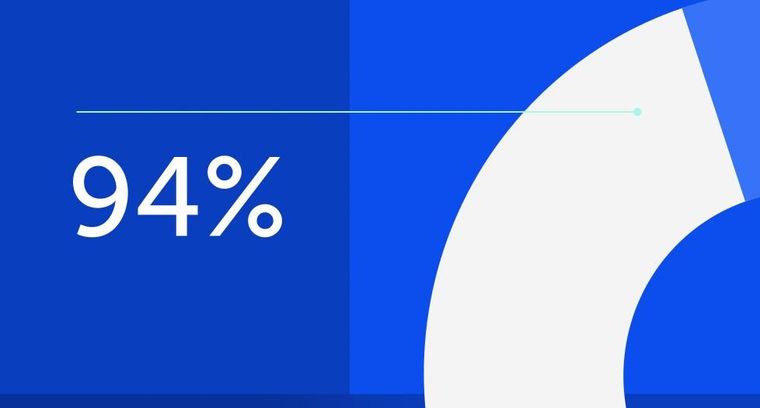
94% of researchers rate our articles as excellent or good
Learn more about the work of our research integrity team to safeguard the quality of each article we publish.
Find out more
ORIGINAL RESEARCH article
Front. Manuf. Technol., 30 January 2023
Sec. Sustainable Life Cycle Engineering and Manufacturing
Volume 3 - 2023 | https://doi.org/10.3389/fmtec.2023.1057537
Data monetization (DM) has become a relevant aspect of the industrial manufacturing. Consequently, this paper proposes a theoretical framework as well as a mathematical model to price industrial data. For this purpose, three characteristics of the data were considered, i.e. 1) quality; 2) entropy and 3) value. Besides, the role of data marketplace’s players was analyzed. In order to validate the economic equation, a case study was carried out by a Spanish manufacturer.
While data is a novel source of revenue and competitive advantage (Hanafizadeh and Nik, 2020) the debate on DM, i.e. “the way an organization pursues to use data for quantifiable economic benefit” (Baecker et al., 2020), has achieved more and more practical and theoretical relevance (Mehta et al., 2019). Consequently, industrial firms are looking for stronger competitive advantages by adopting strategies to become more data-driven in their business decisions (Berndtsson et al., 2018), and struggle to develop more data-based business models (Kühne and Böhmann, 2019). Manufacturing firms are particularly interested in the development of data-driven services (Azkan et al., 2020; Zambetti et al., 2021) and digital servitization (Paschou et al., 2020), which is defined by Paiola and Gebauer (2020) as a research stream focusing on how digital technology enables the supply of services in innovative ways. In parallel, the Industry 4.0 (Lu, 2017) and, consequently, the Internet of Things (IoT) (Rehman et al., 2019), play a key role here in the development and delivery of manufacturers’ advanced services (Schroeder et al., 2020). Nevertheless, shifting from free to fee services (Witell and Löfgren, 2013) is not easy, especially when value comes from intangible assets (Zhang and Beltran, 2020).
That is why considering the data characteristics is so relevant to understand the DM process. In particular, this paper considers the ones related to 1) the data quality (Taleb et al., 2021) and 2) the data information entropy, which prices data goods from the perspective of information amount (Li et al., 2017). While “the proper assessment of data value is the basis of a rigorous and reasonable data pricing model” (Yu and Zhang, 2017), relevant data characteristic 3) is the value of data. In this regard, Monteiro et al. (2020) state that “in terms of economic value, studies are even scarcer, and the existing ones do not share a common view on how to measure this value.”
In parallel, it appears that the journey from data to value should be designed (Rapaccini et al., 2021) by characterising the roles and capabilities of each players in what is called the data economy (Opher et al., 2016). Concretely, Muschalle et al. (2013) looked at the different data market beneficiaries and participants before analysing their willingness to pay and the pricing strategies. That is why this paper will analyse 4) the data marketplace itself.
In line with the considerations above, “we clearly need more research on DM, particularly given that companies have shown an increased interest in it.” (Parvinen et al., 2020). Thus, the research question that this paper addresses is the following one:
• RQ: How industrial manufacturers should define the pricing model to monetize data-driven industrial services?
In order to answer this research question, this paper proposes a theoretical framework that describes two different dimensions, four components and six elements of the DM problem. Based on this framework a second objective is obtained: The development of a quantitative data pricing model, integrating five variables, i.e. supply price, data quality, data entropy, data score and the customer relevance index. The model is then applied to the case of a Spanish industrial manufacturer, analyzing the data from a sensorized industrial machine.
The remainder of this article is structured as follows. Section 2 introduces previous theories on industrial data management, presenting a review of the literature on DM, data characteristics, data marketplaces and data pricing. Section 3 describes the research objectives and methodology, as well as the reference work of Shen et al. (2016) in which the proposed model is based on. Section 4 proposes a theoretical framework and Section 5 and Section 6 presents the pricing model, as well as its application to a case study. Finally, Section 7 mentions the conclusion, limitations and future reseach lines.
Hanafizadeh and Nik (2020) carried out a systematic literature review on this topic, offering a model called ‘‘DM configuration’’, while others (Liozu and Ulaga, 2018; Woroch and Strobel, 2022) identified the main characteristics and components of data-driven business models affecting data-based value creation, pricing and DM process. In addition, Parvinen et al. (2020) analysed companies’ proposals to sell and monetize data, in line with the works of Teece and Linden (2017) and Thomas and Leiponen (2016).
