- 1Department of Earth and Environmental Sciences, Columbia University, New York, NY, USA
- 2Division of Biology and Paleo Environment, Lamont-Doherty Earth Observatory, Columbia University, New York, NY, USA
We investigated the dynamics of heterotrophic bacteria in the coastal western Antarctic Peninsula (WAP), using decadal (2002–2014) time series of two bacterial variables, bacterial production (BP) via 3H-leucine incorporation rates and bacterial biomass (BB) via bacterial abundance, collected at Palmer Antarctica Long Term Ecological Research (LTER) Station B (64.8°S, 64.1°W) over a full austral growing season (October–March). Strong seasonal and interannual variability in the degree of bacterial coupling with phytoplankton processes were observed with varying lags. On average, BP was only 4% of primary production (PP), consistent with low BP:PP ratios observed in polar waters. BP was more strongly correlated with chlorophyll (Chl), than with PP, implying that bacteria feed on dissolved organic carbon (DOC) produced from a variety of trophic levels (e.g., zooplankton sloppy feeding and excretion) as well as directly on phytoplankton-derived DOC. The degree of bottom-up control on bacterial abundance was moderate and relatively consistent across entire growing seasons, suggesting that bacteria in the coastal WAP are under consistent DOC limitation. Temperature also influenced BP rates, though its effect was weaker than DOC. We established generalized linear models (GLMs) for monthly composites of BP and BB via stepwise regression to explore a set of physical and biogeochemical predictors. Physically, high BP and large BB were shaped by a stratified water-column, similar to forcing mechanisms favoring phytoplankton blooms, but high sea surface temperature (SST) also significantly promoted bacterial processes. High BP and large BB were influenced by high PP and bulk DOC concentrations. Based on these findings, we suggest an increasingly important role of marine heterotrophic bacteria in the coastal WAP food-web as climate change introduces a more favorable environmental setting for promoting BP, with increased DOC from retreating glaciers, a more stabilized upper water-column from ice-melt, and a baseline shift of water temperature due to more frequent delivery of warming Upper Circumpolar Deep Water (UCDW) onto the WAP shelf.
Introduction
Heterotrophic bacteria utilize and remineralize dissolved organic carbon (DOC) and organic nutrients, mobilize carbon for upper trophic levels via microzooplankton grazing, and ultimately affect carbon fluxes and cycling in the ocean (Azam et al., 1983; Ducklow, 1983). Antarctic coastal waters are characterized by low bulk DOC concentrations compared to other coastal ecosystems due to the lack of terrestrial and allochthonous inputs of DOC. Therefore, bacteria must ultimately rely on in situ DOC produced by phytoplankton (Baines and Pace, 1991; Nagata, 2000) or from other trophic levels and processes such as zooplankton grazing, sloppy feeding, excretion, and cell lysis (Strom et al., 1997). Bacterial coupling with phytoplankton has been demonstrated in the coastal WAP (Ducklow et al., 2012; Saba et al., 2014), consistent with other observations from Antarctic waters (Morán et al., 2001; Morán and Estrada, 2002). Saba et al. (2014) demonstrated that bacterial blooms occurred in years of positive chlorophyll (Chl) anomalies. Ducklow et al. (2012) showed that the degree of coupling between phytoplankton and bacterial properties was variable depending on the space and time scales analyzed.
The coastal WAP is a very productive ecosystem with high phytoplankton productivity and biomass accumulations (i.e., high Chl) as spring ice-melt drives stratification of the upper water column and subsequently alleviates light limitation to cells (Smith et al., 2008; Vernet et al., 2008). The WAP reflects strong seasonal and interannual variability in a variety of marine ecological and biogeochemical functions as a result of large-scale climate variability including the Southern Annular Mode (SAM) and El Niño-Southern Oscillation (ENSO) and their impacts on local physical drivers such as sea ice, upper ocean physics, and local wind patterns. The teleconnection of ENSO with the WAP has similar effects as the SAM, where El Niño causes fewer storms and colder air temperatures, inducing winter sea ice growth. The negative SAM also creates favorable conditions for winter sea ice growth as a result of increased cold southerly winds blowing across the WAP (Stammerjohn et al., 2008). It was demonstrated that positive Chl anomalies occurred every 4–6 years in a stabilized water column induced by large sea ice extent during preceding winters as a consequence of the negative phase of winter SAM and by reduced wind speeds in the following spring as a result of positive spring SAM (Saba et al., 2014). Biological drawdown of dissolved inorganic nutrients in nearshore waters at Palmer Station is also regulated by a similar suite of climate and physical forcing factors (Kim et al., 2016). However, impacts of these physical forcing factors on other components of food webs, such as marine heterotrophic bacteria or microbial loop dynamics, have not been examined. The coastal WAP ecosystem is ideal for exploring these relationships given its well-established relationships with physical and environmental drivers.
The ratios of bacterial production (BP) to PP (BP:PP) are low in polar waters indicating that only a small portion of PP supports bacterial carbon consumption (Ducklow et al., 2001, 2012; Kirchman et al., 2009a). It has long been hypothesized that bacterial growth in Antarctic waters is inhibited by low temperature (Pomeroy and Wiebe, 2001), low terrestrial fluxes of biologically available dissolved organic material (DOM; Kirchman et al., 2005, 2009a), and strong top-down control via bacteriovore grazing and viral lysis (Bird and Karl, 1999). Recently, Brum et al. (2015) suggested that temperate bacteriophage infection imposed a strong top down control on BP. The review by Kirchman et al. (2009b) suggested that BP:PP ratios significantly increase with temperature, but only below 4°C, possibly due to reduced bacterial uptake rates of organic carbon in cold waters without a significant decrease of PP at the same time. Ducklow et al. (2012) showed that temperature per se does not seem to regulate BP, but that high BP was rather associated with phytoplankton blooms. Their analysis was constrained to limited duration cruises, providing snapshots of microbial loop dynamics in the middle of summer (January). Given that not only the degree of phytoplankton-bacterial coupling but also overall bacterial responses may change across different time and space scales, it is important to revisit this issue with seasonal datasets, and over longer time spans.
Here, we report variability of bacterial dynamics in the coastal waters off Palmer Station in the WAP, using a decadal time series (2002–2014) of two bacterial properties, BP and bacterial biomass (BB), over the phytoplankton growing season (October-March). We use the term bacteria to denote heterotrophic bacteria due to a very small fraction of autotrophic bacterioplankton (usually <1% of total bacterial count) and also a low fraction of archaea in the upper 100 m of the WAP in summer (Church et al., 2003). Based on the two bacterial indices, we addressed seasonal and interannual variability of bacterial dynamics, the degree of bacterial-phytoplankton coupling, and of resource (bottom-up) control on bacterial growth, and how these couplings vary seasonally and interannually. Next, we investigated a potential suite of physical and biogeochemical forcing factors influencing each bacterial variable, using time series of the relevant climate and local physical and biogeochemical parameters. We hypothesized that active bacterial processes are shaped by similar physical forcing factors as phytoplankton blooms (e.g., Saba et al., 2014), however, with complicating effects from temperature in the coastal WAP.
Methods
Site Description
The study area is located at Palmer Station B (64.8°S, 64.1°W) in the coastal WAP (Figure 1, adopted from Kim et al., 2016). Palmer Station B is relatively shallow (~75 m) and located inshore adjacent to the coastal Marr Glacier, which has retreated significantly over the past few decades.
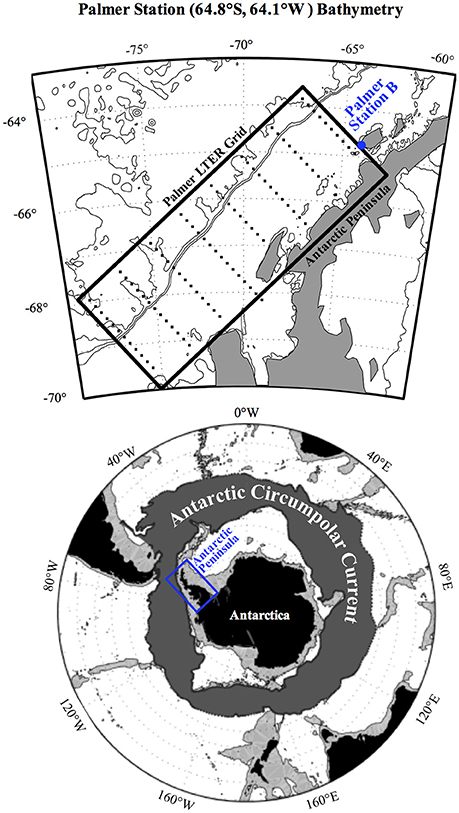
Figure 1. Location of Palmer Station B in the West Antarctic Peninsula (WAP) adopted from Kim et al. (2016).
Sampling
Samples for bacterial and other ecological and biogeochemical properties (e.g., DOC, PP, Chl) were collected at Palmer Station B on a twice-weekly basis over the 2002–2003 (hereafter 2002–2003) to the 2014–2015 Antarctic field seasons, with a Go-Flo bottle cast from a Zodiac boat. Samples were not collected during the 2007–2008 field season due to logistical reasons. Usually 4–6 samples were obtained in the upper 50 m. Initial date of sampling was determined by sea ice retreat allowing small boat access to sampling sites. Termination of each season was determined by vessel scheduling. Typically, sampling lasted from late October to late March.
Measurement of Bacterial Production via 3H-Leucine Incorporation
The 3H-leucine incorporation rate of the collected water samples was measured to derive BP rates. We followed a modified protocol of the original leucine assay proposed by Smith and Azam (1992), as described in Ducklow et al. (2012). The 3H-leucine incorporation rate (pmol l−1 h−1) was converted to BP rates (mgC l−1 h−1) using the factor 1.5 kgC mol−1 leucine incorporated (Ducklow et al., 2000). We recognize that conversion factors may vary (Kirchman et al., 2009b and references therein); but adopt a constant factor to facilitate comparison with other studies (e.g., Ducklow et al., 2012).
Measurement of Bacterial Abundance via Flow Cytometry
BB was derived from bacterial abundance measurements. Samples for bacterial abundance were analyzed within 6-h of collection by flow cytometry following the protocol of Gasol and Del Giorgio (2000) on an Accuri C6 (Becton-Dickinson). Total bacteria concentrations were determined by adding 1 μm microspheres (Polysciences, Warrington, PA) and 5 μM final concentration of SYBR-Green stain to 0.5 ml of samples. The incubated samples were measured for 2-min at a low flow rate. Total bacterial cells and beads were enumerated in cytograms of side scatter (SSC) vs. green fluorescence (FL1). The absolute concentration of stained cells was calculated using the total sample volume analyzed, as determined by the count of the added microspheres. Bacterial abundance was then converted to BB values using 10 fgC cell−1 (Fukuda et al., 1998). Thus, BB in our study simply reflects bacterial abundance integrated in the upper water-column, not incorporating the effect of cell volume changes over time and space. The time series data for both BP and BB values (Dataset 47) are archived at the Palmer LTER Datazoo: (http://oceaninformatics.ucsd.edu/datazoo/data/pallter/datasets). Other complementary datasets for the purpose of comparing with bacterial properties are also available at the Palmer LTER Datazoo: Chl (Dataset 126), 14C-PP or PP (Dataset 127), and DOC (Dataset 70) and full descriptions on their analysis and measurements are found in Supplementary Material.
