- 1Institute for Marine and Antarctic Studies, University of Tasmania, Hobart, TAS, Australia
- 2The Global Change Institute, The University of Queensland, St. Lucia, QLD, Australia
The responses of marine biota to global ocean change is characterized by multiple environmental drivers that interact to cause non-linear changes in organismal performance. Characterizing interactions is critical for us to predict whether multiple drivers will accelerate or mitigate future biological responses. There is now a large body of evidence that drivers do not act independently, a common null model, but rather have synergistic or antagonistic effects on organisms. We review the literature on interactions among environmental drivers such as ocean acidification and warming, and identify three common modes of interaction: physicochemical interactions in the seawater media outside organisms, interactions that operate on organisms directly, for example by altering physiological rates; and interactions that occur through changes in ecosystems, like predation. Interactions can also occur across these levels increasing the number of permutations for interaction, and point to a diverse range of modes of interplay. Identifying the appropriate mode will help generalize interaction types to unstudied contexts.
Introduction
Ocean biota will encounter changes to a wide range of biologically-influential properties, termed here as drivers rather than stressors (see Boyd and Hutchins, 2012) at local, regional and global scales. The challenge presented by climate change is to tease apart the ramifications of a suite of altered environmental properties on organisms and ecosystems—this has parallels with previous environmental problem-solving such as ecotoxicology (Breitburg et al., 1999), or acid rain (Pfister et al., 2014)—but the scale and complexity of the changes and hence the challenge to understand it is more daunting. It is becoming increasing clear that the cumulative effects of climate change will be driven not only by the influence of individual drivers on the biota, but by their interactive effects. Here we define drivers as environmental shifts that cause a quantifiable change in a biological response, like growth or population size (Boyd and Hutchins, 2012). Stressors have previously been defined as environmental drivers that have a negative effect on organism performance or populations when the driver is beyond its normal range of variation (Vinebrooke et al., 2004). Multiple drivers can interact in different ways to affect organisms. For instance, the effect of two drivers may be greater than (synergistic) or less than (antagonistic) their additive effects. Knowledge of the interaction type is essential for developing models that can predict biological outcomes (Boyd et al., 2015) and for ecosystem management (Brown et al., 2013).
The potential for a wide range of combinations of interlinked drivers in turn gives rise to different modes of interactions—which need to be quantified to predict how changing oceanic conditions alter biological and ecological processes. However, we lack a scheme for classifying different modes of interplay among multiple drivers. A scheme for classifying modes would facilitate comparisons across studies and help predict interaction types in unstudied systems. For instance, both synergistic and antagonistic interactions may be equally common when drivers act through foodwebs (Griffith et al., 2012), while antagonistic interactions may be more likely for drivers that act on populations (Brown et al., 2013). The interplay of many different factors—from local point-source perturbations—such as toxicants (Nikinmaa, 2013)—to globally-driven shifts such as Ocean Acidification (OA) (Kroeker et al., 2013) give rise to many different permutations of inter-related change (Boyd and Hutchins, 2012). This mini-review uses illustrative examples of interactions between environmental drivers to characterize these different modes of interactions.
Models for Classifying Interactions
Different categories of interactions can be classified using several different models (Soluk and Collins, 1988; Folt et al., 1999; Vinebrooke et al., 2004; Chait et al., 2007). All the models specify a null model that represents our expectation for the impact of multiple drivers if there were no interactions. The null expectation is calculated as a combination of individual driver effects. Typically, an additive model is used, so the null expectation is the sum the driver's effects when they operate in isolation. For all models, the observed outcome of multiple drivers is then classified as synergistic if the impact is greater than the null and antagonistic if the impact is less than the null. A response that would be classified as antagonistic using one model may be synergistic using another (e.g., Folt et al., 1999). The correct null model to choose depends on the mechanisms controlling how multiple drivers affect the response variable of interest (Table 1). Using an inappropriate null model to classify interactions could lead to inaccurate extrapolations of experimental results to biological outcomes in the field or future. It may also lead to inappropriate management advice (Brown et al., 2013).
When classifying interaction types, it is important to specify the direction of the driver's effects to organism (Boyd and Hutchins, 2012), which can be either negative or positive. For example an antagonistic effect results in a positive outcome for the organism if it reduces the physiological stress imparted by individual drivers. Antagonistic interactions can have a negative effect on organisms if the individual effects are positive, like iron and carbon dioxide fertilization, because the antagonism may indicate an interference mechanism that reduces the positive effects of the drivers.