Table 1 compares different DM definitions, showing that, as yet, no consensus has been reached in the scientific debate. Nevertheless, it is evident that the characteristics of the key concept, i.e. data, should be analyzed in order to obtain an economic benefit. These were described by a set of stakeholders as FAIR Data Principles, i.e. Findable, Accesible, Interoperable and Reusable, “enhancing the ability of machines to automatically find and use the data, in addition to supporting its reuse by individuals” (Wilkinson et al., 2016). This is also confirmed by Demchenko et al. (2018), who use the STREAM properties concept for industrial and commoditised data, i.e. Sovereign, Trusted, Reusable, Exchangeable, Actionable and Measurable data. Data are also nonrivalrous (Pantelis and Aija, 2013), and can be copied perfectly (Thomas and Leiponen, 2016).
Moreover, the quality of data, i.e. the degree to which a set of inherent characteristics of data fulfils requirements (ISO 8000) is considered to be another key characteristic, which should be analysed from an organisational, architectural and computational point of view (Sadiq, 2013). Consequently, it is possible to identify their main dimensions (DAMA United Kingdom (2013) in (DAMA-DMBOK, 2017) and consult some frameworks for determining the quality of (big) data (Wang and Strong, 1996). In the same line of research, Cichy and Rass (2019) proposed an overview of 12 data quality frameworks, including data quality definitions, assessments and improvements, whereas Ehrlinger and Wöß (2022) made a survey of data quality measurement and monitoring tools. Furthermore, the cost of data production and the data pricing strategy relates directly to data quality (Yu and Zhang, 2017), which may give rise to some relevant problems (Zhang et al., 2019), and the need to classify the inflicted costs (Haug et al., 2011). Some data quality attributes are identified by (Abdullah et al., 2015) like accuracy, consistency or timeliness among others.
Based on the mathematical theory of communication (Shannon, 1948), the information entropy refers to a probability distribution function that is used to measure the uncertainty. In this sense, the higher its value, the higher its uncertainty and, therefore, the lower the possibility of correctly estimating its value. This concept was used by Attaran and Zwick (1987) to measure the industrial diversification, whereas Holzinger et al. (2014) used it on data mining. In the case of Monteiro et al. (2020) the information entropy serves to investigate DM in the context of Big Data. In fact, one of their research questions is specifically related to identify the use of the information theory in data value assignation methods. In line with this research, Li et al. (2019) analysed the existing literature on data value evaluation methods, where information management methods like the information value entropy are also identified. This concept is also used in papers like Rao and Keong (2016), Li et al. (2017) and Shen et al. (2016).
On this topic, Lim et al. (2018) pointed out that there is a large gap in the literature between data and value creation. For example, Otto (2015) considers that “data has a value in itself,” whereas the study proposed by GSMA (2018) states that “data value is only realized after it has been put to use and it has no intrinsic value of its own.” In addition, the challenges related to value creation are linked to companies’ internal and external factors. Not surprisingly, “a well-designed business model balances the provision of value to customers with the capture of value by the provider” (Teece and Linden, 2017). That is why, internally, companies should consider developing a methodology capable of generating value from data science (Meierhofer and Meier, 2017), taking in key aspects such as the digital value chain (Rayport and Sviokla, 1995) or the (big) data value chain (Moro Visconti et al., 2017; Faroukhi et al., 2020).
The data value is also created externally, i.e. inside a data ecosystem (Thomas and Leiponen, 2016). In fact, the market structure plays a key role in determining the value of data (Zhang and Beltrán, 2020). Moreover, the “value is created interdependently and mutually rather than in isolation” (Thomas and Leiponen (2016) in Saleh et al., 2013), which means that the data market structures determine the data price. As a consequence of that, the data marketplaces should also be considered. This concept is defined by Koutroumpis et al. (2017) as “a multi-side platform, where digital intermediary connects data providers, data purchasers, and other complementary technology providers.” On this topic, a state of art is proposed by Abbas et al. (2021), whereas Spiekerman (2019) proposes an overview of the concept. Nevertheless, one of the mayor challenges to enable an efficient data marketplace is the definition of a proper pricing model (Liang et al., 2018). Besides, the market value of data is mostly determined through value-based parameters, which are difficult to quantify and model (Heckman et al., 2015). Table 2 summarizes the different players that participates in the data Marketplace.
While DM take place “when the intangible value of data is converted into real value, usually by selling it” (Najjar and Kettinger, 2013), it is important to understand the data pricing models, i.e. setting a price at which data can be sold or purchased (Pei, 2020). This same author considers the pricing models from a global perspective, that is, from economics to data science, whereas.
Fricker and Maksimov (2017) identified two groups of pricing models: The ones that aim at internal consistency and fairness, and those related to game-theoretic approaches for maximizing social welfare or profit. They also considered different variables to determine the price, e.g. the usage or cost of the data. With regard to this last element, Shapiro and Varian (1999) pointed out that the initial cost of collecting data is relevant, while Pantelis and Aija (2013) argued that the marginal cost of copying and disseminating is cheap.
A reversed pricing method is used by Shen et al. (2016). This concept is defined by Bernhardt (2004) as a “dynamic pricing mechanism, where both seller and buyer influence the final price of a transaction.” This is also used by Stahl and Vossen (2017), who state that [their] “model enables data providers to tap the willingness to pay of customers, who would otherwise not buy their relational data product; in turn customers receive a highly custom-tailored data product.” Based on the work of Liang et al. (2018) Table 3 describes six different data pricing models considering four elements.