Calculations of Bacterial Standing Stocks
Due to a weak variability of bacterial indices below 50 m and the shallow depth of Palmer Station B, 3H-leucine incorporation rates and bacterial abundance were depth-integrated to 50 m. This is also the depth at which Chl and PP are typically at or near zero and at which macronutrients first reflect their maximum water-column concentrations (Kim et al., 2016). Thus, depth-integrated standing stocks for bacterial properties (mgC m−2 d−1 for BP and mgC m−2 for BB) provide a consistent basis of comparison across months, seasons, years, and other datasets. Prior to depth-integration, replicate values at the same depth were averaged and the values at the shallowest depth (<5 m) were extrapolated to the surface if the surface samples were missing. The values at 50 m were linearly interpolated if not sampled. The depth-integrated standing stocks were calculated only when the values for at least 3 discrete depths were measured in the upper 50 m. Otherwise, the depth-integrated stocks were estimated using a linear regression between surface and depth-integrated values (r2 = 0.64–0.79, all P < 0.001).
Empirical Orthogonal Function (EOF) Decomposition
We performed EOF analysis to investigate intraseasonal (i.e., variations within each growing season, 2002–2003 to 2014–2015 field seasons) to interannual covariability (i.e., interannual variability of seasonal dynamics) of BP and BB. More details regarding input data matrices and seasonal grids are found in Text S1. Basically, the sample covariance () was estimated by computing biased covariance between time series from all pairs of grid cells, including mutually occupied time steps only. A standard EOF analysis is performed on this estimated covariance matrix to compute eigenstructure, where first 4–5 dominant EOFs (4 EOFs for BP accounting 86.6% of total variability and 5 EOFs for BB accounting 97.9% of total variability after visually examining eigenvalue and scree plots) are combined to provide a smooth, reduced-space interpolant data across the grid in seasons and years (i.e., reduced space optimal analysis (RSOA); Kaplan et al., 1997). More details on EOF computations are available in Text S1.
Generalized Linear Models (GLMs) by Stepwise Regression
We established GLMs for BP and BB by stepwise regression to investigate large-scale climate and local physical and biogeochemical forcing factors predicting high BP and BB, respectively. The predictor (independent) variables tested for model selection are summarized in Table S1 and Text S2. When performing stepwise regression analysis for GLMs, it is critical that a response (dependent) variable being modeled is normally distributed. To ensure each data set comes from as much like a normal distribution as possible, the BP dataset was log10 transformed and the BB dataset was square root transformed.
Model selection of GLMs was based on the corrected Akaike Information Criterion (AICC). Typically, an ordinary, uncorrected version of AIC is used to determine the significance of each predictor variable in the model, where the residual sum of squares is penalized by twice the number of parameters times the residual mean square of the initial model (Akaike, 1974). The usage of the AICC allows, additionally, the selection of the model with the closest to optimal balance between goodness of fit and parameterization, while making a correction for small sample size typically as in the case of ecological time series (Burnham and Anderson, 2004). Predictor variable terms (Text S2, Table S1) were added and removed in order of greatest reduction in the AICC value in a stepwise manner (i.e., a combination of backward elimination and forward selection). Then, the final GLMs were determined from a sequence of the steps, which minimizes the AICC, associated statistics, and has a fewer number of predictor variables, if possible, to avoid overfitting in the model. The models tested include multiple types, including linear models (e.g., constant, linear models) and non-linear models (e.g., quadratic, pure quadratic, and interactions). Finally, statistical stability of the established BP and BB GLMs was assessed based upon two criteria: (1) normality of the residuals of the selected GLMs and (2) a low degree of multicollinearity of the used predictor variables. We confirmed based on normality tests (e.g., Jarque-Bera, Lilliefors, and χ2 goodness-of-fit) that the residuals between modeled and observed BP and BB are from a normal distribution at a 95% confidence interval (CI). This indicates that linear relationships between the predictor and response variables are not affected by outliers or highly skewed predictor variables. Multicollinearity, a statistical phenomenon where predictor variables in a multiple regression model are highly correlated, leads to a reduction in the ability to detect reliable effects of correlated variables. To examine how much the variance of the coefficient estimates are being inflated by multicollinearity in each bacterial GLM, we calculated the variance inflation factors (VIFs) for each predictor variable used in the GLMs. The selected GLMs and associated statistics are summarized in Tables 1–2.
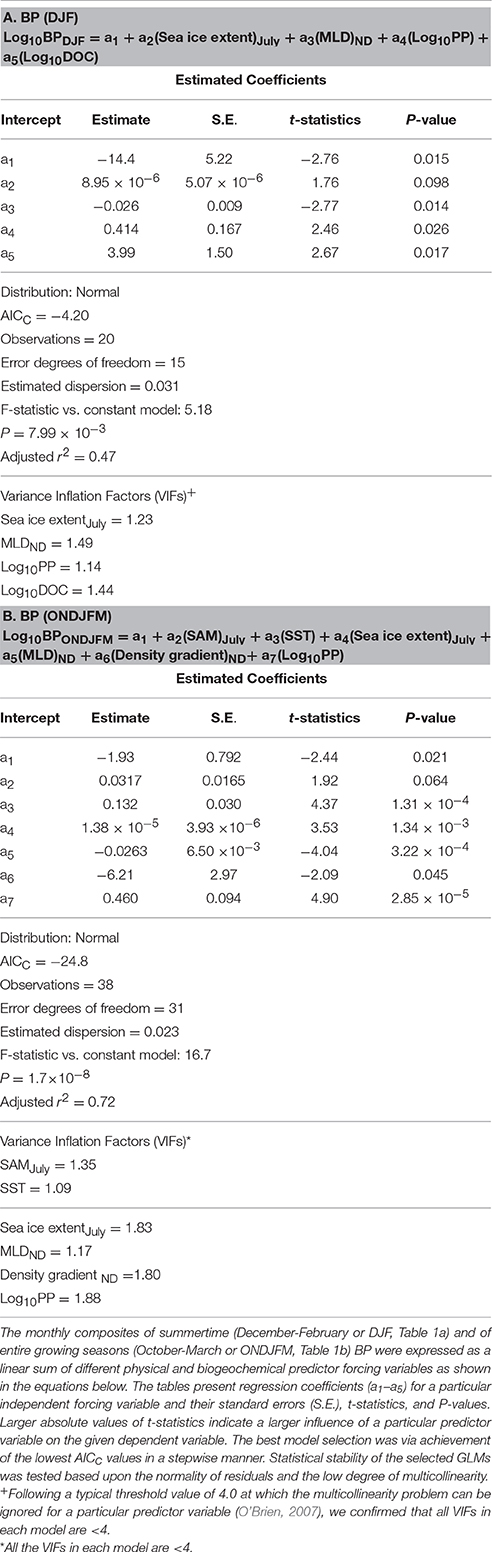
Table 1. Summary of generalized linear models (GLMs) and associated statistics for monthly composites of BP during summertime only (A) and during entire growing seasons (B).
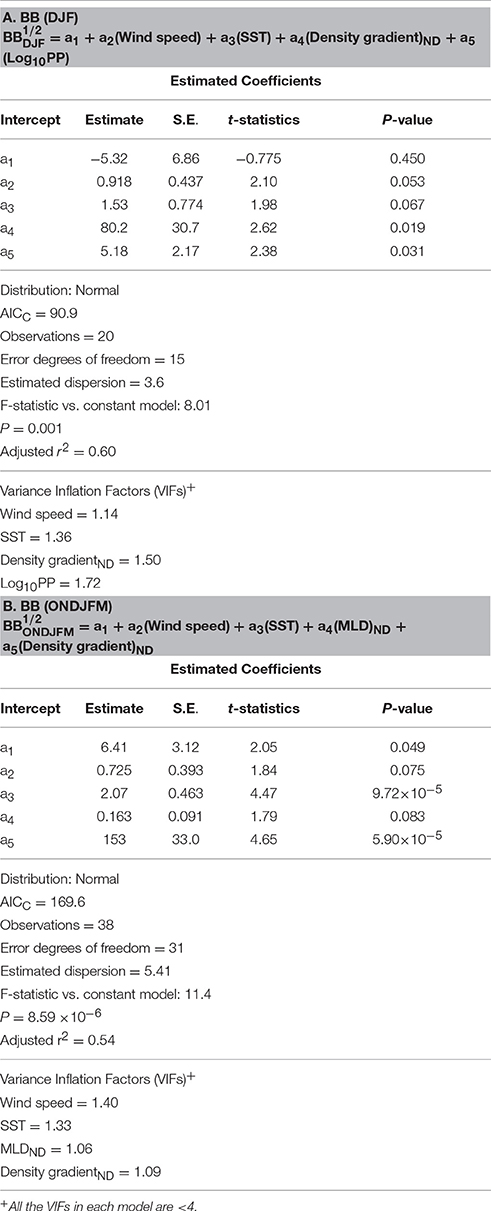
Table 2. Summary of generalized linear models (GLMs) and associated statistics for monthly composites of BB during summertime only (A) and during entire growing seasons (B).
Results
Depth Distributions of Bacterial and Phytoplankton Properties
Depth contours of temperature and salinity showed seasonal evolution in the upper water column with significant interannual variability in terms of the timing. Seasonal warming of the water column started from early to late December (day 340–365) with corresponding decreases of salinity (i.e., freshening) from surface of the water column (Figures 2A,B). High 3H-leucine incorporation (>50 pmol l−1 h−1) or large bacterial abundance (>109 cells l−1) generally followed high phytoplankton values (>100 mgC m−3 d−1; >5–10 mg Chl l−1) with varying lags from a few days (days of year 335–350 in 2012–2013; Figures 2C–E) up to a month (days of year 410–425 in 2005–2006 and days of year 365–395 in 2012–2013; Figures 2C–E), though not always. For example, there was anomalously high 3H-leucine incorporation in 2010–2011 (day of year 365–410; Figure 2E), but with only a slight increase of Chl (Figure 2D) and a lack of high PP (Figure 2C). This discrepancy was also observed in the year 2011–2012. Conversely, high Chl and PP did not result in high 3H-leucine incorporation events as shown in December of 2003–2004. Furthermore, high 3H-leucine incorporation did not always co-occur with large bacterial abundance as shown in years 2010–2011, 2011–2012, and 2012–2013 (Figures 2E,F).