Some authors further distinguish between two types of antagonisms. The first type occurs when the combination of two drivers is simply less than the expectation, such as when the first driver extirpates all sensitive species in a community, leaving only species that are tolerant to the influences of additional drivers (Vinebrooke et al., 2004). Mitigative (Brown et al., 2013) or suppressive (Chait et al., 2007) antagonisms occur when multiple drivers have a smaller effect than one driver alone. For instance, sediment loading and warming can both harm corals, but sediment can reduce the effect of warming on corals by reducing light stress (Anthony et al., 2007).
Multi-Driver Interactions
We have selected illustrative examples from research into how OA influences marine organisms. OA alone has been shown to have pronounced detrimental and beneficial effects on marine life (Wittmann and Pörtner, 2013) and hence it can act as a stressor but also as a driver (see Boyd and Hutchins, 2012). An increasing number of environmental manipulation studies are perturbing biota with OA and additional drivers such as warming (Kroeker et al., 2013). These studies are revealing that environmental drivers caused by human activities at different scales can interact across different scales of biological organization, from physical mechanisms to organisms and the ecosystems they occur in.
An important insight from OA studies is that acidification can interact with drivers that have local, regional and global causes. OA itself is generally regarded as a global driver, because rising atmospheric carbon dioxide has a global cause. OA interacts with global drivers, like warming, can alter the sensitivity of organisms to acidification (Kroeker et al., 2013). This is an example of how the interplay of two globally-altered (by climate change) drivers can influence marine life. The interactions of such global drivers as OA and warming can result in alteration of the distributional patterns of organisms such as in polar regions (Aronson et al., 2007; Sewell and Hofmann, 2011). There are other studies that illustrate how OA can also readily interact with local and regional drivers. For example, OA has been shown to interact with locally produced toxicants (Nikinmaa, 2013) and with nutrient-enriched coastal waters (Cai et al., 2011). At the level of regionally-influential drivers (sensu Boyd and Hutchins, 2012) enhanced UV radiation can also interact with OA resulting in either a detrimental (Gao and Zheng, 2010) or a beneficial (Gordillo et al., 2014) outcome for organisms.
Another important distinction that emerges from these examples is that drivers can interact to affect organisms directly or indirectly. Examples of direct effects include OA altering the effects of toxicants on organism physiology. Indirect effects can occur at multiple scales. For instance, warming can offset OA by decreasing the solubility of carbon dioxide, and fishing may offset the effects of OA on some molluscs by reducing predation on the molluscs (Griffith et al., 2011). The different scales of direct and indirect interactions suggest a scheme for classifying interaction models.
Modes of Driver Interplay
We present evidence of and examples for three modes of interaction that we term the (1) physicochemical, (2) organismal and (3) ecosystem modes (Figure 1A). We treat each in turn, but also acknowledge that drivers may affect organisms in multiple ways across these modes (Figure 1).
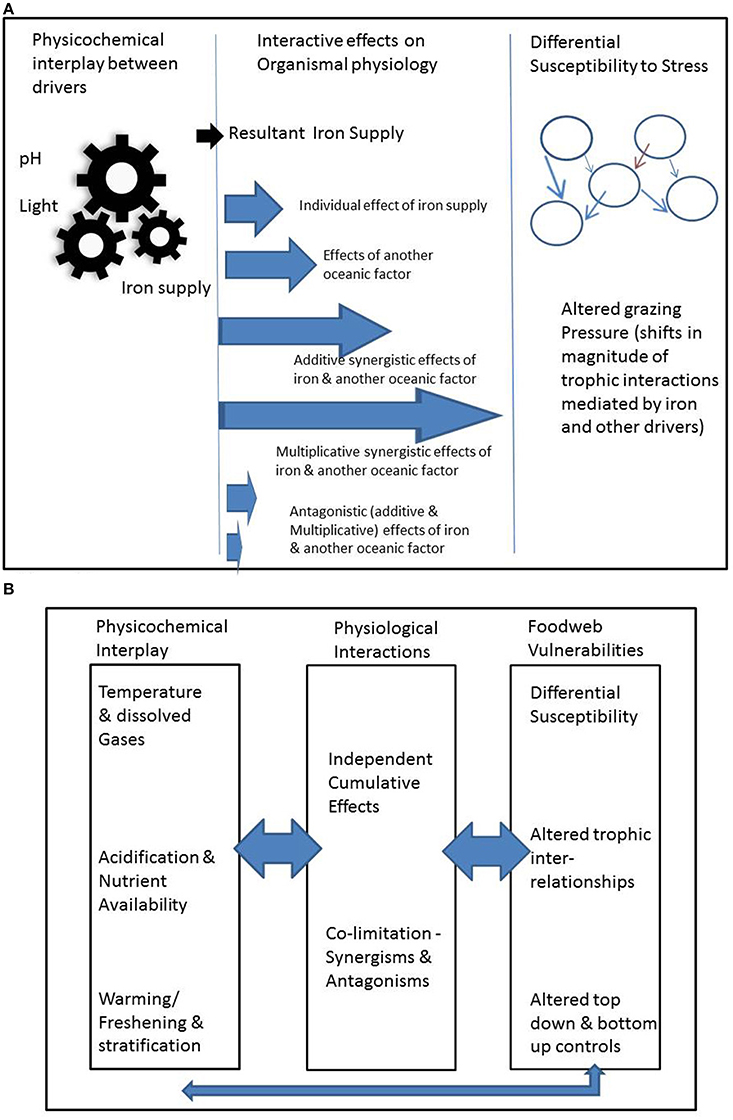
Figure 1. Summary of the range of permutations associated with interlinked different modes of interactions, illustrated in (A) for iron supply for phytoplankton (note brown arrow in right hand panel denotes changes in foodweb interactions driven by floristic and consequent faunistic shifts; see main text for details); (B) provides examples of physicochemical interactions, and how top-down ecological effects will interact across these modes of interactions.
