea surface temperature, salinity, and pelagic fish distribution off northern Chile. ICES J. Mar. Sci. 53, 139–146. doi: 10.1006/jmsc.1996.0014
Chashchin, A. K., and Akselev, O. I. (1990). “Migrations of the stocks and availability of Black Sea anchovy to the fishery to the autumn and winter,” in Biological Resources of the Black Sea, ed V. A. Shlyakhov (Moscow: VNIRO), 80–93.
Chashchin, A., Shlyakhov, V. A., Dubovik, V. E., and Negoda, S. (2015). “Stock assessment of anchovy (Engraulis encrasicolus L.) in Northern Black Sea and Sea of Azov,” in Progressive Engineering Practices in Marine Resource Management Stock Assessment of Anchovy (Engraulis encrasicolus L.) in Northern Black Sea and Sea of Azov, eds I. Zlateva, V. Raykov, and N. Nikolov (Hershey, PA: IGI Global), 209–243.
Checkley, D. M. Jr., Asch, R. G., and Rykaczewski, R. R. (2017). Climate, Anchovy, and Sardine. Ann. Rev. Mar. Sci. 9, 469–493. doi: 10.1146/annurev-marine-122414-033819
Constable, A. J., Melbourne-Thomas, J., Corney, S. P., Arrigo, K. R., Barbraud, C., Barnes, D. K., et al. (2014). Climate change and Southern Ocean ecosystems I: how changes in physical habitats directly affect marine biota. Glob. Chan. Biol. 20, 3004–3025. doi: 10.1111/gcb.12623
Daskalov, G. M. (2003). Long-term changes in fish abundance and environmental indices in the Black Sea. Mar. Ecol. Prog. Ser. 255, 259–270. doi: 10.3354/meps255259
Dommasnes, A., and Røttingen, I. (1984). “Acoustic stock measurement of the Barents Sea capelin 1972-1984. A review,” in The proceedings of the Soviet-Norwegian Symposium on the Barents Sea Capelin, ed H. Gjosxter (Bergen: Institute of Marine Research), 45–108.
Dulvy, N. K., Metcalfe, J. D., Glanville, J., Pawson, M. G., and Reynolds, J. D. (2000). Fishery stability, local extinctions, and shifts in community structure in skates. Conserv. Biol. 14, 283–293. doi: 10.1046/j.1523-1739.2000.98540.x
Einarsson, H., and Gürtürk, N. (1960). Abundance and Distribution of Eggs and Larvae of Anchovy (Engraulis Encrasicolus Ponticus) in the Black Sea. Publishing Hydrobiology Research. Ins., Faculty of Sciences, University of Istanbul, 71–94.
Fach, B. A. (2014). Modeling the influence of hydrodynamic processes on anchovy distribution and connectivity in the black sea. Turk. J. Fish. Aquat. Sci. 14, 353–365. doi: 10.4194/1303-2712-v,14_2_06
FAO (2014). Fishery and Aquaculture Statistics. GFCM Capture Production Statistics 1970-2014, FAO Fish. Aquaculture Department (Rome). Available online at: http://www.fao.org/fishery/statistics/en
Genner, M. J., Sims, D. W., Wearmouth, V. J., Southall, E. J., Southward, A. J., Henderson, P. A., et al. (2004). Regional climatic warming drives long–term community changes of British marine fish. Proc. R. Soc. Lond. B Biol. Sci. 271, 655–661. doi: 10.1098/rspb.2003.2651
Gjøsæter, H. (1998). The population biology and exploitation of capelin (Mallotus villosus) in the Barents Sea. Sarsia 83, 453–496. doi: 10.1080/00364827.1998.10420445
Gjøsæter, H., Dommasnes, A., and Røttingen, B. (1998). The Barents Sea Capelin stock 1972–1997. A synthesis of results from acoustic surveys. Sarsia 83, 497–510. doi: 10.1080/00364827.1998.10420446
