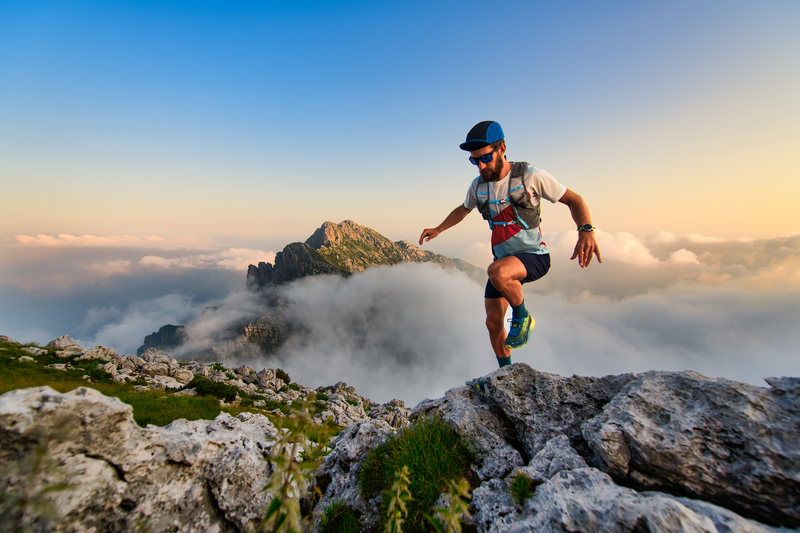
94% of researchers rate our articles as excellent or good
Learn more about the work of our research integrity team to safeguard the quality of each article we publish.
Find out more
REVIEW article
Front. Mar. Sci. , 28 September 2016
Sec. Marine Ecosystem Ecology
Volume 3 - 2016 | https://doi.org/10.3389/fmars.2016.00182
This article is part of the Research Topic Bridging the Gap Between Policy and Science in Assessing the Health Status of Marine Ecosystems View all 31 articles
In Europe and around the world, the approach to management of the marine environment has developed from the management of single issues (e.g., species and/or pressures) toward holistic Ecosystem Based Management (EBM) that includes aims to maintain biological diversity and protect ecosystem functioning. Within the European Union, this approach is implemented through the Marine Strategy Framework Directive (MSFD, 2008/56/EC). Integrated Ecosystem Assessment is required by the Directive in order to assess Good Environmental Status (GES). Ecological modeling has a key role to play within the implementation of the MSFD, as demonstrated here by case studies covering a range of spatial scales and a selection of anthropogenic threats. Modeling studies have a strong role to play in embedding data collected at limited points within a larger spatial and temporal scale, thus enabling assessments of pelagic and seabed habitat. Furthermore, integrative studies using food web and ecosystem models are able to investigate changes in food web functioning and biological diversity in response to changes in the environment and human pressures. Modeling should be used to: support the development and selection of specific indicators; set reference points to assess state and the achievement of GES; inform adaptive monitoring programs and trial management scenarios. The modus operandi proposed shows how ecological modeling could support the decision making process leading to appropriate management measures and inform new policy.
The Ecosystem Based Management (EBM) approach to marine conservation and sustainable use of the natural environment has been promoted by international conventions (e.g., Convention on Biological Diversity UNEP, 1998; CBD, 2014), national legislation across Europe and beyond (Kidd et al., 2011) and global scientific organizations such as the International Council for the Exploration of the Sea (ICES) that provide evidence and advice. EBM recognizes the need to take a holistic approach to understanding ecosystem level change, including explicitly accounting for the governance structures involved in interpreting, enacting and enforcing legislation (Borgström et al., 2015). Tightly linked to these aims, the Marine Strategy Framework Directive (MSFD, 2008/56/EC; European Commission, 2008) aims to achieve Good Environmental Status (GES) for the marine waters within the EU by 2020. During the first cycle of the MSFD (2012–2018), EU Member States prepared initial assessments of their marine waters (Article 8), determined characteristics for GES (Article 9), established environmental targets and associated indicators (Article 10), and established monitoring programs for the ongoing assessment of the environmental status of their marine waters (Article 11). Programs of measures have been identified that will provide the mechanism for changing the system to achieve the individual targets, and the overall aim of GES. EU Member States are required to review each element of the marine strategy every 6 years after their initial establishment (Article 17).
Although the fundamental statistical mechanics of ecosystems are an area of ongoing research (Rodríguez et al., 2012; Rossberg, 2013) many developments have been made by the scientific community in terms of modeling and indicator development (Shin et al., 2012; Piroddi et al., 2015a). Ecological models (hereafter “models”), including a range of conceptual, mathematical, and statistical representations of ecosystem components and processes (e.g., Peck et al., 2016), have an important role to play in the assessment and management cycle. Models provide the means to test how different trophic levels and the biogeochemistry of marine systems respond under specific scenarios of management (e.g., fisheries, Allen and Clarke, 2007) and environmental change (e.g., climate, Artioli et al., 2014). Further, ecosystem models can be used to drive distribution models for species in higher trophic levels, allowing the exploration of management and change scenarios (e.g., fisheries, harmful algal blooms, Gilbert et al., 2010; Sumaila et al., 2015).
A number of studies have shown how ecosystem modeling could support the assessment of different ecosystem components and pressures in several marine regions and also where models require further development (Hyder et al., 2015; Piroddi et al., 2015a; Tedesco et al., 2016; Rossberg et al., 2017). Here we advance on this body of work and demonstrating that modeling is not only useful for the assessment of components, but throughout the entire assessment cycle of the MSFD (Figure 1). Within the MSFD there are 11 themes or features that describe GES, termed descriptors within the directive (hereafter “D,” Annex I, MSFD). Four of these are strongly linked to biological diversity: biological diversity (D1), non-indigenous species (D2), food webs (D4), and seafloor integrity (D6), all of which have the potential to be addressed using models (Piroddi et al., 2015a). The impacts of human activities on the ecosystem can also be addressed using models, particularly those linked to the pressure descriptors commercial fish and shellfish (D3), eutrophication (D5), and hydrological changes (D7). Although developments vary considerably between MSFD descriptors and assessment regions, some descriptors are well-addressed by ecosystem models in all regions (e.g., D4 food webs), and some remain poorly addressed (e.g., D2-non-indigenous species and D6-seafloor integrity; Piroddi et al., 2015a). Models may not always address biological diversity in a traditional sense (species richness and evenness, Tedesco et al., 2016), but they can be used to address simplified representations of natural biological diversity in relation to seafloor integrity and ecosystem functioning (Queirós et al., 2015).
Figure 1. Assessments of Good Environmental Status are made as part of an adaptive management approach with 6-yearly cycle and require modeling support at each step (key aims of the modeling required are presented in the white bubbles within the structure of the MSFD).
Through a selection of case studies, this paper demonstrates how modeling can be used throughout the MSFD assessment cycle. Specifically, in the development and selection of indicators, identification of reference points, informing monitoring programs, assessing ecosystem state, and changes in functioning and trialing management scenarios. We propose a modus operandi through which ecosystem modeling can support the decision-making process leading to appropriate management measures and inform new policy.
The MSFD follows an adaptive management approach with Marine Strategies that must be reviewed every 6 years (Figure 1). Assessing and maintaining GES requires an understanding of the link between pressures on the marine environment and the state of the environment. Marine systems, however, are subject to multiple pressures and the resulting functioning of the system is also influenced by long term climate change such that the expected outcome of management actions is difficult to project. Integrative modeling tools allow researchers to investigate the processes operating in the system and the likely responses of ecosystem components to potential management measures given the prevailing climate.
Indicators are metrics used to determine the state of the ecosystem and to detect changes that occur due to anthropogenic or environmental impacts on the ecosystem. In the specific case of the MSFD, indicators need to be applicable to the descriptors (e.g., biological diversity) and pressures (e.g., fishing, pollution, etc.) that are explicitly listed in the directive (European Commission, 2008). Monitoring programs must be designed to provide data for indicator assessments (ICES, 2016a; Patrício et al., 2016). This is a fundamental step in measuring progress toward targets and evaluating the effectiveness of measures employed to achieve or maintain GES. In addition to measuring the current characteristics of the ecosystem, monitoring programs should consider the wider context within which indicators are measured (such as climate change and the risk of invasions of non-indigenous species). However, ecosystems are complex and we cannot measure “everything everywhere.” Rather, evaluation of trade-offs in monitoring different ecosystem state indicators (Kupschus et al., 2016) and potentially additional pressures (not listed in the MSFD) that modulate or confound these changes is needed (Queirós et al., 2016a). By explicitly linking ecosystem model development to MSFD monitoring program development, modeling can assist by:
• Informing where sampling is required to improve precision of measurements of state and be able to detect change in ecosystem structure (habitats, communities, and connectivity; ICES, 2016a).
• Evaluating where there is a risk of change in biological diversity and ecosystem function (e.g., Pinnegar et al., 2014; Katsanevakis et al., 2016).
• Informing upon which indicators should be assessed to maximize gains in understanding ecosystem processes (Möllmann et al., 2008), improving our ability to give suitable scientific advice in future.
An important step toward EBM is the selection of suitable indicators from a range of proposed options according to objective criteria regarding their scientific standard and applicability to the MSFD (Queirós et al., 2016a). Some of these criteria, such as the quantification of pressure-response relationships, can be evaluated using models. For example, indicators addressing disturbance of marine fish community structure through exploitation (i.e., fishing, a physical pressure) have been investigated (Houle et al., 2012). The indicators evaluated were derived from simulated catch or survey data, for example, for the body mass or current length of individual fish, their expected length at first maturation, or trophic level, which were aggregated according to a number of formulae proposed in the literature (see Houle et al., 2012 and references therein). The model used described interactions between size-structured fish populations with various maturation body sizes. Specificity to fishing was evaluated in comparison to indicators responses to small random model parameter variation, representing, e.g., environmental change. While not identifying a unique “winner,” this analysis revealed clear differences in both sensitivity and specificity among proposed indicators. These results later informed indicator selection (ICES, 2012).
Assessments based on indicators are required by the MSFD at multiple levels: ecosystem, habitats (including their associated communities) and species, and the spatial scale of these assessments must be ecologically meaningful and relevant to the pressures on the ecosystem. Ideally, indicator assessments would support simple advice on status in relation to reference points (thresholds). However, when such assessment reference points are not available, identification of desirable directions of change can be useful (i.e., “reference directions” Jennings and Dulvy, 2005. For indicators where the pressure-state relationship is unknown but the property is considered important to monitor indicators can be used for “surveillance purposes” (Shephard et al., 2015a). Examples are given in the following section for indicators of biological diversity, food webs, sea-floor integrity, and non-indigenous species. There is a risk that the pressure to implement and fulfill legislative requirements could affect the entire process of assessment. Acknowledgment of uncertainty (in both data and models; Carstensen and Lindegarth, 2016; Payne et al., 2016; Peck et al., 2016), recognition of coupled social-ecological systems and that decisions reflect societal choice, and the acknowledgment of these trade-offs are therefore needed in GES indicator development (Long et al., 2015). Models can support these aims, as exemplified below.
The value of coupled biogeochemical-physical ocean models with realistic simulations of phytoplankton responses can be seen in many examples of applied ecology. Aldridge et al. (2012) used a variant of the European Regional Seas Ecosystem Model (ERSEM, Butenschön et al., 2015) to examine the effects of a large-scale seaweed farm in the northern North Sea. Compared to control runs, the model with a seaweed farm displayed altered phytoplankton composition at distances of up to 100 km. The impacts of other large-scale marine engineering projects such as the construction of wind farms at multiple sites in the North Sea can also be probed using biogeochemical models (van der Molen et al., 2014).