Interactions at the Physico-Chemical Level
Physico-chemical interactions can be solely be driven by the interplay of physical processes such as warming and freshening in polar oceans [sea-ice melt, and changes in Evaporation minus Precipitation (i.e., E–P)]. Warming and freshening increase density stratification, which in turn reduces mixing of high oxygen nutrient-poor surface waters with deeper waters that are lower in oxygen, but nutrient-rich (Gruber, 2011). Lower mixing may result in lower delivery of nutrients to primary producers in the surface waters and may also contribute to hypoxic zones (Gruber, 2011). Physical processes such as warming can also alter the solubility of biologically-influential gasses such as oxygen and carbon dioxide, with consequent chemical effects—such as on the thermodynamics of carbonate chemistry. This physicochemical interaction would be further influenced by concurrent freshening (such as in the Arctic) which would alter the alkalinity of seawater (Boyd et al., 2015) and hence the thermodynamics of the carbonate system.
In addition to physical and physicochemical effects, there are also direct chemical interactions such between OA and carbonate chemistry via altered speciation, and between OA and pH-mediated changes to trace metal speciation (important for iron availability to marine biota) or the speciation of influential nutrients such as ammonium that each alters their availability to the biota (The Royal Society, 2005). Physicochemical interactions can occur among more than two drivers. For example recent evidence of the contributing role of OA, light and temperature on altering iron availability for phytoplankton (Sunda and Huntsman, 2011).
Interactions at the Organismal Level
Direct modes of interactions between drivers impact organismal physiology, such as growth rate and/or photosynthetic competence. The differing relationships of physiological interplay between drivers are well summarized by Saito et al. (2008) who categorize the joint limitation of physiological processes by more than one driver (termed co-limitation) into: independent nutrient co-limitation; biochemical substitution co-limitation; and biochemically-dependent co-limitation. In addition to the interactive effects of nutrient supply, there are also interactions between CO2, pH, irradiance and temperature (Boyd et al., 2010). Drivers that interact at the organismal level may also commonly interact at the physicochemical level, suggesting strong interplay across these modes (Figure 1). For example, OA interacts with iron by increasing primary production of phytoplankton in regions where iron is abundantly available (Hoppe et al., 2013). OA also affects iron availability at the physicochemical level by altering its speciation and hence availability to the biota (Sunda and Huntsman, 2011). The co-occurrence together of both interactive modes emphasizes the need to clearly demarcate their effects in experimental settings that attempt to determine the mechanistic underpinnings of interactions. There may be cases in which synergistic positive effects between indirect interactive modes may offset antagonistic negative effects resulting from direct physiological effects (Figure 1). Evidently, as we move beyond consideration of more than two drivers, unless we are aware of the range of indirect and direct effects, misattribution of cause and effect is an ever-present complication.
Interactions at the Ecosystem Level
Drivers that affect an organism may also affect its ecological interactions, including predators, competitors for prey and symbionts (Figure 1B). There are a wide range of interactive effects that act through ecosystems. Here we categorize them broadly based on how the drivers affect ecosystems: (a) variable sensitivities of organisms to drivers; (b) effects that directly alter the characteristics of the predator-prey relationship; (c) effects that indirectly alter the characteristics of the predator-prey relationship; (d) the collective effect of (a) to (c) across many facets of ecosystem functioning.