Goodwin, R. A., Nestler, J. M., Anderson, J. J., Weber, L. J., and Loucks, D. P. (2006). Forecasting 3-D fish movement behavior using a Eulerian-Lagrangian-agent method (ELAM). Ecol. Model. 192, 197–223. doi: 10.1016/j.ecolmodel.2005.08.004
Goulding, I. C., Stobberup, K. A., and O'Higgins, T. (2014). Potential economic impacts of achieving good environmental status in Black Sea fisheries. Ecol. Soc. 19, 32. doi: 10.5751/ES-06817-190332
Gucu, A. C., Genc, Y., Dagtekin, M., Sakinan, S., Ak, O., Ok, M., et al. (2017). On Black Sea Anchovy and Its Fishery. Rev. Fish. Sci. Aquac. 25, 230–244. doi: 10.1080/23308249.2016.1276152
Gucu, A. C., Inanmaz, O. E., Ok, M., and Sakinan, S. (2016). Recent changes in the spawning grounds of Black Sea anchovy, Engraulis encrasicolus. Fish. Ocean. 25, 67–84. doi: 10.1111/fog.12135
Guizien, K., Brochier, T., Duchêne, J. C., Koh, B. S., and Marsaleix, P. (2006). Dispersal of Owenia fusiformis larvae by wind-driven currents: turbulence, swimming behaviour and mortality in a three-dimensional stochastic model. Mar. Ecol. Prog. Ser. 311, 47–66. doi: 10.3354/meps311047
Guraslan, C. (2016). Modeling the Impact of Climate Variability on Anchovy Overwintering Migration in the Black Sea. Dissertation. Middle East Technical University (Erdemli).
Guraslan, C., Fach, B. A., and Oguz, T. (2014). Modeling the impact of climate variability on Black Sea anchovy recruitment and production. Fish. Ocean. 23, 436–457. doi: 10.1111/fog.12080
Haas, H., Rose, K. A., Fry, B., Minello, T. J., and Rozas, L. (2004). Brown shrimp on the edge: linking habitat to survival using an individual-based simulation model. Ecol. Appl. 14, 1232–1247. doi: 10.1890/03-5101
Hare, S. R., Mantua, N. J., and Francis, R. C. (1999). Inverse production regimes: alaska and west coast pacific salmon. Fisheries 24, 6–14. doi: 10.1577/1548-8446(1999)024<0006:IPR>2.0.CO;2
Harte, P. (2002). Toward a synthesis of the Newtonian and Darwinian worldviews. Phys. Today 55, 29–34. doi: 10.1063/1.1522164
Heath, M. R., and Gallego, A. (1998). Bio-physical modelling of the early life stages of haddock, Melanogrammus aeglefinus, in the North Sea. Fish. Ocean. 7, 110–125. doi: 10.1046/j.1365-2419.1998.00061.x
Hofmann, E. E., and Powell, T. M. (1998). Environmental variability effects on marine fisheries: four case histories. Ecol. Appl. 8, 23–32. doi: 10.1890/1051-0761(1998)8[S23:EVEOMF]2.0.CO;2
Huse, G., and Ellingsen, I. (2008). Capelin migrations and climate change–a modelling analysis. Clim. Change 87, 177–197. doi: 10.1007/s10584-007-9347-z
Huse, G., Fernö, A., and Holst, J. C. (2010). Establishment of new wintering areas in herring co-occurs with peaks in the “first time/repeat spawner” ratio. Mar. Ecol. Prog. Ser. 409, 189–198. doi: 10.3354/meps08620
Huse, G., Johansen, G. O., Bogstad, B., and Gjøsæter, H. (2004). Studying spatial and trophic interactions between capelin and cod using individual-based modelling. ICES J. Mar. Sci. 61, 1201–1213. doi: 10.1016/j.icesjms.2004.06.011
Ivanov, L., and Beverton, R. J. H. (1985). The Fisheries Resources of the Mediterranean. pt. 2: Black Sea in: Studies and Reviews-General Fisheries Council for the Mediterranean (FAO), Rome: FAO, 147.
Ivanov, V. A., Kukushkin, A. S., and Samodurov, A. S. (1985). Sixth cruise of the R/V Prof. Kolesnikov in the Aegean Sea and Black Sea (June 24, August 25, 1983). Oceanology 25, 278–281.
