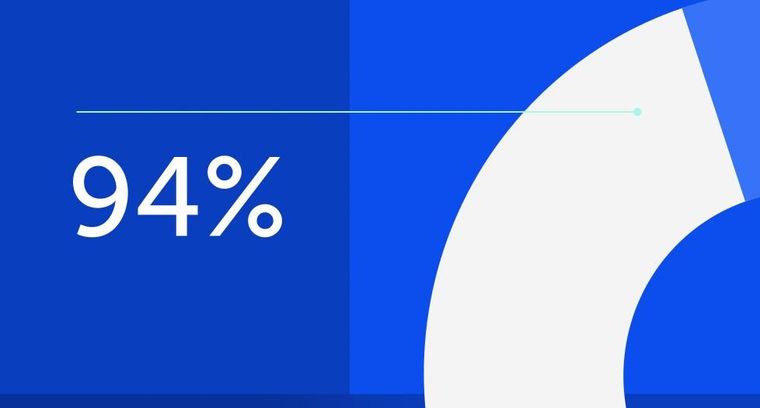
94% of researchers rate our articles as excellent or good
Learn more about the work of our research integrity team to safeguard the quality of each article we publish.
Find out more
METHODS article
Front. Manuf. Technol., 21 December 2023
Sec. Additive Processes
Volume 3 - 2023 | https://doi.org/10.3389/fmtec.2023.1271190
This study presents an overview and a few case studies to explicate the transformative power of diverse imaging techniques for smart manufacturing, focusing largely on various in-situ and ex-situ imaging methods for monitoring fusion-based metal additive manufacturing (AM) processes such as directed energy deposition (DED), selective laser melting (SLM), electron beam melting (EBM). In-situ imaging techniques, encompassing high-speed cameras, thermal cameras, and digital cameras, are becoming increasingly affordable, complementary, and are emerging as vital for real-time monitoring, enabling continuous assessment of build quality. For example, high-speed cameras capture dynamic laser-material interaction, swiftly detecting defects, while thermal cameras identify thermal distribution of the melt pool and potential anomalies. The data gathered from in-situ imaging are then utilized to extract pertinent features that facilitate effective control of process parameters, thereby optimizing the AM processes and minimizing defects. On the other hand, ex-situ imaging techniques play a critical role in comprehensive component analysis. Scanning electron microscopy (SEM), optical microscopy, and 3D-profilometry enable detailed characterization of microstructural features, surface roughness, porosity, and dimensional accuracy. Employing a battery of Artificial Intelligence (AI) algorithms, information from diverse imaging and other multi-modal data sources can be fused, and thereby achieve a more comprehensive understanding of a manufacturing process. This integration enables informed decision-making for process optimization and quality assurance, as AI algorithms analyze the combined data to extract relevant insights and patterns. Ultimately, the power of imaging in additive manufacturing lies in its ability to deliver real-time monitoring, precise control, and comprehensive analysis, empowering manufacturers to achieve supreme levels of precision, reliability, and productivity in the production of components.
The emergence of Industry 4.0 over the last decade has seen manufacturing industries transforming themselves by integrating physical production and operations with smart digital technologies and big data, thereby creating a highly interconnected network of manufacturing devices resulting in efficient and automated production workflows (Zhong et al., 2017). The increasing use of sensor-fusion based technologies in manufacturing, wherein data from multiple sensors are fused to produce reliable information, yields a more robust understanding of the physics behind manufacturing processes and significantly contributes towards improving product quality control (Kumar and Garg, 2004). Imaging techniques have emerged as central tools within the space of additive manufacturing, revolutionizing industry.
The machine vision market, which encompasses imaging systems in manufacturing, has been experiencing significant growth. According to a report by Fortune Business Insights (The global machine vision market, 2023), the global machine vision market was valued at around $9.01 billion in 2022 and is projected to reach $16.82 billion by 2030, with a compound annual growth rate (CAGR) of 8.2% during the forecast period. The integration of AI and machine learning techniques with imaging systems has further fueled the growth by enabling automated decision-making and real-time monitoring, enhancing overall manufacturing efficiency. From machine vision systems that perform automated quality inspections to thermal imaging cameras that detect anomalies in temperature distributions, imaging technologies are a powerful tool to visualize and understand complex manufacturing processes with unique clarity.
In the realm of additive manufacturing, two major imaging techniques exist: in-situ imaging and ex-situ or post-processing imaging. Each technique possesses distinct advantages and disadvantages, but in-situ imaging holds significant importance, particularly in the context of real time process monitoring. In-situ imaging, within the realm of manufacturing and material processing, is a technique that involves the capture and analysis of data directly within the operational environment. This is done without disrupting ongoing processes or necessitating the removal of samples for external examination.
This method allows for the real-time monitoring and analysis of various phenomena, such as alterations in material properties, structural dynamics, and process conditions. These observations provide valuable insights into the underlying mechanisms at work. By studying the process in its natural state, researchers can gain a holistic understanding of the dynamics and behaviors involved. This facilitates the identification of key patterns and trends, as well as potential areas for optimization or intervention. This technique enables the continuous monitoring of manufacturing processes, resulting in the generation of large volumes of data. Subsequently, these data sets can be subjected to analysis, aiming to extract meaningful and valuable information.
While not real-time, ex-situ imaging techniques offer detailed characterization of the manufactured part. Typically, these techniques involve capturing images and relevant data from samples to analyze their mechanical and structural properties. Notable examples include technologies such as scanning electron microscopy, optical microscopy, and profilometer-based scanning. These techniques provide highly accurate and precise characterizations of the samples, making them indispensable for quality control as well as research and development efforts.
Ex-situ imaging has been a mainstay in the industry for decades. However, several compelling reasons have underscored the need for in-situ imaging techniques. Firstly, ex-situ techniques do not provide immediate feedback or the ability to address issues as they arise. This is particularly true for time-sensitive industries such as additive manufacturing, where near real-time adjustments are necessary. Another limitation of ex-situ techniques is their inability to capture the process dynamics as the manufacturing process progresses.
In contrast, in-situ techniques can circumvent some of the redundancies inherent in ex-situ imaging, making them more practical in today’s smart additive manufacturing environment. This paper discusses ex-situ imaging techniques tangentially, with a specific focus on the integration of AI and ML frameworks.
The utilization of these in-situ imaging approaches offers a data-driven perspective, allowing for the identification of patterns, anomalies, and correlations that might remain unnoticed using conventional methods (Iquebal et al., 2020). The continuous monitoring and analysis of data streams enable real-time decision-making, as deviations from desired process conditions or quality specifications can be promptly identified. This timely feedback loop allows for immediate adjustments and interventions to uphold or improve manufacturing performance. However, these in-situ image techniques are prevented in implementation due to high markup prices, which over the time will become feasible for everyone to use such techniques.
While the initial high costs currently deter the widespread adoption of in-situ imaging techniques, ongoing research and technological advancements are anticipated to lead to cost reductions. As these costs decrease, these techniques will become increasingly accessible to a wider range of manufacturers, enabling them to harness the advantages of data-driven decision-making, real-time monitoring, and process optimization. The industry also faces significant challenges, such as the effective development and deployment of an appropriate edge computing setup capable of analyzing large volumes of high-quality image data in real-time. This capability to process vast amounts of data within milliseconds is crucial for applications where prompt decision-making is essential.
At present, the options for edge computing are limited, either due to high capital investment or processing speeds. However, with advancements in GPU technologies, the development of more powerful and energy-efficient hardware, and better-optimized algorithms, it has become feasible to achieve near real-time decision-making, even in implementations where all raw data is used for analysis. On another front, there has been a shift towards the concept of ‘just-the-right data’ or feature extraction. In this approach, only the important features of the raw data are extracted, while the redundant imaging data is discarded, enabling real-time in-situ monitoring.
The generation of high-quality image data for training machine learning and AI models is expensive, posing a challenge for industries to widely adopt such techniques. Even with advancements in imaging technology leading to cheaper options that maintain high image quality, processes such as storage and labeling of such high-resolution datasets are highly resource-intensive, further straining a company’s finances. However, ML/AI methods have evolved to enable the extraction of valuable insights even from low-quality image data. Techniques such as transfer learning and data augmentation have provided the means for artificial data generation and training more robust models with less pristine data.
While there are still some restrictions on the widespread adoption of in-situ imaging techniques, they offer a plethora of advantages over other sensing modalities. They provide high-resolution visual data that works exceptionally well with modern deep learning architectures while also being human-friendly for quick human decision-making or intervention. Intricate visual patterns and anomaly detection are possible, which might evade detection via other sensing technologies. In-situ imaging also provides more flexibility and adaptability when it comes to reproducing the same setup elsewhere, making them versatile for various applications. Most other sensing modalities require some amount of contact with the process or the machine, while these machine vision-based in-situ methods provide for non-contact measurements and monitoring. Such methods also relax the need for specialized signal conditioning or data acquisition system requirements by often providing plug-and-play usage.
The subsequent sections of this paper are structured as follows: Section 2 comprises a comprehensive literature review as listed in Table 1 that specifically concentrates on imaging techniques employed within the field of additive manufacturing. Moreover, detailed case studies are provided in Section 3, showcasing experiments carried out on DED process at Texas A&M University. In-situ imaging methods are discussed, about the utilization of high-speed cameras, thermal cameras, and digital cameras for real-time observation and analysis. Furthermore, comprehensive exploration is undertaken on post-processing imaging techniques, specifically highlighting the application of 3D optical profilometry and the image segmentation toolbox for defect detection. Concluding remarks are presented in Section 4.
This section evaluates key optical sensing technologies in literature for in-situ imaging measurements in additive manufacturing. The imaging techniques are classified based on the type of wave used for imaging: electromagnetic waves (see Figure 1) and mechanical waves (e.g., laser ultrasonic and spatially resolved acoustic spectroscopy imaging). While not exhaustive, the primary focus is on the most significant imaging techniques that offer high-resolution capture of process physics and integration with machine learning and artificial intelligence methods during model training. The objective is to assess the capabilities and performance of these technologies in a scientific context and to highlight their utility for non-destructive evaluation of surface characteristics during additive manufacturing.
Radio waves, characterized by longer wavelengths and lower frequencies relative to other forms of electromagnetic radiation, are not a preferred choice for high-resolution imaging in additive manufacturing. Although their propensity to traverse large distances renders them suitable for communication and data transmission, their interaction with matter does not facilitate non-destructive evaluation and process monitoring in additive manufacturing. Conversely, gamma rays, characterized by extremely short wavelengths and high frequencies are highly energetic. While gamma rays have the ability to penetrate deep into materials and can be used for non-destructive testing, their adoption in additive manufacturing for in-situ process monitoring is limited due to safety concerns.