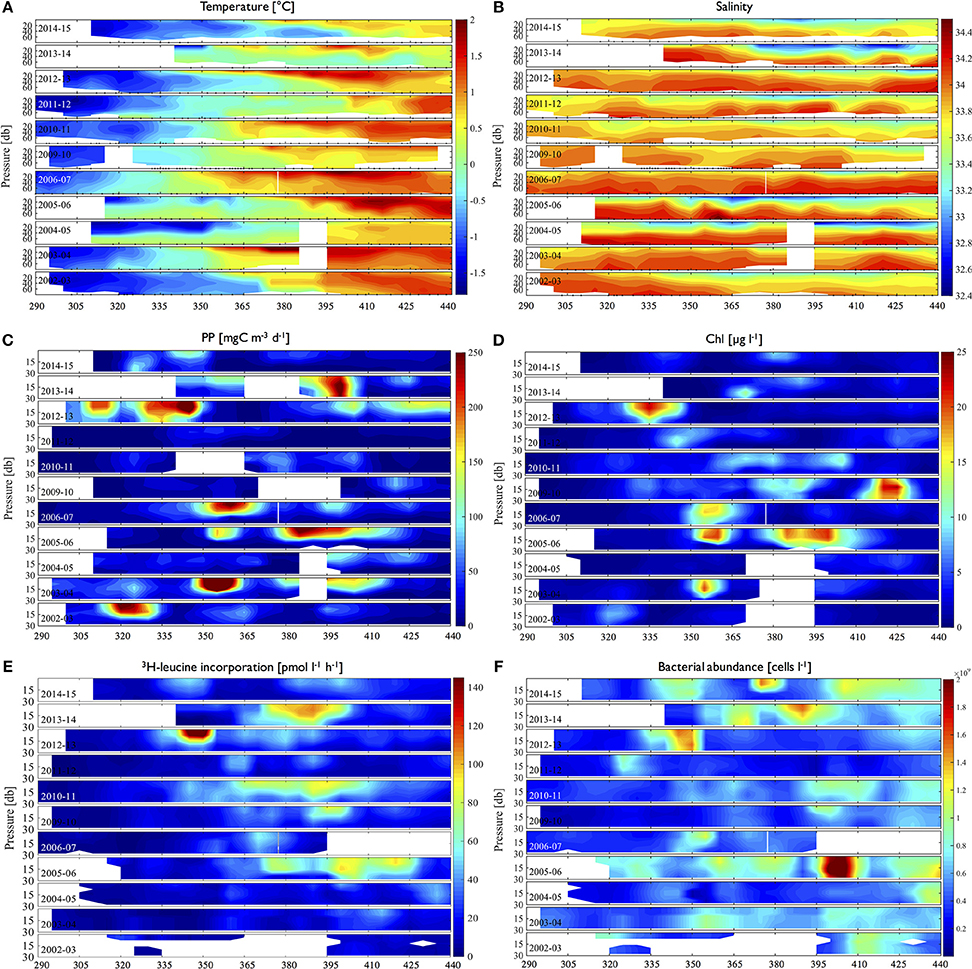
Figure 2. Time-depth distributions of physical properties (A. temperature, B. salinity), volumetric phytoplankton (C. PP, D. Chl) and bacterial properties (E. 3H-leucine incorporation rates, F. bacterial abundance) for individual years (2002–2003 to 2014–2015) over full growing seasons (day of year 290 to 440 or mid-October through March. Black triangle marks on the x-axis indicate sampling time points.
Bottom-Up Control on Bacterial Growth
To assess the degree of bottom up (resource or substrate) control on bacterial growth, we examined slope values from significant log-log regressions of BP on BB (Billen et al., 1990; Ducklow, 1992) both for seasons and individual years (Figure 3). For annual slope values (Figure 3A) slope values were calculated from BP-BB regressions by pooling all monthly data together in each individual year. For seasonal slope values (Figure 3B), slope values were calculated from BP-BB regressions by pooling all yearly data together in each particular month. The slope value plots from BP-BB regressions are also overlaid with slope values from SST-BP regressions to compare bottom-up control with the strength of temperature control on bacterial activity (Morán et al., 2001). When pooled for all seasons and years, the slope value from BP-BB regressions was 0.35 (r2 = 0.26, P < 0.001, n = 333; Figure 3C), while the slope value from SST-BP regressions was 0.14 (r2 = 0.20, P < 0.001, n = 314; Figure 3D), which is slightly lower than the BP-BB regression.
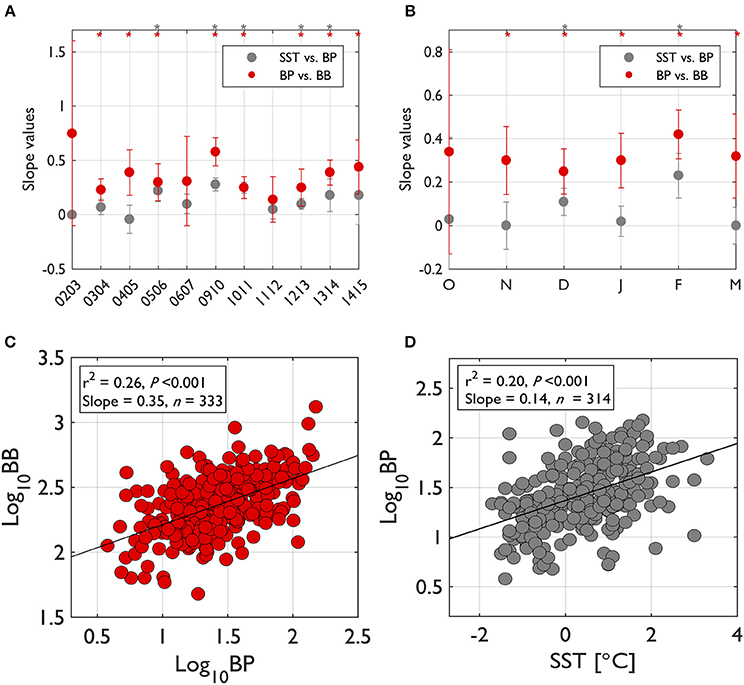
Figure 3. The degree of bottom-up control on bacterial biomass (BB) and of temperature control on bacterial production (BP) based on the slope values from log-log regressions of BP-BB and of sea surface temperature (SST)-BP. The degree of bottom-up control on BB and of temperature control on BP were examined from regression slope values between those variables (asterisk on top of plot indicates significant regressions at P < 0.05). For each year's slope values (A), the slope values for that year were calculated by pooling all seasonal months' data in that particular year. For seasonal slope values (B), the slope values for each month were calculated by pooling all individual years' data in that particular month. When pooled for all seasons and years (C,D), the regression slope value for BP-BB was 0.35 (r2 = 0.26, P < 0.001, n = 333) and the regression slope value for SST-BP was 0.14 (r2 = 0.20, P < 0.001, n = 314). Error bars indicate standard deviation of the slope value at 95% Confidence Interval (CI).
Annual slope values (only from significant relationships) of BP-BB regressions ranged from 0.23 in 2003–2004 to 0.58 in 2009–2010, showing a considerable interannual variability in the degree of bottom-up control (Figure 3A). Unlike significant bottom-up control in most years, temperature control was significant only for half of our study years. Temperature control was never stronger than bottom-up control in any years (Figure 3A).
Seasonally, the regression slope values for BP-BB were very consistent with a value near 0.32 (Figure 3B). Most seasons showed a significant bottom-up control except for October. However, temperature control was only significant for less than the half the growing seasons, only evident in December and February (Figure 3B). The strength of temperature control on BP was higher in February than in December.
Bacterial-Phytoplankton Coupling
To assess the degree of bacterial coupling with phytoplankton processes, we examined slope values from significant log-log regressions between PP or Chl (as an independent variable, X) and BP (as a dependent variable, Y). The regressions were assessed seasonally and annually. When pooled for all seasons and years, the slope value from log-log regression of Chl-BP was 0.32 (r2 = 0.12, P < 0.001, n = 271; Figure 4C), while the slope value from log-log regression of PP-BP was 0.17 (r2 = 0.07, P < 0.001, n = 230; Figure 4D). The BP:PP ratio Figure 5B) was calculated from annually integrated PP and BP (days 290–440; Figure 5A). The 11-year mean BP:PP ratio was 0.04, showing considerable interannual variability in the ratio. Annual-scale PP-BP regressions were largely absent except for 2005–2006 and 2010–2011 with slope values of 0.57 and 0.46, respectively (Figure 4A). In contrast, significant Chl-BP regressions were more frequent with slope values ranging from 0.33 to 0.56 (years 2005–2006, 2009–2010, 2010–2011, 2013–2014, and 2014–2015; Figure 4A) showing a considerable interannual variability in the degree of Chl control on BP.
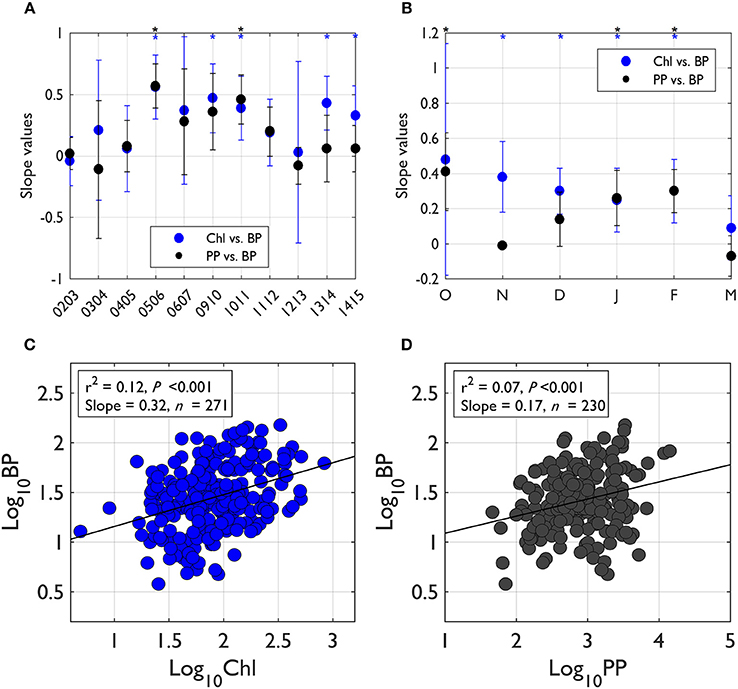
Figure 4. The degree of bacterial-phytoplankton coupling based on the slope values from log-log regressions of phytoplankton properties (PP, Chl)-BP. The degree of BP coupling with phytoplankton activity (PP) and biomass accumulation (Chl) was assessed using the slope values from log-log regressions of PP (or Chl)-BP (asterisk on top of plot indicates significant regressions at P < 0.05). For each individual year's slope values (A), the slope values for each year were calculated by pooling all monthly data in that particular year. For seasonal slope values (B), the slope values for each month were calculated by pooling all individual years' data in that month. When pooled for all seasons and years (C,D), the regression slope value for Chl-BP was 0.32 (r2 = 0.12, P < 0.001, n = 271) and the regression slope value for PP-BP was 0.17 (r2 = 0.07, P < 0.001, n = 230). Error bars indicate standard deviation of the slope value at 95% CI.
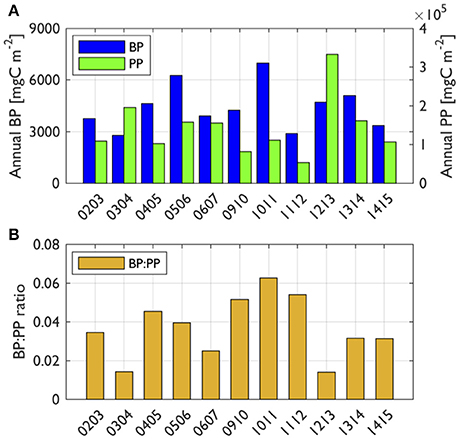
Figure 5. Annually integrated BP, PP (A) and the ratio of BP to PP (B). Reduced space optimal analysis (RSOA)-interpolated values (Figure 6) were annually integrated from days 290 to 440 for BP and PP for each year. Year annotations indicate the starting year of the austral growing season (i.e., 02 for October 2002 to March 2003).
Seasonally, PP-BP regressions were only significant in October, January, and February, while significant Chl-BP regressions were more frequently observed (November to February; Figure 4B). The PP-BP regression slope values (only significant relationships) ranged from 0.26 to 0.41. The slope values from significant Chl-BP regressions ranged from 0.25 to 0.38, showing a similar seasonal variability as PP-BP regressions.