Iversen, S. A. (2002). Changes in the perception of the migration pattern of Northeast Atlantic mackerel during the last 100 years. ICES J. Mar. Sci. 215, 382–390.
Kazmin, A. S., Zatsepin, A. G., and Kontoyiannis, H. (2010). Comparative analysis of the long-term variability of winter surface temperature in the Black and Aegean Seas during 1982–2004 associated with the large-scale atmospheric forcing. Int. J. Clim. 30, 1349–1359. doi: 10.1002/joc.1985
Kideys, A. E., Gordina, A. D., Bingel, F., and Niermann, U. (1999). The effect of environmental conditions on the distribution of eggs and larvae of anchovy (Engraulis encrasicolus L.) in the Black Sea. ICES J. Mar. Sci. 56, 58–64. doi: 10.1006/jmsc.1999.0605
Kideys, A. E., Kovalev, A. V., Shulman, G., Gordina, A., and Bingel, F. (2000). A review of zooplankton investigations of the Black Sea over the last decade. J. Mar. Syst. 24, 355–371. doi: 10.1016/S0924-7963(99)00095-0
Kleckner, R. C., and McCleave, J. D. (1988). The northern limit of spawning by Atlantic eels (Anguilla spp.) in the Sargasso Sea in relation to thermal fronts and surface water masses. J. Mar. Res. 46, 647–667. doi: 10.1357/002224088785113469
Korotaev, G., Oguz, T., Nikiforov, A., and Koblinsky, C. (2003). Seasonal, interannual, and mesoscale variability of the Black Sea upper layer circulation derived from altimeter data. J. Geophys. Res. 108:3122. doi: 10.1029/2002JC001508
Largier, J. L. (1993). Estuarine fronts: how important are they? Estuar. Coasts 16, 1–11. doi: 10.2307/1352760
Lett, C., Veitch, J., Van Der Lingen, C. D., and Hutchings, L. (2007). Assessment of an environmental barrier to transport of ichthyoplankton from the southern to the northern Benguela ecosystems. Mar. Ecol. Prog. Ser. 347, 247–259. doi: 10.3354/meps06982
Lisovenko, L. A., and Andrianov, D. P. (1996). Reproductive biology of anchovy (Engraulis encrasicolus pontics Alexandrov 1927) in the Black Sea. Sci. Mar. 60, 209–218.
Marinone, S. G., Ulloa, M. J., Parés-Sierra, A., Lavín, M. F., and Cudney-Bueno, R. (2008). Connectivity in the northern Gulf of California from particle tracking in a three-dimensional numerical model. J. Mar. Syst. 71, 149–158. doi: 10.1016/j.jmarsys.2007.06.005
McQuatters-Gollop, A., Mee, L. D., Raitsos, D. E., and Shapiro, G. I. (2008). Non-linearities, regime shifts and recovery: the recent influence of climate on Black Sea chlorophyll. J. Mar. Syst. 74, 649–658. doi: 10.1016/j.jmarsys.2008.06.002
Mihailov, M. E., Stefan, S., Diaconu, V., and Lazar, L. (2016). Longterm variability of the water mass structure on the Romanian Black Sea shelf. Roman. Rep. Phys. 68, 377–392.
Miller, C. B., Lynch, D. R., Carlotti, F., Gentleman, W., and Lewis, C. V. W. (1998). Coupling of an individual-based population dynamic model of Calanus finmarchicus to a circulation model for the Georges Bank region. Fish. Ocean. 7, 219–234. doi: 10.1046/j.1365-2419.1998.00072.x
Niermann, U., Bingel, F., Gorban, A., Gordina, A. D., Gucu, A. C., Kideys, A. E., et al. (1994). Distribution of anchovy eggs and larvae (Engraulis encrasicolus Cuv.) in the Black Sea in 1991–1992. ICES J. Mar. Sci. 51, 395–406. doi: 10.1006/jmsc.1994.1041
Nikolsky, V. N. (2013). Application of esterase polymorphism to specify population genetic structure of Engraulis encrasicolus (Pisces: Engraulidae) in the Black and Azov Seas. Morskyi Ekolohichnyi Zhurnal 12, 45.