High speed X-ray imaging and X-ray diffraction studies for in-situ characterization of Laser Bed Powder Fusion (LBPF) process have revealed several significant phenomena such as melt pool dynamics, solidification rates, phase transformation and pore formation mechanism due to keyhole at high spatial and temporal resolutions (Zhao et al., 2017; Leung et al., 2018). Wolff et al. illustrated the use of high-speed synchrotron hard X-ray imaging to study the pore formation dynamics in DED with micrometer spatial resolution and microsecond temporal resolution as shown in Figure 2. Due to the inherent difference in the way DED and LBPF systems are designed, the pore formation dynamics differ for both the processes. Particle deposition was found to be the key driver behind pore formation due to lack of fusion in DED and the presence of voids between the packed particles on the powder bed was the primary reason for porosity due to lack of fusion in LPBF (Wolff et al., 2021a; Wolff et al., 2021b). Owing to the difficulties for X-ray passage in commercial PBF systems, Bidare et al. designed an open architecture for metal PBF system accommodating for two X-ray imaging directions and the entire set up being easily transportable allowing for inspections at different measurement facilities (Bidare et al., 2017). The widespread adoption of in-situ X-ray imaging for DED and other AM processes at an industrial scale faces several challenges. High costs are a significant deterrent, and the requirement for a complex setup that demands regular adjustments complicates operational procedures. Furthermore, environmental factors, such as ionizing radiation, as well as material-specific properties such as emissivity and reflectivity, pose additional challenges to its applicability.
Optical methods that utilize electromagnetic waves in the visible spectrum are among the most frequently employed in-situ monitoring systems for additive manufacturing. These methods leverage different types of detectors to capture high-speed images of the process, providing valuable insights into the material deposition and quality assurance aspects of additive manufacturing.
Stereo vision is a method for reconstructing the 3-D structure of an object from corresponding points from 2 cameras by triangulation. Based on the configuration of the cameras and their intrinsic parameters, one can calibrate the “line of sight” of each pixel, and the intersection of the corresponding lines of sight from both the cameras will help us infer the 3-D position as shown in Figure 3 (Lazaros et al., 2008; Kanatani et al., 2008). With respect to metal AM, stereovision can help capture the geometry of melt pool and identify the size, speed, direction, and age of spatter ejected from the laser melt pool (Eschner et al., 2020). Barrett et al., employed a stereovision camera system to record the selective laser melting of maraging steel, with two cameras in 1,000 fps setting having 1,280 × 720-pixel count resulting in a spatial resolution of about 20 μm/pixel. The cameras were synchronized to measure stereoscopic features of the resulting spatter. Laser spatter can help us infer the process and quality of the part being built in-situ with a qualify-as-you-go methodology (Barrett et al., 2019; Barrett et al., 2018a). Liu et al. investigated a stereo vision-based system for a hybrid (additive and subtractive) manufacturing process to automatically detect the defect area and generate a tool path for repairing the defect (Liu et al., 2017). The resolution of the cameras and proper illumination will act as a bottleneck as we aim to characterize surfaces corresponding to different materials in the sub-micron scale.
The principle of structured-light 3D surface imaging technique is to extract the 3D surface shape based on the information from the distortion of the projected structured-light pattern. The distortions of the fringe pattern due to variations in surface height of the sample can be precisely captured by the cameras. SLS utilizes the triangulation principle to calculate the relative positions of the measuring points on the target surface. The use of multiple fringe patterns and the corresponding reconstruction improves the measurement accuracy of this technique without compromising speed compared to other methods, and therefore is suited for in-situ AM applications as shown in Figure 3 (Zhang et al., 2020; Wang et al., 2021). A dual-camera SLS consists of two cameras and a projector. Usually, the projector acts as a bottleneck in the set up with respect to the resolution. However, implementation of phase shifting method and defocusing technique on the multiple fringe patterns can compensate the shortcomings of the projector resolution, as demonstrated by Wang et al. They were able to achieve a spatial resolution measurement of 5 μm with an accuracy of 0.05 μm, by using 6 different fringe patterns (Wang et al., 2021). Zhang et al. employed a custom designed single projector single camera fringe projection system for in situ metrology in Laser Powder Bed Fusion (LBPF) process. They were able to achieve a spatial resolution of 6.8 μm/pixel and a single point repeatability measure of 0.47 μm for measuring the surface topography at each layer using phase shifting and unwrapping algorithms (Zhang et al., 2016). Li et al. proposed a 4D line-scan hyperspectral imager by employing a camera with a spectrograph for a line-scan fringe projection (c) profilometry and achieved a spectral resolution of 2.8 nm along with the spatial root mean square error of 0.0895 nm when measuring a sphere of diameter 40.234 nm (Li et al., 2021a). Harshavardhan et al. proposed a new fringe projection method using polarized light illumination and a polarization camera which could measure moving objects or varying shape objects with high speed (Harshavardhan et al., 2018).
Interferometry captures the depth information of the surface under study by using the interference pattern of two coincident light beams that travel unequal paths. An important characteristic of the interferometric surface topography measurement is that the sensitivity to variations in the measurement is independent of the field of view unlike other optical metrology techniques involving triangulation, fringes or focus effects (De Groot, 2019). However, the surface under consideration needs to be reflective in nature to obtain accurate measurements. The resolution of interferometers is generally in the order of 10 nm in the longitudinal direction and in the order of 1 μm in the lateral direction (Gomez et al., 2020). Optical Coherence Tomography (OCT) is essentially a low coherence interferometer, samples points in the depth direction and constructs a cross sectional image (Yoshizawa, 2009). Spectral-domain optical coherence tomography (SD-OCT) techniques have been reported in literature for monitoring real time in-situ melt pool morphology changes and surface roughness measurements for each layer in LPBF processes (DePond et al., 2018; Kanko et al., 2016). The interference spectrogram, recorded by a 100 kHz Si CMOS camera is then transformed into a map of intensity as a function of distance above the powder bed surface. SD-OCT has been found to permit a long working distance, high sampling rate and provides micrometer scale axial and lateral resolution.
High accuracy optical displacement sensors can be categorized into two types depending on their working principle being laser triangulation and confocal imaging. Laser profilers (or) laser displacement sensors are used to collect height data across a laser line on the surface instead of a single point. A common example would be the Kinect sensor, which uses an Infra-Red (IR) projector to project a pattern of IR dots onto the surface under consideration and the reflected rays are captured by a monochrome CMOS camera placed a few centimeters apart. The depth information is estimated using the principles of triangulation. In the field of additive manufacturing, Barrett et el. employed a high-resolution laser line profilometer to provide a micron level surface map of each layer after being printed in a LPBF system (Barrett et al., 2018b). Wang et al. employed a stereovision camera coupled with a laser displacement sensor for a hybrid manufacturing setup with CNC milling to achieve a spatial resolution of the order of 10 μm (Wang et al., 2015). Confocal sensors use a white light source that is focused onto the target surface by a multi-lens optical system. The objective of the multi-lens system is to disperse the light into monochromatic stages along the measurement axis. A specific distance to the target is assigned to each color’s wavelength in a factory calibration. The distance or surface topography is accurately captured by detecting the colors from the reflected light (Fu et al., 2020). Confocal sensors are used when laser displacement sensors do not make a measurement either due to the type of material used such as one-sided glass or if measurements need to be captured in tiny spaces in the sub-micron scale.
Optical metrology techniques can help real time in-situ monitoring of the workpiece surface morphology evolution and tool condition because of their non-contact nature. Digital cameras involving CCD (charge-coupled device), or CMOS (complementary metal oxide semiconductor) technology have been reported in literature for in-situ monitoring of surfaces during machining operations. All the pixel’s signals are processed by a single circuit or chip in a CCD camera, whereas a CMOS camera has a processing circuit for each pixel which improves speed but reduces the capture area due to increased complexity (Tapia and Elwany, 2014). Challenges associated with the use of CCD or CMOS include the requirement for high frame rate of capture for continuous streaming and post processing of images in order to extract useful information.
With the rapid development of machine vision, image processing and pattern recognition methods, advanced optical solutions are combined with machine learning technologies for surface defect detection and characterization. Iravani et al. set up an optical system for AM comprising of 3 CCD cameras at 120° apart relative orientation over the substrate plane and used a recurrent neural network model to monitor the layer heights using the images from the CCD cameras (Iravani-Tabrizipour and Toyserkani, 2007). Image segmentation methods and deep learning networks including CNN (Convolutional Neural Networks) and ensemble models have been developed on surface images obtained during additive manufacturing and post processing operations for defect detection and roughness estimation (Gobert et al., 2018; Liu et al., 2019; Giusti et al., 2020; Qi et al., 2020).
High speed digital cameras (see Figure 4) have been found suitable for real time in-situ monitoring of melt pool formation, spatter particle tracking and surface porosity in additive manufacturing. The setup of a high-speed camera involves selecting the right camera based on factors like frame rate and resolution. Proper lighting sources are positioned for adequate illumination, especially in high-speed scenarios. A stable mounting system minimizes vibrations, and synchronization with external devices ensures precise timing for capturing fast events. Frame rates in the range of 1,000 fps to 6,000 fps have been reported on multiple occasions in literature to characterize melt pool dynamics and particle behavior (You et al., 2014; Andani et al., 2017; Repossini et al., 2017). Ly et al. used an ultra-high-speed camera with frame rate of 100,000 fps to discover the spatter causing phenomenon of vapor driven entrainment of microparticles by an ambient gas flow (Ly et al., 2017). Matilainen et al. used a high-speed camera with an active illumination system to monitor the laser interaction time and the formation of keyholes during additive manufacturing of stainless steel (Matilainen et al., 2015).
Next, we will delve into the topic of infrared-based monitoring systems. These systems utilize infrared radiation, a type of electromagnetic radiation with wavelengths longer than those of visible light, to capture information about the process or material under study. Infrared-based monitoring systems are commonly used in additive manufacturing for various applications, such as melt pool monitoring, temperature measurement, and defect detection.
To set up an infrared (IR) camera the appropriate camera must be selected based on the desired temperature range and resolution. Calibration ensures accurate temperature measurement, and lens focus and alignment capture clear thermal images. Controlling ambient temperature and reducing reflective surfaces optimizes thermal contrast. Hu and Kovacevic (Hu and Kovacevic, 2003a) developed a closed-loop control system utilizing infrared (IR) imaging sensing to regulate heat input and the size of the melt pool in the Directed Energy Deposition (DED) process. By employing IR imaging, the resulting images were able to eliminate noise caused by metal powder and facilitate real-time processing. The captured IR images provided temperature information, a crucial parameter influencing the quality of the cladding outcome.
The setup of a pyrometer-based melt pool thermal camera (see Figure 5) involves calibrating the camera for accurate temperature measurements, and choosing the appropriate exposure based on the material’s emissivity. The camera is positioned to capture clear thermal images, and optimal lighting conditions and thermal insulation are implemented. Data analysis software enables real-time monitoring and control of the melt pool temperature. Smoqi et al. (Smoqi et al., 2022) implemented a closed-loop control system in their study, utilizing the melt pool temperature as a feedback signal to adjust the laser power in the additive manufacturing process. They employed a two-wavelength pyrometer to provide the necessary feedback. The researchers reported that the volume porosity observed in components manufactured under the closed-loop control system ranged from 0.036% to 0.043%, which was lower than the range of 0.032%–0.068% observed in components manufactured under an open-loop control system.
FIGURE 5. Pyrometer based melt pool camera (Stratonics ThermaViz System) image during a metal directed energy deposition process.
In addition to in-situ monitoring techniques that utilize electromagnetic waves, methods employing mechanical waves, such as laser ultrasonic imaging and spatially resolved acoustic spectroscopy, have emerged as highly effective non-destructive testing (NDT) methods. These methods are adept at detecting and evaluating surface, subsurface, and internal defects.