Not all species will respond to environmental drivers in the same way. The changes in a species' abundance in response to a driver may also influence its interaction with other species. For instance, ecosystem models have been used to predict the interactive effects of warming, OA and fishing on demersal and pelagic foodwebs (Griffith et al., 2012). OA and fishing were predicted to have an antagonistic effect on benthic molluscs, because while OA increased their mortality rate, fishing reduced predation pressure (Griffith et al., 2012). However, the interactive effect was species-specific, with synergistic and antagonistic effects predicted to occur with similar frequencies across all species in the selected foodweb (Griffith et al., 2011).
Interactions driven by differential sensitivities of species to drivers may be further exacerbated when they also span life histories and life cycles of individual organisms (Wittmann and Pörtner, 2013), and when the effects of other drivers, such as warming, also have distinctive effects across trophic levels, for example on their sensitivity to OA (Kroeker et al., 2013). It is also likely that the number of permutations, of (bottom-up) environmental drivers will vary across trophic levels, with primary producers such as phytoplankton likely to be jointly influenced by the alteration of at least five environmental properties (light, temperature, CO2, nutrients and trace metal supply) whereas higher trophic levels will be influenced by fewer environmental drivers (Boyd and Hutchins, 2012). However, such an imbalance across trophic levels will probably be offset by changes in other ecologically-driven drivers, for example prey availability (Folt et al., 1999; Griffith et al., 2011).
The next trophic characteristic to be influenced by changing interactions between drivers is the nature of the predator-prey relationship. This can be altered directly, for example, OA may alter the ability of prey species (such as sea-grasses, Arnold et al., 2012) to deter predators via loss of their chemical ecological defenses (Kroeker et al., 2013). Poore et al. (2013) report how a shift in environmental conditions can alter the palatability of prey species (Sargassum linearifolium) for marine amphipods. Predators, also are not invulnerable to the effects of OA, with indirect effects on their behavior (Nilsson et al., 2012), also noted by Kroeker et al. (2013). The balance across a predator-prey relationship could also be altered by OA-mediated decreases in structural or skeletal elements due to decreases in net calcification rates (i.e., calcification minus dissolution) (Ries, 2011a,b).
Examples of indirect alteration of the predator-prey relationship include the influence of warming on the size-structure of the prey assemblage (Brose et al., 2012) which again may alter the feeding efficiency of the predators, for example micro- (Fenchel, 1980) or meso-zooplankton (Kiorboe, 2008). Brose et al. (2012, and references therein) also point to a wide range of other fundamental trophic relationships “food-web topologies, community stability and functioning” (summarized in their Figure 1) which will cumulatively alter ecosystem performance and may result in fundamental restructuring of communities. The case studies synthesized by Brose et al. (2012) focus primarily on the effects of warming on terrestrial ecosystems. Clearly the effect of temperature can result in a wide range of thermal cascades (summarized in their Figure 2 by Brose et al., 2012) across trophic levels within foodwebs. However, there is growing evidence in the marine literature of the influence of other confounding (relative to temperature) environmentally-mediated effects (such as OA, Byrne et al., 2013, nutrient supply, Marañón et al., 2014, trace metal supply, Boyd et al., 2012) on the size structure of ecosystems. Hence the cumulative effects of the inter-relationships between the three interactive modes for marine ecosystems represented in Figure 1B will be potentially more complex than those presented in Brose et al. (2012). In marine systems these inter-relationships will be set jointly by the composition and structure of each ecosystem, the nature of and controls upon each prey-predator-prey relationship, and the suite of environmental drivers that control the physiology of each organism, and environmentally-mediated alteration of the geographical distributions of predators and prey.
The combined effects of drivers directly on organisms and indirectly on food-web relationships can alter entire ecosystems when they affect habitat-forming species. Coral reef ecosystems can switch between alternate stable states (termed a regime shift), where either corals or macro-algae dominate (Mumby et al., 2007). The resilience of the coral-dominated state is altered by numerous drivers, including OA and warming that reduce coral growth and cause coral mortality; and overfishing that releases macro-algal populations from control by herbivorous fish (Anthony et al., 2011). For coral reef ecosystems, the direct and indirect interactions of multiple local and global drivers may cause diverse coral dominated ecosystems to be replaced with macro-algal dominated ecosystems (Anthony et al., 2011).