In the case of Zhang and Beltrán (2020) survey on data pricing models is presented, identifying, among others, the work of Tang et al. (2013). In this study, they “assign a price to each source tuple in the database,” a technique that is also used by Shen et al. (2019) and by Balazinska et al. (2011). This leads to the concept of granularity, which “refers to the extent to which a dataset can be subdivided” (Zhang and Beltrán, 2020). Otto (2015) identifies different levels of logical aggregation in relation to this, i.e. item, data records, tables (or files), databases and data resources, while Shen et al. (2016) suppose that a data packet has data tuples, attributes and items. Besides, they developed a model for pricing personal data, where the data packet is considered as a basic sales unit. Their dynamically adjustable method proposed a positive grading and reverse pricing model on tuple granularity, which tried to accurately control the price of each data tuple and reflect its due value. The proposed data pricing equation and the different constraints are described as follows.
It thus appears that data tuples prices are obtained using three main factors, i.e. value weight, information entropy and data reference index, as well as the supply price of one data tuple. A detailed description of each element is given below:
The cost of sharing, analysing and collecting trading platform data (C) is subtracted from the demand price of a data packet (PD) to obtain the supply price of a data packet, named
The value weight is obtained through the experience of the evaluator. Thus,
The entropy of the i-th data tuple in a data packet (5) and the total entropy of all data tuples in a data packet (6) are defined using the following formulas:
Considering these two equations, the ratio of the information content is obtained by assigning
The Data Reference Index considers both the purchase amount and purchase times of the data tuples in order to measure the authority of users. The Data Reference Index value of the j-th user is
The aim of this article is to understand the DM process, which is a central topic of the data-driven business models, as well as the development of digital services. Therefore, the first objective is to propose a theoretical framework that depicts the major insights of DM. Thanks to this theoretical work, the second objective is to develop a quantitative pricing model for assigning a price to data-driven services.
In the first phase of the research, a systematic review of the literature (Okoli and Schabram, 2010) was undertaken, using databases such as Scopus and Google Scholar and considering academic papers published in journals and conference proceedings. The selected papers were written in English, without narrowing the search to any timeframe. More specifically, several combinations of keywords were used, such as “data monetization,” “data pricing,” “data quality,” “data value,” “data properties” and “industrial data.” As a result, the abstracts and keywords of more than 100 articles were analyzed.
In the second phase, a case study was carried out based on a Spanish manufacturer that assembles equipment for manufacturing glass receptacles. Voss et al. (2002) argued that case research can “lead to new and creative insights, development of new theory and have high validity with practitioners—the ultimate user of research.” The reason for selecting it was the fact that this company was implementing a process of (digital) servitization, which required the participation of two of its main departments: The After-Sales Services Department and the R&D Department. Furthermore, this industrial manufacturer was collaborating with a third company in order to use a data monitoring dashboard (Sarikaya et al., 2018) and, therefore, to analyse, process and consult industrial data. In fact, it is important to point out that “Industry 4.0 scenarios require a complete integration of machines, processes and information flows based on advanced information and communication technology” (Freichel et al., 2020). As far as our case study is concerned, the sensor system incorporated into the machine was able to classify data into two main groups based on their location in the machine, namely the bottle making machine (BMM) and the bottle production line (BPL). This technical aspect is in line with the theoretical analysis carried out by Niyato et al. (2016), who stated that in order to “collect a large amount of data with high quality, a number of sensors have to be deployed which will result in high costs.”
The BMM data were located inside the machine, which was made up of different mechanical parts (MP): MP_1 is where the material is placed to begin the extrusion cycle. MP_2 is the optional auxiliary equipment, whose job is to change filters to avoid having to stop the machine. MP_3 is the part of the extruder through which the molten material passes ready for injection into the mould. It regulates the density in the material tube to obtain the best possible quality. Next to the head and just before the twisting point of the rotary unit there is a belt that removes the parison before the process of manufacturing suitable bottles begins. The machine has two rotary joints on each side of the edge which control the entry and exit of mould-cooling water (MP_4). Bottles are collected by a rotating pick-up arm system (MP_5), and MP_6 is the part of the machine where the material to be blown is prepared.
Bottles are transported along a conveyor belt (BPL) on exiting the BMM. The next sensor detects the total number of bottles, and the bottom-removing machine detaches any surplus material from each item. Any quality failures are set aside at the testing stage and reused for further production, while items in good condition are isolated as individual units. There is also a special sensor which checks that surplus material has been correctly removed. This is used just before the finishing stage, where certain product quality aspects are checked and confirmed. Finally, the units are picked up and packaged.
More specifically, six data tuples representing the overall effectiveness of the equipment, its maintenance, consumption, alarms, temperature process and the feeding system process were analysed. These data items, e.g. Table 4 were produced one row per second, and could be consulted and downloaded via a dashboard in the following formats: 1) xls; 2) csv; and 3) xlsx. For the sake of confidentiality, some of the information used in the model are aggregated or exemplified.
In order to develop the proposed model, which is based on Shen et al. (2016), some adaptative changes were required. First, the industrial machine itself is considered as the basic sales unit. Second, the thematic tabs of the dashboard are the equivalent of data tuples. Third, each data tuple has k attributes, i.e. the items available in each tabs of the dashboard. The value weight that Shen et al. (2016) integrates in the equation is similar to the data score variables. This is obtained by a questionnaire based on the Likert scale and completed by four employees of the customer and four employees of the industrial manufacturer and. They were asked to rate the six items mentioned above from one to seven, with seven as the highest value and one as the lowest. For the sake of confidentiality, the acronyms IT1, IT2, IT3, IT4, IT5 and IT6 are used to present the items.