Laser Ultrasonic (LU) Imaging integrates the principles of laser generation and ultrasonic detection to yield high-resolution images of a material’s interior. This technique has been predominantly used for characterizing the surface geometry and morphology of additively manufactured parts. The selection of Ultrasonic Testing (UT) methods for defect detection and material characterization in additive manufacturing largely depends on the manufacturing process, the material under examination, and the capabilities of UT techniques.
Spatially Resolved Acoustic Spectroscopy (SRAS) is a laser ultrasonic technique that employs Surface Acoustic Waves (SAWs) to investigate the elastic properties of a component as shown in Figure 6 (Smith et al., 2014; Pieris et al., 2019). The SRAS system comprises a pulsed laser generator, a mask to define the acoustic wavelength, a continuous detection laser, and a Knife Edge Detector (KED). The generation laser, in conjunction with the mask, rapidly heats the sample within its thermoelastic regime, generating a SAW with a defined wavelength λ, as determined by the fringe spacing imaged onto the sample surface. The frequency f of the SAW is measured by monitoring the perturbation of the detection laser via the KED. This translates to a measured wave velocity of
A major disadvantage of the knife edge detector is that it does not cope well with optically rough surfaces. The optical speckles from a rough surface adversely affect the efficiency of the knife edge detector, because ‘dark’ speckles move synchronously with ‘bright’ speckles, and their contributions to the ultrasonic signal cancel each other out. The SRAS method has demonstrated significant potential for comprehensive material characterization, enabling the assessment of not only surface defects but also grain orientation and microstructures (Sharples et al., 2006; Hirsch et al., 2017; Hirsch et al., 2018; Patel et al., 2018).
The evaluation of various optical sensing technologies documented in the Table for the purpose of in-situ imaging measurements in additive manufacturing. Although not all imaging methods are discussed, the primary focus is on discussing the most prominent imaging techniques that hold substantial significance concerning high resolution in capturing process physics and their integration with ML/AI methods during model training. The objective is to examine the capabilities and performance of these technologies in a scientific context. These technologies encompass a range of methodologies and instruments that enable the non-destructive evaluation of surface characteristics during the additive manufacturing process.
In the following section, we present various case studies that employ distinct imaging methods, such as high-speed cameras, thermal cameras, and digital cameras, to monitor and analyze diverse anomalies that arise during the deposition and process mechanisms. Additionally, we explore ex-situ imaging methods that integrate advanced machine learning (ML) and artificial intelligence (AI) algorithms to enhance defect detection.
A practical example highlighting the contribution of in-situ imaging in smart manufacturing is the implementation of a smart hybrid machine tool (Optomec MTS 500), at Texas A&M University (Botcha et al., 2020). This machine tool incorporates in-situ imaging sensors along with other sensors, enabling the collection of data from 14 different channels. Notably, the machine is equipped with a high-speed camera capable of capturing videos at high frame rates, facilitating the observation and analysis of various process mechanisms. These mechanisms include spatter events, the emergence of powder from nozzles, and the trajectory of chips during milling operations. In an additive-subtractive process lasting 1 hour, the high-speed camera (built: Photron Mini AX200) collects approximately 100–200 GB of data at a frame rate of 5000 FPS. Usually, this involves speed cameras are used for capturing selective segments of the process that involve process mechanisms that occur at sub-second scales. For the 1-h process, around three to four instances of such segments can be captured. High-speed cameras capture processes with exceptional temporal resolution, enabling the collection of detailed visual information.
A thermal camera (Stratonics ThermaViz System) is installed coaxially to the laser assembly, measuring temperature distributions and thermal signatures of the melt pool. The thermal camera captures data at different frame rates, typically ranging from 30 to 60 Hz. For a 1-h process, it collects around 60–120 GB of data. Other imaging systems such as optical cameras in a modern smartphone can record the entire process and generate around 20–25 GB of data if recorded at resolution of 4K at 60 FPS. These imaging devices generate big data (∼200–250 GB per hour) that can be processed and analyzed using advanced analytics and machine learning algorithms.
The installation of high-resolution cameras in manufacturing machines alone does not suffice to address the challenges effectively. It is crucial to establish an appropriate data collection pipeline to ensure the value and usefulness of the collected data for subsequent analysis. Implementing a well-designed data collection pipeline is paramount as it ensures that the acquired data adheres to appropriate protocols, preventing it from becoming inconsequential for analysis purposes (Wang et al., 2022). By collecting data with a well-defined protocol, researchers can ensure data integrity and accuracy. At Texas A&M University, a meticulous data pipeline is implemented to capture data from multiple sensors in real-time (see Figure 7), which is then stored both locally and on cloud-based storages. This dual storage approach allows for real-time monitoring of the cloud-based data and facilitates the transfer of data to other hosts for further analysis. The collected data is subject to analysis through data processing algorithms, providing opportunities to uncover hidden patterns, correlations, and insights that contribute to process optimization, quality improvement, and enhanced manufacturing performance.
FIGURE 7. Data Collection Strategy Implemented at Texas A&M University. The Optomec MTS 500 manufacturing system is integrated with multiple optical sensors for comprehensive data collection. The recorded data is stored both locally and on cloud-based platforms, enabling efficient data management. Real-time monitoring and analysis are conducted to extract valuable insights from the collected data.
In this section, we explore various case studies that pertain to the application of imaging techniques within the space of smart additive manufacturing. These imaging methods are employed to detect and identify different types of defects that may occur during manufacturing processes. Specifically, we focus on the utilization of optical sensing and intensity-based sensing methods for defect monitoring. Liu et al. (Liu et al., 2021) conducted a study wherein they investigated specific defects occurring in the context of DED. These defects can arise due to a combination of multiple factors. Here we discuss two primary categories of anomalies in DED process: process faults and product defect monitoring.
Process faults comprise irregularities that transpire during the deposition process itself, such as spatter events, laser focus issues (discussed in Section 3.1), and other similar occurrences. These faults can have a detrimental impact on the overall quality and integrity of the manufactured product. This detrimental impact implies negative effects or consequences that these process faults can introduce. They can result in reduced quality where the product does not meet the required standards or specifications, compromised integrity where the structural strength of the product is weakened leading to potential failure under stress. On the other hand, product defect monitoring relates to the identification and tracking of various irregularities in the final product. This includes dimensional variations, surface waviness, morphological variations, and the detection of porosity. Monitoring these defects is crucial as they can significantly impact the functional properties and performance of the manufactured parts.
To address these challenges, optical sensing and intensity-based sensing methods have proven to be effective tools. Optical sensing techniques utilize light-based systems to capture and analyze data related to the manufacturing process. These methods enable real-time monitoring and analysis of process faults and product defects. In addition to the utilization of imaging techniques, the integration of Machine Learning (ML) and Artificial Intelligence (AI) algorithms plays a crucial role in extracting hidden information and patterns from the captured images. ML and AI techniques enable advanced analysis and interpretation of the imaging data, leading to enhanced defect detection and monitoring in smart manufacturing.
By employing ML/AI algorithms, we can train models to recognize and classify different types of defects based on the patterns and features extracted from the images. These algorithms learn from a large dataset of labeled images, allowing them to identify subtle variations and anomalies that may not be easily discernible to the human eye (discussed in Section 3.2). This capability enables automated and accurate defect detection, minimizing the reliance on manual inspection processes that can be time-consuming and prone to human error.
In-situ monitoring plays a crucial role in the additive manufacturing process as it enables constant supervision of the process physics and facilitates the detection of anomaly events occurring during the process. The selection of accurate process parameters is paramount to avoid various defects such as porosity, dimensional inaccuracies, and surface waviness. These defects tend to have a multiplier effect, meaning that when a defect arises, it propagates through subsequent layers if not corrected. Therefore, it becomes imperative to identify the origin of defects as soon as they occur and implement corrective measures to mitigate their presence. In this context, high-speed cameras can be employed to monitor multiple process mechanisms, specifically in observing the laser focus during the printing process, spatter events, powder particle trajectory, and tracking chips mechanism in milling operation.
High-speed cameras can capture rapid events with high temporal resolution, enabling the monitoring of the laser focus during the additive manufacturing process. By recording the laser’s behavior in real-time, these cameras provide valuable insights into the dynamics of the laser beam and its interaction with the printing material. Monitoring the laser focus using high-speed cameras involves capturing detailed images or videos of the laser spot during the printing process. These cameras can be synchronized with the printing system to capture images at specific time intervals or triggered by specific process events.
Through the analysis of high-speed camera footage, it becomes possible to assess the laser’s focal position and evaluate its stability and consistency. Any deviations or fluctuations in the laser focus can be detected and correlated with potential defects observed in the printed part. Furthermore, the use of image processing techniques and algorithms can provide quantitative measurements of the laser spot size, shape, and intensity distribution, aiding in the identification of potential issues related to the laser focus.
Figure 8, shows a series of images obtained through the utilization of a high-speed camera during the additive manufacturing printing process. Figure 8A effectively demonstrates the laser focus at the printing track, specifically emphasizing the amalgamation of powder streams within the melt pool on the deposition track. The significance of laser focus in determining surface quality becomes apparent upon observation of these images. When the laser is appropriately focused, consecutive tracks are laid down without any observable irregularities. Conversely, when the laser deviates from its optimal focus (see Figure 8B), it becomes unfeasible to achieve a level layer. This phenomenon becomes increasingly conspicuous as the number of layers accumulates, ultimately leading to undulations in the surface. Consequently, the continuous monitoring of laser focus throughout the printing process assumes paramount importance in upholding print quality and attaining the desired surface characteristics.
FIGURE 8. High-speed camera captures the laser-material interaction: (A) Powder particles emerging from the nozzles meet the laser at the focal point on the depositing layer, resulting in precise build dimensions and high surface quality. (B) Powder particles interacting with the laser above the depositing layer depicting a shift in the laser focus, which leads to surface waviness.
Another essential capability offered by high-speed cameras is their ability to accurately detect and analyze spatter events. These spatters can have a detrimental effect on the overall quality of the built part, potentially leading to the occurrence of various defects. By employing advanced filtering techniques such as the Kalman filter, it becomes possible to effectively track and quantify the spatters in real-time as they are expelled from the melt pool.
In a study conducted by Iquebal et al. (Iquebal et al., 2022), it was observed that a substantial proportion of the powder material (approximately 89%) is wasted even before it enters the melt pool. Consequently, only around 11% of the powder particles successfully reaches the melt pool. Within this deposition zone, approximately 12% of the material is ejected through spattering phenomena. These spatter events involve the expulsion of molten droplets or particles from the melt pool, which can negatively impact the integrity and quality of the final build. The detection and quantification of spatters in real-time using high-speed cameras (see Figure 9), in conjunction with advanced filters like the Kalman filter, provide valuable insights into the spattering phenomenon, enabling the development of strategies to minimize its occurrence and mitigate its detrimental effects on the built part.
FIGURE 9. Melt pool formation at the substrate captured using high speed camera. Spatter particles ejecting from the melt pool tracked using Kalman filter.