Nye, J. A., Link, J. S., Hare, J. A., and Overholtz, W. J. (2009). Changing spatial distribution of fish stocks in relation to climate and population size on the Northeast United States continental shelf. Mar. Ecol. Prog. Ser. 393, 111–129. doi: 10.3354/meps08220
Oguz, T., Akoglu, E., and Salihoglu, B. (2012). Current state of overfishing and its regional differences in the Black Sea. Ocean Coast. Man. 58, 47–56. doi: 10.1016/j.ocecoaman.2011.12.013
Oguz, T., and Besiktepe, S. (1999). Observations on the Rim Current structure, CIW formation and transport in the western Black Sea. Deep Sea Res. I Oceanogr. Res. Pap. 46, 1733–1753. doi: 10.1016/S0967-0637(99)00028-X
Oguz, T., Aubrey, D., Latun, V., Demirov, E., Kolesnikov, L., Sur, H., et al. (1994). Mesoscale circulation and thermohaline structure of the Black Sea observed during HydroBlack'91. Deep Sea Res. I Oceanogr. Res. Pap. 41, 603–628. doi: 10.1016/0967-0637(94)90045-0
Oguz, T., Salihoglu, B., and Fach, B. A. (2008a). A coupled plankton-anchovy population dynamics model assessing nonlinear controls of anchovy and gelatinous biomass in the Black Sea. Mar. Ecol. Prog. Ser. 369, 229–256. doi: 10.3354/meps07540
Oguz, T., Fach, B. A., and Salihoglu, B. (2008b). Invasion dynamics of the alien ctenophore Mnemiopsis leidyi and its impact on anchovy collapse in the Black Sea. J. Plank. Res. 30, 1385–1397. doi: 10.1093/plankt/fbn094
Owen, R. (1981). “Fronts and eddies in the sea: mechanisms, interactions and biological effects,” in Analysis of Marine Ecosystems, ed A. R. Longhurst (London: Academic Press), 197–233.
Ozhigin, V. K., and Luka, G. I. (1985). “Some peculiarities of capelin migrations depending on thermal conditions in the Barents Sea,” in The Proceedings of the Soviet–Norwegian Symposium on the Barents Sea Capelin (Bergen: Institute of Marine Research), 135–147.
Ozturk, B., Fach, B. A., Keskin, C., Arkin, S., Topaloglu, B., and Ozturk, A. A. (2017). Prospects for Marine Protected Areas in the Turkish Black Sea. Managem. MPAs: A Network Perspective, 247–262.
Panov, B. N., and Chashchin, A. K. (1990). Aspects of the water structure dynamics in the southeastern Black Sea as prerequisites for the formation of winter aggregations of Black Sea anchovy off the coast of Georgia. Oceanology 30, 242–247.
Panov, B. N., and Spiridonova, E. O. (1998). Hydro-meteorological prerequisites of the formation of commercial aggregations and migrations of the anchovy in the southeastern part of the Black Sea. Oceanology 38, 518–529.