Within the DEVOTES project, a case study has been performed in the Bay of Biscay to investigate the potential to estimate chlorophyll-a by each of two bio-optical models applied to MODIS-AQUA imagery for the assessment of status and trends, and to support the definition of reference values and targets for chlorophyll concentration in the water column, as an indicator that responds to eutrophication and suitable for the MSFD D5 (human-induced eutrophication). These data may also be potentially used to support other indicators under other descriptors, such as those relating to pelagic habitat structure within D1 or Harmful Algal Blooms (HABs) within D5. This study revisits and updates the work performed by Novoa et al. (2012) by extending the dataset to 2014. However, satellite data also have limitations and uncertainties (Hooker and McClain, 2000). Firstly, only surface layers are sensed so subsurface peaks of chlorophyll may be missed (Jacox et al., 2013). Bio-optical algorithms perform well in waters where the main optical constituent is phytoplankton, but the accuracy decreases in waters with more optical constituents such as dissolved or suspended matter.
The 90th percentile of chlorophyll-a values were evaluated over the defined growing season in a 6 year sliding window. This indicator is already used by the Water Framework Directive (WFD, 2000/60/EC) and is a candidate for the MSFD (Ferreira et al., 2010). The modeled values were compared, using MODIS-AQUA data with the OC5 (Gohin et al., 2002) and OCI (Hu et al., 2012) bio-optical algorithms, to traditional in situ datasets from the Basque Littoral Monitoring Network (Revilla et al., 2009; Figure 2). The reference values applied are those set by the North Eastern Atlantic geographical inter-calibration group for the coastal water type “Spain North East Cantabrian,” as these target chlorophyll concentrations/ranges are determined locally for different water types and water categories, based on the results of the inter-calibration exercises (European Commission, 2013). Despite the improvements of the algorithms to better estimate chlorophyll-a values, the overestimation of satellite estimations (particularly in values higher than 1 mg per m3) result in significant differences in assessments based on the 90th percentile indicator: where the in situ dataset classifies water bodies as “high quality” class, the MODIS-AQUA OC5 satellite dataset classifies them in “good quality” and the MODIS-Aqua OCI dataset algorithm) in “poor quality” class. However, statistics other than the 90th percentile such as the median or averages are less sensitive to the inaccuracies and classifications from these statistics are agree with the in situ data.
Figure 2. Location of the area of study. (A) Western Europe overlaid with the P90-chla calculated with the MODIS-Aqua OC5 dataset between 2003 and 2013. (B) Detailed information about the location of the sampled stations from the Basque Littoral Monitoring Network, overlaid with main rivers pouring into the coastal zone, WFD water bodies and the P90-chla calculated with the MODIS-Aqua OC5 dataset between 2003 and 2013.
The rate of production of new cells or carbon by phytoplankton is an important indicator for food webs, and as such, has been proposed for use under the MSFD for food webs (D4). Whilst the instantaneous rate of carbon fixation can be measured directly, the daily, seasonal or annual integral of production can be modeled as a function of phytoplankton biomass, underwater light, and photosynthetic activity (Smyth et al., 2005; Carr et al., 2006). Many models of primary production are available, from relatively simple empirical functions through to complex equations describing the underwater light field and plankton response in detail. Marine biogeochemical models such as the ERSEM and the Biogeochemical Flux Model (BFM, Vichi et al., 2015) contain complex productivity calculations in their core code, which, when driven by high quality atmospheric forcing data and accurate physical ocean responses, provide the ability to dynamically generate realistic inputs of new carbon in space and time.
Changes in marine primary production over annual to decadal periods may be driven by changes in underwater light availability, caused for example by increased sediment loading (Dupont and Aksnes, 2013; Capuzzo et al., 2015), or by changing nutrient concentrations or stoichiometry. A long-term study of modeled annual primary production in the eastern Scheldt estuary showed a decreasing trend between 1991 and 2011 (Smaal et al., 2013), which could not be related to changes in the dissolved nutrients or the concentration of suspended matter, but rather indicated an overgrazing of the larger, more active phytoplankton due to expanding aquaculture activities. Biogeochemical models such as ERSEM and food webs tools such as Ecopath with Ecosim (EwE, Christensen and Walters, 2004) could be deployed to investigate the wider ecosystem effects of such a prolonged decrease in overall production, and shift in prey size at the base of the food chain.
The links between primary production and fisheries production are now becoming well-established. Following pioneering work by Ryther (1969) and others, the recent availability of satellite-based primary production estimates for the global ocean has allowed size-based fisheries production models to be constructed for many large marine ecosystems (Jennings et al., 2008; Jennings and Collingridge, 2015; Fogarty et al., 2016). Further, work is required to regionalize satellite production algorithms for European seas, and to establish methods for the automated analysis of phytoplankton size-structure for use in size-based models suitable for marine policy purposes.
Modeling of physical habitats, their associated species and connectivity between them can contribute toward the identification of ecologically important areas in need of protection (Baker and Harris, 2012), and form the basis for designing cost-effective monitoring programs (De Jonge et al., 2006). A range of modeling tools (Piroddi et al., 2015a; Peck et al., 2016) have been developed to map habitats and species assemblages and include: distribution modeling techniques to predict the spatial patterns in species distribution, abundance and habitats using observations of environmental variables (e.g., bathymetric and seabed types distribution; Stephens and Diesing, 2015) and new techniques to model connectivity between communities (Chust et al., 2016). Statistical models, in particular, can generate outputs that are easy to communicate (Reiss et al., 2014) and provide information on the uncertainty in the estimates (Figure 3). Such uncertainty information can indicate where monitoring is required in order to reduce the variance in the distribution model, or if multiple indicators are supported by one monitoring program this can be optimized by minimizing a weighted average of the indicators' variances (Carstensen and Lindegarth, 2016). Representing model uncertainty spatially (Figure 3) is especially useful as the MSFD relies on spatial assessments and species distribution indicators will be directly affected by the quality of the data used to model distributions. The location and frequency of multiple-objective monitoring programs can be modeled and the power needed to detect change in given indicators can be assessed leading to operational decisions on how many data types can be collected whilst maintaining sufficient overall precision and accuracy (Shephard et al., 2015b). As an example the Cefas integrated ecosystem survey program in the western Channel collected multibeam data from which seabed conditions were inferred for the entire area. The modeling process revealed areas of high heterogeneity and low predictability which can be prioritized in future surveys to reduce uncertainty and improve the reliability of species distribution modeling and make actual changes in distribution and extent (related MSFD D1 indicators) more reliable.
Figure 3. High resolution multibeam sonar data collected opportunistically during a multi-year integrated ecosystem survey program (van der Kooij et al., 2011; ICES, 2015). (A) spatial plot of survey lines showing strength of returned acoustic signal (backscatter intensity, dB) revealing changes in the physical properties of the seabed; (B) prediction interval (PI) of modeled seabed acoustic intensity return using random forest regression on broadscale auxiliary environmental variables (e.g., Mascaro et al., 2014), areas with high prediction interval show greater variance and require higher resolution data or additional variables to reduce the uncertainty; (C) final modeled seabed acoustic intensity (dB) return map, revealing broadscale variability in the physical conditions and facilitating better planning of future survey activity.
Anthropogenic and environmental sources are major threats to marine ecosystems throughout the world (Naylor et al., 2000; Pauly et al., 2005; Diaz and Rosenberg, 2008). Effective marine resource management must take into account a variety of both current and future pressures on marine ecosystems, including fishing, eutrophication, climate change, and ocean acidification. This is explicit to the MSFD which considers that GES must be achieved with consideration for prevailing climatic conditions. Up to now, a large body of work has focused on the impact of single pressures on specific components of the marine environment, while the assessment of cumulative and synergetic effects of these threats remains poorly studied and such studies are now emerging (Link et al., 2010; Hobday and Pecl, 2014; Queirós et al., 2016b).
van Leeuwen et al. (2016) applied a modeling approach to examine the potential higher level effects of the impacts of climate change and ocean acidification on marine ecosystems. Ocean acidification research has been focused largely on individual species and changes in their local environment, and less frequently considered wider ecosystem and societal impacts (Doney et al., 2009; Griffith et al., 2012; Le Quesne and Pinnegar, 2012; Queirós et al., 2016b). Understanding the combined effects of direct (species level) and indirect (abiotic environment level) changes due to ocean acidification across the food webs are thus also critical to support the evidence base for management decisions. van Leeuwen et al. (2016) applied a coupled ecosystem model (consisting of a hydro-biogeochemical model and a higher trophic level size-based model) in the North Sea in three hydro-dynamically different sites: seasonally stratified, transition waters, and permanently mixed. Three different impacts affecting fishing yields were studied separately and in combination: climatic impacts (medium emission scenario), a proxy for abiotic impacts of ocean acidification (reduced pelagic nitrification), and a description of potential biological impacts of ocean acidification (reduced detritivore growth rate). Results showed a high regional variability and an overall shift toward more pelagic-oriented systems. Fisheries yields appeared to increase due the climate effects in large areas of the North Sea, but results indicated that ocean acidification could severely mediate this impact for permanently mixed areas. Although there is already evidence for a physiological response to ocean acidification, this does not necessarily lead to an ecosystem level response (Le Quesne and Pinnegar, 2012). Modeling tools used in this case study have enabled an indication of individual and combined effects of direct and indirect impacts of climate change and ocean acidification in a marine food web, and highlights that interactions between pressures can lead to less than or more than the additive response of the system.
Piroddi et al. (2015b) used an ecosystem modeling approach for a small area of the Mediterranean Sea (Amvrakikos Gulf, Greece) to assess temporal structural and functional changes of its ecosystem under the combined effect of anthropogenic pressures such as river runoff, fish farms, and fisheries. The model derived indicators highlighted a general degradation of the demersal compartments of the food web but a relative stability of the pelagic compartments. Since the model has showed a marginal role of local fishery in the Gulf's food web and on its dynamics, as also observed by other studies (Koutsikopoulos et al., 2008), eutrophication was considered the only major pressure affecting the system. Specifically, the model suggested that fish farms represented a secondary contribution to nutrients and organic matter to the Gulf, whereas the two major rivers were the main drivers of the Gulf eutrophication. Contrasting results were observed by Piroddi et al. (2010) for another area of the Mediterranean Sea (the Inner Ionian Sea Archipelago, Greece), which is extremely oligotrophic, not influenced by river run off and with a marginal low impact of fish farms. Here, model derived indicators showed a consistent decline with time while the demersal/pelagic biomass ratio and the mean trophic level of the catches have increased linearly. The model pointed to decline of small pelagic fish biomass, particularly sardines, the main target of the local fisheries, and an increase in biomass of demersal species as the likely cause of the change in the ecosystem. Despite the fact that changes in ocean productivity were observed in the area, the model suggested that the degradations of the system were mainly caused by intensive overexploitation of marine resources as suggested also by other studies (Tsikliras et al., 2013; Gonzalvo et al., 2014). Here, the modeling tool pointed strongly to the underlying causes for ecosystem level change and would be useful to managers attempting to improve the environmental state.
Single and combined effects in the North Sea food web were also studied by Lynam and Mackinson (2015) in this case focusing on the response of indicators to direct impacts of fishing and climate change. In the observation based model projections, community composition indicators (Large Species Index, mean maximum length) were found to respond to fishing. In contrast, the trophic level of fish and elasmobranchs was responsive to climate with a marginal effect of fishing only. Importantly, the modeled temperature effect suggested that the biomasses of certain trophic guilds (piscivores and bentho-piscivores) may be suppressed by warming and, if not taken into account during the setting of assessment thresholds, these indicators could conceivably not reach their desired levels due to climate effects. Modeling tools here facilitate scientific advice on the combined effects of fishing and climate impacts on the food web and can be used to demonstrate the likelihood of an indicator reaching its assessment threshold in the future given the prevailing climate and pressure.