The utilization of pyrometer based thermal cameras in monitoring the behavior of the melt pool during the additive manufacturing printing process is witnessing a growing trend. The melt pool is a crucial phenomenon to monitor as it offers opportunities for achieving tailored properties in the manufactured components by tuning the laser power in real time. The implementation of thermal cameras enables the acquisition of comprehensive insights into the characteristics of the melt pool. Through the analysis of real-time data obtained from the thermal camera, including parameters such as peak temperature, melt pool geometry, and temperature distribution, it becomes possible to establish a closed-loop control system. By comparing the observed parameters with the desired values, adjustments can be made to the process parameters in real-time, such as adjusting the laser power. This closed-loop control mechanism ensures that the melt pool remains within the desired temperature range and exhibits the intended geometry, leading to the desired properties in the printed component.
Furthermore, the detailed insights provided by thermal cameras allow for a deeper understanding of the melt pool behavior. By studying the temperature distribution and variations as shown in Figure 10, it becomes possible to optimize process parameters and identify potential issues that may impact the quality of the printed part. By utilizing this information, adjustments can be made to mitigate defects such as porosity, incomplete melting, or excessive heat accumulation, which can negatively impact the properties and structural integrity of the final component.
FIGURE 10. Melt pool geometry, with major axis and minor dimensions calculated using melt pool frames captured by the thermal camera. Additionally, the temperature distribution curve along the x-y direction exhibits a smooth profile, indicating uniform heat distribution within the melt pool and ensuring the absence of anomalies during the deposition process.
The incorporation of the Stratonics Therma Viz, installed coaxially in the Optomec MTS 500 system, enables real-time monitoring and analysis of the melt pool during the deposition process. Through the utilization of advanced algorithms, various mechanisms can be understood and quantified, leading to enhanced process control and quality assurance.
1. Melt Pool Geometry: The precise measurement and characterization of the melt pool geometry are crucial for evaluating the stability and behavior of the deposition process. By capturing and analyzing real-time melt pool images, the circularity of the pool, as well as dimensions such as major and minor axis lengths, can be quantified.
2. Temperature Distribution: This information is critical for assessing the thermal profile and heat dissipation characteristics during the deposition process. Real-time monitoring of temperature distribution aids in identifying potential temperature variations or gradients that may affect the material’s solidification behavior, resulting in defects such as porosity, cracking, or incomplete fusion.
3. Thermal Gradients: The algorithms integrated with the thermal camera enable the calculation of thermal gradients within the melt pool as shown in Figure 11. Thermal gradients represent the rate of temperature change across the material. Monitoring these gradients in real-time provides valuable information on heat transfer phenomena, including cooling rates and heat dissipation patterns. Ripple phenomena, observed in DED processes, are often influenced by thermal gradients, alloying effects, and recoil pressure. By quantifying thermal gradients, the dynamics of ripple formation (Balhara et al., 2023) and its correlation with process parameters can be analyzed, contributing to improved process optimization and defect detection.
FIGURE 11. A custom MATLAB algorithm used to calculate the thermal gradients from the raw melt pool frame. Quiver plots depict the temperature decrease from the edge of the melt pool towards its center, representing a crucial step in the formation of ripples DED process.
Optical cameras have provided an excellent means of in-situ monitoring due to their convenience, affordability, and accessibility for a long time. The advancement in the smartphone industry has made it even more convenient given the instrumentation of these modern smartphones with high-tech sophisticated optical cameras with easy-to-use interfaces and the form factor. Smartphones can capture high resolution videos or images during manufacturing giving a great overall picture of the process. This translates to a foundation for operators to visually inspect the process, identify any artifacts of interest, and monitor several metrics associated with the process.
By using appropriate filters or image post-processing techniques, smartphones are even capable of capturing the thermal history of the process. This thermal monitoring allows for the identification of abnormal heating or cooling patterns in the process. By strategically positioning the smartphone camera, operators can capture close-up images and videos of the process. Moreover, there are a lot of computer vision techniques that can be used to further analyze the images or videos, opening a possibility for analyses such as object detection and tracking. In-situ monitoring using smartphone cameras (see Figure 12) can also allow for process optimization by extracting valuable data in the form of adjustable and refinable process parameters for the operators. Over time, this iterative feedback assists in improving the overall process in terms of quality and efficiency.
FIGURE 12. A smartphone digital camera recording (A) laser material deposition during the printing cycle, (B) milling operation carried out after printing in Hybrid DED process.
Defects in manufacturing manifest in varied forms and attributes which add to the woes of developing one-shot detection methodologies, while it is also expensive to generate a dataset of images capturing the variety to train these models. Therefore, many image processing techniques have been implemented that specialize in detecting certain defects due to the surface’s nature. Indeed, while in-situ imaging techniques offer numerous advantages, post-process imaging techniques are equally, and sometimes more, important for analyzing specific properties of manufactured components. Advanced imaging methods, such as 3D optical profilometry, Scanning Electron Microscopy (SEM), and Energy Dispersive Spectroscopy (EDS), play a significant role in providing high-resolution images suitable for detailed analysis.
Post-process imaging techniques enable the examination of manufactured components at a higher level of magnification and resolution, facilitating the inspection of fine details and surface characteristics. 3D optical profilometry, for instance, allows for the precise measurement of surface profiles, enabling the evaluation of dimensional accuracy, surface roughness, and features at a microscale level. Scanning Electron Microscopy (SEM) provides exceptional imaging capabilities with high-resolution and magnification. It allows for the observation of surface morphology, microstructure, and topographical features at nanoscale levels (see Figure 13A). SEM coupled with EDS provides valuable elemental analysis, enabling the identification and mapping of different chemical elements present in the sample.
FIGURE 13. (A) SEM micrographs showing the formation of cellular structure in the DED printed 316L components, (B) 3D optical profilometer capturing the surface morphology (ripples) and their Ra variation along the scan track, (C) optical micrograph showing voids and pores spread across the scan surface of DED-printed component (D,E) surface waviness captured using 3D optical profilometer, (F) artifacts captured using ultrasound imaging.
Recent advancements in machine learning and artificial intelligence have further enhanced post-process imaging techniques. These technologies enable defect localization and analysis based on imaging data. Machine learning algorithms can be trained to detect and classify various types of defects, aiding in quality control and process optimization.
The utilization of big data in post-process imaging techniques offers significant contributions to the field. It enables efficient collection, storage, and management of large-scale imaging datasets, while advanced analytics techniques facilitate automated feature extraction, defect detection, and classification. Integration with other data sources allows for a comprehensive analysis of the manufacturing process, while predictive analytics models based on historical imaging data assist in forecasting potential defects. Real-time monitoring and feedback are facilitated through the streaming and processing of data, enabling timely intervention and corrective actions. Furthermore, big data technologies provide tools for data visualization and reporting, aiding in the interpretation and communication of findings.
Pores and voids in DED processes exhibit a stochastic formation pattern as they emerge from multiple physical mechanisms over multiple sizes (ranging 10−5–10−3 m), and at diverse locations including the surface, sub-surface or between deposited layers (see Figure 13C). Digital images and optical micrographs can be used to characterize surface porosity that manifest as a result of various mechanisms such as (a) micro-void formations caused due to insufficient overlap and fusion between adjacent printing tracks, and (b) pore formation due to the spatter events during the DED process.
In the past decade, deep learning models have been widely used in the manufacturing sector for real-time process monitoring and anomaly detection, leveraging multimodal sensor data. However, the complex, non-linear nature of these models often results in a lack of transparency, making it difficult to interpret their predictions and thereby reducing trust in the model. In response to this challenge, there has been a significant shift towards the use of Explainable AI (XAI) techniques, which aim to increase the interpretability and transparency of AI models. XAI techniques along with thermal images of the melt pool proved to better understand the laser-material interactions and discover the nature of spatter events that result in the occurrence of porosity in localized surface elements (Karthikeyan et al., 2023). Recently, Karthikeyan et al. (Karthikeyan et al., 2022) developed an approach that integrates explainable AI (XAI) into ultrasound imaging for rapid detection of artifacts, including product defects, in 3D printed components. Leveraging ultrasound imaging offers a non-destructive means of inspecting the internal structure of 3D printed parts, particularly useful for identifying defects that are not visible on the surface. The incorporation of XAI into this process serves to validate AI-driven decisions, providing decision-makers with an enhanced transparency into the discernible features within the images that contribute to defect classification.
Although advanced machine learning techniques have been employed to analyze manufacturing data and enhance production strategies, the challenge of labeling data for supervised learning methods remains a significant obstacle. Defects in manufacturing exhibit diverse forms and characteristics, complicating the development of one-shot detection methodologies. Furthermore, the generation of an extensive dataset encompassing the various defect types for model training purposes can be prohibitively costly. Consequently, numerous specialized image processing techniques have been implemented to address this issue, focusing on the detection of specific defects based on the surface properties.
With the emergence of Manufacturing-as-a-Service (MaaS) paradigm, it becomes imperative to secure essential products against counterfeiting and cyber-attacks (Tiwari et al., 2021). Anticounterfeit tags, such as QR codes introduced on the surface of physical products can be tampered with, thus destroying the ability to identify these parts. Hence, in order to introduce anticounterfeit tags inside the physical product, the 3D printed parts were embedded with spherical markers of diameter 0.48 mm (see Figure 13F). The markers were dispersed at both shallow (1–3 mm depth) and deeper (9–12 mm depth) locations, emulating the occurrence of defects (e.g., internal pores and voids) and embedded authentication codes in a product. The authors employed a fast-scanning ultrasound imaging technique coupled with a convolutional deep learning software framework to obtain speed and accuracy in scanning the internal markers present inside 3D printed parts. They were able to accurately detect the internal markers at different locations within the product to accuracies exceeding 80%.
In Iquebal et al. (Iquebal and Bukkapatnam, 2022), the authors propose a fully unsupervised segmentation approach using a continuous max-flow formulation over the image domain while optimally estimating the flow parameters from the image characteristics. Image segmentation approaches based on the energy minimization framework, particularly graph-based methods, offer an elegant means to segment images without extensive training. The framework is based on iteratively estimating the image labels by solving the maxflow problem while optimally estimating the flow capacities from the image characteristics. The segmentation problem is set up as a maximum posteriori estimation (MAP) of the image labels and shows that it is equivalent to solving the max-flow problem given the flow capacities are known. By using the current optimum of the max-flow problem, the flow capacities are estimated by employing a Markov random field (MRF) prior over the flow capacities. For a detailed understanding of the framework and algorithm, we direct the readers to their paper (Iquebal and Bukkapatnam, 2022).
The current case study presents the segmentation capabilities of the above-mentioned framework for common defects on additively manufactured surfaces, namely, balling effect, and porosity. Figure 14A and Figure 15A show the representative surface from two samples that were additively manufactured. Figures 14B–F, Figures 15B–F give the comparative results from different segmentation algorithms, namely-means with 2 clusters, Gaussian mixture model with expectation maximization, spatially constrained Gaussian mixture model with k-means initialization (Nguyen and Wu, 2013), mean shift (Comaniciu and Meer, 2002), and Unsupervised min cut (Iquebal and Bukkapatnam, 2022).
FIGURE 14. Comparative results of different algorithms tested for the segmentation of defects. (A) Original image for sample A (B) k-means with 2 clusters (C) Gaussian mixture model with expectation maximization (D) spatially constrained Gaussian mixture model with k-means initialization and (E) mean shift (F) proposed method (Iquebal and Bukkapatnam, 2022).