Other research fields, such as the ecosystem-level responses to increased UV radiation (Bothwell et al., 1994; Cabera et al., 1997; Mostajir et al., 1999) or nutrients and toxic trace metals (Breitburg et al., 1999) also offer valuable insights into the cumulative effects of bottom-up and top-down effects presented in Figure 1B. However, in the case of alteration of iron supply (Boyd et al., 2012) or increased UV radiation (Mostajir et al., 1999), these studies only offer insights into the change in an individual environmental property on an ecosystem, not into concurrent shifts in multiple properties (Figure 1). Hence there may be many ways/mechanisms to drive differential susceptibility to the cumulative effects of multiple drivers. For example, do some drivers act in an additive (sensu Folt et al., 1999) manner for one trophic level but in multiplicative ways for others. Breitburg et al. (1999) have reported how such differential sensitivity to drivers across trophic levels can result in the transmission of the effects of environmental drivers through foodwebs. Such transmission of environmental stress effects can form potent linkages between the lower and upper components of foodwebs, such as in estuarine systems (Bundy et al., 2003). Other examples of complex ecosystem-level interactions, include composite effects of multiple drivers: for example in polar waters, warming may result in the range extension and introduction of additional predators such as crabs (Aronson et al., 2007), that may be able to exploit the OA-mediated thinning of the calcite skeletons of prey (Sewell and Hofmann, 2011). One further confounding interaction that was not included in Figure 1B is the additional interactive environmental stress associated with concurrent fisheries exploitation (for example see Breitburg et al., 2009).
Future Directions for Quantifying Modes of Interactions
Our scheme for classifying the modes of interactions provides a framework for identifying future directions for research into modes of interactions. We have three recommendations for future research.
(1) The mode or modes of study should be identified explicitly when studying interacting drivers. Doing so will facilitate isolation of the mechanisms causing an observed interaction. Interactions may occur across multiple modes and the mode of study will depend on the scale of the experiment. For practical purposes, most experiments are conducted at the laboratory level, which precludes studying some modes of interaction, like trophic interactions over larger scales. Ocean experiments, like the holistic ecosystem-wide mesoscale (i.e., 10 km length-scale) iron-enrichment studies provide widespread evidence of “knock-on” restructuring of grazer communities by bottom-up environmentally-mediated floristic shifts (Boyd et al., 2012). Even in this relatively simple example—in which only one environmental driver (iron supply) was altered, such faunistic shifts may also mediate shifts in the marine environment [release of potential (Sulfur-based) cloud condensation nuclei, Turner et al., 2004], which may induce further environmental feedbacks on the biota.
Experiments may also confound different modes if they do not isolate them a priori. For instance, a lab experiment may conflate physicochemical and organismal modes of interactions (see discussion in Sunda and Huntsman, 2011), resulting in inaccurate inferences when outcomes are translated to the ocean environment.
(2) To quantify the net effect of multiple drivers to biota, we need to know the effects of drivers at different modes (Figure 1B). However, many studies only focus on one or two of the modes we have proposed. For instance, ecosystem models typically examine interactions that result from changes in predator prey abundance, but do not consider physicochemical modes. Adding models for other modes can provide fruitful insights into where drivers have the greatest effects on biota (e.g., Mumby and van Woesik, 2014).
(3) Across all studies, the most common null model for classifying interaction types is the additive model. However, many of the mechanisms underlying the modes we propose here may be better described by non-additive models, like the multiplicative model or the risk model. For instance, the additive model is often used to classify interactive effects on organism abundance (e.g., Griffith et al., 2012; Hoppe et al., 2013). Abundance may also be modeled as a multiplicative process, because abundance in the future is a multiple of the historical number of breeding organisms. An important area for future theoretical work is to conceptualize which null models are most appropriate for which modes.
Future research into the biological effects of multiple drivers should use modes of interactions as a platform to design manipulation studies. Although we have an incomplete understanding of the outcomes of the interplay between these different modes of interactions, it is already clear that they will vary between ecosystems, and regionally, and hence must be considered on a case-by-case basis, which may initially make inter-comparisons between systems problematic. However, by demarcation of these different interactive modes we can begin to develop a mechanistic understanding of the relative influence on biological systems of each mode, and of their contribution to the cumulative effect on ecosystems.
Conflict of Interest Statement
The authors declare that the research was conducted in the absence of any commercial or financial relationships that could be construed as a potential conflict of interest.
