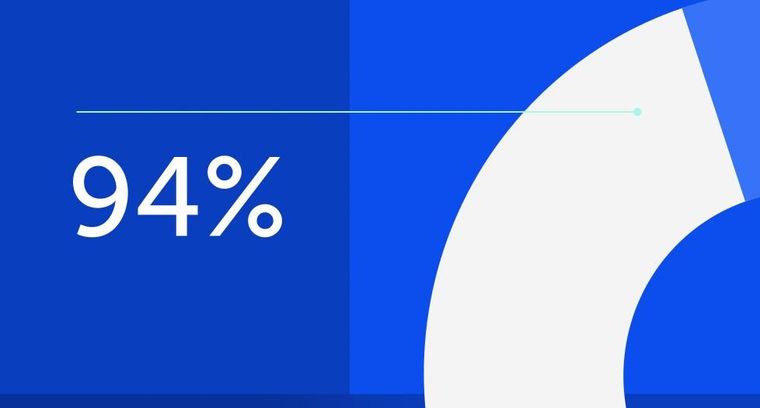
94% of researchers rate our articles as excellent or good
Learn more about the work of our research integrity team to safeguard the quality of each article we publish.
Find out more
ORIGINAL RESEARCH article
Front. Malar., 26 April 2024
Sec. Vectors
Volume 2 - 2024 | https://doi.org/10.3389/fmala.2024.1347790
This article is part of the Research TopicWomen in Malaria ResearchView all 14 articles
Introduction: Alternative splicing (AS) is a highly conserved mechanism that allows for the expansion of the coding capacity of the genome, through modifications of the way that multiple isoforms are expressed or used to generate different phenotypes. Despite its importance in physiology and disease, genome-wide studies of AS are lacking in most insects, including mosquitoes. Even for model organisms, chromatin associated processes involved in the regulation AS are poorly known.
Methods: In this study, we investigated AS in the mosquito Anopheles gambiae in the context of tissue-specific gene expression and mosquito responses to a Plasmodium falciparum infection, as well as the relationship between patterns of differential isoform expression and usage with chromatin accessibility changes. For this, we combined RNA-seq and ATAC-seq data from A. gambiae midguts and salivary glands, infected and non-infected.
Results: We report differences between tissues in the expression of 392 isoforms and in the use of 247 isoforms. Secondly, we find a clear and significant association between chromatin accessibility states and tissue-specific patterns of AS. The analysis of differential accessible regions located at splicing sites led to the identification of several motifs resembling the binding sites of Drosophila transcription factors. Finally, the genome-wide analysis of tissue-dependent enhancer activity revealed that approximately 20% of A. gambiae transcriptional enhancers annotate to a differentially expressed or used isoform, and that their activation status is linked to AS differences between tissues.
Conclusion: This research elucidates the role of AS in mosquito vector gene expression and identifies regulatory regions potentially involved in AS regulation, which could be important in the development of novel strategies for vector control.
Alternative splicing (AS) is an important source of phenotypic variation in eukaryotes that allows the generation of multiple transcripts from a single gene, expanding the coding capacity and functionality of the genome. AS regulates gene expression by acting on mRNA transcripts at a post-transcriptional level and is critical for diverse cellular processes, including cell differentiation and development as well as cell reprogramming and tissue remodeling. AS is mediated by four types of basic events: alternative 5′ splice-site, alternative 3′ splice-site, cassette-exon inclusion or skipping, and intron retention. These processes can be combined to obtain dozens of isoforms from a single gene. On the other hand, AS regulation includes different mechanisms such as the temporal and spatial differential expression of the isoforms, the activation of the RNA Pol II, or the on–off regulation by nonsense-mediated decay (Wang and Cooper, 2007; Nilsen and Graveley, 2010; Kelemen et al., 2013; Shenasa and Hertel, 2019; Ule and Blencowe, 2019; Wright et al., 2022). Another mechanism associated with AS regulation is isoform usage, which is defined as the participation of each isoform in gene expression. Therefore, when these proportions switch between two conditions, some isoforms are considered to be differentially used (Vitting-Seerup and Sandelin, 2017; Merino et al., 2019; De La Fuente et al., 2020; Li et al., 2020; Chen et al., 2022). As a result, the function of a gene might change because different variant proteins are produced, while the total gene expression can remain unaltered.