FIGURE 15. Comparative results of different algorithms tested for the segmentation of defects. (A) Original image for sample B (B) k-means with 2 clusters (C) Gaussian mixture model with expectation maximization (D) spatially constrained Gaussian mixture model with k-means initialization and (E) mean shift (F) proposed method (Iquebal and Bukkapatnam, 2022).
Numerous studies have proposed various techniques in image processing, machine learning, and neural network models to address defect detection and segmentation in specific defect domains. However, the diverse nature and characteristics of defects in manufacturing pose challenges in developing comprehensive one-shot detection methods. Furthermore, creating a dataset encompassing the wide range of defect variations is costly when training a one-shot machine learning model. Nakkina et al. (Nakkina et al., 2022) introduces a framework that comprises three mind-maps aimed at capturing the fundamental aspects of defect detection.
The first mind-map proposes a classification system for manufacturing defects based on their visual attributes. The objective of the second mind-map is to identify relevant image processing methodologies including thresholding, Fourier analysis, line detection, neural networks, and more. The third mapping aims to establish connections between specific defect classes and corresponding image processing techniques. These three mind-maps collectively serve as a foundation for developing or adapting defect detection approaches tailored to specific use cases. Additionally, the authors introduce an empirical recommendation formula utilizing three image metrics: entropy, universal Quality Index (UQI), and Rosenberger’s. This formula facilitates the evaluation of method performance across a given class of images. Nakkina et al. (Nakkina et al., 2022) showcases the implementation of a Smart Defect Segmentation Toolbox assimilating methodologies like Wavelet Analysis, Morphological Component Analysis (MCA), Basic Line Detector (BLD) and presents case studies to support the working of the recommendation formula.
The MVTecAD dataset identifies industrial inspection tasks as the ideal scenarios introducing a comprehensive dataset for unsupervised anomaly detection (Bergmann et al., 2019). Figure 16 tabulates the findings of the toolbox when tested on three sample images from a wooden, metal nut, and tile categories from the MVTecAD dataset respectively. The recommendations based on the formula given by the toolbox for defect detection in each of these cases are also mentioned.
FIGURE 16. Outputs from the Toolbox for sample images from (A) Wooden (B) Metal nut and (C) Tile categories from the MVTecAD dataset (Nakkina et al., 2022).
This chapter delves into the rapidly increasing significance of advanced imaging techniques, specifically in the domain of fusion-based additive manufacturing. Optical cameras, thermal cameras, and via high-speed cameras can enable real-time monitoring, facilitating prompt defect detection, deviations from standard operating procedures, or any anomalous behavior. Thereby, timely intervention is possible which can lead to improved product quality, reduction in downtime, and improved uptime and productivity. Such monitoring also allows for a deeper understanding of the underlying physics involved in the manufacturing process, offering neat opportunities for process optimization. Key takeaways from the chapter are listed as follows.
• High-speed imaging demonstrates its efficacy in capturing spatter particles expelled from the melt pool during the additive manufacturing process. The real-time detection and quantification of spatters using high-speed cameras, combined with advanced filtering techniques like the Kalman filter, offer valuable insights into the spattering phenomenon. This information can then be leveraged to implement closed-loop control strategies within the manufacturing system. Furthermore, the high-speed imaging technique proves effective in identifying deviations in the laser focus, such as when it moves away from the intended printing track. This timely identification enables prompt optimization of process parameters to mitigate defects such as dimensional inaccuracies and surface waviness.
• The thermal camera exhibits its capability to monitor key characteristics of the melt pool, including peak temperature and melt pool geometry during additive manufacturing processes. Deviations from the standard characteristics serve as indicative signatures for optimizing process parameters. Real-time analysis of thermal data allows for the assessment of heat distribution and thermal gradients within the melt pool, providing insights for the implementation of closed-loop control strategies. By adjusting the laser power in real-time based on the observed thermal information, tailored properties of the components can be achieved.
• Digital cameras integrated into smartphones offer a practical solution for recording the complete manufacturing process. The captured data from these cameras can subsequently be utilized to synchronize and align the multi-modal sensor data collected during the process. Leveraging the capabilities of smartphone cameras in this manner provides a cost-effective and accessible means to enhance data fusion and analysis in smart manufacturing.
• Ex-situ imaging methods, when combined with the integration of machine learning/artificial intelligence (ML/AI) techniques, offer the opportunity to analyze data with enhanced depth and sophistication. The high-resolution images captured via ex-situ imaging systems serve as excellent inputs to state-of-the-art deep learning models for a variety of applications.
Given the remarkable progress and use of advanced imaging technology in smart manufacturing, there is potential for future research work in several avenues. One such area involves integration of the imaging data with advanced ML/AI methodologies. Another aspect lies in the use of multimodal nature of data. Given the plethora of imaging options, most manufacturers employ more than one at the same time to monitor their process. Multimodal data fusion that incorporates diverse types of image-based data is a blooming area of research, especially in healthcare and wearable devices industry. By fusing data from optical, thermal, high-speed, and other types of cameras with ex-situ imaging, researchers can extract synergistic information to have a more holistic understanding of the process. Another challenge lies in the fact that the data resulting from such advanced imaging techniques is usually large and can sometimes slow down real-time processing and analyses. Developing data pipelines that provide capabilities for real-time processing of such data without any latency issues or lag is essential for immediate decision-making in such scenarios. To further optimize the processes, creation of seamless and data-driven ecosystem comprised of integrated imaging technologies and IoT sensors is preferred. A common disadvantage that is being tackled is the heavy cost expense that comes from some of these imaging systems. Developing cost-effective yet reliable imaging technologies will lead to widespread adoption in the industry, especially for small- and medium-sized enterprises. By lowering the entry barriers and easy-to-use interfaces of such imaging systems will democratize access to advanced capabilities, empowering a broader range of industries.
The original contributions presented in the study are included in the article/Supplementary material, further inquiries can be directed to the corresponding author.
HB: Data curation, Formal Analysis, Investigation, Methodology, Writing–original draft. AK: Data curation, Formal Analysis, Writing–review and editing. AH: Data curation, Formal Analysis, Writing–review and editing. TN: Data curation, Formal Analysis, Writing–review and editing. SB: Conceptualization, Funding acquisition, Project administration, Resources, Supervision, Writing–review and editing.
The author(s) declare financial support was received for the research, authorship, and/or publication of this article. This work was supported by the Rockwell International Professorship, Texas A&M University XGrants and National Science Foundation (S&AS grant award #1849085 and ECCS 1953694).
The authors declare that the research was conducted in the absence of any commercial or financial relationships that could be construed as a potential conflict of interest.
All claims expressed in this article are solely those of the authors and do not necessarily represent those of their affiliated organizations, or those of the publisher, the editors and the reviewers. Any product that may be evaluated in this article, or claim that may be made by its manufacturer, is not guaranteed or endorsed by the publisher.
Abhilash, P. M., and Ahmed, A. (2023). An image-processing approach for polishing metal additive manufactured components to improve the dimensional accuracy and surface integrity. Int. J. Adv. Manuf. Technol. 125 (7), 3363–3383. doi:10.1007/s00170-023-10916-1
Akbari, M., and Kovacevic, R. (2019). Closed loop control of melt pool width in robotized laser powder–directed energy deposition process. Int. J. Adv. Manuf. Technol. 104 (5–8), 2887–2898. doi:10.1007/s00170-019-04195-y
Ali, N., Tomesani, L., Ascari, A., and Fortunato, A. (2022). Fabrication of thin walls with and without close loop control as a function of scan Strategy via direct energy deposition. Lasers Manuf. Mater. Process. 9 (1), 81–101. doi:10.1007/s40516-022-00164-8
Allam, A., Alfahmi, O., Patel, H., Sugino, C., Harding, M., Ruzzene, M., et al. (2022). Ultrasonic testing of thick and thin Inconel 625 alloys manufactured by laser powder bed fusion. Ultrasonics 125, 106780. doi:10.1016/j.ultras.2022.106780
Andani, M. T., Dehghani, R., Karamooz-Ravari, M. R., Mirzaeifar, R., and Ni, J. (2017). Spatter formation in selective laser melting process using multi-laser technology. Mater. Des. 131, 460–469. doi:10.1016/j.matdes.2017.06.040
Arnold, C., and Körner, C. (2021). Electron-optical in-situ metrology for electron beam powder bed fusion: calibration and validation. Meas. Sci. Technol. 33 (1), 014001. doi:10.1088/1361-6501/ac2d5c
Bakre, C., Meyer, T., Jamieson, C., Nassar, A. R., Reutzel, E. W., and Lissenden, C. J. (2022). In-situ laser ultrasound-based Rayleigh wave process monitoring of DED-AM metals. Res. Nondestruct. Eval. 33 (4–5), 218–242. doi:10.1080/09349847.2022.2120652
Balhara, H., Botcha, B., Wolff, S. J., and Bukkapatnam, S. T. S. (2023). Ripple formations determine the heterogeneous microstructure of directed energy deposition (DED)-printed 316L components. Mater. Des. 227, 111756. doi:10.1016/j.matdes.2023.111756
Barrett, C., Carradero, C., Harris, E., Rogers, K., MacDonald, E., and Conner, B. (2019). Statistical analysis of spatter velocity with high-speed stereovision in laser powder bed fusion. Prog. Addit. Manuf. 4 (4), 423–430. doi:10.1007/s40964-019-00094-6
Barrett, C., Carradero, C., Harris, E., McKnight, J., Walker, J., MacDonald, E., et al. (2018a). “Low cost, high speed stereovision for spatter tracking in laser powder bed fusion,” in 2018 International Solid Freeform Fabrication Symposium (Austin: University of Texas at Austin).
Barrett, C., MacDonald, E., Conner, B., and Persi, F. (2018b). Micron-level layer-wise surface profilometry to detect porosity defects in powder bed fusion of Inconel 718. JOM 70 (9), 1844–1852. doi:10.1007/s11837-018-3025-7
Becker, D., Boley, S., Eisseler, R., Stehle, T., Möhring, H. C., Onuseit, V., et al. (2021). Influence of a closed-loop controlled laser metal wire deposition process of S Al 5356 on the quality of manufactured parts before and after subsequent machining. Prod. Eng. Res. Devel. 15 (3–4), 489–507. doi:10.1007/s11740-021-01030-w
Bergmann, P., Fauser, M., Sattlegger, D., and Steger, C. (2019). “MVTec ad -- A comprehensive real-world dataset for unsupervised anomaly detection,” in presented at the Proceedings of the IEEE/CVF Conference on Computer Vision and Pattern Recognition, 9592–9600. Accessed: Jul. 14, 2023.