Climatology of Seasonal Bacterial Dynamics
The individual year plots indicate strong seasonal and interannual variability of BP and BB values (Figure 6). From a climatological (2002–2014) perspective, on average, there was a gradual, seasonal increase of BP rates from a seasonal minimum of 11.4 mgC m−2 d−1 to maximum at 46.4 mgC m−2 d−1 in mid-January (Figure 7A). BP declined to ~109% of its starting value (seasonal minimum). In contrast, climatological BB showed somewhat a different pattern than BP, with a sustained increase as the mean growing season progressed, reaching a maximum of 419 mgC m−2 at the end of the sampling period (Figure 7B). Thus, we recognize the possibility of increasing BB values after the end of our sampling period, but an analysis for extended seasons until Austral winter is beyond the scope of our study. PP (Figure 7C) and Chl climatologies (Figure 7D) showed similar patterns to the BP climatology, suggesting a close phytoplankton-bacterial coupling.
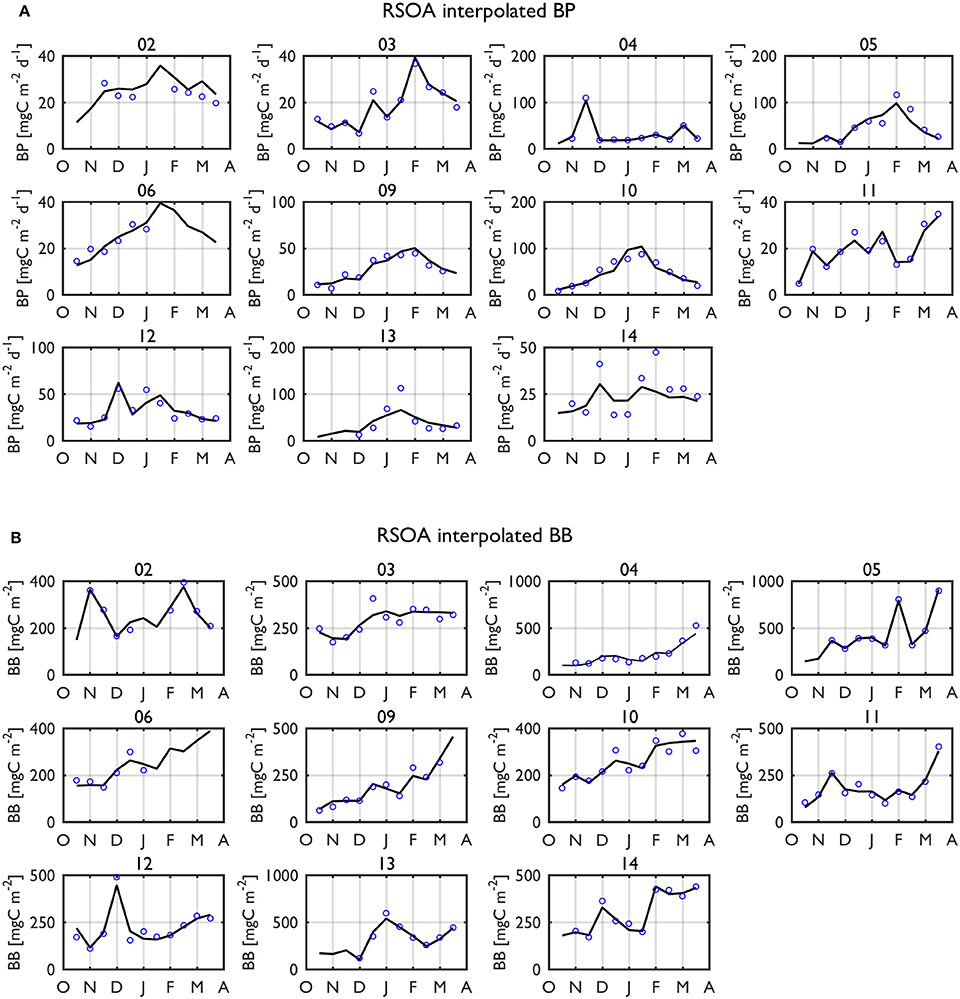
Figure 6. Interpolated (black solid line) BP rates (A) and BB standing stocks (B) in the upper 50-m using the first 4 and 5 dominant EOF modes, respectively (i.e., RSOA interpolation) for individual years over entire growing seasons. The blue dots indicate 15-d means of measured values of BP and BB. Year annotations indicate the starting year of the austral growing season (i.e., 02 for October 2002 to March 2003).
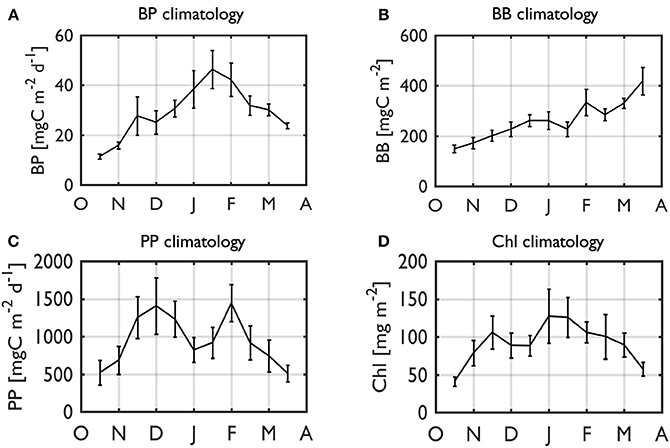
Figure 7. Climatology of BP (A), BB (B), PP (C), and Chl (D). Climatology (2002–2003 to 2014–2015) of depth-integrated (0–50 m) BP (A), BB (B), PP (C), and Chl (D) for a full growing season. The data in the calculation are RSOA-interpolated data. Error bars indicate 1 standard error.
Interannual Variability of Seasonal Bacterial Dynamics
This section describes seasonal variability patterns (i.e., EOF) of BP and BB, and how these patterns vary interannually (i.e., PC). We report first the two dominant EOF modes (EOF1-2; Figures 8A,D) and their PC time series (PC1-2; Figures 8C,F) to consider major seasonal patterns (phenology) of bacterial processes. Next, we present anomalies relative to the 11-year climatology of the given seasonal time bins (RSOA anomalies; Figures 8B,E).
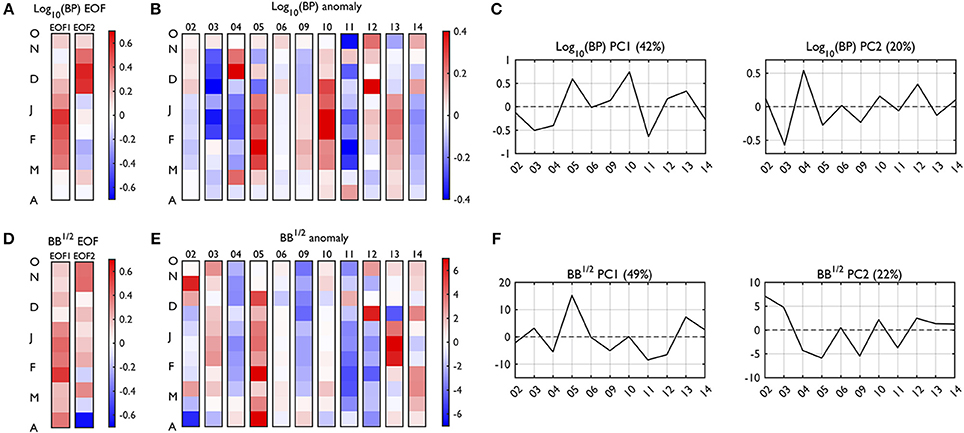
Figure 8. Empirical orthogonal function (EOF) and anomaly plots of depth-integrated BP and BB. The EOF (A,D) patterns and RSOA-interpolated anomaly plots (B,E) reconstructed using the first 4 and 5 dominant EOF modes, accounting for 86.6 and 97.9% of total BP and BB variability, respectively. The percentages captured by each EOF mode are presented in the corresponding principal component (PC) time series (C,F), which indicates interannual variability of the seasonal patterns detected by the corresponding EOF. The red colors represent positive anomalies, and blue colors represent negative anomalies relative to the climatology (2002–2003 to 2014–2015; Figure 7) for each seasonal time bin. Year annotations indicate the starting year of the austral growing season (i.e., 02 for October 2002 to March 2003). Note that there are year gaps (no data in 07 and 08).
BP mode 1 (42% of total BP variability) was characterized by a gradual increase of BP rates starting with a slight increase in late October, a peak in January, and then decreases after February (BP EOF1, Figure 8A), similar to the climatology. The seasonal maximum of BP occurs from December to February (DJF) and is therefore termed “DJF bacterial productivity.” The years of high DJF bacterial productivity were represented as high PC1 values (years 2005–2006, 2010–2011, 2012–2013, and 2013–2014; Figure 8C). BP mode 2 (20% of total BP variability) mainly captured the rest of the variability left out from BP mode 1, showing elevated BP rates during November to the first half of December. The years of this high November-early December, “spring bacterial productivity” were observed as high PC2 values (years 2004–2005 and 2012–2013; Figure 8C).
BB mode 1 (49% of total BB variability) represented high BB throughout growing seasons with slightly higher biomass during DJF than other months (BB EOF1, Figure 8D). This overall, seasonally increased BB pattern was captured by large PC1 (BB PC1, Figure 8F; years 2005–2006, 2013–2014, and 2014–2015). Relatively speaking, BB mode 2 (22% of total BB variability) represented large BB especially in the beginning of the season (October to first half of November) and corresponded to large PC2 (BB PC2, Figure 8F; years 2002–2003, 2010–2011, and 2012–2013).
Physical and Biogeochemical Forcing Factors of Bacterial Dynamics
Finally, based on our results from bacterial GLMs (BP and BB GLMs) we explored a potential suite of physical and biogeochemical forcing factors responsible for the observed variability of each bacterial property. We first examined monthly summer (DJF) composites of BP and BB since they show shared strong positive responses from the EOF plots during these months (Figures 8A,D). To see if bacterial responses to physical and biogeochemical forcing factors differ outside of the DJF period, we additionally examined BP and BB GLMs over entire phytoplankton growing seasons (October to March or ONDJFM). Thus, bacterial GLMs (2002–2014) here explain an occurrence of years with high monthly composites of BP and BB standing stocks during summertime (DJF) periods (Tables 1A, 2A), as well as over entire growing seasons (Tables 1B, 2B).
During summertime (DJF), significant physical and biogeochemical forcing variables shaping high BP included shallow MLD, high bulk DOC, and high PP (in order from stronger to weaker forcing; Table 1A). If extended to entire growing seasons, high SST was additionally observed as a significant physical predictor variable for high BP rates: high BP rates during ONDJFM were influenced by high PP, high SST, and shallow MLD (stronger to weaker forcing; Table 1B).
The BB GLMs during summertime (DJF) showed that large BB was influenced by high salinity-driven density gradient and high PP (stronger to weaker forcing; Table 2A). SST was also a significant forcing factor for the BB GLM, but its effect is comparatively lower than other forcing variables based on its lower absolute t-statistic value (Table 2A). As observed in the BP GLM during entire growing seasons, high SST was also shown as a significant predictor variable (in this case, as a “stronger” forcing variable based on a higher absolute t-statistic value than BB GLM for DJF) for large BB if extended to entire growing seasons: large BB was shaped by high salinity-driven density gradient and high SST (stronger to weaker forcing; Table 2B).