AS is highly conserved in eukaryotes, however, the type of events and mechanisms of AS vary significantly among species (Nilsen and Graveley, 2010; Kelemen et al., 2013; Martinez and Lynch, 2013; Bush et al., 2017; Vitting-Seerup and Sandelin, 2017; Ule and Blencowe, 2019; Zhao, 2019; Li et al., 2020; Song et al., 2020; Verta and Jacobs, 2022; Wright et al., 2022). A simple way to study AS is to quantify and compare the expression of isoforms that are generated in different tissues or experimental conditions (Katz et al., 2010; Glaus et al., 2012). Transcriptomic data obtained by RNA-seq is mostly used for gene-level analysis (Vitting-Seerup and Sandelin, 2017; Zhao, 2019), but it also offers the possibility to study AS, analyzing and counting known transcripts or detecting new splicing events (Zhao, 2019).
The regulation of AS is intrinsically related to chromatin dynamics. To obtain diverse transcripts, cis- and trans-regulatory elements participate both in the generation of transcripts and in the marking of splice sites (Bush et al., 2017; Shenasa and Hertel, 2019; Zhao, 2019; Verta and Jacobs, 2022). In addition, AS regulation can be affected by posttranslational histone modifications, nucleosome positioning, and chromatin accessibility (Davuluri et al., 2008; Luco et al., 2011; Montes et al., 2012; Klemm et al., 2019; Nussinov et al., 2021). However, how these different epigenetic layers interact and how they relate with the type of events and AS regulation, as well as the great diversity of gene functions through AS, have been poorly investigated (Davuluri et al., 2008; Luco et al., 2011; Klemm et al., 2019). The integrated analysis of transcriptomic and epigenomic data could provide new insights into how AS is regulated and how it changes under different conditions.
AS has been widely studied in model organisms like Drosophila melanogaster, Danio rerio, or Caenorhabditis elegans (Zahler, 2005; Venables et al., 2012; Lin et al., 2022). In Drosophila melanogaster, AS plays important roles in sex determination or muscle and neural development, among other biological processes (Venables et al., 2012). More precisely, the relationship between AS and chromatin has been investigated in Drosophila gonads. In that study, it was reported that the enrichment of several splicing factors in the undifferentiated cells coincided with highly expressed chromatin-remodeling factors and histone-modifying enzymes, which would act in concert to maintain the transcription network through post-transcriptional mechanisms (Gan et al., 2010). Despite these scarce studies, there is a lack of knowledge on how the AS interacts with chromatin to regulate gene function under different physiological or cellular conditions (Nilsen and Graveley, 2010; Luco et al., 2011; Kelemen et al., 2013).
In mosquitoes, little is known about AS and its regulation. Given that AS is evolutionarily conserved, one would expect that functions and mechanisms are maintained across insects, particularly in Diptera. There is, however, a marked inter-species variation in alternative splicing that probably resulted from selective pressures exerted by factors such as the environment and lifestyle (Kurtz and Armitage, 2006; Malko et al., 2006). In Aedes aegypti, previous studies have focused on sex differentiation (Salvemini et al., 2011, 2013; Calkins et al., 2015). In the human malaria mosquito Anopheles gambiae, the study of AS is limited to a few genes, such as doublesex and Dscam (Tsujimoto et al., 2013; Krzywinska et al., 2016; Sinkins, 2016; Djihinto et al., 2022), to tissues like salivary glands (Dixit et al., 2009), and to biological functions such as signaling pathways (Meister et al., 2005). These studies suggest that AS could play a crucial role in life history traits that are relevant in the context of a malaria infection, such as vector competence and mosquito immunity. However, a more systematic analysis addressing the role of AS and its regulation in mosquitoes is lacking.