Bernauer, C., Zapata, A., and Zaeh, M. F. (2022a). Toward defect-free components in laser metal deposition with coaxial wire feeding through closed-loop control of the melt pool temperature. J. Laser Appl. 34 (4), 042044. doi:10.2351/7.0000773
Bernauer, C. J., Zapata, A., Kick, L., Weiss, T., Sigl, M. E., and Zaeh, M. F. (2022b). Pyrometry-based closed-loop control of the melt pool temperature in Laser Metal Deposition with coaxial wire feeding. Procedia CIRP 111, 296–301. doi:10.1016/j.procir.2022.08.025
Bidare, P., Maier, R. R. J., Beck, R. J., Shephard, J. D., and Moore, A. J. (2017). An open-architecture metal powder bed fusion system for in-situ process measurements. Addit. Manuf. 16, 177–185. doi:10.1016/j.addma.2017.06.007
Black, D., Henderson, J., Klocke, P., Shumway, L., and Crane, N. B. (2023). Dark field optical observation of polymer powder bed fusion for process monitoring and control. Addit. Manuf. 74, 103715. doi:10.1016/j.addma.2023.103715
Boschetto, A., Bottini, L., and Vatanparast, S. (2023). Powder bed monitoring via digital image analysis in additive manufacturing. J. Intell. Manuf. doi:10.1007/s10845-023-02091-7
Boschetto, A., Bottini, L., Vatanparast, S., and Veniali, F. (2022). Part defects identification in selective laser melting via digital image processing of powder bed anomalies. Prod. Eng. Res. Devel. 16 (5), 691–704. doi:10.1007/s11740-022-01112-3
Botcha, B., Iquebal, A. S., and Bukkapatnam, S. T. S. (2020). Smart manufacturing multiplex. Manuf. Lett. 25, 102–106. doi:10.1016/j.mfglet.2020.08.004
Bugatti, M., and Colosimo, B. M. (2022). Towards real-time in-situ monitoring of hot-spot defects in L-PBF: a new classification-based method for fast video-imaging data analysis. J. Intell. Manuf. 33 (1), 293–309. doi:10.1007/s10845-021-01787-y
Chabot, A., Laroche, N., Carcreff, E., Rauch, M., and Hascoët, J.-Y. (2020). Towards defect monitoring for metallic additive manufacturing components using phased array ultrasonic testing. J. Intell. Manuf. 31 (5), 1191–1201. doi:10.1007/s10845-019-01505-9
Chebil, G., Bettebghor, D., Renollet, Y., Lapouge, P., Davoine, C., Thomas, M., et al. (2023). Deep learning object detection for optical monitoring of spatters in L-PBF. J. Mater. Process. Technol. 319, 118063. doi:10.1016/j.jmatprotec.2023.118063
Chechik, L., Goodall, A. D., Christofidou, K. A., and Todd, I. (2023). Controlling grain structure in metallic additive manufacturing using a versatile, inexpensive process control system. Sci. Rep. 13 (1), 10003. doi:10.1038/s41598-023-37089-x
Chen, L., Yao, X., Chew, Y., Weng, F., Moon, S. K., and Bi, G. (2020). Data-driven adaptive control for laser-based additive manufacturing with automatic controller tuning. Appl. Sci. 10 (22), 7967. doi:10.3390/app10227967
Cherif, L., Safdar, M., Lamouche, G., Wanjara, P., Paul, P., Wood, G., et al. (2023). Evaluation of key spatiotemporal learners for print track anomaly classification using melt pool image streams. doi:10.48550/arXiv.2308.14861
Comaniciu, D., and Meer, P. (2002). Mean shift: a robust approach toward feature space analysis. IEEE Trans. Pattern Analysis Mach. Intell. 24 (5), 603–619. doi:10.1109/34.1000236
D’Accardi, E., Chiappini, F., Giannasi, A., Guerrini, M., Maggiani, G., Palumbo, D., et al. (2023). Online monitoring of direct laser metal deposition process by means of infrared thermography. Prog. Addit. Manuf. doi:10.1007/s40964-023-00496-7
Davis, G., Nagarajah, R., Palanisamy, S., Rashid, R. A. R., Rajagopal, P., and Balasubramaniam, K. (2019). Laser ultrasonic inspection of additive manufactured components. Int. J. Adv. Manuf. Technol. 102 (5), 2571–2579. doi:10.1007/s00170-018-3046-y
De Groot, P. J. (2019). A review of selected topics in interferometric optical metrology. Rep. Prog. Phys. 82 (5), 056101. doi:10.1088/1361-6633/ab092d
Delacroix, T., Lomello, F., Schuster, F., Maskrot, H., Jacquier, V., Lapouge, P., et al. (2023). Measurement of powder bed oxygen content by image analysis in laser powder bed fusion. Mater. Des. 226, 111667. doi:10.1016/j.matdes.2023.111667
DePond, P. J., Guss, G., Ly, S., Calta, N. P., Deane, D., Khairallah, S., et al. (2018). In situ measurements of layer roughness during laser powder bed fusion additive manufacturing using low coherence scanning interferometry. Mater. Des. 154, 347–359. doi:10.1016/j.matdes.2018.05.050
el Farsy, A., Tighidet, E. C., Ballage, C., and Minea, T. (2023). Spatiotemporal characterization of evaporated atoms during electron beam melting additive manufacturing by advanced laser diagnostics. J. Appl. Phys. 133 (4), 044901. doi:10.1063/5.0131102
Eschner, E., Staudt, T., and Schmidt, M. (2020). Correlation of spatter behavior and process zone formation in powder bed fusion of metals. CIRP Ann. 69 (1), 209–212. doi:10.1016/j.cirp.2020.04.092
Farshidianfar, M. H., Khajepour, A., and Gerlich, A. (2016). Real-time control of microstructure in laser additive manufacturing. Int. J. Adv. Manuf. Technol. 82 (5–8), 1173–1186. doi:10.1007/s00170-015-7423-5
Farshidianfar, M. H., Khodabakhshi, F., Khajepour, A., and Gerlich, A. P. (2021a). Closed-loop control of microstructure and mechanical properties in additive manufacturing by directed energy deposition. Mater. Sci. Eng. A 803, 140483. doi:10.1016/j.msea.2020.140483
Farshidianfar, M. H., Khodabakhshi, F., Khajepour, A., and Gerlich, A. P. (2021b). Closed-loop deposition of martensitic stainless steel during laser additive manufacturing to control microstructure and mechanical properties. Opt. Lasers Eng. 145, 106680. doi:10.1016/j.optlaseng.2021.106680
Fischer, F. G., Birk, N., Rooney, L., Jauer, L., and Schleifenbaum, J. H. (2021). Optical process monitoring in Laser Powder Bed Fusion using a recoater-based line camera. Addit. Manuf. 47, 102218. doi:10.1016/j.addma.2021.102218
Freeman, F., Chechik, L., Thomas, B., and Todd, I. (2023). Calibrated closed-loop control to reduce the effect of geometry on mechanical behaviour in directed energy deposition. J. Mater. Process. Technol. 311, 117823. doi:10.1016/j.jmatprotec.2022.117823
Fu, S., Kor, W. S., Cheng, F., and Seah, L. K. (2020). In-situ measurement of surface roughness using chromatic confocal sensor. Procedia CIRP 94, 780–784. doi:10.1016/j.procir.2020.09.133
Gardfjell, M., Reith, M., Franke, M., and Körner, C. (2023). In situ inclusion detection and material characterization in an electron beam powder bed fusion process using electron optical imaging. Materials 16 (12), 4220. Art. no. 12. doi:10.3390/ma16124220
Gibson, B. T., Bandari, Y., Richardson, B., Henry, W., Vetland, E., Sundermann, T., et al. (2020). Melt pool size control through multiple closed-loop modalities in laser-wire directed energy deposition of Ti-6Al-4V. Addit. Manuf. 32, 100993. doi:10.1016/j.addma.2019.100993
Gibson, B. T., Richardson, B. S., Sundermann, T. W., and Love, L. J. (2019). Beyond the toolpath: site-specific melt pool size control enables printing of extra-toolpath geometry in laser wire-based directed energy deposition. Appl. Sci. 9 (20), 4355. doi:10.3390/app9204355
Giusti, A., Dotta, M., Maradia, U., Boccadoro, M., Gambardella, L. M., and Nasciuti, A. (2020). Image-based measurement of material roughness using machine learning techniques. Procedia CIRP 95, 377–382. doi:10.1016/j.procir.2020.02.292
Gobert, C., Reutzel, E. W., Petrich, J., Nassar, A. R., and Phoha, S. (2018). Application of supervised machine learning for defect detection during metallic powder bed fusion additive manufacturing using high resolution imaging. Addit. Manuf. 21, 517–528. doi:10.1016/j.addma.2018.04.005
Gomez, C., Su, R., De Groot, P., and Leach, R. (2020). Noise reduction in coherence scanning interferometry for surface topography measurement. Nanomanufacturing Metrology 3 (1), 68–76. doi:10.1007/s41871-020-00057-4
Grasso, M. (2021). In situ monitoring of powder bed fusion homogeneity in electron beam melting. Materials 14 (22), 7015. Art. no. 22. doi:10.3390/ma14227015
Guerra, M. G., Errico, V., Fusco, A., Lavecchia, F., Campanelli, S. L., and Galantucci, L. M. (2022). High resolution-optical tomography for in-process layerwise monitoring of a laser-powder bed fusion technology. Addit. Manuf. 55, 102850. doi:10.1016/j.addma.2022.102850
Harshavardhan, A., Venugopal, T., and Babu, S. (2018). “3D surface measurement through easy-snap phase shift fringe projection,” in Progress in advanced computing and intelligent engineering (Cham: Springer), 179–186.
Hirsch, M., Catchpole-Smith, S., Patel, R., Marrow, P., Li, W., Tuck, C., et al. (2017). Meso-scale defect evaluation of selective laser melting using spatially resolved acoustic spectroscopy. Proc. R. Soc. A Math. Phys. Eng. Sci. 473 (2205), 20170194. doi:10.1098/rspa.2017.0194
Hirsch, M., Dryburgh, P., Catchpole-Smith, S., Patel, R., Parry, L., Sharples, S., et al. (2018). Targeted rework strategies for powder bed additive manufacture. Addit. Manuf. 19, 127–133. doi:10.1016/j.addma.2017.11.011
Honarvar, F., Patel, S., Vlasea, M., Amini, H., and Varvani-Farahani, A. (2021). Nondestructive characterization of laser powder bed fusion components using high-frequency phased array ultrasonic testing. J. Materi Eng Perform 30 (9), 6766–6776. doi:10.1007/s11665-021-05988-7
Hu, D., and Kovacevic, R. (2003a). Modelling and measuring the thermal behaviour of the molten pool in closed-loop controlled laser-based additive manufacturing. Proc. Institution Mech. Eng. Part B J. Eng. Manuf. 217 (4), 441–452. doi:10.1243/095440503321628125
Hu, D., and Kovacevic, R. (2003b). Sensing, modeling and control for laser-based additive manufacturing. Int. J. Mach. Tools Manuf. 43 (1), 51–60. doi:10.1016/S0890-6955(02)00163-3
Huang, C., Wang, G., Song, H., Li, R., and Zhang, H. (2022). Rapid surface defects detection in wire and arc additive manufacturing based on laser profilometer. Measurement 189, 110503. doi:10.1016/j.measurement.2021.110503
Iquebal, A. S., Botcha, B., and Bukkapatnam, S. (2020). Towards rapid, in situ characterization for materials-on-demand manufacturing. Manuf. Lett. 23, 29–33. doi:10.1016/j.mfglet.2019.11.002
Iquebal, A. S., and Bukkapatnam, S. (2022). Consistent estimation of the max-flow problem: towards unsupervised image segmentation. IEEE Trans. Pattern Analysis Mach. Intell. 44 (5), 2346–2357. doi:10.1109/TPAMI.2020.3039745
Iquebal, A. S., Yadav, A., Botcha, B., Krishna Gorthi, R., and Bukkapatnam, S. (2022). Tracking and quantifying spatter characteristics in a laser directed energy deposition process using Kalman filter. Manuf. Lett. 33, 692–700. doi:10.1016/j.mfglet.2022.07.086
Iravani-Tabrizipour, M., and Toyserkani, E. (2007). An image-based feature tracking algorithm for real-time measurement of clad height. Mach. Vis. Appl. 18 (6), 343–354. doi:10.1007/s00138-006-0066-7
Jiang, Z., Zhang, A., Chen, Z., Ma, C., Yuan, Z., Deng, Y., et al. (2023). A deep convolutional network combining layerwise images and defect parameter vectors for laser powder bed fusion process anomalies classification. J. Intell. Manuf. doi:10.1007/s10845-023-02183-4
Kanatani, K., Sugaya, Y., and Niitsuma, H. (2008). Triangulation from two views revisited: hartley-Sturm vs. optimal correction. practice 4 (5).