A research framework was used to begin with the theory-building research, as proposed by Miles and Huberman (1994). Consequently, the following framework considers the two main dimensions that should be considered in order to monetize industrial data: 1) Data characteristics; 2) data marketplace. Besides, the above-mentioned dimensions are composed by four components, i.e. quality, entropy, value and players, and six elements: Number of recorded lines, the probability of uncertainty, the questionnaire and the data marketplace players, i.e. data producer, information producer and third company. In order to integrate this framework to the proposed equation and thus to the case study, five mathematical variables are proposed: The supply price, data quality, data entropy, data score and CRI. For more information see Table 5.
Although the sale of machines takes place between the industrial manufacturer and the customer, data-driven services involves a change in role for these economic agents. As a consequence, the data marketplace dynamics should be understood, as well as the roles of each player. For instance, the customer becomes the data producer through using the machine and the sensors incorporated into it. In fact, it is important to understand and optimize the IoT system, which is the key element in such data production. (Schroeder et al. (2020) in Suppatvech et al., 2019) posit that “the core contribution of the IoT in an advanced services context is premised on a customer authorising the manufacturer to closely monitor its product as it forms an integral part of the customer’s business—a concession that requires trust in the manufacturer–customer relationship.” Meanwhile, based on the analysis, enrichment and transformation of this data, the industrial manufacturer becomes the information producer. At the same time, there is a third company that bridges the gap between the data and information producers. To do so, a dashboard is provided based on the characteristics of the data and the final format of the information. Finally, it is important to mention that each of the economic agents values the data differently. Therefore, the use of a questionnaire will help to assign a value to each of the items, offering a quantifiable measure of their own valuation.
In parallel, three data characteristics are required to monetize data: Data quality, data entropy and data value. In fact, all of them are closely related. Ones the data is obtained through the sensors, the analysis of the recorded lines will offer a concrete idea of the data quality: One line per second, i.e. 3600 lines per hour. Moreover, the number of recorded lines is assigned to each range of groups, availing the estimation of the probabilities and ratios, i.e. entropy. Not surprisingly, these two characteristics will influence the value assignation of the items.
The model proposed is related to the supply price and four main factors: Data score, data quality, data entropy and customer relevance index (CRI).
The following constraint should also be considered:
The characteristics of each of the four variables are detailed below.
As mentioned by Heckman et al. (2015), (the operational value of data is the cost of producing the data by the seller). To obtain this price, the marginal cost of generating, storing, and sharing the data should be considered. Therefore, it is important to analyse the three data marketplace players (the data producer, the intermediate phase and the information production phase) and the elements affecting the cost of data: 1) The cost of the energy consumed by the machine; 2) the cost of sensors; 3) the payout by the manufacturer to the third company; 4) the data analysis software; and (5) the salary of the data analyst. For more information see Figure 1.
In theory, the sensor system in the industrial machine analysed emits one line of data per second. The acronym for the total amount of line for each item is
Based on Shannon’s information theory, this variable refers to the uncertainty that a range of data took place.
The data score variable captures the numerical value that the buyer and the manufacturer give to the items analysed. The practical nature of the survey also means that there is an exchange of knowledge between the two parties. Therefore, the buyer can use it as an opportunity to make their needs known to the manufacturer, and the latter can use it as a tool for information.
In cases where the customer awards a lower score, the manufacturer can impose its own criteria and set a minimum aligned with its own interests. If the opinion is higher, the industrial manufacturer can adopt the strategy of increasing its own score to match that of the buyer. Consequently, a third column is proposed for the adjusted score.
Once the survey had been completed, a three-step method was used to obtain the ratios: Firstly, each rating given in the two blocks (the producer and the customer) was divided by the number of participants in the survey, which in this case was four. Secondly, the new data obtained were added together for each of the two blocks. Finally, the result of this sum was divided by the new data obtained. The results obtained in the survey are shown below.
This variable provides the logic for measuring the significance of each customer to the industrial manufacturer. It contains three main elements: 1) The significance of the customer in terms of their contribution to the operating account; 2) the number of machines for which the digital service is contracted; 3) whether or not the digital services are contracted by the customer. Thus, one tenth of a point is awarded for each percentage point that the customer accounts for in the manufacturer’s operating account. Finally, one point is awarded if the customer contracts the services and one more for each machine for which the product is contracted.
This section describes the case study that was undertaken after the final framework was defined to show its suitability. Some conceptual adjustments were made to adapt the above paper. In particular, the machine analysed was considered to be a data package, while the tabs displayed on the industrial manufacturer’s dashboard were considered to be the data tuples. The variables represented in the interface were those considered by Shen et al. (2016) as attributes.
The supply price (Ps) is obtained by subtracting the data cost (Dc) from the demand price (Pd). To define Dc, a data marketplace analysis was conducted, where the different players were identified, i.e. data producer, third company and information producer. The first one uses the sensor system on the industrial machine, grouped into two main units: The bottle making machine (BMM) and the bottle production line sensors (BPL). Various problems arose during this process. If the computer programming language used to capture data was inefficient it could cause several problems, such as non-available (NA) data, less data captured than expected or even a duplication of data recorded. In this sense, the elements that affected the production cost of data were 1) the cost of the sensors; and 2) the cost of the energy consumed by the machine.
A third company was hired by the manufacturer to store and create a dashboard. This interface was used to display the industrial machine data, but problems could have arisen from the company hired having a long learning curve and cybersecurity risks. Not surprisingly, the element that affected the cost of data was 3) the payout by the manufacturer to the third company.