Discussion
Studies have investigated dynamics of heterotrophic bacteria in the WAP and adjacent Antarctic ecosystems, with a focus on a variety of processes, including bacterial responses to environmental gradients, coupling with phytoplankton, and community structures (e.g., Karl and Tien, 1991; Karl et al., 1991; Morán et al., 2001; Morán and Estrada, 2002; Ducklow et al., 2012; Luria et al., 2014, 2016; Nikrad et al., 2014; Bowman and Ducklow, 2015). However, these studies are mostly short-term and usually constrained to just 1 or 2 years. Our seasonally extended (October–March) and decadal time series analysis of bacteria highlights a set of patterns that have not been identified in the previous work, including time-varying temperature control on BP and consistent DOC limitation throughout the phytoplankton growing season. Our discussion begins with a broad overview of bacterial dynamics using the depth contour plots for each season and year (see below).
Overview of Bacterial Dynamics Based on Depth Profiles
To first order, depth profiles showed that high 3H-leucine incorporation rates generally followed large phytoplankton blooms and were also almost always found in the upper water column coinciding with high PP, thereby indicating a frequent, but variable coupling between bacterial and phytoplankton processes in the coastal WAP waters. Bacteria and phytoplankton appeared to be coupled (i.e., high bacterial events following large phytoplankton events), with varying degrees of lag between the two. The different degrees of bacterial-phytoplankton coupling could be attributed to different production rates of DOM from phytoplankton assemblages and its rate of subsequent bacterial utilization, which is often a function of different bacterial clades (Straza et al., 2009; Nikrad et al., 2014). It was shown in Antarctic waters that up to a month was needed for bacterial blooms to respond to diatom blooms due to delayed production and utilization of macromolecular, polymeric DOM (Billen and Becquevort, 1991; Lancelot et al., 1991), compared to shorter lags of a few days at lower latitudes (Billen et al., 1990). If bacterial blooms are dominated by fast growing bacteria such as gammaproteobacterial clades (e.g., Ant4D3, Arctic96B-16), there may be a relatively short lag between high BP and phytoplankton bloom events, in response to low-molecular-weight, readily utilizable DOM than when dominated by slowly growing bacterial clades such as SAR86 (Nikrad et al., 2014). Conversely, if bacterial metabolism is mainly based on high-molecular-weight DOM, it likely leads to a longer lag. Luria et al. (2016) demonstrated that different individual bacterial taxa were dominant over the course of the bacterial bloom in the coastal WAP, which contributed to different time lags in the bacterial responses to DOM. On the other hand, varying lags of bacterial-phytoplankton coupling might also happen if bacteria rely not only on LDOC but also on semi-labile DOC (SDOC) from previous months' or years' phytoplankton accumulations (Kirchman et al., 2001; Davis and Benner, 2005), which leads to a seemingly uncoupling or a coupling with a long lag (more detailed discussion on SDOC is found in Section Biogeochemical Forcing Factors of Bacterial Dynamics). In the Ross Sea, it was shown that bacterial growth was strongly dependent on previously accumulated SDOC, at different rates over the course of growing seasons, but SDOC was eventually entirely consumed by the end of the season (Ducklow et al., 2001; Ducklow, 2003). Using 16S rRNA gene phylotype-derived prediction of microbial metabolic pathways, Bowman and Ducklow (2015) also showed that bacterial communities presented a differential distribution for pathways associated with the degradation of DOC pools along the WAP, which likely implies different bacterial clades might be responsible for utilization of different DOC pools in our study.
In contrast, BP was occasionally decoupled from PP or Chl (e.g., December of 2003–2004; Figures 2C–E) where high phytoplankton processes did not lead to high BP events. This implies that some other environmental factor prevents high bacterial activity despite availability of organic carbon sources for bacteria. One possibility is low temperature, which has long been hypothesized to cause low BP to PP ratios in polar waters (Pomeroy and Wiebe, 2001). In our depth profiles, bacterial accumulations were rarely observed in early spring when SST was still low (< ~0.5°C; Figures 2A,E,F), implying that there might be at least some degree of temperature control on bacterial activity in the coastal WAP waters. However, presumably due to small sample size (n = 9) during the time of the observed decoupling (December of 2003–2004; Figures 2C–E), SST was not significantly correlated to 3H-leucine incorporation rates, thereby precluding a test of a direct effect of temperature. Regulation of diverse bottom up control factors (e.g., Chl, PP) on BP is often manifested at larger scales (e.g., Dufour and Torreton, 1996; Ducklow et al., 2012). Thus, by pooling all years' data in each seasonal month, we subsequently investigated the degree of temperature control on BP with greater sample size, using the slope values from SST-BP regressions seasonally (See Section Bottom-Up Control on Bacterial Abundance).
Our observations also showed that high BP did not always lead to large BB accumulations (e.g., 2010–2011, 2011–2012, and 2012–2013). Here, top down control factors might play a significant role in causing BP-BB decoupling, given that BB could only increase if bacterial growth exceeds removal by grazers or bacterivore grazers are not capable of balancing the production of enlarging bacterial cells (Gasol et al., 1999). Antarctic waters are often characterized with a high grazing pressure of protozoan grazers on bacteria, which strongly limits large bacterial accumulations (Bird and Karl, 1999). In the coastal WAP, microzooplankton were specifically shown to exert a substantial grazing pressure on bacteria, often removing >100% of BP (Garzio et al., 2013). Viral infection and lysis were also shown as critical factors for causing significant bacterial mortality in Antarctic waters (Guixa-Boixereu et al., 2002; Brum et al., 2015). In the following Section Bottom-Up Control on Bacterial Abundance, we discuss in detail the effect of bottom-up control on bacterial abundance from our data, primarily using the slope values from BP-BB regressions.
Bottom-Up Control on Bacterial Abundance
In a steady-state system where the bacterial utilization rate of DOM is coupled to its rate of supply, BP may be considered a proxy for DOM input flux and thus indicative of bottom-up control (Billen et al., 1990). Traditionally, predominance of bottom-up control on biomass of a certain trophic level has been explored from log-log relationships between abundance (biomass) of that trophic level and presumed controlling factors (McQueen et al., 1986). Following this approach, the strength of bottom-up control on bacterial abundance has been assessed in diverse ecosystems using the slope of significant log-log regressions of BP (DOC)-BB (Shiah and Ducklow, 1995; Dufour and Torreton, 1996; Gasol et al., 2002; Hale et al., 2006; Garneau et al., 2008; Morán et al., 2010; Calvo-Díaz et al., 2014; Ducklow, 1992). Higher slope values (i.e., stronger degree of BB dependence on BP) indicates stronger responses of BB to increases in substrate (DOC) supply or BP, implying that bacteria experience substantial DOC limitation. Conversely, if BB is not responsive to increases in BP, bacteria are not DOC-limited; or possibly are efficiently removed by grazers and viruses.
The regression slope values from our BP and BB data (Figure 3B) indicate a very consistent bottom-up control across seasons with an average slope value of around 0.32. This value reflects a weak to moderate bottom-up control on bacterial growth (Ducklow, 1992). The slope value was never below the threshold slope value of 0.2 (Ducklow, 1992), implying that bacteria in this system were always under some sort of resource limitation over entire growing seasons. Resource-wise, bacteria could be limited by DOC, dissolved inorganic nutrients (e.g., nitrate and phosphate) or trace metals such as iron. However, there is no evidence of macronutrient and iron limitation on phytoplankton at this site (Kim et al., 2016 Sherrell, personal comm.). Experiments by Ducklow et al. (2011) also suggested that the summertime bacterial community is not nitrogen limited in the WAP coastal waters, given that bulk bacterial properties (e.g., BP and abundance) significantly increased in response to experimental additions of glucose, but not to ammonium enrichment. Calvo-Díaz et al. (2014) suggested a seasonal switch from temperature to substrate supply as a major controlling factor for bacterial abundance in the coastal Bay of Biscay, based on their observations of decreasing slope values from temperature-BP regressions and increasing slope values from BP-BB regressions as seasons progress. The WAP system differs substantially from their observations from estuaries (e.g., Shiah et al., 2003). In our study, temperature control on BP was only significant in December and February, while consistent degrees of bottom-up control on BB were evident over all months (Figure 3B). Similar patterns were observed from individual years' slope values (Figure 3A). Only the half of years showed a weak to moderate degree of temperature control on BP, while most years were under weak to moderate DOC limitations. When pooled for all years and seasons, temperature control was considerably weaker than bottom-up control (Figures 3C,D). Based on these observations, we suggest (1) that bacteria in coastal WAP waters are always under some degree of DOC limitation and (2) that both yearly and seasonally temperature also influences BP but the effect may not be as large and consistent as DOC limits bacterial processes.
Bacterial-Phytoplankton Coupling
In the coastal WAP ecosystem heterotrophic bacteria may feed principally on in situ photosynthetic products released from phytoplankton to fuel BP (Baines and Pace, 1991; Nagata, 2000) due to minimal inputs of terrestrial DOM and low bulk DOC concentrations (40–50 μmol C l−1) in the water column (Ducklow et al., 2012). The mean BP:PP ratio across all years and seasons indicated that BP was equivalent to only 4% of PP, similar to the greater WAP region and other polar areas (Kirchman et al., 2009b; Ducklow et al., 2012). The degree of the dependence of bacteria on phytoplankton is often assessed as BP:PP ratios with the reasoning that higher values mean tighter coupling. However, we based our discussion regarding the covariation between bacteria and phytoplankton or bacterial-phytoplankton coupling on the slope values of significant regressions between bacterial and phytoplankton variables (Morán et al., 2001; Morán and Estrada, 2002), not the absolute value of the connection. When pooled for all seasons and years, the PP-BP slope value (i.e., the degree of bacterial-phytoplankton coupling or PP control of BP) was 0.17 in our study (Figure 4D). This is equivalent to 0.004 if recalculated from the untransformed (linear) regression, not significantly different from the regression slope value reported from Ducklow et al. (2012) for the peninsula-wide offshore region during January.
BP was coupled with PP only in October, January, and February, while BP was mostly coupled to Chl with stronger degrees of control (i.e., higher regression slope values; Figure 4B). Most years showed weak to strong coupling between Chl and BP with strong interannual variability in the degree of Chl control on BP (Figure 4B), while only two years showed a significant direct control of PP on BP. On average (over all seasons and years), the degree of Chl control on BP (i.e., slope value of 0.32) also appeared to be stronger than that of PP control on BP (i.e., slope value of 0.17). Thus, these results consistently suggest an overall tighter coupling of BP with Chl than with PP. This implies that overall accumulations of phytoplankton biomass might support BP, but not necessarily carbon flux from PP directly; i.e., release of DOC from active phytoplankton with the timescale of the 14C-PP measurements (~1 day). This point was also raised by Ducklow et al. (2012) to support their observation of tighter coupling between Chl and BP as well, which they attributed to bacterial utilization of DOC from other sources like zooplankton grazing and sloppy feeding on the timescale of phytoplankton stock turnover (~10 days).
Our analysis indicates that only a very minimal portion (i.e., 4%) of fixed PP supports BP in the coastal WAP, corresponding to other studies in polar oceans (Ducklow et al., 2001, 2012; Kirchman et al., 2009a). In the Ross Sea, Carlson et al. (1998) showed that up to ~90% of phytoplankton-production is partitioned to the POC pool, rather than routed through the DOC pool, which accounts for only about 10% of total carbon fixed. Low accumulations of DOC were previously reported after phytoplankton blooms in the WAP region (Tupas et al., 1994). These findings support our conclusion that the limited supply of DOC is a primary reason for low BP:PP ratios. Such a low BP fraction of PP begs a question about the fate of the large amount of unused PP or POC within the food-web of the coastal WAP ecosystem. Using in situ O2:Ar measurements at Palmer Station, Tortell et al. (2014) showed there was a period of intense net heterotrophy during the crash of a phytoplankton bloom. They implicated increased respiration by zooplankton as a major fate of bloom production. Stukel et al. (2015) also demonstrated with 15 uptake and 234Th measurements that new production exceeded export production by a factor of five at Palmer Station. Hence, it is likely that a majority of PP is respired by intense heterotrophic respiration in the upper water column, rather than appearing as bacterial production or being directly exported to depth.