Parada, C., Van Der Lingen, C. D., Mullon, C., and Penven, P. (2003). Modelling the effect of buoyancy on the transport of anchovy (Engraulis capensis) eggs from spawning to nursery grounds in the southern Benguela: an IBM approach. Fish. Ocean. 12, 170–184. doi: 10.1046/j.1365-2419.2003.00235.x
Pelletier, D., and Parma, A. M. (1994). Spatial distribution of Pacific halibut (Hippoglossus stenolepis): an application of geostatistics to longline survey data. Can. J. Fish. Aquat. Sci. 51, 1506–1518. doi: 10.1139/f94-150
Peraltilla, S., and Bertrand, S. (2014). In situ measurements of the speed of Peruvian anchovy schools. Fish. Res. 149, 92–94. doi: 10.1016/j.fishres.2013.09.002
Perry, A. L., Low, P. J., Ellis, J. R., and Reynolds, J. D. (2005). Climate change and distribution shifts in marine fishes. Science 308, 1912–1915. doi: 10.1126/science.1111322
Petitgas, P., Reid, D., Carrera, P., Iglesias, M., Georgakarakos, S., Liorzou, B., et al. (2001). On the relation between schools, clusters of schools, and abundance in pelagic fish stocks. ICES J. Mar. Sci. 58, 1150–1160. doi: 10.1006/jmsc.2001.1130
Politikos, D. V., Huret, M., and Petitgas, P. (2015). A coupled movement and bioenergetics model to explore the spawning migration of anchovy in the Bay of Biscay. Ecol. Model. 313, 212–222. doi: 10.1016/j.ecolmodel.2015.06.036
Polonsky, A. B., Bardin, M. Y., and Voskresenskaya, E. N. (2007). Statistical characteristics of cyclones and anticyclones over the Black Sea in the second half of the 20th century. Phys. Oceanogr. 17, 348–359. doi: 10.1007/s11110-008-9002-x
Punzón, A., and Villamor, B. (2009). Does the timing of the spawning migration change for the southern component of the Northeast Atlantic Mackerel (Scomber scombrus, L. 1758)? An approximation using fishery analyses. Cont. Shel. Res. 29, 1195–1204. doi: 10.1016/j.csr.2008.12.024
Railsback, S. F., Lamberson, R. H., Harvey, B. C., and Duffy, W. E. (1999). Movement rules for individual-based models of stream fish. Ecol. Model. 123, 73–89. doi: 10.1016/S0304-3800(99)00124-6
Reese, D. C., O'Malley, R. T., Brodeur, R. D., and Churnside, J. H. (2011). Epipelagic fish distributions in relation to thermal fronts in a coastal upwelling system using high-resolution remote-sensing techniques. ICES J. Mar. Sci. 68, 1865–1874. doi: 10.1093/icesjms/fsr107
Roberts, P. E. (1980). Surface distribution of albacore tuna, Thunnus alalunga Bonnaterre, in relation to the subtropical convergence zone east of New Zealand. N. Z. J. Mar. Fresh. Res. 14, 373–380. doi: 10.1080/00288330.1980.9515879
Roth, B. M., Rose, K. A., Rozas, L. P., and Minello, T. J. (2008). Relative influence of habitat fragmentation and inundation on brown shrimp Farfantepenaeus aztecus production in northern Gulf of Mexico salt marshes. Mar. Ecol. Prog. Ser. 359, 185–202. doi: 10.3354/meps07380
STECF (2015). Scientific, Technical and Economic Committee for Fisheries (STECF)–Black Sea Assessments (STECF-15-16). Luxembourg, EUR 27517 EN, JRC 98095: Publications Office of the European Union. 284
Satilmis, H. H., Gordina, A. D., Bat, L., Bircan, R., Culha, M., Akbulut, M., et al. (2003). Seasonal distribution of fish eggs and larvae off Sinop (the southern Black Sea) in 1999-2000. Acta Oecol. 24, 275–280. doi: 10.1016/S1146-609X(03)00022-5
Shulman, G. E. (2002). Anchovies of the Azov and the Black Sea: regularities of wintering migrations. Mar. Ecol. J. 1, 67–77.
Shulman, G. E., Nikolsky, V. N., Yuneva, T. V., Shchepkina, A. M., Bat, L., and Kideys, A. (2008). Significance of physiological and biochemical approaches for Black Sea fishery investigations. Int. J. Nat. Eng. Sci. 2, 107–112.