In earlier studies, we combined transcriptomic and chromatin accessibility data of infected and non-infected A. gambiae salivary glands and midguts aiming at identifying cis-regulatory networks involved in tissue-specific gene expression regulation and mosquito responses to the malaria parasite infection (Ruiz et al., 2019, 2021). In this study, we used these previous datasets and new data to investigate the differential expression and usage of isoforms belonging to multisoform genes and their utilization across various mosquito tissues infected with the malaria parasite Plasmodium falciparum. We also investigated AS regulation by addressing the potential involvement of chromatin-associated mechanisms in these processes (Love et al., 2014; Vitting-Seerup et al., 2019). We contend that a deeper understanding of the mechanisms of AS regulation can reveal key cis- and trans-regulatory elements, like splicing transcription factors and enhancers, involved in mosquito responses to P. falciparum infection and/or in vector competence.
The external RNA-seq data analyzed in this study was downloaded from GEO accession number GSE120076 and corresponded to infected and non-infected midgut samples (Ruiz et al., 2019). The GEO accession number GSE152924 corresponds to previously published ATAC-seq data of infected tissues, both midguts and salivary glands (Ruiz et al., 2021).
This study includes new RNA-seq data from infected and non-infected salivary glands (two paired samples/infections). These were obtained in the same experiment as the samples/data in Ruiz et al. (2019). In brief, to obtain Non-Inf vs. Inf SG, 2- to 5-day-old female A. gambiae s.s. mosquitoes from a genetically outbred laboratory colony at the “Institut des Sciences et Techniques (INSTech)” of Burkina Faso were fed through membranes on gametocyte-infected blood from malaria patients or, in the case of controls, on the same blood after heat treatment to eliminate the parasite. Dissection of the salivary glands was performed 14 days after the infection. Tissues were immediately processed for RNA analyses. Total RNA was extracted using mirVana™ RNA Isolation Kit (Ambion®) according to the manufacturer’s protocol and used for mRNA library preparation. RNA concentration was quantified using Qubit® 2.0 Fluorometer, and RNA integrity was determined with Agilent 2100 Bioanalyzer. Illumina libraries were prepared and sequenced at the HudsonAlpha Institute for Biotechnology, using an Illumina HiSeq 2000 sequencer, standard directional RNA-seq library construction, and 50-bp paired end reads with ribosomal reduction (RiboMinus™ Eukaryote Kit, Ambion®).
To study the role of AS in A. gambiae, we considered genes that are susceptible to be regulated by this mechanism. Only 1,263 genes annotated in the A. gambiae genome (13,094 genes) have more than one transcript and could be classified as multisoform genes. We used the Anopheles gambiae PEST reference genome obtained from Vectorbase release 54 (Giraldo-Calderón et al., 2015).
Overall, considering the information about the datasets in the previous section, we used 12 RNA-seq and four ATAC-seq datasets corresponding eight A. gambiae-infected (Inf) and four non-infected (control, Non-Inf) samples from two mosquito tissues (midguts, MG, and salivary glands, SG) (Ruiz et al., 2019, 2021). Our experimental design distributed the samples in three different comparisons: (1) non-infected vs. infected midguts, (2) non-infected vs. infected salivary glands, and (3) infected midguts vs. infected salivary glands. All of these included two replicates per condition.
Quality control of RNA-seq data was performed with FastQC v0.11.9 (Babraham Bioinformatics, n.d). We used SortMeRNA v4.2.0 (Kopylova et al., 2012) to determine and eliminate possible ribosomal RNA contamination. Filtered reads were trimmed 10 bp using bbduk.sh v38.18 (Bushnell, 2014) and mapped to the reference genome (Anopheles gambiae PEST genome version P4.12) available in VectorBase release 54 (Giraldo-Calderón et al., 2015) using Salmon v1.5.2 (Patro et al., 2017). This software is specifically used for the alignment and quantification of isoforms (Zhang et al., 2016) and was run using the default parameters -l A –validateMappings –go 4 –mismatchSeedSkip 5. We used the aligner STAR v2.7.8 to map the reads against the reference genome (Dobin et al., 2013) and visualized coverages in IGV (Thorvaldsdóttir et al., 2013). After this step, we also performed a quality control of the STAR alignments with Qualimap v2.2.2 (Okonechnikov et al., 2016). This quality control included the assessment of 5′ and 3′ coverage biases in our data. Supplementary Table S1 contains information about the number of raw reads, the results of the filtering by SortMeRNA, the quality control of the alignments, and the mapping statistics. Replicability analyses based on principal coordinates analyses (PCoA) and unsupervised hierarchical clustering were performed on log2 normalized counts by Salmon using the quality control module of the reanalyzerGSE pipeline (Ruiz et al., 2023). These analyses supported the use of the samples as replicates in the differential analyses (Figure 1; Supplementary Figure S1).