The information producer uses an statistical analysis and monitoring of data, which served to design the data-driven services to be offered subsequently to the customer. The problems that could have arisen were related to the data consulted and downloaded from the dashboard, which could require homogenisation. The elements that affected the cost of data were 4) the data analysis software; and 5) the salary of the data analyst.
For exemplification matters the supply price has been set to 5,000€.
While the sensor system emits one line of data per second, i.e. 3,600 lines per hour, the data quality variable serves to confirm the validity of data. In fact, a range of lines is established: If the figure is under 3,550 it is considered as invalid, whereas the quality of data is considered as valid if between 3,550 and 3,600 lines are recorded. Once this “quality filter” is used, the new data samples for each of the six items are defined as DQi, with the sum of all of them being DQ. The aim is to “rewarded” with a higher final value the items that have lost few lines. Table 6 offers the final data quality results.
The readings for each of the six items were divided into five main groups, with the corresponding number of lines assigned to each range of values. Once the probability for each range was calculated, the ratios were estimated based on formulae [5] and [6]. The data is displayed in Table 7.
The data in Table 8 describes the results of the data score.
The final CRI ratio obtained was the same for the six items under consideration at 0.167. The set of ratios needed to obtain the final price are presented in Figure 2.
Besides, a possible combination of the four factors (i.e.
The digital transformation faced by industrial manufacturers requires new economic logic to monetize their data. Specifically, this study sets out to investigate a practical pricing model by carrying out research at a manufacturer in Spain. Consequently, this research makes three main contributions to the literature on DM. Firstly, it adapts the model produced by Shen et al. (2016) to the industrial data field, where there is an urgent need to develop a model for data pricing. Secondly, it provides industrial manufacturers with an overall perspective on their data, from production through the intermediate stage and on to the final production of information. Thirdly, based on a case study, it considers variables like the quality and entropy of data, integrating customers’ and manufacturers’ evaluations of data into the equation.
Nevertheless, there are some difficulties in the existing data-pricing mechanisms that makes them “simplistic and inflexible and can create undesirable arbitrage situations” (Tang et al., 2013). In this sense, the model presented is linear, i.e. the relationships between the constituent variables is lineal. That is why non-lineal relationships could be explored for validating the robustness of the results. Secondly, the case study was based on the data generated by the sensor system on one of the customer’s machines, adapting the guidelines used to measure data cost to its own characteristics. Consequently, the analysis of the different steps needs to be adapted if another industrial manufacturer or machine is considered.
As far as future research is concerned, more cases need to be examined for generalisation purposes, considering more items as well as data tuples for assessment.
The original contributions presented in the study are included in the article/Supplementary material, further inquiries can be directed to the corresponding author.
GM-A designed and implemented the model, writing the draft. EC-F contributed to the design and implementation of the research. MR analyzed the draft, providing critical feedback.
This research was funded by the BIKAINTEK 2018 scholarships awarded by the Basque Government.
We thank Full Professor Mario Tucci for assistance with data quality aspects.
The authors declare that the research was conducted in the absence of any commercial or financial relationships that could be construed as a potential conflict of interest.
All claims expressed in this article are solely those of the authors and do not necessarily represent those of their affiliated organizations, or those of the publisher, the editors and the reviewers. Any product that may be evaluated in this article, or claim that may be made by its manufacturer, is not guaranteed or endorsed by the publisher.
Abbas, A. E., Agahari, W., van de Ven, M., Zuiderwijk, A., and de Reuver, M. (2021). Business data sharing through data marketplaces: A systematic literature review. J. Theor. Appl. Electron. Commer. Res. 16 (7), 3321–3339. doi:10.3390/jtaer16070180
Abdullah, N., Ismail, S. A., Sophiayati, S., and Sam, S. M. (2015). Data quality in big data: A review. Int. J. Adv. Soft Comput. its Appl. 7 (3), 17–27.
Attaran, M., and Zwick, M. (1987). Entropy and other measures of industrial diversification. Q. J. Bus. Econ. 26 (4), 17–34.
Azkan, C., Iggena, L., Gür, I., Möller, F., and Otto, B. (June 2020). A taxonomy for data-driven services in manufacturing industries, in Proceedings of the twenty fourth Pacific Asia Conference on Information Systems, Dubai, UAE, https://aisel.aisnet.org/pacis2020/184.
Baecker, J., Engert, M., Pfaff, M., and Krcmar, H. (2020). Business strategies for data monetization: Deriving insights from practice. Wirtschaftsinformatik, 972–987. doi:10.30844/wi_2020_j3-baecker
Balazinska, M., Howe, B., and Suciu, D. (2011). Data markets in the cloud: An opportunity for the database community. Proc. Very Large Data Bases Endow. 4 (12), 1482–1485. doi:10.14778/3402755.3402801
Berndtsson, M., Forsberg, D., Stein, D., and Svahn, T. (2018). “Becoming a data-driven organisation,” in Proceedings of the 26th European Conference on Information Systems. https://aisel.aisnet.org/ecis2018_rip/43.
Cichy, C., and Rass, S. (2019). An overview of data quality frameworks. IEEE Access 7, 24634–24648. doi:10.1109/ACCESS.2019.2899751
Dama international, (2017). DAMA-DMBOK, data management body of knowledge. New Jersey, NJ: Technics Publications.
Demchenko, Y., Los, W., and de Laat, C. (2018). Data as economic goods: Definitions, properties, challenges, enabling technologies for future data markets. Int. Telecommun. Union J. ICT Discov. 2 (23). http://handle.itu.int/11.1002/pub/812388fe-en.