References
Anthony, K. R. N., Connolly, S. R., and Hoegh-Guldberg, O. (2007). Bleaching, energetics, and coral mortality risk: effects of temperature, light, and sediment regime. Limnol. Oceanogr. 52, 716–726. doi: 10.4319/lo.2007.52.2.0716
Anthony, K. R. N., Maynard, J. A., Diaz-Pulido, G., Mumby, P. J., Marshall, P. A., Cao, L., et al. (2011). Ocean acidification and warming will lower coral reef resilience. Glob. Change Biol. 17, 1798–1808. doi: 10.1111/j.1365-2486.2010.02364
Pubmed Abstract | Pubmed Full Text | CrossRef Full Text | Google Scholar
Arnold, T., Mealey, C., Leahey, H., Miller, A. W., Hall-Spencer, J., Milazzo, M., et al. (2012). Ocean acidification and the loss of phenolic substances in marine plants. PLoS ONE 7:e35017. doi: 10.1371/journal.pone.0035107
Aronson, R. B., Thatje, S., Clarke, A., Peck, L. S., Blake, D. B., Wilga, C. D., et al. (2007). Climate change and invasibility of the Antarctic benthos. Annu. Rev. Ecol. System. 38, 129–154. doi: 10.1146/annurev.ecolsys.38.091206.095525
Bothwell, M. L., Sherbot, D. M. J., and Pollock, C. W. (1994). Ecosystem response to solar ultraviolet-B radiation: influence of trophic-level interactions. Science 265, 97–100. doi: 10.1126/science.265.5168.97
Pubmed Abstract | Pubmed Full Text | CrossRef Full Text | Google Scholar
Boyd, P. W., Bakker, D. C. E., and Chandler, C. (2012). A new database to explore the findings from large-scale ocean iron enrichments experiments. Oceanography 25, 64–71. doi: 10.5670/oceanog.2012.104
Boyd, P. W., and Hutchins, D. A. (2012). Understanding the responses of ocean biota to a complex matrix of cumulative anthropogenic change. Mar. Ecol. Prog. Ser. 470, 125–135. doi: 10.3354/meps10121
Boyd, P. W., Lennartz, S. T., Glover, D. M., and Doney, S. C. (2015). Biological ramifications of climate-change mediated oceanic multi-stressors. Nat. Clim. Change 5, 71–79. doi: 10.1038/nclimate2441
Boyd, P. W., Strzepek, R., Fu, F. X., and Hutchins, D. A. (2010). Environmental control of open-ocean phytoplankton groups: now and in the future. Limnol. Oceanogr. 55, 1353–1376. doi: 10.4319/lo.2010.55.3.1353
Breitburg, D. L., Craig, J. K., Fulford, R. S., Rose, K. A., Boynton, W. R., Brady, D. C., et al. (2009). Nutrient enrichment and fisheries exploitation: interactive effects on estuarine living resources and their management. Hydrobiologia 629, 31–47. doi: 10.1007/s10750-009-9762-4
Breitburg, D. L., Sanders, J. G., Gilmour, C. C., Hatfield, C. A., Osman, R. W., Riedel, G., et al. (1999). Variability in responses to nutrients and trace metals, and transmission of stressor effects through an estuarine food web. Limnol. Oceanogr. 44, 837–863. doi: 10.4319/lo.1999.44.3_part_2.0837
Brose, U., Dunne, J. A., Montoya, J. M., Petchey, O. L., Schneider, F. D., and Jacob, U. (2012). Climate change in size-structured ecosystems. Philos. Trans. R. Soc. B 367, 2903–2912. doi: 10.1098/rstb.2012.0232
Pubmed Abstract | Pubmed Full Text | CrossRef Full Text | Google Scholar
Brown, C. J., Saunders, M. I., Possingham, H. P., and Richardson, A. J. (2013). Managing for interactions between local and global stressors of ecosystems. PLoS ONE 8:e65765. doi: 10.1371/journal.pone.0065765
Bundy, M. H., Breitburg, D. L., and Sellner, K. G. (2003). The responses of Patuxent River upper trophic levels to nutrient and trace element induced changes in the lower food web. Estuaries 26, 365–384. doi: 10.1007/BF02695974
Byrne, M., Foo, S., Soars, N., Wolfe, K., Nguyen, H., Hardy, N., et al. (2013). Ocean warming will mitigate the effects of acidification on calcifying sea urchin larvae (Heliocidaris tuberculata) from the Australian global warming hot spot. J. Exp. Mar. Biol. Ecol. 448, 250–257. doi: 10.1016/j.jembe.2013.07.016