Models can be used to investigate the environmental status of a system when prevailing conditions are far removed from those at present, as is expected to occur in the future ocean under global stressors such as warming and ocean acidification. By forecasting future ocean conditions, some models can thus help overcome traditional hurdles in forecasting ecosystem state based on observational data alone, which are bound to historical conditions (Barnsley, 2007; Szuwalski and Hollowed, 2016). Complexity in language choice in reporting modeling results and of the uncertainty associated with such projections has, at times, limited the uptake of the wealth of information generated by models by policy around the world (Hyder et al., 2015). The scientific community is now addressing this issue, for instance, through the use of lay language, more accessible to policy makers, in the expression of confidence attributed to modeling results (Pörtner et al., 2014). Further to this, the partitioning of sources of uncertainty in climate change impact projections, and the explicit assessment of their contributions, are paramount to improve the perception of confidence in modeling results in research-policy communication (Payne et al., 2016). For instance, though explicit recognition and quantification of how physical and biogeochemical model structure, initialization, internal variability, parametric, and scenario uncertainties are carried forward into fish distribution models (Gårdmark et al., 2013; Cheung et al., 2016; Payne et al., 2016) used to derive GES indicators such as those described here. These are important steps toward breaking down of uncertainty propagation and the attribution of confidence to modeling forecasts used to support policy. This effort is key to the uptake of modeling studies within the MSFD process too.
Ecological impacts of non-indigenous species (NIS) range from single-species interactions and reduction in individual fitness of native species to population declines, local extinctions, changes in community composition, and effects on entire ecosystem processes and wider ecosystem function (Blackburn et al., 2014; Katsanevakis et al., 2014). One of the MSFD requirements is to assess the consequences of pressures arising from NIS, through measurements of their impacts on the natural systems. A risk-based approach has been generally adopted by Regional Sea Conventions (RSC) and also by EU Member States in their MSFD initial assessments, but usually without clarification of the type of adverse effects in biological diversity or the magnitude of impacts observed (Micheli et al., 2013; Palialexis et al., 2014; Berg et al., 2015). Understanding, quantifying and mapping the impacts of invasive non-indigenous species across the seascape is a prerequisite for the efficient prioritization of actions to prevent new invasions or for developing mitigation measures (Katsanevakis et al., 2016). A new index CIMPAL (Katsanevakis et al., 2016) for measuring the cumulative impact of invasive alien species in the ecosystems provides a spatially explicit quantification of cumulative impacts. To illustrate the potential of ecological-niche modeling (ENM) in the cumulative impact index, DEVOTES used species distribution models (Kaschner et al., 2013) to create a vulnerability map for the whole the Mediterranean Sea under the current conditions, taking a trial group of 17 species (DEVOTES Deliverable 4.2 and Teixeira et al. unpublished). The CIMPAL index calculated using the future projections of these species distributions predicted an increase up to two and a half times the area likely to suffer the effects of cumulative impacts from multiple invasive NIS, with respect to the currently impacted area. Such trends can be easily linked to specific habitats, species or pathways of introduction, facilitating identification of ecosystem components, processes, and services more at risk. Early-warning indicators can be of utmost importance to identify vulnerable spots or preferential pathways of introduction (Thuiller et al., 2005; Hulme et al., 2008; Essl et al., 2015) and to anticipate a joint set of actions in target areas or sectorial activities. These ENM approaches are also effective tools to forecast changes in distribution of invasive NIS under large scale scenarios of climate change or addressing cross sectorial policies to better manage invasions pressures in the marine environment. Still, there are challenges to the use of these modeling approaches for effectively predicting distribution patterns of NIS in conservation and policy related contexts. For example, to obtain meaningful risk maps of the cumulative impact of invasive NIS it is required to consider the complete set of species targeted but, only recently, developments on multispecies distribution models are overcoming limitations of modeling for a large number of species (e.g., Fitzpatrick et al., 2011). Other relevant modeling developments aim at incorporating species co-occurrence data into a species distribution model (e.g., Pollock et al., 2014) or by integrating traits, namely dispersal strategies, into the modeling (e.g., Miller and Holloway, 2015).
Food web functioning can be investigated through the use of models that capture the complexity and diversity of trophic flows in an ecosystem. The ecological properties of a network of trophic flows can be characterized through Ecological Network Analysis (ENA, e.g., Ulanowicz, 1997). ENA aims to characterize the structure and the functioning of a food web through a set of indices that describe the connections between compartments through an analysis of the inputs and outputs of a compartment, the trophic structure (based on a linearization of the network), the rates of recycling, and the topology of the flows (how redundant/specialized the flows are). Numerical methods recently developed to allow the evaluation of ENA indices and their uncertainty, so that statistical tests can be made to compare changes in observed states or between simulated scenarios (Lassalle et al., 2014; Chaalali et al., 2015, 2016; Guesnet et al., 2015; Tecchio et al., 2016).
ENA indices have been proved useful to evaluate the impacts of human pressures on ecosystem functioning and to simulate likely impacts given climatic change scenarios. A change in ecosystem functioning was modeled by ENA when comparing the ecological network before and after the extension of the Le Havre harbor in the Seine Estuary (Tecchio et al., 2016). Adjacent to the harbor, the food web demonstrated increased detritivory and recycling and the likely cause was a combination of pressures, as human direct effects were associated with hydrological changes induced by climatic conditions.
Scenarios are particularly useful to study cumulative effects and disentangle effects from various pressures. For example, the Bay of Biscay ecosystem was studied to investigate the effect of climate change on the distribution of small pelagic fish and its consequences on food web functioning (Chaalali et al., 2016). Here, ENA analysis suggested that the ecosystem would adapt to the simulated increased production of small pelagic fish in the Bay of Biscay within 100 years and suggested that this fish group would transport carbon toward higher trophic levels. Model derived ENA indices can offer a unique view on change in the ecosystem as a whole and demonstrate promise as food web indicators.
Multiple stressors such as climate, fishing, eutrophication, and invasive species, have caused major reorganization of the aquatic ecosystem, and these have been interpreted as regime shifts in many areas including the Baltic, Black, and North Seas (Alheit et al., 2005; Möllmann et al., 2009; Diekmann and Möllmann, 2010; Lindegren et al., 2010, 2012; Llope et al., 2011). This reorganization is likely to be reflected in multiple MSFD descriptors, such as those of biological diversity, food webs, commercial seafood production, and seafloor integrity.
Anticipating regime shifts is difficult since these abrupt changes usually come as surprises (Doak et al., 2008). Recently, Big Data analytics has been employed to evaluate whether regime shifts could be predicted based on unexpected patterns in the data, i.e., anomaly detection. Models such as non-stationary dynamic Bayesian networks (Tucker and Liu, 2004; Robinson and Hartemink, 2009; Ceccon et al., 2011) can be employed to learn from past data but adapt to the fact that the relationships between the ecosystem components may change. This approach has its challenges in the ecological domain, where data is often relatively scarce, but some examples already exist (Trifonova et al., 2015). These models could help identify upcoming regime shifts based on data patterns, and could be used to inform models describing the underlying ecosystem processes.
It is important to note that systems that have experienced regime shifts often show hysteresis effects, i.e., reduction of external drivers need to have substantial stronger driver forcing to recover to the original state (Beisner et al., 2003; Scheffer et al., 2009). Although the existence of alternative ecosystem states is contentious (Cardinale and Svedäng, 2011; Möllmann et al., 2011), it is assumed that ecosystems that have experienced regime shifts have reorganized into novel states (e.g., in terms of species composition, population size, and species interaction strength), and the altered environmental and anthropogenic conditions may limit their recovery potential (Lotze et al., 2011). A recent example from the Baltic Sea is the apparent recovery of Eastern Baltic cod (Gadus morhua) predicted by linear, steady-state models (Eero et al., 2012), but challenged by food web models incorporating threshold dynamics (Blenckner et al., 2015a). This emphasized the need of constant evaluation and development of models, but also highlights that these processes can be incorporated into modeling frameworks.
When choosing management measures to attain GES, decision makers need to have a strong evidence base to understand the consequences of management options and make informed decisions given a cost-benefit analyses of the options. However, food web interactions are fundamental to any ecosystem such that food web models could be required to fully evaluate changes due to management. For example, Piroddi et al. (2011) used a higher trophic level model of the Inner Ionian Sea Archipelago (Greece) to assess reduction in fishing effort or total closure (e.g., no-take zone) for the main fleets operating in the area as a measure to recover a resident population of common dolphins. Results from forecast scenarios highlighted that closing the area only to the industrial sector would lead to an increase in forage fish and thus a gradual recovery of common dolphins, but by closing the entire area to fisheries (industrial and artisanal) a recovery of common dolphin would be more pronounced. Lynam and Mackinson (2015) modeled the response of the North Sea food web, and a suite of ecological indicators, given a climate change scenario and a strategy in which fisheries management measures may be implemented, in order to achieve maximum sustainable yield targets for fishing mortality associated with the Common Fisheries Policy (CFP; European Commission, 2013). The authors demonstrated that a reduction in fishing effort consistent with CFP targets, would contribute to the attainment of GES as measured by improvements in indicators of biological diversity and food webs, thus linking the MSFD pressure descriptor D3-commercial fish and shellfish to the state descriptors D1-biological diversity and D4-food webs. Given the need for managers to consider environmental targets for indicators alongside traditional fisheries mortality targets for stocks, scientific advice is required on the combined effects of fishing and climate impacts on the food web (Brown et al., 2010). Modeling is one of the only tools able to provide this evidence base to facilitate management decisions on which measures to take.
Lack of consideration of uncertainty and the use of single model parameterizations can be seen as a common limitation to some of the above studies (Jones and Cheung, 2015). Thorpe et al. (2016) used an ensemble approach with 188 plausible parameterizations of a size-based multispecies model (Thorpe et al., 2015) with four fishing fleets to assess the effects of 10,000 alternate fishing scenarios of the ecosystem. They demonstrated that the risk of stock depletions could be related to the value of indicators of biological diversity and food webs (i.e., the Large Fish Indicator and Size Spectrum Slope, respectively) and this approach can be particularly useful for identifying assessment thresholds for indicators. Thorpe et al. (2016) also demonstrated a way to present risks (i.e., of stock depletion and thus loss of biological diversity) and potential rewards (value of the catch) associated with the scenarios tested. Similarly, a management strategy evaluation tool has been developed for the EwE software, capable of exploring the complete parameter space, and multiple fisheries management strategies, having been tested using 1000 model configurations (STECF, 2015). Ecosystems are difficult to model and project so that model uncertainty is also important to capture in addition to parameter uncertainty. The STECF workshop (STECF, 2015) approached this by using four differing models (EwE, Fcube, Simfish, and Fishrent) and contrasting the outcome of the fishing strategies. In the assessment of impacts of climate change on marine ecosystems, large scale intercomparisons of models and configurations are now standard practice particularly to inform global studies such as assessment reports from the Intergovernmental Panel on Climate Change (Coupled Model Intercomparison Project of the World Climate Research Programme).
Predicting the outcomes of the management actions with precision becomes progressively more challenging as the number of major forcing factors and pressures increase since they can occur in previously unseen combinations (Dickey-Collas et al., 2014). Uusitalo et al. (2016) approached this problem in the Baltic Sea case by using three distinct modeling approaches to evaluate how different combinations of fisheries management and nutrient abatement can be expected to affect the ecosystem status of the Baltic Sea, thus linking the MSFD pressure descriptors D5-eutrophication and D3-commerical fish and shellfish. The modeling approaches they chose, (1) a spatial model for cumulative impacts (additive approach), (2) a food web simulation model, and (3) a Bayesian model harnessing expert knowledge, have all been used for management strategy design or evaluation, and all have their strengths and weaknesses in predicting the effects of the management scenarios (Uusitalo et al., 2016). While all of these models were at least implicitly based on the abundant research on the effects of nutrient loading and fishing pressure on the Baltic Sea ecosystem (see e.g., Gårdmark et al., 2013; Tomczak et al., 2013; Korpinen and Bonsdorff, 2014; Blenckner et al., 2015b; Fleming-Lehtinen et al., 2015), these three models were all based on a different logical construct, had different mathematical formulations, and very different specifications in terms of how explicitly they accounted for spatial and temporal aspects and the different ecosystem types of the Baltic Sea. Therefore, the authors concluded that any agreement between the models could be interpreted as representing relatively well-known, or robust, management response, while disagreement between the models imply that the management response may be more uncertain. This highlights the usefulness of multiple, mutually different modeling frameworks in discerning the uncertainties in future predictions.