Simpson, S. D., Jennings, S., Johnson, M. P., Blanchard, J. L., Schön, P. J., Sims, D. W., et al. (2011). Continental shelf-wide response of a fish assemblage to rapid warming of the sea. Curr. Biol. 21, 1565–1570. doi: 10.1016/j.cub.2011.08.016
Strand, E., Huse, G., and Giske, J. (2002). Artificial evolution of life history and behavior. Am. Nat. 159, 624–644. doi: 10.1086/339997
Sunday, J. M., Pecl, G. T., Frusher, S., Hobday, A. J., Hill, N., Holbrook, N. J., et al. (2015). Species traits and climate velocity explain geographic range shifts in an ocean-warming hotspot. Ecol. Lett. 18, 944–953. doi: 10.1111/ele.12474
Tameishi, H. (1996). Understanding Japanese sardine migrations using acoustic and other aids. ICES J. Mar. Sci. 53, 167–171. doi: 10.1006/jmsc.1996.0018
Tu, C. Y., Tsenf, Y. H., Chiu, T. S., Shen, M. L., and Hsieh, C. H. (2012). Using coupled fish behavior–hydrodynamic model to investigate spawning migration of Japanese anchovy, Engraulis japonicus, from the East China Sea to Taiwan. Fish. Ocean. 21, 255–268. doi: 10.1111/j.1365-2419.2012.00619.x
TUIK (2013). Turkish Statistical Institute) Fisheries Statistics Report, Ankara: Publication number: 4349. TUIK.
Turchin, P., and Omland, K. S. (1999). “Migration and movement,” in Ecological Entomology, 2nd Edn. eds C. B. Huffaker and A. P. Gutierrez (New York, NY: John Wiley & Sons), 463–502.
Utne, K. R., and Huse, G. (2012). Estimating the horizontal and temporal overlap of pelagic fish distribution in the Norwegian Sea using individual-based modelling. Mar. Biol. Res. 8, 548–567. doi: 10.1080/17451000.2011.639781
Valchev, N. N., Trifonova, N. K., and Andreeva, N. K. (2012). Past and recent trends in the western Black Sea storminess. Nat. Hazards Earth Syst. Sci. 12, 961–977. doi: 10.5194/nhess-12-961-2012
Wang, Y., Wei, H., and Kishi, M. J. (2013). Coupling of an individual-based model of anchovy with lower trophic level and hydrodynamic models. J. Ocean Uni. China 12, 45–52. doi: 10.1007/s11802-013-1901-x
Watkins, K. S., and Rose, K. A. (2013). Evaluating the performance of individual-based animal movement models in novel environments. Ecol. Model. 250, 214–234. doi: 10.1016/j.ecolmodel.2012.11.011
Xu, Y., Chai, F., Rose, K. A., Ñiquen, C. M., and Chavez, F. P. (2013). Environmental influences on the inter-annual variation and spatial distribution of Peruvian anchovy (Engraulis ringens) population dynamics from 1991 to 2007: a three-dimensional modeling study. Ecol. Model. 264, 64–82. doi: 10.1016/j.ecolmodel.2013.01.009
Zwolinski, J. P., Demer, D. A., Byers, K. A., Cutter, G. R., Renfree, J. S., Sessions, T. S., et al. (2012). Distributions and abundances of Pacific sardine (Sardinops sagax) and other pelagic fishes in the California Current Ecosystem during spring 2006, 2008, and 2010, estimated from acoustic-trawl surveys. Fish. Bull. 110, 110–122.
Keywords: Black Sea, anchovy (Engraulis encrasicolus), overwintering migration, climate variability, Common Fisheries Policy
Citation: Guraslan C, Fach BA and Oguz T (2017) Understanding the Impact of Environmental Variability on Anchovy Overwintering Migration in the Black Sea and its Implications for the Fishing Industry. Front. Mar. Sci. 4:275. doi: 10.3389/fmars.2017.00275
Received: 28 February 2017; Accepted: 10 August 2017;
Published: 29 August 2017.
Edited by:
Massimiliano Cardinale, Swedish University of Agricultural Sciences, SwedenReviewed by:
Otello Giovanardi, Istituto Superiore per la Protezione e la Ricerca Ambientale, ItalyGeorgi M. Daskalov, Institute of Biodiversity and Ecosystem Research (BAS), Bulgaria
Copyright © 2017 Guraslan, Fach and Oguz. This is an open-access article distributed under the terms of the Creative Commons Attribution License (CC BY). The use, distribution or reproduction in other forums is permitted, provided the original author(s) or licensor are credited and that the original publication in this journal is cited, in accordance with accepted academic practice. No use, distribution or reproduction is permitted which does not comply with these terms.
*Correspondence: Ceren Guraslan, Y2VyZW5AaW1zLm1ldHUuZWR1LnRy