Cabera, S., Lopez, M., and Tartarotti, B. (1997). Phytoplankton and zooplankton respone to ultraviolet radiation in a high-altitude Andean lake: short versus long term effects. J. Plank. Res. 19, 1565–1582. doi: 10.1093/plankt/19.11.1565
Cai, W.-J., Hu, X., Huang, W.-J., Murrell, M. C., Lehrter, J. C., Lohrenz, S. E., et al. (2011). Acidification of subsurface coastal waters enhanced by eutrophication. Nat. Geosci. 4, 766–770. doi: 10.1038/ngeo1297
Pubmed Abstract | Pubmed Full Text | CrossRef Full Text | Google Scholar
Chait, R., Craney, A., and Kishony, R. (2007). Antibiotic interactions that select against resistance. Nature 446, 668–671. doi: 10.1038/nature05685
Pubmed Abstract | Pubmed Full Text | CrossRef Full Text | Google Scholar
Fenchel, T. (1980). Relation between particle size selection and clearance in suspension-feeding ciliates. Limnol. Oceanogr. 25, 733–738. doi: 10.4319/lo.1980.25.4.0733
Folt, C. L., Chen, C. Y., Moore, M. V., and Burnaford, J. (1999). Synergism and antagonism among multiple stressors. Limnol. Oceanogr. 44, 864–877. doi: 10.4319/lo.1999.44.3_part_2.0864
Gao, K., and Zheng, Y. (2010). Combined effects of ocean acidification and solar UV radiation on photosynthesis, growth, pigmentation and calcification of the coralline alga Corallina sessilis (Rhodophyta). Glob. Change Biol. 16, 2388–2398. doi: 10.1111/j.1365-2486.2009.02113.x
Gordillo, F. J., Aguilera, J., Wiencke, C., and Jiménez, C. (2014). Ocean acidification modulates the response of two Arctic kelps to ultraviolet radiation. J. Plant Physiol. 173C, 41–50. doi: 10.1016/j.jplph.2014.09.008
Pubmed Abstract | Pubmed Full Text | CrossRef Full Text | Google Scholar
Griffith, G. P., Fulton, E. A., Gorton, R., and Richardson, A. J. (2012). Predicting interactions among fishing, ocean warming, and ocean acidification in a marine system with whole-ecosystem models. Conserv. Biol. 26, 1145–1152. doi: 10.1111/j.1523-1739.2012.01937.x
Pubmed Abstract | Pubmed Full Text | CrossRef Full Text | Google Scholar
Griffith, G. P., Fulton, E. A., and Richardson, A. J. (2011). Effects of fishing and acidification-related benthic mortality on the southeast Australian marine ecosystem. Glob. Change Biol. 17, 3058–3074. doi: 10.1111/j.1365-2486.2011.02453.x
Gruber, N. (2011). Warming up, turning sour, losing breath: ocean biogeochemistry under global change. Philos. Trans. A Math. Phys. Eng. Sci. 369, 1980–1996. doi: 10.1098/rsta.2011.0003
Pubmed Abstract | Pubmed Full Text | CrossRef Full Text | Google Scholar
Hoppe, C. J., Hassler, C. S., Payne, C. D., Tortell, P. D., Rost, B., and Trimborn, S. (2013). Iron limitation modulates ocean acidification effects on southern ocean phytoplankton communities. PLoS ONE 8:e79890. doi: 10.1371/journal.pone.0079890
Pubmed Abstract | Pubmed Full Text | CrossRef Full Text | Google Scholar
Kiorboe, T. (2008). A Mechanistic Approach to Plankton Ecology. Princeton, NJ: Princeton University Press.
Kroeker, K. J., Kordas, R. L., Crim, R., Hendriks, I. E., Ramajo, L., Gattuso, G. S., et al. (2013). Impacts of ocean acidification on marine organisms: quantifying sensitivities and interaction with warming. Glob. Change Biol. 19, 1884–1896, doi: 10.1111/gcb.12179
Pubmed Abstract | Pubmed Full Text | CrossRef Full Text | Google Scholar
Marañón, E., Cermeño, P., Huete-Ortega, M., López-Sandoval, D. C., Mouriño-Carballido, B., and Rodríguez-Ramos, T. (2014). Resource supply overrides temperature as a controlling factor of marine phytoplankton growth. PLoS ONE 9:e99312. doi: 10.1371/journal.pone.0099312
Pubmed Abstract | Pubmed Full Text | CrossRef Full Text | Google Scholar
Mostajir, B., Demers, S., de Mora, S., Belzile, C., Chanut, J-.P., Roy, S., et al. (1999). Experimental test of the effect of ultra-violet-B radiation in a planktonic community. Limnol. Oceangr. 44, 586–596.