Alternatively, uncertainty in ecosystem response can be reduced by focusing indicator studies on high-level ecosystem properties known to be more predictable than future projections. Either models are built directly for the high-level properties, or models describing systems at a lower level are used but analyses focus on emergent properties, i.e., high-level responses. Both approaches have been applied to predict recovery of fish community size structure. Examples of the former, direct approach are size spectrum models. The Species Size Spectrum Model (Rossberg, 2012), for example, is sufficiently simple to be solved analytically and this pinpointed key mechanisms slowing recovery: competition for food among fish species of very different size and predator-prey reversal. This was confirmed using a much more detailed, species-resolved food webs model (Fung et al., 2013), which was then used to predict recovery processes a range of different indicators of fish-community size structure. Applying this method to the Celtic Sea, Shephard et al. (2013) predict that recovery of the Large Fish Indicator to proposed target levels would require drastic reductions in fishing pressures and may yet last 30–50 years.
GES is defined in the MSFD for “…seas which are clean, healthy and productive within their intrinsic conditions….” Intrinsic conditions are not clearly defined and it is not all clear, what sort of ecosystem would occur in the absence of human interference in a given physical environment. If we considered that the major physical regimes in the sea (i.e., short-term/seasonal/permanent stratified conditions/mixed conditions) promote a particular life form of primary producer over others and thus structure the food web then these regimes are the relevant level in which to assess change in the plankton. Within the DEVOTES project, we modeled physical processes with a coupled hydro-biogeochemical model (GETM-ERSEM-BFM) and determined Ecohydrodynamic zones that capture differing intrinsic conditions (van Leeuwen et al., 2015). The spatial stability of the Ecohydrodynamic zones suggests that carefully selected monitoring locations can be used to represent much larger areas. As such these zones are being used as the spatial basis for plankton (D1 and D4) and oxygen (D5) indicators in the OSPAR region (Greater North Sea and Celtic Seas).
HELCOM engages in modeling to define its nutrient reduction schemes (i.e., with reference to eutrophication D5) through use of the coupled physical-biogeochemical model BALSEM (BAltic sea Long-Term large Scale Eutrophication Model, Gustafsson, 2003; Savchuk et al., 2012) to calculate maximum allowable inputs (MAI, Table 1). MAI are the maximal level of annual inputs of water- and airborne nitrogen and phosphorus to Baltic Sea sub-basins that can be allowed while still achieving GES in terms of eutrophication, that is given GES boundaries for eutrophication indicators like nitrogen, phosphorous and chlorophyll-a concentrations, water transparency and oxygen debt. BALTSEM is a time-dependent ecosystem model available through the Nest Decision Support System (www.balticnest.org/nest). It has been used also as the major scientific tool for the development of the HELCOM Baltic Sea Action Plan (HELCOM, 2007). The HELCOM Contracting Parties annually report atmospheric emissions and waterborne inputs of nitrogen and phosphorous from rivers and direct point sources to the Baltic Sea sub-basins. Nutrient input data are compiled in accordance with specific HELCOM guidelines for nine Baltic Sea sub-basins, whose boundaries coincide with the main terrestrial river basin catchments. The BALTSEM model has instead divided the whole Baltic Sea into seven sub-basins in accordance with natural marine boundaries and the MAIs are calculated accordingly through an optimization technique: finding the highest possible inputs that will satisfy given eutrophication targets. A revised HELCOM nutrient reduction scheme was adopted in the 2013 HELCOM Ministerial Declaration (HELCOM, 2013) in which reduction requirements for nitrogen inputs to the Baltic Proper, Gulf of Finland, and Kattegat and for phosphorus inputs to the Baltic Proper, Gulf of Finland, and Gulf of Riga were set (Table 1A). The progress of countries in reaching their share of the country-wise allocation of nutrient reduction targets (CART, Table 1B) is then assessed separately.
Table 1. (A) Maximum Allowable Inputs (MAIs) to the Baltic Sea and (B) country allocated reduction targets (CARTs) as revised by HELCOM using the BALSEM model (HELCOM, 2013).
Assessments of GES within the MSFD are made as part of an adaptive management approach with 6 year cycle (Figure 1). In this review, we demonstrate the important role that modeling has throughout the MSFD assessment cycle from generating understanding, underpinning assessments, and investigating the impact of changes in prevailing climatic conditions, invasions of non-indigenous species and multiple human pressures, and in the exploration of potential impacts through projections that consider management scenarios. Only through modeling can such scenarios be tested in order to help select appropriate management measures to maintain or recover ecosystems. We have reported on case studies, many of them resulting from DEVOTES project work, to illustrate how models should be used in the MSFD implementation cycle, and suggest that in many areas this is already happening but it is not always recognized nor is it considered a matter of routine.
Prior to each new assessment cycle, indicators should be re-evaluated given new data and refined where necessary. During the 6 year period since the previous selection of indicators, new indicators may have been generated and proposed to support indicator assessment, particularly if new pressures on the system have emerged. Thus, at the beginning of each cycle, a review of the indicator set and a model based study to select the most appropriate indicators in relation to pressures (Houle et al., 2012) would be prudent along with the evaluation of their scientific standard and applicability (Queirós et al., 2016a). Once an assessment of state for GES has been made, the next question likely to be faced by mangers is: are we moving in the right direction to maintain or recover GES? Here again, modeling studies are key since trends in state and indicators can be projected incorporating the prevailing climatic conditions and a range of anthropogenic pressures (Lynam and Mackinson, 2015; Piroddi et al., 2015b; Uusitalo et al., 2016). Such studies can inform the policy makers on the likelihood or reaching previously agreed reference points given the prevailing climate. In some instances, prevailing conditions (such as trends in temperature or changes in storminess) may alter the trajectory of change in the system such that managers may wish to alter targets to account for this (e.g., Lynam and Mackinson, 2015; van Leeuwen et al., 2016). Once these decisions have been made, monitoring programs must be steered to ensure that data are collected to support those areas of the assessment that are most uncertain, and/or showing the strongest degradation (Shephard et al., 2015b). Adaptive monitoring in this way should be most cost-effective and lead to information being generated where it is most needed.
If the ecosystem in question has not met GES, or if the trends assessment suggests the system is likely to be degrading given the range of current pressures, policy makers will wish to implement management measures to maintain biological diversity and food web functioning. However, given the range of pressures in any system and the differing options of measures that managers may consider implementing, further evidence is required to enable an informed choice to be made. Here, once again, modeling can assist through environmental impact assessments and management strategy evaluation. Ecosystem and higher trophic level modeling, through which projections are made for indicators given a range of scenarios that include a suite of management strategies coupled to climate change trajectories, can be used to estimate risk and reward of each potential option. From this set, managers can then choose the most socially acceptable solution.
DEVOTES assessed the capabilities of state-of-art models to provide information about current and candidate indicators outlined in the MSFD, particularly on biological diversity, food webs, non-indigenous species and seafloor integrity (Piroddi et al., 2015a; Tedesco et al., 2016). We demonstrated that models are largely able to inform on food webs, but that non-indigenous species habitats and seafloor integrity are often poorly addressed. Notably, however, mechanistic models such as ERSEM have been explicitly designed to represent benthic processes associated with seafloor functioning (Butenschön et al., 2015; Queirós et al., 2015; Lessin et al., 2016). In this project, we used modeling tools to refine some of the existing indicators and to develop novel indicators. To address the gaps related with indicators on non-indigenous species, we developed the CIMPAL index (Katsanevakis et al., 2016). To help refine existing seafloor integrity indicators, we employed benthic trait analysis (van der Linden et al., 2016) to specifically understand benthic community function in relation to habitats of the Bay of Biscay and in the North Sea, and identified typological groups of benthic macroinvertebrates, based on response and effect traits as potential indicators for MSFD D6 and D1 (Lynam et al., 2015).
Understanding the maintenance of the relationship between biological diversity and environmental disturbance is simultaneously challenging and key to supporting an ecosystem-based management approach. Traits-based approaches emphasize the functional characteristics of species to study this relationship, and availability of such information for marine species has rapidly increased in recent years, particularly in Europe (Costello et al., 2015). Mechanistic modeling approaches often utilize functional classifications to represent marine organisms (Queirós et al., 2015) providing a route to investigate how ecosystem processes may change under future environmental conditions, despite the complexity inherent to the process (Bremner, 2008; Queirós et al., 2015; van der Linden et al., 2016). Several studies have suggested model developments are needed to support a more comprehensive use of traits-based approaches (e.g., Savage et al., 2007; Webb et al., 2010; Verberk et al., 2013).
Understanding the connectivity attributes of each species is central to establishing effective management and conservation strategies such as the creation of networks of Marine Protected Areas (MPAs). For instance, Webb et al. (2010) suggests that a quantitative framework combining Bayesian multilevel models, dynamical systems models and hybrid approaches has the potential to meaningfully advance traits-based ecology. Reiss et al. (2014) stresses the importance of considering multiple biological traits and benthic ecosystems functions in Distribution Modeling techniques and their high potential to assist in a marine management context (e.g., MPA designations). The fragmentation of habitats is a threat to the maintenance of biological diversity, thus dispersal traits of species and the connectivity within and between population and communities are important attributes of species distributional patterns (Chust et al., 2016). As multiple species traits are likely to influence trophic interactions and functioning, approaches which seek to integrate trait-based methods with the food webs framework are also emerging with many recent advances stemming from modeling work (e.g., Thompson et al., 2012; Eklöf et al., 2013; Poisot et al., 2013; Nordström et al., 2015). These have shown to be successful at predicting network structure (Eklöf et al., 2013), in determining the strength of individual trophic links (Klecka and Boukal, 2013), highlighting that multiple traits are needed for more complete descriptions of interactions (Eklöf et al., 2013) and that functional and trophic attributes should be assessed in an integrated manner to provide accurate assessments in a changing environment (Boukal, 2014).
There are significant challenges still surrounding the uptake and use of complex models by decision makers. Many of these relate to understanding of models, outputs in the right currency, treatment of uncertainty, rigorous quality standards, and availability of user-friendly model products (Hyder et al., 2015). There are few direct examples of how outputs have led to decisions either related to policy or management, but this is not surprising since decision making is normative and incorporates societal values alongside the evidence base (Fletcher, 2007). Models can contribute to the evidence base that underpins decision making, but this is at an early stage with many other factors accounted for after compilation of the scientific knowledge (see e.g., van den Hove, 2007). However, it is clear that models have a vital role to play in decision making, as many policy or management options cannot be tested experimentally or in real ecosystems. The key to improving the uptake of models by decision makers is to build understanding both of the methods and issues through multidisciplinary communities that co-develop models (Hyder et al., 2015). Decision making timescales are often at odds with model development, so it is important to be able to adapt existing models to address these needs at short notice, and to provide outputs in the right currencies (monetary, stocks, natural capital, ecosystem services) understandable by policy makers (Hyder et al., 2015; Queirós et al., 2016b). For instance, many of the examples above focus on the biological aspects of decision making, whereas many decisions are based on economic or social outcomes (Watson, 2005; Papathanasopoulou et al., 2013). However, including the human dimension is very important as it may represent the largest source of uncertainty (Fulton et al., 2011). Integration of human dimensions like governance into models is vital to increase understanding of the likely outcome of management decisions, and has driven the development of social-ecological models (e.g., Griffith et al., 2012). There is a constant drive to resolve technical challenges around complexity, uncertainty and model skill (Allen and Somerfield, 2009; Payne et al., 2016) but models can provide useful insight despite being wrong (Box, 1979). Hence, modelers need to understand the needs of decision makers and work closely with them to build trust in their models, but ensure that uncertainty and key assumptions are highlighted through quality statements (ICES, 2016b; Queirós et al., 2016b). Without this trust, models will not be included in the decision making process or the implementation cycle.