Kanko, J. A., Sibley, A. P., and Fraser, J. M. (2016). In situ morphology-based defect detection of selective laser melting through inline coherent imaging. J. Mater. Process. Technol. 231, 488–500. doi:10.1016/j.jmatprotec.2015.12.024
Karthikeyan, A., Balhara, H., Lianos, A. K., Hanchate, A., and Bukkapatnam, S. T. (2023). In-situ surface porosity prediction in DED (directed energy deposition) printed SS316L parts using multimodal sensor fusion. arXiv Available at: https://arxiv.org/abs/2304.08658.
Karthikeyan, A., Tiwari, A., Zhong, Y., and Bukkapatnam, S. T. S. (2022). Explainable AI-infused ultrasonic inspection for internal defect detection. CIRP Ann. 71 (1), 449–452. doi:10.1016/j.cirp.2022.04.036
Kumar, M., and Garg, D. P. (2004). “Intelligent multi-sensor fusion techniques in flexible manufacturing workcells,” in presented at the Proceedings of the 2004 American Control Conference, Boston, MA, USA, 30 June 2004 - 02 July 2004 (IEEE), 5375–5380.
Lapointe, S., Guss, G., Reese, Z., Strantza, M., Matthews, M. J., and Druzgalski, C. L. (2022). Photodiode-based machine learning for optimization of laser powder bed fusion parameters in complex geometries. Addit. Manuf. 53, 102687. doi:10.1016/j.addma.2022.102687
Lazaros, N., Sirakoulis, G. C., and Gasteratos, A. (2008). Review of stereo vision algorithms: from software to hardware. Int. J. Optomechatronics 2 (4), 435–462. doi:10.1080/15599610802438680
Leung, C. L. A., Marussi, S., Atwood, R. C., Towrie, M., Withers, P. J., and Lee, P. D. (2018). In situ X-ray imaging of defect and molten pool dynamics in laser additive manufacturing. Nat. Commun. 9 (1), 1355. Art. no. 1. doi:10.1038/s41467-018-03734-7
Li, J., Zheng, Y., Liu, L., and Li, B. (2021a). 4D line-scan hyperspectral imaging. Opt. Express 29 (21), 34835–34849. doi:10.1364/oe.441213
Li, Y., Li, X., Zhang, G., Horváth, I., and Han, Q. (2021b). Interlayer closed-loop control of forming geometries for wire and arc additive manufacturing based on fuzzy-logic inference. J. Manuf. Process. 63, 35–47. doi:10.1016/j.jmapro.2020.04.009
Liang, Z., Chang, B., Zhang, H., Li, Z., Peng, G., Du, D., et al. (2022). Electric current evaluation for process monitoring in electron beam directed energy deposition. Int. J. Mach. Tools Manuf. 176, 103883. doi:10.1016/j.ijmachtools.2022.103883
Liang, Z., Liao, Z., Zhang, H., Li, Z., Wang, L., Chang, B., et al. (2023). Improving process stability of electron beam directed energy deposition by closed-loop control of molten pool. Addit. Manuf. 72, 103638. doi:10.1016/j.addma.2023.103638
Liao, S., Webster, S., Huang, D., Council, R., Ehmann, K., and Cao, J. (2022). Simulation-guided variable laser power design for melt pool depth control in directed energy deposition. Addit. Manuf. 56, 102912. doi:10.1016/j.addma.2022.102912
Lin, X., Wang, Q., Fuh, J. Y. H., and Zhu, K. (2022). Motion feature based melt pool monitoring for selective laser melting process. J. Mater. Process. Technol. 303, 117523. doi:10.1016/j.jmatprotec.2022.117523
Liu, C., Wang, R., Kong, Z., Babu, S., Joslin, C., and Ferguson, J. (2019). “Real-time 3D surface measurement in additive manufacturing using deep learning,” in presented at the 2019 International Solid Freeform Fabrication Symposium (University of Texas at Austin).
Liu, M., Kumar, A., Bukkapatnam, S., and Kuttolamadom, M. (2021). A review of the anomalies in directed energy deposition (DED) processes & potential solutions - Part Quality & defects. Procedia Manuf. 53, 507–518. doi:10.1016/j.promfg.2021.06.093
Liu, P., Yang, L., Yi, K., Kundu, T., and Sohn, H. (2023). Application of nonlinear ultrasonic analysis for in situ monitoring of metal additive manufacturing. Struct. Health Monit. 22 (3), 1760–1775. doi:10.1177/14759217221113447
Liu, R., Wang, Z., Sparks, T., Liou, F., and Nedic, C. (2017). Stereo vision-based repair of metallic components. Rapid Prototyp. J. 23 (1), 65–73. doi:10.1108/RPJ-09-2015-0118
Liu, T., Lough, C. S., Sehhat, H., Ren, Y. M., Christofides, P. D., Kinzel, E. C., et al. (2022). In-situ infrared thermographic inspection for local powder layer thickness measurement in laser powder bed fusion. Addit. Manuf. 55, 102873. doi:10.1016/j.addma.2022.102873
Lu, Q., Grasso, M., Le, T.-P., and Seita, M. (2022). Predicting build density in L-PBF through in-situ analysis of surface topography using powder bed scanner technology. Addit. Manuf. 51, 102626. doi:10.1016/j.addma.2022.102626
Lu, Q. Y., Nguyen, N. V., Hum, A. J. W., Tran, T., and Wong, C. H. (2020). Identification and evaluation of defects in selective laser melted 316L stainless steel parts via in-situ monitoring and micro computed tomography. Addit. Manuf. 35, 101287. doi:10.1016/j.addma.2020.101287
Ly, S., Rubenchik, A. M., Khairallah, S. A., Guss, G., and Matthews, M. J. (2017). Metal vapor micro-jet controls material redistribution in laser powder bed fusion additive manufacturing. Sci. Rep. 7 (1), 4085–4112. doi:10.1038/s41598-017-04237-z
Ma, D., Jiang, P., Shu, L., Qiu, Y., Zhang, Y., and Geng, S. (2023). DBN-based online identification of porosity regions during laser welding of aluminum alloys using coherent optical diagnosis. Opt. Laser Technol. 165, 109597. doi:10.1016/j.optlastec.2023.109597
Matilainen, V.-P., Piili, H., Salminen, A., and Nyrhilä, O. (2015). Preliminary investigation of keyhole phenomena during single layer fabrication in laser additive manufacturing of stainless steel. Phys. Procedia 78, 377–387. doi:10.1016/j.phpro.2015.11.052
Mi, J., Zhang, Y., Li, H., Shen, S., Yang, Y., Song, C., et al. (2023). In-situ monitoring laser based directed energy deposition process with deep convolutional neural network. J. Intell. Manuf. 34 (2), 683–693. doi:10.1007/s10845-021-01820-0
Millon, C., Vanhoye, A., Obaton, A.-F., and Penot, J.-D. (2018). Development of laser ultrasonics inspection for online monitoring of additive manufacturing. Weld. World 62 (3), 653–661. doi:10.1007/s40194-018-0567-9
Min, Y., Shen, S., Li, H., Liu, S., Mi, J., Zhou, J., et al. (2022). Online monitoring of an additive manufacturing environment using a time-of-flight mass spectrometer. Measurement 189, 110473. doi:10.1016/j.measurement.2021.110473
Nakkina, T. G., Vinayaka, M., Masad, A., Mansori, M. E., and Bukkapatnam, S. (2022). Smart defect identification for manufacturing applications. Surf. Topogr. Metrol. Prop. 10 (3), 035045. doi:10.1088/2051-672X/ac9247
Nguyen, T. M., and Wu, Q. M. J. (2013). Fast and robust spatially constrained Gaussian mixture model for image segmentation. IEEE Trans. Circuits Syst. Video Technol. 23 (4), 621–635. doi:10.1109/TCSVT.2012.2211176
O’Dowd, N. M., Wachtor, A. J., and Todd, M. D. (2021). Effects of digital fringe projection operational parameters on detecting powder bed defects in additive manufacturing. Addit. Manuf. 48, 102454. doi:10.1016/j.addma.2021.102454
Özsoy, K., Aksoy, B., and Salman, O. K. M. (2021). Investigation of the dimensional accuracy using image processing techniques in powder bed fusion. Proc. Institution Mech. Eng. Part E J. Process Mech. Eng. 235 (5), 1587–1597. doi:10.1177/09544089211011011
Patel, R., Hirsch, M., Dryburgh, P., Pieris, D., Achamfuo-Yeboah, S., Smith, R., et al. (2018). Imaging material texture of as-deposited selective laser melted parts using spatially resolved acoustic spectroscopy. Appl. Sci. 8 (10), 1991. Art. no. 10. doi:10.3390/app8101991
Pieris, D., Patel, R., Dryburgh, P., Hirsch, M., Li, W., Sharples, S. D., et al. (2019). Spatially resolved acoustic spectroscopy towards online inspection of additive manufacturing. Insight - Non-Destructive Test. Cond. Monit. 61 (3), 132–137. doi:10.1784/insi.2019.61.3.132
Qi, S., Yang, J., and Zhong, Z. (2020). “A review on industrial surface defect detection based on deep learning technology,” in presented at the 2020 the 3rd international conference on machine learning and machine intelligence, 24–30.