Climatology of Seasonal Bacterial Dynamics
Consistent with strong seasonal and interannual variability of other ecological and biogeochemical properties along the WAP (Ross et al., 2008; Smith et al., 2008; Vernet et al., 2008; Ducklow et al., 2012; Saba et al., 2014; Kavanaugh et al., 2015; Kim et al., 2016), BP and BB also showed strong seasonal and interannual covariability (See Section Interannual Variability of Seasonal Bacterial Dynamics). Accordingly, the climatologies did not reflect occurrence of high BP or bacterial blooms for any one particular year. Both BP and BB values commenced with very low values, 4-fold and 2.8-fold lower than seasonal maxima, respectively, consistent with very low BP values during austral winter seasons (i.e., leucine incorporation rates of typically <5 pmol l−1 h−1).
Notably, seasonal progression patterns were largely different between BP and BB where the annual BP maximum occurred in the middle of the growing season, while the BB maximum was observed at the end of or possibly even after our growing seasons due to its sustained increase throughout the growing season. The slope of the increase of climatological BB over time (our sampling period from October to March) was ~1.8 mgC m−2 per day, <5% of the maximum production rate, indicating the longer-tem balance between bacterial growth and removal.
Interannual Variability of Seasonal Bacterial Dynamics
From the BP and BB EOF plots, the first two dominant modes mostly captured high BP and large BB from the start to nearly end of our sampling period. However, the leading modes (EOF1) of BP and BB did not show the same dominant seasonal patterns between the two properties, in accord with our aforementioned observations (Section Overview of Bacterial Dynamics Based on Depth Profiles), climatologies and individual year plots (Section Climatology of Seasonal Bacterial Dynamics). It was previously shown that the leading EOF modes of two different biogeochemical variables might become alike, if similar physical or biological processes drive variability of each variable (e.g., nitrate and phosphate EOF patterns in Kim et al., 2016). There is no a priori reason that BP needs to have the same EOF patterns with BB if the two properties are shaped by different regulatory causes, again supporting such factors' roles (e.g., temperature, SDOC, DOC from other sources, grazing) described in previous Sections Overview of Bacterial Dynamics Based on Depth Profiles and Bacterial-Phytoplankton Coupling. The interplay of these factors in influencing the interannual variability of BB and PP is considered in the next section using GLMs via stepwise linear regression.
Physical and Biogeochemical Forcing Factors of Bacterial Dynamics
It is well demonstrated that large-scale climate and associated local physical forcing (e.g., sea ice and ice melt driven-stratification of the upper water column) control the timing and extent of phytoplankton blooms and PP along the WAP (Smith et al., 2008; Vernet et al., 2008; Venables et al., 2013; Saba et al., 2014) and adjacent regions (Schloss et al., 2012). Thus far, we have shown evidence of close coupling between bacteria and phytoplankton. In accord with this, we hypothesized that due to a close coupling between bacteria and phytoplankton, similar but possibly slightly different regulatory mechanisms to those of phytoplankton blooms may influence active bacterial processes as a consequence of complicating effects from other controlling factors.
Physical Forcing Factors of Bacterial Dynamics
A stabilized upper water-column via shallow MLD or high salinity-driven density gradient was a significant physical predictor in driving high BP and large BB during summertime (DJF) as well as over entire growing seasons. This is similar to regulatory factors for large phytoplankton accumulations in inshore waters off Palmer Station (Saba et al., 2014; Kim et al., 2016). In the coastal WAP, phytoplankton blooms are primarily induced once they are free from light limitation when the upper MLD begins to shoal (Mitchell et al., 1991; Venables et al., 2013). In turn, shoaling of the upper MLD is controlled by seasonal sea-ice dynamics (e.g., timing of retreat, winter ice extent) since ice melt water plays an important role in stratifying the upper water column (Smith et al., 1998).
It is noteworthy that high SST was also a significant physical predictor for both high BP and large BB, if extended from summertime to entire growing seasons. However, SST neither shaped high BP during summertime alone (DJF) nor played an important role for large BB during DJF as it did over entire growing seasons. It is possible that simply due to small sample size, a correlation between SST and BP (or BB) was absent (or low) during DJF or that a response to temperature only manifested when looking at a longer temporal scale, in this case the seasonal scale, due to a more important role of DOC.
Besides temperature effects, other physical and climate forcings could directly control BP, not modulated through phytoplankton processes. Large winter (July) sea ice extent was a significant physical predictor although its effect was lower than other stronger physical forcings (e.g., MLD, SST). This winter sea ice effect seemed to be somewhat stronger when extended to entire growing seasons (BP GLM for ONDJFM) compared to summertime (BP GLM for DJF). Observations of bacterial and phytoplankton properties were not available in early spring (October-November) of 2004–2005, but RSOA-interpolated anomalies indicated that BP was characterized by strong positive anomalies in contrast to negative anomalies for Chl and PP during that time (Figure 8B, Figure S1). The RSOA-interpolated plot for 2004–2005 also showed the similar patterns (Figure 6A, Figure S2). Thus, there was a high BP event during early spring months of 2004–2005, where bacteria did not depend on phytoplankton-derived fresh LDOC. Notably, the year 2004 was an anomalously heavy sea-ice year compared to the 11-year climatology during our field seasons, with large winter (July) sea ice extent (193,073 km2) and total ice cover (12,066 % days) as well as late retreat (day 395). Winter sea ice had not yet totally retreated at the time of the high BP event. One possibility in support of such high BP rates under high sea ice conditions is an additional supply of DOM from ice melt. It was shown by several studies that DOC could be captured in sea ice during ice formation and utilized by bacteria as ice melts (Kähler et al., 1997; Fortier et al., 2002; Müller et al., 2013).
Biogeochemical Forcing Factors of Bacterial Dynamics
High PP was observed as a common biogeochemical predicting factor for both high BP and large BB. Unlike BP GLMs where PP increased BP regardless of seasons, BB increased in response to PP only during summertime. Bulk DOC was a significant predictor for high BP only if extended to entire growing seasons, not during summertime, even though our data showed no significant relationship between bulk DOC concentrations and Chl or PP (data not shown). Semi-labile DOC (SDOC, turnover time of seasons) is biologically available for bacterial incorporation in polar waters (Kirchman et al., 2001; Davis and Benner, 2005). In the Ross Sea, the entire SDOC pool was utilized for bacterial metabolism by the end of growing season (Ducklow, 2003). This result implies that bacteria in the Ross Sea were under DOC limitation even during phytoplankton blooms, similar to our results of consistent DOC limitation yearly and throughout seasons. Given possible turnover times of some SDOC fractions of up to several years (Kirchman et al., 2001), and the lack of correlations between bulk DOC and Chl or PP from our datasets, it is likely that SDOC produced from previous years or months support bacterial growth in the WAP system similar to the Ross Sea (Carlson et al., 2000; Ducklow, 2003).
Implications for Climate Change along the Coastal Antarctic Ecosystem
The WAP has responded to regional climate change with significant winter atmospheric warming, ocean warming, reduced sea ice duration, and retreat of glaciers and ice sheets (Liu et al., 2004; Cook et al., 2005; Vaughan, 2006; Stammerjohn et al., 2008). The Marr Glacier, located adjacent to Palmer Station B, has retreated, potentially releasing L- or SDOC available to heterotrophs in coastal waters (Cook et al., 2005; Hood et al., 2009, 2015). The heat content of Upper Circumpolar Deep Water (UCDW), regularly spilled into WAP shelf waters, has also increased (Martinson et al., 2008), and its delivery is further expected to increase as a consequence of an increasing trend in the positive SAM phase (Lubin et al., 2008). This may contribute to an overall baseline shift in temperature or increasing UCDW events on the shelf, which may influence BP rates.
In our study, the results from bacterial GLMs showed that bulk DOC was a significant forcing predictor for BP. Together with the results from bottom-up control (Section Bottom-Up Control on Bacterial Abundance), this implies that bacterial growth in the coastal WAP is still under-saturated “resource-wise” (i.e., DOC-limited). The current trend of climate change will enhance water-column stratification through higher surface water temperature and increased freshwater flux from sea ice and glacial melts. As a result, LDOC pools may significantly increase, resulting in more efficient activity of heterotrophic bacteria in the WAP food-web. Recent model findings demonstrated that instead of dominance by the efficient diatom-krill-penguin food chain, the WAP presents a strong interannual variability in food-web dynamics, with an important role of microbial food-webs initiated by small cells such as cryptophytes (Sailley et al., 2013). Retreating glaciers as a result of warming could also increase abundances of freshwater favorable cryptophytes (Moline et al., 2000; Garibotti et al., 2005), further contributing to a shift to a microbial food web dominated system, where heterotrophic bacterial roles in carbon and biogeochemical recycling in the coastal ecosystem also become more prominent.
Conclusions
In conclusion, our seasonally-extended analysis of bacterial dynamics using a decadal (2002–2014) time series of BP and BB revealed a set of patterns which have not been identified previously due largely to the short-term, temporally limited focus of most previous studies. Our major findings highlight strong seasonal and interannual variability in the degree of bacterial coupling (or decoupling) with phytoplankton properties, with varying lags presumably due to different bacterial clades' roles in DOM uptake and bacterial reliance on SDOC in the system. BP was more tightly coupled with Chl than with PP, suggesting that bacteria in the WAP system also rely on DOC produced from diverse trophic levels and processes, not solely on LDOC of recent phytoplankton origin. The degree of bottom-up control on bacterial growth showed that bacteria in this system are under a consistent degree of DOC limitation during entire growing seasons, with a great interannual variability in the strength of such control. Temperature also seems to play an important role, but not as much as DOC in causing low BP:PP ratios (i.e., only ~4% of PP supports BP) in coastal WAP waters. Top-down effects of microzooplankton grazing and viral lysis also seem to exert important roles in decoupling of BB from BP. All these aforementioned factors accordingly appeared as significant regulatory physical and biogeochemical forcing factors in bacterial GLMs. Given the current state of heterotrophic bacteria in the WAP system, which are under substantial DOC and temperature control, an ongoing trend of climate change along the WAP may increase BP rates as a consequence of a potential increase of DOC from retreating coastal glaciers and an increasing trend of temperature, ice melt, and ocean heat content of UCDW and their subsequent impacts on the upper water-column.
Author Contributions
Substantial contributions to the conception or design of the work (HK, HD); The acquisition, analysis, or interpretation of data for the work (HK, HD): Drafting the work (HK); Revising the work critically (HD); Final approval of the version to be published (HD).
Funding
Palmer LTER program was supported by U.S. National Science Foundation awards OPP 0217282, OPP 0823101, and GEO-PLR 1440435. HK was supported in part by the Department of Earth and Environmental Sciences at Columbia University, and by a subcontract to Lamont-Doherty Earth Observatory from NASA ROSES award NNX14AL86G to Scott Doney (Woods Hole Oceanographic Institution).
Conflict of Interest Statement
The authors declare that the research was conducted in the absence of any commercial or financial relationships that could be construed as a potential conflict of interest.
Acknowledgments
We thank Jeff Bowman and XAG Morán for helpful discussion as well as Palmer LTER field assistants for collection of bacterial samples and sample analyses.