Our overview shows that modeling can support the review of objectives, targets, and indicators for the MSFD. Modeling is the only option to evaluate different management strategies and thus help select appropriate management measures. We recommend that indicator assessments are supported by modeling studies, so that linkages between descriptors and global pressures on the marine environment (such as climate change and ocean acidification) and cumulative impacts are more fully grasped. Models can be used to highlight recovery trajectories of indicators and a range of management strategies should be explored through scenarios to provide support to decision makers. Specifically, the likely synergistic and antagonistic effects of management measures and concurrent changes in prevailing climatic conditions should be investigated at each assessment cycle of the MSFD. Sources of uncertainty (measurement error, uncertainty in pressure-state relationships, model uncertainty) must be considered and communicated during indicator assessments and model studies (Cartwright et al., 2016). Utilizing modeling support as a routine in the assessment cycle would ultimately improve long term planning for the marine environment.
There is still a wide gap between modelers and decision makers, and the full utility of models has not yet been realized. To enable models to better support marine environmental management and the MSFD, it is important to ensure that communities of policy makers and scientists are set up to co-develop ecosystem models. At a national level, interdisciplinary groups are required to support assessments and policy making, including internationally, groups of modelers compare approaches and harmonize methods across regional seas to support MSFD assessments. Internationally, independent technical reviews of national groups' progress should be made to ensure high quality advice and promote harmonization between regional seas.
CL conceived of the paper and all authors contributed to the paper writing.
The authors declare that the research was conducted in the absence of any commercial or financial relationships that could be construed as a potential conflict of interest.
This manuscript is a result of DEVOTES (DEVelopment Of innovative Tools for understanding marine biological diversity and assessing good Environmental Status) project, funded by the European Union under the 7th Framework Programme, “The Ocean of Tomorrow” Theme (grant agreement no. 308392), www.devotes-project.eu.
Aldridge, J., van der Molen, J., and Forster, R. M. (2012). Wider Ecological Implications of Macroalgae Cultivation. Marine Estate Research Report, The Crown Estate.
Alheit, J., Möllmann, C., Dutz, J., Kornilovs, G., Loewe, P., Mohrholz, V., et al. (2005). Synchronous regime shifts in the Central Baltic and the North Sea in the late 1980s. ICES J. Mar. Sci. 62, 1205–1215. doi: 10.1016/j.icesjms.2005.04.024
Allen, J. I., and Clarke, K. R. (2007). Effects of demersal trawling on ecosystem functioning in the North Sea: a modeling study. Mar. Ecol. Prog. Ser. 336, 63–75. doi: 10.3354/meps336063
Allen, J., and Somerfield, P. (2009). A multivariate approach to model skill assessment. J. Mar. Syst. 76, 83–94. doi: 10.1016/j.jmarsys.2008.05.009
Artioli, Y., Blackford, J., Nondal, G., Bellerby, R. G. J., Wakelin, S. L., Holt, J. T., et al. (2014). Heterogeneity of impacts of high CO2 on the North Western European Shelf. Biogeosci. Discuss. 11, 601–612. doi: 10.5194/bg-11-601-2014
Baker, E. K., and Harris, P. T. (2012). “Habitat mapping and marine management,” in Seafloor Geomorphology as Benthic Habitat, eds P. T. Harris and E. K. Baker (London: Elsevier), 23–38.
Barnsley, M. J. (2007). Environmental Modeling: A Practical Introduction. Boca Raton, FL: CRC Press.
Beisner, B. E., Haydon, D. T., and Cuddington, K. (2003). Alternative stable states in ecology. Front. Ecol. Environ. 1, 376–382. doi: 10.1890/1540-9295(2003)001[0376:ASSIE]2.0.CO;2
Berg, T., Fürhaupter, K., Teixeira, H., Uusitalo, L., and Zampoukas, N. (2015). The marine strategy framework directive and the ecosystem approach-pitfalls and solutions. Mar. Pollut. Bull. 96, 18–28. doi: 10.1016/j.marpolbul.2015.04.050
Blackburn, T. M., Essl, F., Evans, T., Hulme, P. E., Jeschke, J. M., Kühn, I., et al. (2014). A unified classification of alien species based on the magnitude of their environmental impacts. PLoS Biol. 12:e1001850. doi: 10.1371/journal.pbio.1001850
Blenckner, T., Llope, M., Möllmann, C., Voss, R., Quaas, M. F., Casini, M., et al. (2015b). Climate and fishing steer ecosystem regeneration to uncertain economic futures. Proc. Biol. Sci. 282:20142809. doi: 10.1098/rspb.2014.2809
Blenckner, T., Österblom, H., Larsson, P., Andersson, A., and Elmgren, R. (2015a). Baltic Sea ecosystem-based management under climate change: synthesis and future challenges. Ambio 44, 507–515. doi: 10.1007/s13280-015-0661-9
Borgström, S., Bodin, Ö., Sandström, A., and Crona, B. (2015). Developing an analytical framework for assessing progress toward ecosystem-based management. Ambio 44, 357–369. doi: 10.1007/s13280-015-0655-7
Boukal, D. S. (2014). Trait- and size-based descriptions of trophic links in freshwater food webs: current status and perspectives. J. Limnol. 73, 171–185. doi: 10.4081/jlimnol.2014.826
Box, G. E. P. (1979). “Robustness in the strategy of scientific model building,” in Robustness in Statistics, eds R. L. Launer and G. N. Wilkinson (Academic Press), 201–236.
Bremner, J. (2008). Species' traits and ecological functioning in marine conservation and management. J. Exp. Mar. Biol. Ecol. 366, 37–47. doi: 10.1016/j.jembe.2008.07.007
Brown, C., Fulton, E., Hobday, A., Matear, R., Possingham, H., Bulman, C., et al. (2010). Effects of climate−driven primary production change on marine food webs: implications for fisheries and conservation. Glob. Change Biol. 16, 1194–1212. doi: 10.1111/j.1365-2486.2009.02046.x
Butenschön, M., Clark, J., Aldridge, J., Allen, J., Artioli, Y., Blackford, J., et al. (2015). ERSEM 15.06: a generic model for marine biogeochemistry and the ecosystem dynamics of the lower trophic levels. Geosci. Model Dev. Discuss. 8, 7063–7187. doi: 10.5194/gmdd-8-7063-2015
Capuzzo, E., Stephens, D., Silva, T., Barry, J., and Forster, R. M. (2015). Decrease in water clarity of the southern and central North Sea during the 20th century. Glob. Change Biol. 21, 2206–2214. doi: 10.1111/gcb.12854
Cardinale, M., and Svedäng, H. (2011). The beauty of simplicity in science: Baltic cod stock improves rapidly in a ‘cod hostile’ ecosystem state. Mar. Ecol. Prog. Ser. 425, 297–301. doi: 10.3354/meps09098
Carr, M. E., Friedrichs, M. A. M., Schmeltza, M., Aita, M. N., Antoined, D., Arrigoe, K. R., et al. (2006). A comparison of global estimates of marine primary production from ocean color. Deep Sea Res. II 53, 741–770. doi: 10.1016/j.dsr2.2006.01.028
Carstensen, J., and Lindegarth, M. (2016). Confidence in ecological indicators: a framework for quantifying uncertainty components from monitoring data. Ecol. Indic. 67, 306–317. doi: 10.1016/j.ecolind.2016.03.002
Cartwright, S. J., Bowgen, K. M., Collop, C., Hyder, K., Nabe-Nielsen, J., Stafford, R., et al. (2016). Communicating complex ecological models to non-scientist end users. Ecol. Modell. 338, 51–59. doi: 10.1016/j.ecolmodel.2016.07.012
CBD (2014). Ecosystem Approach Sourcebook. Available online at: https://www.cbd.int/ecosystem/sourcebook/default.shtml
Ceccon, S., Garway-Heath, D., Crabb, D., and Tucker, A. (2011). The dynamic stage bayesian network: identifying and modelling key stages in a temporal process. Adv. Intell. Data Anal. X, 101–112. doi: 10.1007/978-3-642-24800-9_12
Chaalali, A., Beaugrand, G., Raybaud, V., Lassalle, G., Saint-Béat, B., Le Loc'h, F., et al. (2016). From species distributions to ecosystem structure and function: a methodological perspective. Ecol. Modell. 334, 78–90. doi: 10.1016/j.ecolmodel.2016.04.022
Chaalali, A., Saint-Béat, B., Lassalle, G., Le Loc', H. F., Tecchio, S., Safi, G., et al. (2015). A new modeling approach to define marine ecosystems food-web status with uncertainty assessment. Prog. Oceanogr.135, 37–47. doi: 10.1016/j.pocean.2015.03.012
Cheung, W. W., Jones, M. C., Reygondeau, G., Stock, C. A., Lam, V. W., and Frölicher, T. L. (2016). Structural uncertainty in projecting global fisheries catches under climate change. Ecol. Modell. 325, 57–66. doi: 10.1016/j.ecolmodel.2015.12.018
Christensen, V., and Walters, C. J. (2004). Ecopath with ecosim: methods, capabilities and limitations. Ecol. Modell. 172, 109–139. doi: 10.1016/j.ecolmodel.2003.09.003
Chust, G., Villarino, E., Chenuil, A., Irigoien, X., Bizsel, N., Bode, A., et al. (2016). Dispersal similarly shapes population genetics and community patterns in the marine realm. Sci. Rep. 6:28730. doi: 10.1038/srep28730
Costello, M. J., Claus, S., Dekeyzer, S., Vandepitte, L., Tuama, É. Ó., Lear, D., et al. (2015). Biological and ecological traits of marine species. PeerJ 3:e1201. doi: 10.7717/peerj.1201
De Jonge, V. N., Elliott, M., and Brauer, V. S. (2006). Marine monitoring: its shortcomings and mismatch with the EU Water Framework Directive's objectives. Mar. Pollut. Bull. 53, 5–19. doi: 10.1016/j.marpolbul.2005.11.026
Diaz, R. J., and Rosenberg, R. (2008). Spreading dead zones and consequences for marine ecosystems. Science. 321, 926–929. doi: 10.1126/science.1156401
Dickey-Collas, M., Payne, M. R., Trenkel, V. M., and Nash, R. D. M. (2014). Hazard warning: model misuse ahead. ICES J. Mar. Sci. 71, 2300–2306. doi: 10.1093/icesjms/fst215
Diekmann, R., and Möllmann, C. (Eds). (2010). Integrated Ecosystem Assessments of Seven Baltic Sea Areas Covering the Last Three Decades. ICES Cooperative Research Report No. 302, 90. Available online at: http://www.balticnest.org/download/18.373a4c5113540591b7414ca/1381789015726/Integrated+ecos+ass+of+BS_2010_WGIAB.pdf
Doak, D. F., Estes, J. A., Halpern, B. S., Jacob, U., Lindberg, D. R., Lovvorn, J., et al. (2008). Understanding and predicting ecological dynamics: are major surprises inevitable? Ecology 89, 952–961. doi: 10.1890/07-0965.1
Doney, S. C., Fabry, V. J., Feely, R. A., and Kleypas, J. A. (2009). Ocean acidification: the other CO2 problem. Annu. Rev. Mar. Sci. 1, 169–192. doi: 10.1146/annurev.marine.010908.163834
Dupont, N., and Aksnes, D. L. (2013). Centennial changes in water clarity of the Baltic Sea and the North Sea. Estuar. Coast. Shelf. Sci. 131, 282–289. doi: 10.1016/j.ecss.2013.08.010
Eero, M., Koster, F. W., and Vinther, M. (2012). Why is the Eastern Baltic cod recovering? Mar. Policy 36, 235–240. doi: 10.1016/j.marpol.2011.05.010
Eklöf, A., Jacob, U., Kopp, J., Bosch, J., Castro-Urgal, R., Chacoff, N. P., et al. (2013). The dimensionality of ecological networks. Ecol. Lett. 16, 577–583. doi: 10.1111/ele.12081
Essl, F., Bacher, S., Blackburn, T. M., Booy, O., Brundu, G., Brunel, S., et al. (2015). Crossing frontiers in tackling pathways of biological invasions. BioScience 65, 769–782. doi: 10.1093/biosci/biv082
European Commission (2008). EU Directive 2008/56/EC of the European Parliament and of the Council of 17 June 2008 establishing a framework for community action in the field of marine environmental policy. Off. J. Eur. Commun. L164, 19–40.