Mumby, P. J., Hastings, A., and Edwards, H. J. (2007). Thresholds and the resilience of Caribbean coral reefs. Nature 450, 98–101. doi: 10.1038/nature06252
Pubmed Abstract | Pubmed Full Text | CrossRef Full Text | Google Scholar
Mumby, P. J., and van Woesik, R. (2014). Consequences of ecological, evolutionary and biogeochemical uncertainty for coral reef responses to climatic stress. Curr. Biol. 24, R413–R423. doi: 10.1016/j.cub.2014.04.029
Pubmed Abstract | Pubmed Full Text | CrossRef Full Text | Google Scholar
Nikinmaa, M. (2013). Climate change and ocean acidification—Interactions with aquatic toxicology. Aquat. Toxicol. 126, 365–372. doi: 10.1016/j.aquatox.2012.09.006
Pubmed Abstract | Pubmed Full Text | CrossRef Full Text | Google Scholar
Nilsson, G. E., Dixson, D. L., Domenici, P., McCormick, M. I., Sørensen, C., Watson, S.-A., et al. (2012). Near-future CO2 levels alter fish behaviour by interference with neurotransmitter function. Nat. Clim. Change, 2, 201–204. doi: 10.1038/nclimate1352
Pfister, C. A., Esbaugh, A. J., Frieder, C. A., Baumann, H., Bockmon, E. E., White, M. M., et al. (2014). Detecting the unexpected: a research framework for ocean acidification. Environ. Sci. Technol. 48 9982–9994. doi: 10.1021/es501936p
Pubmed Abstract | Pubmed Full Text | CrossRef Full Text | Google Scholar
Poore, A. G. B., Graba-Landry, A., Favret, M., Brennand, H. S., Byrne, M., and Dworjanyn, S. A. (2013). Direct and indirect effects of ocean acidification and warming on a marine plant–herbivore interaction. Oecologia 173, 1113–1124. doi: 10.1007/s00442-013-2683-y
Pubmed Abstract | Pubmed Full Text | CrossRef Full Text | Google Scholar
Ries, J. B. (2011b). A physicochemical framework for interpreting the biological calcification response to CO2-induced ocean acidification. Geochim. Cosmochim. Acta 75, 4053–4064. doi: 10.1016/j.gca.2011.04.025
Saito, M. A., Goepfert, T. J., and Ritt, J. T. (2008). Some thoughts on the concept of colimitation: three definitions and the importance of bioavailability. Limnol. Oceanogr. 53, 276–290. doi: 10.4319/lo.2008.53.1.0276
Sewell, M. A., and Hofmann, G. E. (2011). Antarctic echinoids and climate change: a major impact on the brooding forms. Glob. Change Biol. 17, 734–744. doi: 10.1111/j.1365-2486.2010.02288.x
Soluk, D. A., and Collins, N. C. (1988). Synergistic interactions between fish and stoneflies: facilitation and interference among stream predators. Oikos 52, 94–100. doi: 10.2307/3565987
Sunda, W. G., and Huntsman, S. A. (2011). Interactive effects of light and temperature on iron limitation in a marine diatom: implications for marine productivity and carbon cycling. Limnol. Oceanogr. 56, 1475–1488. doi: 10.4319/lo.2011.56.4.1475
The Royal Society. (2005). Ocean Acidification due to Increasing Atmospheric Carbon Dioxide. Cardiff: The Clyvedon Press Ltd.
Turner, S. M., Harvey, M. J., Law, C. S., Nightingale, P. D., and Liss, P. S. (2004). Iron-induced changes in oceanic sulfur biogeochemistry. Geophys. Res. Lett. 31, L14307. doi: 10.1029/2004GL020296
Vinebrooke, R. D., Cottingham, K. L., Norberg, J., Scheffer, M., Dodson, S. I., Maberly, S.C., et al. (2004). Impacts of multiple stressors on biodiversity and ecosystem functioning: the role of species co-tolerance. Oikos 104, 451–457. doi: 10.1111/j.0030-1299.2004.13255.x
Keywords: global change biology, multiple drivers, modes of interactions, marine ecology, organismal physiology
Citation: Boyd PW and Brown CJ (2015) Modes of interactions between environmental drivers and marine biota. Front. Mar. Sci. 2:9. doi: 10.3389/fmars.2015.00009
Received: 04 November 2014; Accepted: 03 February 2015;
Published online: 27 February 2015.
Edited by:
Corinna Schrum, University of Bergen, NorwayReviewed by:
Periyadan K. Krishnakumar, King Fahd University of Petroleum and Minerals, Saudi ArabiaMarie Maar, Aarhus University, Denmark
Copyright © 2015 Boyd and Brown. This is an open-access article distributed under the terms of the Creative Commons Attribution License (CC BY). The use, distribution or reproduction in other forums is permitted, provided the original author(s) or licensor are credited and that the original publication in this journal is cited, in accordance with accepted academic practice. No use, distribution or reproduction is permitted which does not comply with these terms.
*Correspondence: Philip W. Boyd, Institute for Marine and Antarctic Studies, University of Tasmania, IMAS Waterfront Building, 20 Castray Esplanade, Battery Point, TAS 7004, Australia e-mail:cGhpbGlwLmJveWRAdXRhcy5lZHUuYXU=
†Present address: Christopher J. Brown, Australian Rivers Institute, Griffith University, Nathan, QLD, Australia