Radel, S., Diourte, A., Soulié, F., Company, O., and Bordreuil, C. (2019). Skeleton arc additive manufacturing with closed loop control. Addit. Manuf. 26, 106–116. doi:10.1016/j.addma.2019.01.003
Raffestin, M., Domashenkov, A., Bertrand, P., Faverjon, P., and Courbon, C. (2023). Ultrasonic diagnostic for in situ control in metal additive manufacturing. Measurement 206, 112244. doi:10.1016/j.measurement.2022.112244
Regulin, D., and Barucci, R. (2023). A benchmark of approaches for closed loop control of melt pool shape in DED. Int. J. Adv. Manuf. Technol. 126 (1–2), 829–843. doi:10.1007/s00170-023-11042-8
Reich, S., Göbel, A., Goesmann, M., Heunoske, D., Schäffer, S., Lueck, M., et al. (2022). 2D and 3D triangulation are suitable in situ measurement tools for high-power large spot laser penetration processes to visualize depressions and protrusions before perforating. Materials 15 (11), 3743. Art. no. 11. doi:10.3390/ma15113743
Renner, J., Breuning, C., Markl, M., and Körner, C. (2022). Surface topographies from electron optical images in electron beam powder bed fusion for process monitoring and control. Addit. Manuf. 60, 103172. doi:10.1016/j.addma.2022.103172
Repossini, G., Laguzza, V., Grasso, M., and Colosimo, B. M. (2017). On the use of spatter signature for in-situ monitoring of Laser Powder Bed Fusion. Addit. Manuf. 16, 35–48. doi:10.1016/j.addma.2017.05.004
Sharples, S. D., Clark, M., and Somekh, M. G. (2006). Spatially resolved acoustic spectroscopy for fast noncontact imaging of material microstructure. Opt. Express, OE 14 (22), 10435–10440. doi:10.1364/OE.14.010435
Smith, R. J., Li, W., Coulson, J., Clark, M., Somekh, M. G., and Sharples, S. D. (2014). Spatially resolved acoustic spectroscopy for rapid imaging of material microstructure and grain orientation. Meas. Sci. Technol. 25 (5), 055902. doi:10.1088/0957-0233/25/5/055902
Smoqi, Z., Bevans, B. D., Gaikwad, A., Craig, J., Abul-Haj, A., Roeder, B., et al. (2022). Closed-loop control of meltpool temperature in directed energy deposition. Mater. Des. 215, 110508. doi:10.1016/j.matdes.2022.110508
Sotelo, L. D., Karunakaran, R., Pratt, C. S., Sealy, M. P., and Turner, J. A. (2021). Ultrasound in situ characterization of hybrid additively manufactured Ti6Al4V. J. Acoust. Soc. Am. 150 (6), 4452–4463. doi:10.1121/10.0008972
Su, Y., Wang, C., Xu, X., Luo, K., and Lu, J. (2023). Pore defects and corrosion behavior of AISI 316L stainless steel fabricated by laser directed energy deposition under closed-loop control. Surf. Coatings Technol. 463, 129527. doi:10.1016/j.surfcoat.2023.129527
Su, Y., Wang, Z., Lu, H., Luo, K., and Lu, J. (2022b). Improved wear resistance of directed energy deposited Fe-Ni-Cr alloy via closed-loop controlling laser power. J. Manuf. Process. 75, 802–813. doi:10.1016/j.jmapro.2022.01.047
Su, Y., Wang, Z., Xu, X., Luo, K., and Lu, J. (2022a). Effect of closed-loop controlled melt pool width on microstructure and tensile property for Fe-Ni-Cr alloy in directed energy deposition. J. Manuf. Process. 82, 708–721. doi:10.1016/j.jmapro.2022.08.049
Sun, Z., Guo, W., and Li, L. (2020). In-process measurement of melt pool cross-sectional geometry and grain orientation in a laser directed energy deposition additive manufacturing process. Opt. Laser Technol. 129, 106280. doi:10.1016/j.optlastec.2020.106280
Tapia, G., and Elwany, A. (2014). A review on process monitoring and control in metal-based additive manufacturing. J. Manuf. Sci. Eng. 136 (6). doi:10.1115/1.4028540
The global machine vision market (2023). The global machine vision market size was valued at $9.01 billion in 2022 & is projected to grow from $9.68 billion in 2023 to $16.82 billion by 2030.pdf.
Tiwari, A., Villasenor, E. J., Gupta, N., Reddy, N., Karri, R., and Bukkapatnam, S. T. S. (2021). “Protection against counterfeiting attacks in 3D printing by streaming signature-embedded manufacturing process instructions,” in Proceedings of the 2021 Workshop on Additive Manufacturing (3D Printing) Security, in AMSec ’21, New York, NY, USA, November 2021 (New York, NY, USA: Association for Computing Machinery), 11–21. doi:10.1145/3462223.3485620
Vallabh, C. K. P., Xiong, Y., and Zhao, X. (2021). “In-situ monitoring of laser powder bed fusion process anomalies via a comprehensive analysis of off-Axis camera data,” in presented at the ASME 2020 15th International Manufacturing Science and Engineering Conference, American Society of Mechanical Engineers Digital Collection. doi:10.1115/MSEC2020-8300
Wang, K., Dave, P., Hanchate, A., Sagapuram, D., Natarajan, G., and Bukkapatnam, S. T. (2022). Implementing an open-source sensor data ingestion, fusion, and analysis capabilities for smart manufacturing. Manuf. Lett. 33, 893–901. doi:10.1016/j.mfglet.2022.07.109
Wang, R., Law, A. C., Garcia, D., Yang, S., and Kong, Z. (2021). Development of structured light 3D-scanner with high spatial resolution and its applications for additive manufacturing quality assurance. Int. J. Adv. Manuf. Technol. 117 (3), 845–862. doi:10.1007/s00170-021-07780-2
Wang, Z., Liu, R., Sparks, T., Liu, H., and Liou, F. (2015). Stereo vision based hybrid manufacturing process for precision metal parts. Precis. Eng. 42, 1–5. doi:10.1016/j.precisioneng.2014.11.012
Wolff, S. J., Wang, H., Gould, B., Parab, N., Wu, Z., Zhao, C., et al. (2021b). In situ X-ray imaging of pore formation mechanisms and dynamics in laser powder-blown directed energy deposition additive manufacturing. Int. J. Mach. Tools Manuf. 166, 103743. doi:10.1016/j.ijmachtools.2021.103743
Wolff, S. J., Webster, S., Parab, N. D., Aronson, B., Gould, B., Greco, A., et al. (2021a). In-situ observations of directed energy deposition additive manufacturing using high-speed X-ray imaging. JOM 73 (1), 189–200. doi:10.1007/s11837-020-04469-x
Wong, H. (2020). Pilot investigation of surface-tilt and gas amplification induced contrast during electronic imaging for potential in-situ electron beam melting monitoring. Addit. Manuf. 35, 101325. doi:10.1016/j.addma.2020.101325
Wong, H., Neary, D., Shahzad, S., Jones, E., Fox, P., and Sutcliffe, C. (2019). Pilot investigation of feedback electronic image generation in electron beam melting and its potential for in-process monitoring. J. Mater. Process. Technol. 266, 502–517. doi:10.1016/j.jmatprotec.2018.10.016
Xie, J., Jiang, T., and Chen, X. (2022). An image segmentation framework for in-situ monitoring in laser powder bed fusion additive manufacturing. IFAC-PapersOnLine 55 (37), 800–806. doi:10.1016/j.ifacol.2022.11.280
Xiong, J., Yin, Z., and Zhang, W. (2016). Closed-loop control of variable layer width for thin-walled parts in wire and arc additive manufacturing. J. Mater. Process. Technol. 233, 100–106. doi:10.1016/j.jmatprotec.2016.02.021
Yan, H., Grasso, M., Paynabar, K., and Colosimo, B. M. (2022). Real-time detection of clustered events in video-imaging data with applications to additive manufacturing. IISE Trans. 54 (5), 1–28. doi:10.1080/24725854.2021.1882013
Yang, H.-C., Huang, C.-H., Adnan, M., Hsu, C.-H., Lin, C.-H., and Cheng, F.-T. (2021). An online AM quality estimation architecture from pool to layer. IEEE Trans. Autom. Sci. Eng. 18 (1), 269–281. doi:10.1109/TASE.2020.3012622
Yang, Z., Zhu, L., Dun, Y., Ning, J., Wang, S., Xue, P., et al. (2023). In-situ monitoring of the melt pool dynamics in ultrasound-assisted metal 3D printing using machine learning. Virtual Phys. Prototyp. 18 (1), e2251453. doi:10.1080/17452759.2023.2251453
You, D., Gao, X., and Katayama, S. (2014). WPD-PCA-based laser welding process monitoring and defects diagnosis by using FNN and SVM. IEEE Trans. Industrial Electron. 62 (1), 628–636. doi:10.1109/tie.2014.2319216
Zhang, B., Ziegert, J., Farahi, F., and Davies, A. (2016). In situ surface topography of laser powder bed fusion using fringe projection. Addit. Manuf. 12, 100–107. doi:10.1016/j.addma.2016.08.001
Zhang, W., Wang, J., Tang, M., Ma, H., Wang, L., Zhang, Q., et al. (2023b). 2-D transformer-based approach for process monitoring of metal 3-D printing via coaxial high-speed imaging. IEEE Trans. Industrial Inf., 1–11. doi:10.1109/TII.2023.3314071
Zhang, X., Shen, W., Suresh, V., Hamilton, J., Yeh, L. H., Jiang, X., et al. (2021). In situ monitoring of direct energy deposition via structured light system and its application in remanufacturing industry. Int. J. Adv. Manuf. Technol. 116 (3), 959–974. doi:10.1007/s00170-021-07495-4
Zhang, X., Zheng, Y., Suresh, V., Wang, S., Li, B., et al. (2020). Correlation approach for quality assurance of additive manufactured parts based on optical metrology. J. Manuf. Process. 53, 310–317. doi:10.1016/j.jmapro.2020.02.037
Zhang, Y., Zhang, P., Jiang, X., Zhang, S., Zhong, K., and Li, Z. (2023a). In-situ 3D contour measurement for laser powder bed fusion based on phase guidance. Theor. Appl. Mech. Lett. 13 (2), 100405. doi:10.1016/j.taml.2022.100405
Zhao, C., Fezzaa, K., Cunningham, R. W., Wen, H., De Carlo, F., Chen, L., et al. (2017). Real-time monitoring of laser powder bed fusion process using high-speed X-ray imaging and diffraction. Sci. Rep. 7 (1), 3602. Art. no. 1. doi:10.1038/s41598-017-03761-2
Keywords: fusion-based metal additive manufacturing, high speed imaging, thermal imaging, optical imaging, vibration-based imaging
Citation: Balhara H, Karthikeyan A, Hanchate A, Nakkina TG and Bukkapatnam STS (2023) Imaging systems and techniques for fusion-based metal additive manufacturing: a review. Front. Manuf. Technol. 3:1271190. doi: 10.3389/fmtec.2023.1271190
Received: 01 August 2023; Accepted: 06 December 2023;
Published: 21 December 2023.
Edited by:
Prahalada Rao, Virginia Tech, United StatesReviewed by:
Yuanbin Wang, Northwestern Polytechnical University, ChinaCopyright © 2023 Balhara, Karthikeyan, Hanchate, Nakkina and Bukkapatnam. This is an open-access article distributed under the terms of the Creative Commons Attribution License (CC BY). The use, distribution or reproduction in other forums is permitted, provided the original author(s) and the copyright owner(s) are credited and that the original publication in this journal is cited, in accordance with accepted academic practice. No use, distribution or reproduction is permitted which does not comply with these terms.
*Correspondence: Satish T. S. Bukkapatnam, c2F0aXNoQHRhbXUuZWR1
Disclaimer: All claims expressed in this article are solely those of the authors and do not necessarily represent those of their affiliated organizations, or those of the publisher, the editors and the reviewers. Any product that may be evaluated in this article or claim that may be made by its manufacturer is not guaranteed or endorsed by the publisher.
Research integrity at Frontiers
Learn more about the work of our research integrity team to safeguard the quality of each article we publish.