European Commission. (2013). Commission Decision of 20 September 2013 establishing, pursuant to Directive 2000/60/EC of the European Parliament and of the Council, the values of the Member State monitoring system classifications as a result of the intercalibration exercise and repealing Decision 2008/915/EC. Off. J. Eur. Union L266, 1–47.
Ferreira, J. G., Andersen, J. H., Borja, A., Bricker, S. B., Camp, J., Cardoso da Silva, M., et al. (2010). Marine Strategy Framework Directive Task Group 5 Report Eutrophication. European Union and ICES, 58.
Fitzpatrick, M. C., Sanders, N. J., Ferrier, S., Longino, J. T., Weiser, M. D., and Dunn, R. (2011). Forecasting the future of biodiversity: a test of single- and multi-species models for ants in North America. Ecography 34, 836–847. doi: 10.1111/j.1600-0587.2011.06653.x
Fleming-Lehtinen, V., Andersen, J. H., Carstensen, J., Łysiak-Pastuszak, E., Murray, C., Pyhälä, M., et al. (2015). Recent developments in assessment methodology reveal that the Baltic Sea eutrophication problem is expanding. Ecol. Indic. 48, 380–388. doi: 10.1016/j.ecolind.2014.08.022
Fletcher, S. (2007). Converting science to policy through stakeholder involvement: an analysis of the European Marine Strategy Directive. Mar. Pollut. Bull. 54, 1881–1886. doi: 10.1016/j.marpolbul.2007.08.004
Fogarty, M. J., Rosenberg, A. A., Cooper, A. B., Dickey-Collas, M., Fulton, E. A., Gutiérrez, N. L., et al. (2016). Fishery production potential of large marine ecosystems: a prototype analysis. Environ. Dev. 17, 211–219 doi: 10.1016/j.envdev.2016.02.001
Fulton, E. A., Smith, A. D. M., Smith, D. C., and Van Putten, I. E. (2011). Human behaviour: the key source of uncertainty in fisheries management. Fish Fish. 12, 2–17. doi: 10.1111/j.1467-2979.2010.00371.x
Fung, T., Farnsworth, K. D., Shephard, S., Reid, D. G., and Rossberg, A. G. (2013). Why the size structure of marine communities can require decades to recover from fishing. Mar. Ecol. Prog. Ser. 484, 155–171. doi: 10.3354/meps10305
Gårdmark, A., Lindegren, M., Neuenfeldt, S., Blenckner, T., Heikinheimo, O., Müller-Karulis, B., et al. (2013). Biological ensemble modeling to evaluate potential futures of living marine resources. Ecol. Appl. 23, 742–754. doi: 10.1890/12-0267.1
Gilbert, P. M., Allen, J. I., Bouwman, A. F., Brown, C. W., Flynn, K. J., Lewitus, A. J., et al. (2010). Modeling of HABs and eutrophication: status, advances, challenges. J. Mar. Syst. 83, 262–275. doi: 10.1016/j.jmarsys.2010.05.004
Gohin, F., Druon, J. N., and Lampert, L. (2002). A five channel chlorophyll algorithm applied to SeaWiFS data processed by SeaDAS in coastal waters. Int. J. Remote Sens. 23, 1639–1661. doi: 10.1080/01431160110071879
Gonzalvo, J., Forcada, J., Grau, E., and Aguilar, A. (2014). Strong site-fidelity increases vulnerability of common bottlenose dolphins Tursiops truncatus in a mass tourism destination in the western Mediterranean Sea. J. Mar. Biol. Assoc. U. K 94, 1227–1235. doi: 10.1017/s0025315413000866
Griffith, G. P., Fulton, E. A., Gorton, R., and Richardson, A. J. (2012). Predicting interactions among fishing, ocean warming, and ocean acidification in a marine system with whole-ecosystem models. Cons. Biol. 26, 1145–1152. doi: 10.1111/j.1523-1739.2012.01937.x
Guesnet, V., Lassalle, G., Chaalali, A., Kearney, K., Saint-Béat, B., Karimi, B., et al. (2015). Incorporating food-web parameter uncertainty into Ecopath-derived ecological network indicators. Ecol. Modell. 313, 29–40. doi: 10.1016/j.ecolmodel.2015.05.036
Gustafsson, B. G. (2003). A Time-Dependent Coupled-Basin Model of the Baltic Sea. Report C47. Earth Sciences Centre, Gotenburg University.
HELCOM (2007). Baltic Sea Action Plan. HELCOM Ministerial Meeting. Adopted in Krakow, Poland on 15th of November 2007, Technical Report.
HELCOM (2013). HELCOM COPENHAGEN Ministerial Declaration: Taking Further Action to Implement the Baltic Sea Action Plan Reaching Good Environmental Status for a Healthy Baltic Sea. Technical Report.
Hobday, A. J., and Pecl, G. T. (2014). Identification of global marine hotspots: sentinels for change and vanguards for adaptation action. Rev. Fish Biol. Fisher. 24, 415–425. doi: 10.1007/s11160-013-9326-6
Hooker, S., and McClain, C. (2000). The calibration and validation of SeaWiFS data. Prog. Oceanogr. 45, 427–465. doi: 10.1016/S0079-6611(00)00012-4
Houle, J. E., Farnsworth, K. D., Rossberg, A. G., and Reid, D. G. (2012). Assessing the sensitivity and specificity of fish community indicators to management action. Can. J. Fish. Aquat. Sci. 69, 1065–1079. doi: 10.1139/f2012-044
Hu, C., Lee, Z., and Franz, B. A. (2012). Chlorophyll-a algorithms for oligotrophic oceans: A novel approach based on three-band reflectance difference. J. Geophys. Res. 117, C01011. doi: 10.1029/2011JC007395
Hulme, P. E., Bacher, S., Kenis, M., Klotz, S., Kühn, I., Minchin, D., et al. (2008). Grasping at the routes of biological invasions: a framework for integrating pathways into policy. J. Appl. Ecol. 45, 403–414. doi: 10.1111/j.1365-2664.2007.01442.x
Hyder, K., Rossberg, A. G., Allen, J. I., Austen, M. C., Barciela, R. M., Bannister, H. J., et al. (2015). Making modeling count - increasing the contribution of shelf-seas community and ecosystem models to policy development and management. Mar. Policy 62, 291–302. doi: 10.1016/j.marpol.2015.07.015
ICES (2012). Report of the Working Group on the Ecosystem Effects of Fishing Activities (WGECO), 11–18 April 2012. Copenhagen, ICES CM 2012/ACOM:26.
ICES (2015). Interim Report of the Working Group to Demonstrate a Celtic Seas wide approach to the application of fisheries related science to the implementation of the Marine Strategy Framework Directive (WGMSFDEMO). ICES CM 2015/SSGIEA:12.
ICES (2016a). Report of the Workshop to Plan an Integrated Monitoring Programme in the North Sea in Q3 (WKPIMP), 22-26 February 2016. ICES HQ, Copenhagen. ICES CM 2016/SSGIEOM:11.
ICES (2016b). Report of the Working Group on Multispecies Assessment Methods (WGSAM), 9–13 November 2015. Woods Hole, MA. ICES CM 2015/SSGEPI:20.
Jacox, M. G., Edwards, C. A., Kahru, M., Rudnick, D. L., and Kudela, R. M. (2013). The potential for improving remote primary productivity estimates through subsurface chlorophyll and irradiance measurement. Deep-Sea Res. Part II. 112, 107–116. doi: 10.1016/j.dsr2.2013.12.008
Jennings, S., and Collingridge, K. (2015). Predicting consumer biomass, size-structure, production, catch potential, responses to fishing and associated uncertainties in the World's marine ecosystems. PLoS ONE 10:e0133794. doi: 10.1371/journal.pone.0133794
Jennings, S., and Dulvy, N. K. (2005). Reference points and reference directions for size based indicators of community structure. ICES J. Mar. Sci. 62, 397–404. doi: 10.1016/j.icesjms.2004.07.030
Jennings, S., Mélin, F., Blanchard, J. L., Forster, R. M., Dulvy, N. K., and Wilson, R. W. (2008). Global-scale predictions of community and ecosystem properties from simple ecological theory. Proc. R. Soc. B Biol. Sci. 275, 1375–1383. doi: 10.1098/rspb.2008.0192
Jones, M. C., and Cheung, W. W. (2015). Multi-model ensemble projections of climate change effects on global marine biodiversity. ICES J. Mar. Sci. 72, 741–752. doi: 10.1093/icesjms/fsu172
Kaschner, K., Rius-Barile, J., Kesner-Reyes, K., Garilao, C., Kullander, S. O., Rees, T., et al. (2013). AquaMaps: Predicted Range Maps for Aquatic Species. World-Wide Web Electronic Publication. Available online at: www.aquamaps.org, Version 08/2013
Katsanevakis, S., Tempera, F., and Teixeira, H. (2016). Mapping the impact of alien species on marine ecosystems: the Mediterranean Sea case study. Divers. Distrib. 22, 694–707, doi: 10.1111/ddi.12429
Katsanevakis, S., Wallentinus, I., Zenetos, A., Leppäkoski, E., Çinar, M. E., Oztürk, B., et al. (2014). Impacts of marine invasive alien species on ecosystem services and biodiversity: a pan-European review. Aquat. Invasions 9, 391–423. doi: 10.3391/ai.2014.9.4.01
Kidd, S., Maltby, E., Robinson, L., and Barker, A. (2011). “The ecosystem approach and planning and management of the marine environment,” in The Ecosystem Approach to Marine Planning and Management, eds S. Kidd, A. Plater, and C. Frid (London: Earthscan), 1–33.
Klecka, J., and Boukal, D. S. (2013). Foraging and vulnerability traits modify predator-prey body mass allometry: freshwater macroinvertebrates as a case study. J. Anim. Ecol. 82, 1031–1041. doi: 10.1111/1365-2656.12078
Korpinen, S., and Bonsdorff, E. (2014). “Impacts of eutrophication on marine biodiversity,” in Marine Ecosystems: Human Impacts on Biodiversity, Functioning and Services, eds T. P. Crowe and C. L. J. Frid (Cambridge: Cambridge University Press), 202–243.
Koutsikopoulos, C., Ferentinos, G., Papatheodorou, G., Geraga, M., Christodoulou, D., Fakiris, H., et al. (2008). Fishing Activity in Amvrakikos Gulf: Current Situation and Perspectives. Final Report. Ministry of rural development and food of Greece, Direction of Fisheries, 157.