Supplementary Material
The Supplementary Material for this article can be found online at: http://journal.frontiersin.org/article/10.3389/fmars.2016.00214/full#supplementary-material
References
Akaike, H. (1974). A new look at the statistical model identification. IEEE Trans. Automat. Control 19, 716–723. doi: 10.1109/TAC.1974.1100705
Azam, F., Fenchel, T., Field, J. G., Gray, J. S., Meyer-Reil, L. A., and Thingstad, F. (1983). The ecological role of water-column microbes in the sea. Mar. Ecol. Prog. Ser, 10, 257–263.
Baines, S. B., and Pace, M. L. (1991). The production of dissolved organic matter by phytoplankton and its importance to bacteria: patterns across marine and freshwater systems. Limnol. Oceanogr. 36, 1078–1090. doi: 10.4319/lo.1991.36.6.1078
Billen, G., and Becquevort, S. (1991). Phytoplankton-bacteria relationship in the Antarctic marine ecosystem. Polar Res. 10, 245–254.
Billen, G., Servais, P., and Becquevort, S. (1990). Dynamics of bacterioplankton in oligotrophic and eutrophic aquatic environments: bottom-up or top-down control? Hydrobiologia 207, 37–42. doi: 10.1038/nn.4417
Bird, D. F., and Karl, D. M. (1999). Uncoupling of bacteria and phytoplankton during the austral spring bloom in Gerlache Strait, Antarctic Peninsula. Aquatic Microb. Ecol. 19, 13–27. doi: 10.3354/ame019013
Bowman, J. S., and Ducklow, H. W. (2015). Microbial communities can be described by metabolic structure: a general framework and application to a seasonally variable, depth-stratified microbial community from the coastal west antarctic peninsula. PLoS ONE 10:e0135868. doi: 10.1371/journal.pone.0135868
Brum, J. R., Hurwitz, B. L., Schofield, O., Ducklow, H. W., and Sullivan, M. B. (2015). Seasonal time bombs: dominant temperate viruses affect Southern Ocean microbial dynamics. ISME J. 10, 437–449. doi: 10.1038/ismej.2015.125
Burnham, K. P., and Anderson, D. R. (2004). Multimodel inference understanding AIC and BIC in model selection. Sociol. Methods Res. 33, 261–304. doi: 10.1177/0049124104268644
Calvo-Díaz, A., Franco-Vidal, L., and Morán, X. A. G. (2014). Annual cycles of bacterioplankton biomass and production suggest a general switch between temperature and resource control in temperate coastal ecosystems. J. Plankton Res. 36, 859–865. doi: 10.1093/plankt/fbu022
Carlson, C. A., Ducklow, H. W., and Hansel, D. A. (1998). Organic carbon partitioning during spring phytoplankton blooms in the Ross Sea polynya and the Sargasso Sea. Oceanography 43, 375–386.
Carlson, C. A., Hansell, D. A., Peltzer, E. T., and Smith, W. O. (2000). Stocks and dynamics of dissolved and particulate organic matter in the southern Ross Sea, Antarctica. Deep Sea Res II 47, 3201–3225. doi: 10.1016/S0967-0645(00)00065-5
Church, M. J., DeLong, E. F., Ducklow, H. W., Karner, M. B., Preston, C. M., and Karl, D. M. (2003). Abundance and distribution of planktonic Archaea and Bacteria in the waters west of the Antarctic Peninsula. Limnol. Oceanogr. 48, 1893–1902.
Cook, A. J., Fox, A. J., Vaughan, D. G., and Ferrigno, J. G. (2005). Retreating glacier fronts on the Antarctic Peninsula over the past half-century. Science 308, 541–544. doi: 10.1126/science.1104235
Davis, J., and Benner, R. (2005). Seasonal trends in the abundance, composition and bioavailability of particulate and dissolved organic matter in the Chukchi/Beaufort Seas and western Canada Basin. Deep Sea Res. II 52, 3396–3410. doi: 10.1016/j.dsr2.2005.09.006
Ducklow, H. (1992). Factors regulating bottom-up control of bacteria biomass in open ocean plankton communities. Arch. Hydrobiol. Beih. Ergebn. Limnol. 37, 207–217.
Ducklow, H., Carlson, C., Church, M., Kirchman, D., Smith, D., and Steward, G. (2001). The seasonal development of the bacterioplankton bloom in the Ross Sea, Antarctica, 1994-1997. Deep Sea Res. II 48, 4199–4221. doi: 10.1016/S0967-0645(01)00086-8
Ducklow, H. W., Dickson, M. L., Kirchman, D. L., Steward, G., Orchardo, J., Marra, J., et al. (2000). Constraining bacterial production, conversion efficiency and respiration in the Ross Sea, Antarctica, January–February, 1997. Deep Sea Res. II Top. Stud. Oceanogr. 47, 3227–3247.
Ducklow, H. W., Myers, K. M., Erickson, M., Ghiglione, J. F., and Murray, A. E. (2011). Response of a summertime Antarctic marine bacterial community to glucose and ammonium enrichment. Aquat. Microb. Ecol. 64, 205–220. doi: 10.3354/ame01519
Ducklow, H. W. (1983). Production and fate of bacteria in the oceans. Bioscience 33, 494–501. doi: 10.2307/1309138
Ducklow, H. W. (2003). Seasonal production and bacterial utilization of DOC in the Ross Sea, Antarctica. Biogeochem. Ross Sea 143–157.
Ducklow, H. W., Schofield, O., Vernet, M., Stammerjohn, S., and Erickson, M. (2012). Multiscale control of bacterial production by phytoplankton dynamics and sea ice along the western Antarctic Peninsula: a regional and decadal investigation. J. Mar. Syst. 98, 26–39. doi: 10.1016/j.jmarsys.2012.03.003
Dufour, P. H., and Torreton, J. P. (1996). Bottom-up and top-down control of bacterioplankton from eutrophic to oligotrophic sites in the tropical northeastern Atlantic Ocean. Deep Sea Res. I 8, 1305–1320. doi: 10.1016/0967-0637(96)00060-X
Fortier, M., Fortier, L., Michel, C., and Legendre, L. (2002). Climatic and biological forcing of the vertical flux of biogenic particles under seasonal Arctic sea ice. Mar. Ecol. Prog. Ser. 225, 1–16. doi: 10.3354/meps225001
Fukuda, R., Ogawa, H., Nagata, T., and Koike, I. (1998). Direct determination of carbon and nitrogen contents of natural bacterial assemblages in marine environments. Appl. Environ. Microbiol. 64, 3352–3358.
Garibotti, I. A., Vernet, M., Smith, R. C., and Ferrario, M. E. (2005). Marine phytoplankton distribution during three summers in the seasonal sea-ice zone west of the Antarctic Peninsula. J. Plankton Res. 27, 825–843. doi: 10.1093/plankt/fbi056
Garneau, M. È., Roy, S., Lovejoy, C., Gratton, Y., and Vincent, W. F. (2008). Seasonal dynamics of bacterial biomass and production in a coastal arctic ecosystem: Franklin Bay, western Canadian Arctic. J. Geophys. Res. Oceans 113:C07S91. doi: 10.1029/2007JC004281
Garzio, L. M., Steinberg, D. K., Erickson, M., and Ducklow, H. W. (2013). Microzooplankton grazing along the Western Antarctic Peninsula. Aquat. Microb. Ecol. 70, 215–232.
Gasol, J. M., and del Giorgio, P. A. (2000). Using flow cytometry for counting natural planktonic bacteria and understanding the structure of planktonic bacterial communities. Sci. Mar. 64, 197–224. doi: 10.3989/scimar.2000.64n2197
Gasol, J. M., Pedrós-Alió, C., and Vaqué, D. (2002). Regulation of bacterial assemblages in oligotrophic plankton systems: results from experimental and empirical approaches. Antonie Van Leeuwenhoek, 81, 435–452. doi: 10.1023/A:1020578418898
Gasol, J. M., Zweifel, U. L., Peters, F., Fuhrman, J. A., and Hagström, Å. (1999). Significance of size and nucleic acid content heterogeneity as measured by flow cytometry in natural planktonic bacteria. Appl. Environ. Microbiol. 65, 4475–4483.
Guixa-Boixereu, N., Vaqué, D., Gasol, J. M., Sánchez-Cámara, J., and Pedrós-Alió, C. (2002). Viral distribution and activity in Antarctic waters. Deep Sea Res. II 49, 827–845. doi: 10.1016/S0967-0645(01)00126-6
Hale, M. S., Rivkin, R. B., Matthews, P., Agawin, N. S., and Li, W. K. (2006). Microbial response to a mesoscale iron enrichment in the NE subarctic Pacific: heterotrophic bacterial processes. Deep Sea Res. II 53, 2231–2247. doi: 10.1016/j.dsr2.2006.05.039
Hood, E., Battin, T. J., Fellman, J., O'Neel, S., and Spencer, R. G. (2015). Storage and release of organic carbon from glaciers and ice sheets. Nat. Geosci. 8, 91–96. doi: 10.1038/ngeo2331
Hood, E., Fellman, J., Spencer, R. G., Hernes, P. J., Edwards, R., D'Amore, D., et al. (2009). Glaciers as a source of ancient and labile organic matter to the marine environment. Nature 462, 1044–1047. doi: 10.1038/nature08580
Kähler, P., Bjornsen, P. K., Lochte, K., and Antia, A. (1997). Dissolved organic matter and its utilization by bacteria during spring in the Southern Ocean. Deep Sea Res. II Top. Stud. Oceanogr. 44, 341–353.
Kaplan, A., Kushnir, Y., Cane, M. A., and Blumenthal, M. B. (1997). Reduced space optimal analysis for historical data sets: 136 years of Atlantic sea surface temperatures. J. Geophys. Res. Oceans 102, 27835–27860. doi: 10.1029/97JC01734
Karl, D. M., and Tien, G. (1991). Bacterial abundances during the 1989-1990 austral summer phytoplankton bloom in the Gerlache Strait. Antarct. J. U.S.A. 26, 147–149.
Karl, D. M., Holm-Hansen, O., Taylor, G. T., Tien, G., and Bird, D. F. (1991). Microbial biomass and productivity in the western Bransfield Strait, Antarctica during the 1986–87 austral summer. Deep Sea Res. A 38, 1029–1055. doi: 10.1016/0198-0149(91)90095-W
Kavanaugh, M. T., Abdala, F. N., Ducklow, H. W., Glover, D. M., Fraser, W. R., Martinson, D. G., et al. (2015). Effect of continental shelf canyons on phytoplankton biomass and community composition along the western Antarctic Peninsula. Mar. Ecol. Prog. Ser. 524, 11–26.
Kim, H., Doney, S. C., Iannuzzi, R. A., Meredith, M. P., Martinson, D. G., and Ducklow, H. W. (2016). Climate forcing for dynamics of dissolved inorganic nutrients at Palmer Station, Antarctica: an interdecadal (1993–2013) analysis. J. Geophys. Res. 121, 2369–2389.
Kirchman, D. L., Hill, V., Cottrell, M. T., Gradinger, R., Malmstrom, R. R., and Parker, A. (2009a). Standing stocks, production, and respiration of phytoplankton and heterotrophic bacteria in the western Arctic Ocean. Deep Sea Res. II 56, 1237–1248. doi: 10.1016/j.dsr2.2008.10.018
Kirchman, D. L., Malmstrom, R. R., and Cottrell, M. T. (2005). Control of bacterial growth by temperature and organic matter in the Western Arctic. Deep Sea Res. II 52, 3386–3395. doi: 10.1016/j.dsr2.2005.09.005
Kirchman, D. L., Meon, B., Ducklow, H. W., Carlson, C. A., Hansell, D. A., and Steward, G. F. (2001). Glucose fluxes and concentrations of dissolved combined neutral sugars (polysaccharides) in the Ross Sea and Polar Front Zone, Antarctica. Deep Sea Res. II Top. Stud. Oceanogr. 48, 4179–4197.