Đogatović, M., and Đogatović, V. R. (2021). "Users perceived pricing model for big data," in 2021 International conference on E-business Technologies. Belgrade, Serbia.
Ehrlinger, L., and Wöß, W. (2022). A survey of data quality measurement and monitoring tools. Front. Big Data 28, 850611. doi:10.3389/fdata.2022.850611
Eisenmann, T. R., Parker, G., and Van Alstyne, M. W. (2006). Strategies for two-sided markets. Harv. Bus. Rev. 84 (10), 92–101.
Faroukhi, A. Z., El Alaoui, I., Gahi, Y., and Amine, A. (2020). Big data monetization throughout big data value chain: A comprehensive review. J. Big Data 7 (1), 3–22. doi:10.1186/s40537-019-0281-5
Freichel, C., Fuchs, A., and Werner, P. (December 2020). Smart factory–requirements for exchanging machine data, Proceedings of the International Conference on Design Science Research in Information Systems and Technology, Berlin, Germany. Springer, 347–359. doi:10.1007/978-3-030-64823-7_32
Fricker, S. A., and Maksimov, Y. V. (2017). “Pricing of data products in data marketplaces,” in Software business. Proceedings of the international conference of software business. Editors A. Ojala, H. Holmström Olsson, and K. Werder, 304, 49–66. doi:10.1007/978-3-319-69191-6_4
Gsma, (2018). Data value chain. https://www.gsma.com/publicpolicy/resources/the-data-value-chain.
Hanafizadeh, P., and Nik, M. R. H. (2020). Configuration of data monetization: A review of literature with thematic analysis. Glob. J. Flexible Syst. Manag. 21 (1), 17–34. doi:10.1007/s40171-019-00228-3
Haug, A., Zachariassen, F., and van Liempd, D. (2011). The costs of poor data quality. J. Industrial Eng. Manag. 4 (2), 168–193. doi:10.3926/jiem.2011.v4n2.p168-193
Heckman, J. R., Boehmer, E. L., Peters, E. H., Davaloo, M., and Kurup, N. G. (March 2015). A pricing model for data markets,” in Proceedings of the iConference. http://hdl.handle.net/2142/73449.
Holzinger, A., Hörtenhuber, M., Mayer, C., Bachler, M., Wassertheurer, S., Pinho, A. J., et al. (2014). “On entropy-based data mining,” in Interactive knowledge discovery and data mining in biomedical informatics (Berlin, Heidelberg: Springer), 209–226. doi:10.1007/978-3-662-43968-5_12
Koutroumpis, P., Leiponen, A., and Thomas, L. D. (2017). The (unfulfilled) potential of data marketplaces ETLA working papers, The Research Institute of the Finnish Economy, Helsinki, Finland, http://hdl.handle.net/10419/201268.
Kühne, B., and Böhmann, T. (2019). “Data-driven business models-building the bridge between data and value,” in Proceedings of the European Conference on Information Systems. uppsala, sweden, https://aisel.aisnet.org/ecis2019_rp/167.
Li, X., Yao, J., Liu, X., and Guan, H. (2017). “A first look at information entropy-based data pricing,” in Proceedings of the 2017 IEEE 37th International Conference on Distributed Computing Systems (ICDCS), June 2017, Atlanta, GA, USA (IEEE), 2053–2060. doi:10.1109/ICDCS.2017.45
Li, Z., Ni, Y., Gao, X., and Cai, G. (2019). “Value evaluation of data assets: Progress and enlightenment,” in Proceedings of the 2019 4th IEEE International Conference on Big Data Analytics, March 2019, Suzhou, China, 88–93. doi:10.1109/ICBDA.2019.8713240
Liang, F., Yu, W., An, D., Yang, Q., Fu, X., and Zhao, W. (March 2018). A survey on big data market: Pricing, trading and protection. Ieee Access 6, 15132–15154. Suzhou, China, doi:10.1109/ACCESS.2018.2806881
Lim, C., Kim, K. H., Kim, M. J., Heo, J. Y., Kim, K. J., and Maglio, P. P. (2018). From data to value: A nine-factor framework for data-based value creation in information-intensive services. Int. J. Inf. Manag. 39, 121–135. doi:10.1016/j.ijinfomgt.2017.12.007
Liozu, S., and Ulaga, W. (2018). Monetizing data: A practical roadmap for framing, pricing and selling your B2B digital offers. Anthem, Arizona (USA): VIA publishing.
Lu, Y. (2017). Industry 4.0: A survey on technologies, applications and open research issues. J. Industrial Inf. Integration 6, 1–10. doi:10.1016/j.jii.2017.04.005
Mehta, S., Dawande, M., Janakiraman, G., and Mookerjee, V. (2021). How to sell a data set? Pricing policies for data monetization. Inf. Syst. Res. 32 (4), 1281–1297. doi:10.1287/isre.2021.1027
Meierhofer, J., and Meier, K. (2017). “From data science to value creation,” in Proceedings of the International Conference on Exploring Services Science (Berlin, Germany: Springer), 173–181. doi:10.1007/978-3-319-56925-3_14
Miles, H., and Huberman, M. (1994). Qualitative data analysis: A sourcebook. Beverly Hills, CA, USA: Sage.