Kupschus, S., Schratzberger, M., and Righton, D. (2016). Practical implementation of ecosystem monitoring for the ecosystem approach to management. J. Appl. Ecol. 53, 1236–1247. doi: 10.1111/1365-2664.12648
Lassalle, G., Bourdaud, P., Saint-Béat, B., Rochette, S., and Niquil, N. (2014). A toolbox to evaluate data reliability for whole-ecosystem models: application on the Bay of Biscay continental shelf food-web model. Ecol. Modell. 285, 13–21. doi: 10.1016/j.ecolmodel.2014.04.002
Le Quesne, W. J. F., and Pinnegar, J. K. (2012). The potential impacts of ocean acidification: scaling from physiology to fisheries. Fish Fish. 13, 333–344. doi: 10.1111/j.1467-2979.2011.00423.x
Lessin, G., Artioli, Y., Queirós, A. M., Widdicombe, S., and Blackford, J. C. (2016). Modeling impacts and recovery in benthic communities exposed to localised high CO2. Mar. Poll. Bull. 109, 267–280. doi: 10.1016/j.marpolbul.2016.05.071
Lindegren, M., Blenckner, T., and Stenseth, N. C. (2012). Nutrient reduction and climate change cause a potential shift from pelagic to benthic pathways in a eutrophic marine ecosystem. Glob. Chang. Biol. 18, 3491–3503. doi: 10.1111/j.1365-2486.2012.02799.x
Lindegren, M., Diekmann, R., and Möllmann, C. (2010). Regime shifts, resilience and recovery of a cod stock. Mar. Ecol. Prog. Ser. 402, 239–253. doi: 10.3354/meps08454
Link, J. S., Ihde, T. F., Townsend, H. M., Osgood, K. E., Schirripa, M. J., Kobayashi, D. R., et al. (eds.). (2010). Report of the 2nd National Ecosystem Modeling Workshop (NEMoW II): Bridging the Credibility Gap – Dealing with Uncertainty in Ecosystem Models. US Department of Commerce, NOAA Technical Memorandum NMFS-F/SPO-102, Silver Spring, MD.
Llope, M., Daskalov, G. M., Rouyer, T. A., Mihneva, V., Chan, K.-S., Grishin, A. N., et al. (2011). Overfishing of top predators eroded the resilience of the Black Sea system regardless of the climate and anthropogenic conditions. Glob. Chang. Biol. 17, 1251–1265. doi: 10.1111/j.1365-2486.2010.02331.x
Long, R. D., Charles, A., and Stephensen, R. L. (2015). Key principles of marine ecosystem-based management. Mar. Policy 57, 53–60. doi: 10.1016/j.marpol.2015.01.013
Lotze, H. K., Coll, M., Magera, A. M., Ward-Paige, C., and Airoldi, L. (2011). Recovery of marine animal populations and ecosystems. Trends Ecol. Evol. 26, 595–605. doi: 10.1016/j.tree.2011.07.008
Lynam, C. P., and Mackinson, S. (2015). How will fisheries management measures contribute towards the attainment of Good Environmental Status for the North Sea ecosystem? Glob. Ecol. Conserv. 4, 160–175. doi: 10.1016/j.gecco.2015.06.005
Lynam, C. P., Tempera, F., Teixeira, H., Diesing, M., Stephens, S., van der Leeuwen, S., et al. (2015). Report Describing Spatial Ecosystem Models. Linking Habitats to Functional Biodiversity and Modeling Connectivity between Regional Seas. Deliverable 4.2 100pp + 9 Annexes. DEVOTES FP7 Project.
Mascaro, J., Asner, G. P., Knapp, D. E., Kennedy-Bowdoin, T., Martin, R. E., Anderson, C., et al. (2014). A tale of two “forests”: random forest machine learning aids tropical forest carbon mapping. PLoS ONE 9:e85993. doi: 10.1371/journal.pone.0085993
Micheli, F., Halpern, B. S., Walbridge, S., Ciriaco, S., Ferretti, F., Fraschetti, S., et al. (2013). Cumulative human impacts on Mediterranean and Black Sea marine ecosystems: assessing current pressures and opportunities. PLoS ONE 8:e79889. doi: 10.1371/journal.pone.0079889
Miller, J. A., and Holloway, P. (2015). Incorporating movement in species distribution models. Prog. Phys. Geogr. 39, 837–849. doi: 10.1177/0309133315580890
Möllmann, C., Blenckner, T., Casini, M., Gårdmark, A., and Lindegren, M. (2011). Beauty is in the eye of the beholder: management of Baltic cod stock requires an ecosystem approach. Mar. Ecol. Prog. Ser. 431, 293–297. doi: 10.3354/meps09205
Möllmann, C., Diekman, R., Müller-Karulis, B., Kornilovs, G., Plikshs, M., and Axe, P. (2009). Reorganization of a large marine ecosystem due to atmospheric and anthropogenic pressure: a discontinuous regime shift in the Central Baltic Sea. Glob. Chang. Biol. 15, 1377–1393. doi: 10.1111/j.1365-2486.2008.01814.x
Möllmann, C., Müller-Karulis, B., Kornilovs, G., and St John, M. A. (2008). Effects of climate and overfishing on zooplankton dynamics and ecosystem structure: regime shifts, trophic cascade, and feedback loops in a simple ecosystem. ICES J. Mar. Sci. 65, 302–310. doi: 10.1093/icesjms/fsm197
Naylor, R. L., Goldburg, R. J., Primavera, J. H., Kautsky, N., Beveridge, M. C. M., and Clay, J. (2000). Effect of aquaculture on world fish supplies. Nature 405, 1017–1024. doi: 10.1038/35016500
Nordström, M. C., Aarnio, K., Törnroos, A., and Bonsdorff, E. (2015). Nestedness of trophic links and biological traits in a marine food web. Ecosphere 6, 161. doi: 10.1890/es14-00515.1
Novoa, S., Chust, G., Sagarminaga, Y., Revilla, M., Franco, J., Valencia, V., et al. (2012). Chlorophyll-a variability within the Basque coastal waters and the Bay of Biscay, between 2005 and 2010, using MODIS imagery. Rev. Invest. Mar. 19, 92–107.
Palialexis, A., Tornero, V., Barbone, E., Gonzalez, D., Hanke, G., Cardoso, AC., et al. (2014). In-Depth Assessment of the EU Member States' Submissions for the Marine Framework Strategy Framework Directive under Articles 8, 9 and 10 JRC Scientific and Technical Reports. Publications Office of the European Union.
Papathanasopoulou, E., Beaumont, N., Lear, D., Fisher, E., Walker, P., Midlen, A., et al. (2013). Social Impacts of Fisheries, Aquaculture, Recreation, Tourism and Marine Protected Areas (MPAs) in Marine Plan Areas in England. MMO report 1035. Available online at: http://webarchive.nationalarchives.gov.uk/20140108121958/http:/www.marinemanagement.org.uk/evidence/documents/1035.pdf
Patrício, J., Little, S., Mazik, K., Papadopoulou, K.-N., Smith, C. J., Teixeira, H., et al. (2016). European biodiversity monitoring networks: strengths, weaknesses, opportunities and threats. Front. Mar. Sci. 3:161. doi: 10.3389/fmars.2016.00161
Pauly, D., Watson, R., and Alder, J. (2005). Global trends in world fisheries: impacts on marine ecosystems and food security. Philos. Trans. R. Soc. Lond. B Biol. Sci. 360, 5–12. doi: 10.1098/rstb.2004.1574
Payne, M. R., Barange, M., Cheung, W. W. L., MacKenzie, B. R., Batchelder, H. P., Cormon, X., et al. (2016). Uncertainties in projecting climate-change impacts in marine ecosystems. ICES J. Mar. Sci. 73, 1272–1282. doi: 10.1093/icesjms/fsv231
Peck, M. A., Christos, A., Momme, B., Melaku, C. D., Eva, C., Andrea, C., et al. (2016). Projecting changes in the distribution and productivity of living marine resources: a critical review of the suite of modeling approaches used in the large European project VECTORS. Estuar Coast. Shelf. S. doi: 10.1016/j.ecss.2016.05.019
Pinnegar, J. K., Tomczak, M. T., and Link, J. S. (2014). How to determine the likely indirect food-web consequences of a newly introduced non-native species: a worked example. Ecol. Modell. 272, 379–387. doi: 10.1016/j.ecolmodel.2013.09.027
Piroddi, C., Bearzi, G., and Christensen, V. (2010). Effects of local fisheries and ocean productivity on the northeastern Ionian Sea ecosystem. Ecol. Modell. 221, 1526–1544. doi: 10.1016/j.ecolmodel.2010.03.002
Piroddi, C., Bearzi, G., Gonzalvo, J., and Christensen, V. (2011). From common to rare: the case of the Mediterranean common dolphin. Biol. Conserv. 144, 2490–2498 doi: 10.1016/j.biocon.2011.07.003
Piroddi, C., Noutopoulos, D. K., Gonzalvo, J., and Libralato, S. (2015b). Ecosystem health of a Mediterranean semi-enclosed embayment (Amvrakikos Gulf, Greece): assessing changes using a modeling approach. Cont. Shelf Res. 121, 61–73, doi: 10.1016/j.csr.2015.10.007
Piroddi, C., Teixeira, H., Lynam, C. P., Smith, C., Alvarez, M. C., Mazik, K., et al. (2015a). Using ecological models to assess ecosystem status in support of the European Marine Strategy Framework Directive. Ecol. Indic. 58, 175–191. doi: 10.1016/j.ecolind.2015.05.037
Poisot, T., Mouquet, N., and Gravel, D. (2013). Trophic complementarity drives the biodiversity-ecosystem functioning relationship in food webs. Ecol. Lett. 16, 853–861. doi: 10.1111/ele.12118
Pollock, L. J., Tingley, R., Morris, W. K., Golding, N., O'Hara, R. B., Parris, K. M., et al. (2014). Understanding co-occurrence by modeling species simultaneously with a Joint Species Distribution Model (JSDM). Methods Ecol. Evol. 5, 397–406. doi: 10.1111/2041-210X.12180
Pörtner, H.-O., Karl, D. M., Boyd, P. W., Cheung, W. W. L., Lluch-Cota, S. E., Nojiri, Y., et al. (2014). “Ocean systems,” in Climate Change 2014: Impacts, Adaptation, and Vulnerability. Part A: Global and Sectoral Aspects. Contribution of Working Group II to the Fifth Assessment Report of the Intergovernmental Panel on Climate Change, eds C. B. Field, V. R. Barros, D. J. Dokken, K. J. Mach, M. D. Mastrandrea, T. E. Bilir, M. Chatterjee, K. L. Ebi, Y. O. Estrada, R. C. Genova, B. Girma, E. S. Kissel, A. N. Levy, S. MacCracken, P. R. Mastrandrea, and L. L. White (Cambridge; New York, NY: Cambridge University Press), 411–484.
Queirós, A. M., Bruggeman, J., Stephens, N., Artioli, Y., Butenschön, M., Blackford, J. C., et al. (2015). Placing biodiversity in ecosystem models without getting lost in translation. J. Sea Res. 98, 83–90. doi: 10.1016/j.seares.2014.10.004
Queirós, A. M., Huebert, K. B., Keyl, F., Fernandes, J. A., Stolte, W., Maar, M., et al. (2016b). Solutions for ecosystem-level protection of ocean systems under climate change. Glob. Chang. Biol. doi: 10.1111/gcb.13423. [Epub ahead of print].
Queirós, A. M., Strong, J. A., Mazik, K., Carstensen, J., Bruun, J., Somerfield, P., et al. (2016a). An objective framework to test the quality of candidate indicators of good environmental status. Front. Mar. Sci. 3:73. doi: 10.3389/fmars.2016.00073
Reiss, H., Birchenough, S., Borja, A., Buhl-Mortensen, L., Craeymeersch, J., Dannheim, J., et al. (2014). Benthos distribution modeling and its relevance for marine ecosystem management. ICES J. Mar. Sci. 72, 297–315. doi: 10.1093/icesjms/fsu107
Revilla, M., Franco, J., Bald, J., Borja, Á., Laza, A., Seoane, S., et al. (2009). The assessment of the phytoplankton ecological status in the Basque coast (northern Spain) according to the European Water Framework Directive. J. Sea Res. 61, 60–67. doi: 10.1016/j.seares.2008.05.009
Robinson, J., and Hartemink, A. (2009). “Non-stationary dynamic Bayesian networks,” in Advances in Neural Information Processing Systems 21 (NIPS08), eds D. Koller, D. Schuurmans, Y. Bengio, and L. Bottou (Cambridge, MA: MIT Press), 1369–1376.