Kirchman, D. L., Morán, X. A. G., and Ducklow, H. (2009b). Microbial growth in the polar oceans—role of temperature and potential impact of climate change. Nat. Rev. Microbiol. 7, 451–459. doi: 10.1038/nrmicro2115
Lancelot, C., Billen, G., Veth, C., Becquevort, S., and Mathot, S. (1991). Modelling carbon cycling through phytoplankton and microbes in the Scotia—Weddell Sea area during sea ice retreat. Mar. Chem. 35, 305–324. doi: 10.1016/s0304-4203(09)90024-x
Liu, J., Curry, J. A., and Martinson, D. G. (2004). Interpretation of recent Antarctic sea ice variability. Geophys. Res. Lett. 31:L02205. doi: 10.1029/2003gl018732
Lubin, D., Wittenmyer, R. A., Bromwich, D. H., and Marshall, G. J. (2008). Antarctic Peninsula mesoscale cyclone variability and climatic impacts influenced by the SAM. Geophys. Res. Lett. 35:L02808. doi: 10.1029/2007GL032170
Luria, C. M., Amaral-Zettler, L. A., Ducklow, H. W., and Rich, J. J. (2016). Seasonal succession of free-living bacterial communities in coastal waters of the Western Antarctic Peninsula. Front. Microbiol. 7:1731.
Luria, C. M., Ducklow, H. W., and Amaral-Zettler, L. A. (2014). Marine bacterial, archaeal and eukaryotic diversity and community structure on the continental shelf of the western Antarctic Peninsula. Aquat. Microb. Ecol. 37, 107–121. doi: 10.3354/ame01703
Martinson, D. G., Stammerjohn, S. E., Iannuzzi, R. A., Smith, R. C., and Vernet, M. (2008). Western Antarctic Peninsula physical oceanography and spatio–temporal variability. Deep Sea Res. II 55, 1964–1987. doi: 10.1016/j.dsr2.2008.04.038
McQueen, D. J., Post, J. R., and Mills, E. L. (1986). Trophic relationships in freshwater pelagic ecosystems. Can. J. Fish. Aquat. Sci. 43, 1571–1581. doi: 10.1139/f86-195
Mitchell, B. G., Brody, E. A., Holm-Hansen, O., McClain, C., and Bishop, J. (1991). Light limitation of phytoplankton biomass and macronutrient utilization in the Southern Ocean. Limnol. Oceanogr. 36, 1662–1677. doi: 10.4319/lo.1991.36.8.1662
Moline, M. A., Claustre, H., Frazer, T. K., Grzymski, J., and Vernet, M. (2000). “Changes in phytoplankton assemblages along the Antarctic Peninsula and potential implications for the Antarctic food web,” in Antarctic Ecosystems: Models for Wider Ecological Understanding, eds C. Howard-Williams, P. Broady, W. Davison (caldwell, ID: Caxton Press). 263–271.
Morán, X. A. G., Calvo-Díaz, A., and Ducklow, H. W. (2010). Total and phytoplankton mediated bottom-up control of bacterioplankton change with temperature in NE Atlantic shelf waters. Aqua. Microb. Ecol. 58, 229–239. doi: 10.3354/ame01374
Morán, X. A. G., Gasol, J. M., Pedrós-Alió, C., and Estrada, M. (2001). Dissolved and particulate primary production and bacterial production in offshore Antarctic waters during austral summer: coupled or uncoupled? Mar. Ecol. Prog. Ser. 222, 25–39. doi: 10.1016/j.nbt.2016.10.008
Morán, X. A. G., and Estrada, M. (2002). Phytoplanktonic DOC and POC production in the Bransfield and Gerlache Straits as derived from kinetic experiments of 14C incorporation. Deep Sea Res. II 49, 769–786. doi: 10.1016/S0967-0645(01)00123-0
Müller, S., Anssi, V. V., Colin, A. S., Mats, A. G., Louiza, N., Shazia, N. A., et al. (2013). Selective incorporation of dissolved organic matter (DOM) during sea ice formation. Mar. Chem. 155, 148–157. doi: 10.1016/j.marchem.2013.06.008
Nagata, T. (2000). “Production mechanisms of dissolved organic matter”, in Microbial Ecology of the Oceans eds D. L. Kirchmann (New York, NY: Wiley Series in Ecological and Applied Microbiology), 121–152.
Nikrad, M. P., Cottrell, M. T., and Kirchman, D. L. (2014). Growth activity of gammaproteobacterial subgroups in waters off the west Antarctic Peninsula in summer and fall. Environ. Microbiol. 16, 1513–1523.
O'Brien, R. M. (2007). A caution regarding rules of thumb for variance inflation factors. Qual. Quant. 41, 673–690.
Pomeroy, L. R., and Wiebe, W. J. (2001). Temperature and substrates as interactive limiting factors for marine heterotrophic bacteria. Aquat. Microb. Ecol. 23, 187–204. doi: 10.3354/ame023187
Ross, R. M., Quetin, L. B., Martinson, D. G., Iannuzzi, R. A., Stammerjohn, S. E., and Smith, R. C. (2008). Palmer LTER: patterns of distribution of five dominant zooplankton species in the epipelagic zone west of the Antarctic Peninsula, 1993–2004. Deep Sea Res. II 55, 2086–2105. doi: 10.1016/j.dsr2.2008.04.037
Sailley, S, F., Ducklow, H. W., Moeller, H. V., Fraser, W. R., Schofield, O. M., Steinberg, D. K., et al. (2013). Carbon fluxes and pelagic ecosystem dynamics near two western Antarctic Peninsula Adélie penguin colonies: an inverse model approach. 492, 253–272. doi: 10.3354/meps10534
Saba, G. K., Fraser, W. R., Saba, V. S., Iannuzzi, R. A., Coleman, K. C., Doney, S. C., et al. (2014). Winter and spring controls on the summer food web of the coastal West Antarctic Peninsula. Nat. Commun. 5:4318. doi: 10.1038/ncomms5318
Schloss, I. R., Abele, D., Moreau, S., Demers, S., Bers, A. V., González, O., et al. (2012). Response of phytoplankton dynamics to 19-year (1991–2009) climate trends in Potter Cove (Antarctica). J. Mar. Syst. 92, 53–66. doi: 10.1016/j.jmarsys.2011.10.006
Shiah, F. K., Gong, G. C., and Chen, C. C. (2003). Seasonal and spatial variation of bacterial production in the continental shelf of the East China Sea: possible controlling mechanisms and potential roles in carbon cycling. Deep Sea Res II 50, 1295–1309. doi: 10.1016/S0967-0645(03)00024-9
Shiah, F.-K., and Ducklow, H. W. (1995). Regulation of bacterial abundance and production by substrate supply and bacterivory: a mesocosm study. Microb. Ecol. 30, 239–256. doi: 10.1007/BF00171932
Smith, D. C., and Azam, F. (1992). A simple, economical method for measuring bacterial protein synthesis rates in seawater using 3H-leucine. Mar. Microb. Food Webs 6, 107–114.
Smith, R. C., Baker, K. S., and Vernet, M. (1998). Seasonal and interannual variability of phytoplankton biomass west of the Antarctic Peninsula. J. Mar. Syst. 17, 229–243. doi: 10.1016/S0924-7963(98)00040-2
Smith, R. C., Martinson, D. G., Stammerjohn, S. E., Iannuzzi, R. A., and Ireson, K. (2008). Bellingshausen and western Antarctic Peninsula region: pigment biomass and sea-ice spatial/temporal distributions and interannual variabilty. Deep Sea Res. II 55, 1949–1963. doi: 10.1016/j.dsr2.2008.04.027
Stammerjohn, S. E., Martinson, D. G., Smith, R. C., Yuan, X., and Rind, D. (2008). Trends in Antarctic annual sea ice retreat and advance and their relation to El Niño–Southern Oscillation and Southern Annular Mode variability. J. Geophys. Res. 108:C03S90. doi: 10.1029/2007JC004269
Straza, T. R., Cottrell, M. T., Ducklow, H. W., and Kirchman, D. L. (2009). Geographic and phylogenetic variation in bacterial biovolume as revealed by protein and nucleic acid staining. Appl. Environ. Microbiol. 75, 4028–4034. doi: 10.1128/AEM.00183-09
Strom, S. L., Benner, R., Ziegler, S., and Dagg, M. J. (1997). Planktonic grazers are a potentially important source of marine dissolved organic carbon. Limnol. Oceanogr. 42, 1364–1374. doi: 10.4319/lo.1997.42.6.1364
Stukel, M. R., Asher, E., Couto, N., Schofield, O., Strebel, S., Tortell, P., et al. (2015). The imbalance of new and export production in the Western Antarctic Peninsula, a potentially “leaky” ecosystem. Global Biogeochem. Cycles 29, 1400–1420.
Tortell, P. D., Asher, E. C., Ducklow, H. W., Goldman, J. A., Dacey, J. W., Grzymski, J. J., et al. (2014). Metabolic balance of coastal Antarctic waters revealed by autonomous pCO2 and ΔO2/Ar measurements. Geophys. Res. Lett. 41, 6803–6810.
Tupas, L. M., Koike, I., Karl, D. M., and Holm-Hansen, O. (1994). Nitrogen metabolism by heterotrophic bacterial assemblages in Antarctic coastal waters. Polar Biol. 14, 195–204. doi: 10.1007/BF00240524
Vaughan, D. G. (2006). Recent trends in melting conditions on the Antarctic peninsula and their implications for ice-sheet mass balance and sea level. Arct. Antarct. Alp. Res. 38, 147–152. doi: 10.1657/1523-0430(2006)038[0147:RTIMCO]2.0.CO;2
Venables, H. J., Clarke, A., and Meredith, M. P. (2013). Wintertime controls on summer stratification and productivity at the western Antarctic Peninsula. Limnol. Oceanogr. 58, 1035–1047. doi: 10.4319/lo.2013.58.3.1035
Keywords: heterotrophic bacteria, bacterial production, bacterial biomass, phytoplankton, the western Antarctic Peninsula, Palmer LTER, climate change
Citation: Kim H and Ducklow HW (2016) A Decadal (2002–2014) Analysis for Dynamics of Heterotrophic Bacteria in an Antarctic Coastal Ecosystem: Variability and Physical and Biogeochemical Forcings. Front. Mar. Sci. 3:214. doi: 10.3389/fmars.2016.00214
Received: 01 August 2016; Accepted: 14 October 2016;
Published: 04 November 2016.
Edited by:
Ingrid Obernosterer, Observatoire Océanologique de Banyuls-sur-Mer (CNRS), FranceReviewed by:
Michael R. Twiss, Clarkson University, USAMeinhard Simon, University of Oldenburg, Germany
Copyright © 2016 Kim and Ducklow. This is an open-access article distributed under the terms of the Creative Commons Attribution License (CC BY). The use, distribution or reproduction in other forums is permitted, provided the original author(s) or licensor are credited and that the original publication in this journal is cited, in accordance with accepted academic practice. No use, distribution or reproduction is permitted which does not comply with these terms.
*Correspondence: Hyewon Kim, hyewon@ldeo.columbia.edu