Mišura, K., and Žagar, M. (October 2016). “Data marketplace for internet of things,” in Proceedings of the 2016 International Conference on Smart Systems and Technologies (SST), 255–260. Osijek, Croatia, doi:10.1109/SST.2016.7765669IEEE
Monteiro, D., Monteiro, L., Ferraz, F., and Meira, S. (2020). “Big data monetization: Discoveries from a systematic literature review,” in Proceedings of the Ninth International Conference on Data Analytics.9
Moro, V. R., Larocca, A., and Marconi, M. (2017). Big data-driven value chains and digital platforms: From value Co-creation to monetization. SSRN Electron. J. doi:10.2139/ssrn.2903799
Muschalle, A., Stahl, F., Löser, A., and Vossen, G. (2013). Pricing approaches for data markets. Int. Workshop Bus. Intell. Real-Time Enterp. 154, 129–144. doi:10.1007/978-3-642-39872-8_10
Najjar, M. S., and Kettinger, W. J. (2013). Data monetization: Lessons from a retailer's journey. MIS Q. Exec. 12 (4). https://aisel.aisnet.org/misqe/vol12/iss4/4.
Niyato, D., Hoang, D. T., Luong, N. C., Wang, P., Kim, D. I., and Han, Z. (2016). Smart data pricing models for the internet of things: A bundling strategy approach. IEEE Netw. 30 (2), 18–25. doi:10.1109/MNET.2016.7437020
Okoli, C., and Schabram, K. (2010). A guide to conducting a systematic literature review of information systems research. SSRN Electron. J 10 (26). doi:10.2139/ssrn.1954824
Opher, A., Chou, A., Onda, A., and Sounderrajan, K. (2016). The rise of the data economy: Driving value through internet of things data monetization. Somers, NY, USA: IBM Corporation.
Otto, B. (2015). Quality and value of the data resource in large enterprises. Inf. Syst. Manag. 32 (3), 234–251. doi:10.1080/10580530.2015.1044344
Paiola, M., and Gebauer, H. (2020). Internet of things technologies, digital servitization and business model innovation in BtoB manufacturing firms. Ind. Mark. Manag. 89, 245–264. doi:10.1016/j.indmarman.2020.03.009
Pantelis, K., and Aija, L. (2013), October 2013. “Understanding the value of (big) data,” in Proceedings of the The IEEE International Conference on Big Data (IEEE), 38–42. Silicon Valley, CA, USA, doi:10.1109/BigData.2013.6691691
Parvinen, P., Pöyry, E., Gustafsson, R., Laitila, M., and Rossi, M. (2020). Advancing data monetization and the creation of data-based business models. Commun. Assoc. Inf. Syst. 47, 25–49. doi:10.17705/1CAIS.04702
Paschou, T., Rapaccini, M., Adrodegari, F., and Saccani, N. (2020). Digital servitization in manufacturing: A systematic literature review and research agenda. Ind. Mark. Manag. 89, 278–292. doi:10.1016/j.indmarman.2020.02.012
Pei, J. (2020). A survey on data pricing: From economics to data science. IEEE Trans. Knowl. Data Eng. 34, 4586–4608. doi:10.1109/TKDE.2020.3045927
Rao, D., and Keong, N. W. (2016), July 2016. A method to price your information asset in the information market. Proceedings of the 2016 IEEE International Congress on Big Data, 307–314. San Francisco, CA, USA.
Rapaccini, M., Adrodegari, F., and Saccani, N. (2021). Digitally-enabled advanced services: Managing the journey form data to value. 10, Spring Servitization Conf., 54–62.
Rayport, J. F., and Sviokla, J. J. (1995). Exploiting the virtual value chain. Harv. Bus. Rev. 73 (6), 75. https://hbr.org/1995/11/exploiting-the-virtual-value-chain.
Rehman, M. H., Yaqoob, I., Salah, K., Imran, M., Jayaraman, P. P., and Perera, C. (2019). The role of big data analytics in industrial Internet of Things. Future Gener. Comput. Syst. 99, 247–259. doi:10.1016/j.future.2019.04.020
Sadiq, S. (2013). Handbook of data quality: Research and practice. Springer Science & Business Media. Berlin, Germany, doi:10.1007/978-3-642-36257-6
Saleh, T., Brock, J., Yousif, N., and Luers, A. (2013). The age of digital ecosystems: Thriving in a world of Big Data. BCG IT Advant., 33–36.
Sarikaya, A., Correll, M., Bartram, L., Tory, M., and Fisher, D. (2018). What do we talk about when we talk about dashboards? IEEE Trans. Vis. Comput. Graph. 25 (1), 682–692. doi:10.1109/TVCG.2018.2864903
Schomm, F., Stahl, F., and Vossen, G. (2013). Marketplaces for data: An initial survey. ACM SIGMOD Rec. 42 (1), 15–26. doi:10.1145/2481528.2481532
Schroeder, A., Naik, P., Bigdeli, A. Z., and Baines, T. (2020). Digitally enabled advanced services: A socio-technical perspective on the role of the internet of things (IoT). Int. J. Operations Prod. Manag. 40 (7/8), 1243–1268. doi:10.1108/IJOPM-03-2020-0131
Shannon, C. E. (1948). A mathematical theory of communication. Bell Syst. Tech. J. 27 (3), 379–423. doi:10.1002/j.1538-7305.1948.tb01338.x
Shapiro, C., Varian, H. R., and Carl, S. (1998). Information rules: A strategic guide to the network economy. Massachusetts, MA: Harvard Business Press.