Rodríguez, R. A., Herrera, A. M., Otto, R., Delgado, J. D., Fernández-Palacio, J. M., and Arévalo, J. R. (2012). Ecological state equation. Ecol. Modell. 224, 18–24. doi: 10.1016/j.ecolmodel.2011.10.020
Rossberg, A. G. (2012). A complete analytic theory for structure and dynamics of populations and communities spanning wide ranges in body size. Adv. Ecol. Res. 46, 429–522. doi: 10.1016/b978-0-12-396992-7.00008-3
Rossberg, A. G., Uusitalo, L., Berg, T., Zaiko, A., Chenuil, A., Uyarra, M. C., et al. (2017). Quantitative criteria for choosing targets and indicators for sustainable use of ecosystems. Ecol. Indic. 72, 215–224. doi: 10.1016/j.ecolind.2016.08.005
Ryther, J. H. (1969). Photosynthesis and Fish Production in the Sea. Science 166, 72–76. doi: 10.1126/science.166.3901.72
Savage, V. M., Webb, C. T., and Norberg, J. (2007). A general multi-trait-based framework for studying the effects of biodiversity on ecosystem functioning. J. Theor. Biol. 247, 213–229. doi: 10.1016/j.jtbi.2007.03.007
Savchuk, O. P., Gustafsson, B. G., and Muller-Karulis, B. (2012). BALTSEM A Marine Model for Decision Support within the Baltic Sea Region, Tech. Rep. 7. Baltic Nest Institute, Sweden.
Scheffer, M., Bascompte, J., Brock, W. A., Brovkin, V., Carpenter, S. R., Dakos, V., et al. (2009). Early-warning signals for critical transitions. Nature 461, 53–59. doi: 10.1038/nature08227
Shephard, S., Fung, T., Rossberg, A. G., Farnsworth, K. D., Reid, D. G., Greenstreet, S. P. R., et al. (2013). Modeling recovery of Celtic Sea demersal fish community size-structure. Fish. Res. 140, 91–95. doi: 10.1016/j.fishres.2012.12.010
Shephard, S., Greenstreet, S. P. R., Piet, G. J., Rindorf, A., and Dickey-Collas, M. (2015a). Surveillance indicators and their use in implementation of the marine strategy framework directive. ICES J. Mar. Sci. 72, 2269–2277. doi: 10.1093/icesjms/fsv131
Shephard, S., van Hal, R., de Boois, I., Birchenough, S. N. R., Foden, J., and O'Connor, J. (2015b). Making progress towards integration of existing sampling activities to establish Joint Monitoring Programmes in support of the MSFD. Mar. Policy 59, 105–111. doi: 10.1016/j.marpol.2015.06.004
Shin, Y., Bundy, A., Shannon, L. J., Blanchard, J. L., Chuenpagdee, R., Coll, M., et al. (2012). Global in scope and regionally rich: an IndiSeas workshop helps shape the future of marine ecosystem indicators. Rev. Fish Biol. Fish. 22, 835–845. doi: 10.1007/s11160-012-9252-z
Smaal, A. C., Schellekens, T., van Stralen, M. R., and Kromkamp, J. C. (2013). Decrease of the carrying capacity of the Oosterschelde estuary (SW Delta, NL) for bivalve filter feeders due to overgrazing? Aquaculture 404, 28–34. doi: 10.1016/j.aquaculture.2013.04.008
Smyth, T. J., Tilstone, G. H., and Groom, S. B. (2005). Integration of radiative transfer into satellite models of ocean primary production. J. Geophys. Res. C Oceans 110, 1–11. doi: 10.1029/2004JC002784
STECF (2015). (Scientific, Technical and Economic Committee for Fisheries) Evaluation of Management Plans: Evaluation of the Multi-Annual Plan for the North Sea Demersal Stocks (STECF-15-04). Luxembourg: Publications Office of the European Union, 152.
Stephens, D., and Diesing, M. (2015). Towards quantitative spatial models of seabed sediment composition. PLoS ONE 10:e0142502. doi: 10.1371/journal.pone.0142502
Sumaila, U. R., Lam, V. W. Y., Miller, D. D., Teh, L., Watson, R. A., Zeller, D., et al. (2015). Winners and losers in a world where the high seas is closed to fishing. Sci. Rep. 5:8481. doi: 10.1038/srep08481
Szuwalski, C. S., and Hollowed, A. B. (2016). Climate change and non-stationary population processes in fisheries management. ICES J. Mar. Sci. 73, 1297–1305. doi: 10.1093/icesjms/fsv229
Tecchio, S., Chaalali, A., Raoux, A., Rius, A. T., Lequesne, J., Girardin, V., et al. (2016). Evaluating ecosystem-level anthropogenic impacts in a stressed transitional environment: the case of the Seine estuary. Ecol. Indic. 61, 833–845. doi: 10.1016/j.ecolind.2015.10.036
Tedesco, L., Piroddi, C., Kämäri, M., and Lynam, C. (2016). Capabilities of Baltic Sea models to assess environmental status for marine biodiversity. Mar. Policy 70, 1–12. doi: 10.1016/j.marpol.2016.04.021
Thompson, R. M., Brose, U., Dunne, J. A., Hall, R. O. Jr., Hladyz, S., Kitching, R. L., et al. (2012). Food webs: reconciling the structure and function of biodiversity. Trends Ecol. Evol. 27, 689–697 doi: 10.1016/j.tree.2012.08.005
Thorpe, R. B., Dolder, P. J., Reeves, S., Robinson, P., and Jennings, S. (2016). Assessing fishery and ecological consequences of alternate management options for multispecies fisheries. ICES J. Mar. Sci. 73, 1503–1512. doi: 10.1093/icesjms/fsw028
Thorpe, R. B., Le Quesne, W. J. F., Luxford, F., Collie, J. S., and Jennings, S. (2015). Evaluation and management implications of uncertainty in a multispecies size-structured model of population and community responses to fishing. J. Methods Ecol. Evol. 6, 49–58. doi: 10.1111/2041-210X.12292
Thuiller, W., Richardson, D. M., Pysek, P., Midgley, G. F., Hughes, G. O., and Rouget, M. (2005). Niche-based modeling as a tool for predicting the risk of alien plant invasions at a global scale. Glob. Chang. Biol. 11, 2234–2250. doi: 10.1111/j.1365-2486.2005.001018.x
Tomczak, M., Heymans, J. J., Yletyinen, J., Niiranen, S., Otto, S. A., and Blenckner, T. (2013). Ecological network indicators of ecosystem status and change in the Baltic Sea. PLoS ONE 8:e75439. doi: 10.1371/journal.pone.0075439
Trifonova, N., Maxwell, D., Duplisea, D., Fernandes, J., and Tucker, A. (2015). Spatio-temporal Bayesian network models with latent variables for revealing trophic dynamics and functional networks in fisheries ecology. Ecol. Inform. 30, 142–158. doi: 10.1016/j.ecoinf.2015.10.003
Tsikliras, A. C., Dinouli, A., and Tsalkou, E. (2013). Exploitation trends of the Mediterranean and Black Sea fisheries. ACTA ADRIAT 54, 273–282.
Tucker, A., and Liu, X. (2004). A Bayesian network approach to explaining time series with changing structure. Intell. Data Anal. 8, 469–480.
UNEP (1998). “Report of the workshop on the ecosystem approach,” in Conference of the Parties to the Convention on Biological Diversity (Lingongwe: UNEP).
Uusitalo, L., Korpinen, S., Andersen, J. H., Niiranen, S., Valanko, S., Heiskanen, A.-S., et al. (2016). Exploring methods for predicting multiple pressures on ecosystem recovery: a case study on marine eutrophication and fisheries. Cont. Shelf Res. 121, 48–60. doi: 10.1016/j.csr.2015.11.002
van den Hove, S. (2007). A rationale for science–policy interfaces. Futures 39, 807–826. doi: 10.1016/j.futures.2006.12.004
van der Kooij, J., Kupschus, S., and Scott, B. E. (2011). Delineating the habitat of demersal fish assemblages with acoustic seabed technologies. ICES J. Mar. Sci. 68, 1973–1985. doi: 10.1093/icesjms/fsr124
van der Linden, P., Borja, A., Rodriguez, J. G., Muxica, I., Galparsoro, I., Patrício, J., et al. (2016). Spatial and temporal response of multiple trait-based indices to natural and anthropogenic seafloor disturbance. Ecol. Indic. 69, 617–628 doi: 10.1016/j.ecolind.2016.05.020
van der Molen, J., Smith, H. C. M., Lepper, P., Limpenny, S., and Rees, J. (2014). Predicting the large-scale consequences of offshore wind turbine array development on a North Sea ecosystem. Cont. Shelf Res. 85, 60–72, doi: 10.1016/j.csr.2014.05.018
van Leeuwen, S. M., Le Quesne, W. F., and Parker, E. R. (2016). Potential future fisheries yields in shelf waters: a model study of the effects of climate change and ocean acidification. Biogeosciences 13, 441–454, doi: 10.5194/bg-13-441-2016
van Leeuwen, S. M., Tett, P., Mills, D., and van der Molen, J. (2015). Stratified and nonstratified areas in the North Sea: Long-term variability and biological and policy implications. J. Geophys. Res. Oceans 120, 4670–4686. doi: 10.1002/2014jc010485
Verberk, W. C. E. P., van Noordwijk, C. G. E., and Hildrew, A. G. (2013). Delivering on a promise: integrating species traits to transform descriptive community ecology into a predictive science. Freshw. Sci. 32, 531–547. doi: 10.1899/12-092.1
Vichi, M., Lovato, T., Lazzari, P., Cossarini, G., Gutierrez Mlot, E., Mattia, G., et al. (2015). The Biogeochemical Flux Model (BFM): Equation Description and User Manual. BFM version 5.1. BFM Report series N. 1, Release 1.1, July 2015, Bologna, Italy, 104. Available online at: http://bfm-community.eu
Watson, R. T. (2005). Turning science into policy: challenges and experiences from the science-policy interface. Philos. Trans. R. Soc. Lond. B Biol. Sci. 360, 471–477. doi: 10.1098/rstb.2004.1601
Keywords: ecosystem modeling, good environmental status, marine strategy framework directive, indicators, assessment cycle, marine management
Citation: Lynam CP, Uusitalo L, Patrício J, Piroddi C, Queirós AM, Teixeira H, Rossberg AG, Sagarminaga Y, Hyder K, Niquil N, Möllmann C, Wilson C, Chust G, Galparsoro I, Forster R, Veríssimo H, Tedesco L, Revilla M and Neville S (2016) Uses of Innovative Modeling Tools within the Implementation of the Marine Strategy Framework Directive. Front. Mar. Sci. 3:182. doi: 10.3389/fmars.2016.00182
Received: 17 June 2016; Accepted: 06 September 2016;
Published: 28 September 2016.
Edited by:
Maria C. Uyarra, AZTI Tecnalia, SpainReviewed by:
Rodrigo Riera, Atlantic Environmental Marine Center (CIMA SL), SpainCopyright © 2016 Lynam, Uusitalo, Patrício, Piroddi, Queirós, Teixeira, Rossberg, Sagarminaga, Hyder, Niquil, Möllmann, Wilson, Chust, Galparsoro, Forster, Veríssimo, Tedesco, Revilla and Neville. This is an open-access article distributed under the terms of the Creative Commons Attribution License (CC BY). The use, distribution or reproduction in other forums is permitted, provided the original author(s) or licensor are credited and that the original publication in this journal is cited, in accordance with accepted academic practice. No use, distribution or reproduction is permitted which does not comply with these terms.
*Correspondence: Christopher P. Lynam, Y2hyaXMubHluYW1AY2VmYXMuY28udWs=
Disclaimer: All claims expressed in this article are solely those of the authors and do not necessarily represent those of their affiliated organizations, or those of the publisher, the editors and the reviewers. Any product that may be evaluated in this article or claim that may be made by its manufacturer is not guaranteed or endorsed by the publisher.
Research integrity at Frontiers
Learn more about the work of our research integrity team to safeguard the quality of each article we publish.