Shen, Y., Guo, B., Shen, Y., Duan, X., Dong, X., and Zhang, H. (2016). A pricing model for big personal data. Tinshhua. Sci. Technol. 21 (5), 482–490. doi:10.1109/tst.2016.7590317
Shen, Y., Guo, B., Shen, Y., Duan, X., Dong, X., and Zhang, H. (2019). Pricing personal data based on information entropy. Proc. ACM Int. Conf., 143–146. doi:10.1145/3305160.3305204
Shen, Y., Guo, B., Shen, Y., Wu, F., Zhang, H., Duan, X., et al. (2019). Pricing personal data based on data provenance. Appl. Sci. 9 (16), 3388. doi:10.3390/app9163388
Spiekerman, M. (2019). Data marketplaces: Trends and monetisation of data goods. Intereconomics 54 (4), 208–216. doi:10.1007/s10272-019-0826-z
Stahl, F., and Vossen, G. (2017). Name your own price on data marketplaces. Informatica 28 (1), 155–180. doi:10.15388/Informatica.2017.124
Suppatvech, C., Godsell, J., and Day, S. (2019). The roles of internet of things technology in enabling servitized business models: A systematic literature review. Ind. Mark. Manag. 82, 70–86. doi:10.1016/j.indmarman.2019.02.016
Taleb, I., Serhani, M. A., Bouhaddioui, C., and Dssouli, R. (2021). Big data quality framework: A holistic approach to continuous quality management. J. Big Data 8 (1), 76–41. doi:10.1186/s40537-021-00468-0
Tang, R., Wu, H., Bao, Z., Bressan, S., and Valduriez, P. (2013). The price is right. Proc. Int. Conf. Database Expert Syst. Appl. 8056, 380–394. doi:10.1007/978-3-642-40173-2_31
Teece, D. J., and Linden, G. (2017). Business models, value capture, and the digital enterprise. J. Organ. Des. 6 (1), 8–14. doi:10.1186/s41469-017-0018-x
Thomas, L. D. W., and Leiponen, A. (2016). Big data commercialization. IEEE Eng. Manag. Rev. 44 (2), 74–90. doi:10.1109/EMR.2016.2568798
Tian, Y., Ding, Y., Fu, S., and Liu, D. (2022). Data boundary and data pricing based on the shapley value. IEEE Access 10, 14288–14300. doi:10.1109/ACCESS.2022.3147799
Voss, C., Tsikriktsis, N., and Frohlich, M. (2002). Case research in operations management. Int. J. Operations Prod. Manag. 22 (2), 195–219. doi:10.1108/01443570210414329
Wang, R. Y., and Strong, D. M. (1996). Beyond accuracy: What data quality means to data consumers. J. Manag. Inf. Syst. 12 (4), 5–33. doi:10.1080/07421222.1996.11518099
Wilkinson, M. D., Dumontier, M., Aalbersberg, I. J., Appleton, G., Axton, M., Baak, A., et al. (2016). The FAIR Guiding Principles for scientific data management and stewardship. Sci. data 3 (1), 160018–160019. doi:10.1038/sdata.2016.18
Witell, L., and Löfgren, M. (2013). From service for free to service for fee: Business model innovation in manufacturing firms. J. Serv. Manag. 24 (5), 520–533. doi:10.1108/JOSM-04-2013-0103
Woroch, R., and Strobel, G. (2022). Show me the Money: How to monetize data in data-driven business models? https://aisel.aisnet.org/wi2022/digital_business_models/digital_business_models/13.
Yang, J., Zhao, C., and Xing, C. (2019). Big data market optimization pricing model based on data quality. Complexity 2019, 1–10. doi:10.1155/2019/5964068
Yu, H., and Zhang, M. (2018). Data pricing strategy based on data quality. Comput. Industrial Eng. 112, 1–10. doi:10.1016/j.cie.2017.08.008
Zambetti, M., Adrodegari, F., Pezzotta, G., Pinto, R., Rapaccini, M., and Barbieri, C. (2021). From data to value: Conceptualising data-driven product service system. Prod. Plan. Control, 1–17. 34, 2, doi:10.1080/09537287.2021.1903113
Zhang, M., and Beltrán, F. (2020). A survey of data pricing methods. SSRN J. doi:10.2139/ssrn.3609120
Keywords: data monetization, data pricing, data quality, data entropy, data value, data marketplaces
Citation: Mendizabal-Arrieta G, Castellano-Fernández E and Rapaccini M (2023) A pricing model to monetize your industrial data. Front. Manuf. Technol. 3:1057537. doi: 10.3389/fmtec.2023.1057537
Received: 29 September 2022; Accepted: 09 January 2023;
Published: 30 January 2023.
Edited by:
David Romero, Monterrey Institute of Technology and Higher Education (ITESM), MexicoReviewed by:
Jiewu Leng, Guangdong University of Technology, ChinaCopyright © 2023 Mendizabal-Arrieta, Castellano-Fernández and Rapaccini. This is an open-access article distributed under the terms of the Creative Commons Attribution License (CC BY). The use, distribution or reproduction in other forums is permitted, provided the original author(s) and the copyright owner(s) are credited and that the original publication in this journal is cited, in accordance with accepted academic practice. No use, distribution or reproduction is permitted which does not comply with these terms.
*Correspondence: Gorka Mendizabal-Arrieta, Z21lbmRpemFiYWxAbW9uZHJhZ29uLmVkdQ==
Disclaimer: All claims expressed in this article are solely those of the authors and do not necessarily represent those of their affiliated organizations, or those of the publisher, the editors and the reviewers. Any product that may be evaluated in this article or claim that may be made by its manufacturer is not guaranteed or endorsed by the publisher.
Research integrity at Frontiers
Learn more about the work of our research integrity team to safeguard the quality of each article we publish.