Figure 1 Principal coordinate analyses and unsupervised hierarchical clustering analyses for the comparison between infection midguts and infection salivary glands. The graphs illustrates the similarity between the groups of samples. Samples from similar experimental infections tend to group within the same condition compared to those between different conditions.
The counts generated by Salmon were used to perform various analyses. First, to study the most prevailing alternative splicing mechanisms in each condition, we used the R package IsoformSwitchAnalyzeR v2.2.0 (Vitting-Seerup et al., 2019). We applied the function analyzeAlternativeSplicing with the argument onlySwitchingGenes=F. We then compared the frequencies of the AS events between conditions (tissues and infection status) to determine how are they altered in the different comparisons (see Section 2.2).
The differential expression analyses were performed using the R package (v4.3.2) DESeq2 (v1.42.0) (Love et al., 2014). Taking only the set of multisoform genes (see Section 2.2), we considered isoforms corresponding to differentially expressed multisoform genes (DEMG), those that have differential expression between conditions (the isoform not the gene). To be differentially expressed, isoforms must have a significant P-adjust of less than 0.05 and a log2 fold change (FC) greater than 1 or less than −1 (corresponding to a 2× change). In the comparison Inf MG vs. Inf SG, a FC <0 means overexpression in MG, whereas a FC >0 means overexpression in SG. In the other two comparisons, Non-Inf vs. Inf at each tissue, a FC >0 means more expression in the infected tissue. For this analysis, only isoforms with a count value greater than 10 were considered to be expressed. A total number of 1,561 (Inf MG vs. Inf SG comparison), 1,394 (Non-Inf vs. Inf MG), and 1,679 (Non-Inf vs. Inf SG) isoforms passed this threshold and were included in all subsequent analysis.
The study of the differential usage of the isoforms was performed using IsoformSwitchAnalyzeR v2.2.0 (Vitting-Seerup et al., 2019). In this case, we used the parameter difference in isoform fraction (dIF) to measure the magnitude of change. The isoform fraction (IF) is a measurement that determines the proportion of the total expression of a parent gene that comes from a particular isoform (isoform_exp/gene_exp). Differentially used isoforms (DUI) were those with a dIF >0.1 or dIF <−0.1 (a threshold of 10% of change). We also applied a significance threshold of P-adjust (FDR) <0.05. In the comparison between Inf MG vs. Inf SG, dIF <0 means more usage in MG, and dIF >0 means more usage in SG. In the other two comparisons, Non-Inf vs. Inf at each tissue, dIF <0 means more usage in the infected tissue. For this analysis, only isoforms with a count value greater than 1 were considered to be expressed and included in subsequent analyses. A total number of 2,386 (Inf MG vs. Inf SG comparison), 1,913 (Non-Inf vs. Inf MG), and 2,406 (Non-Inf vs. Inf SG) isoforms passed this threshold and were included in all subsequent analyses. We performed Gene Ontology (GO) terms overrepresentation tests for the sets of differentially expressed or used multisoform genes in the comparison Inf MG vs. Inf SG using VectorBase Overrepresentation Test, and we chose a threshold of P-value <0.05. We also used the ImmunoDB database to assess whether the isoforms could be immunologically relevant based on the presence of the coding gene in this database (EZlab | Computational Evolutionary Genomics group, n.d; Giraldo-Calderón et al., 2015).
For the analysis of ATAC-seq data in relation to AS, we used the processed data from our article (Ruiz et al., 2021), which includes the nucleosome-free reads, the ATAC peaks resulting from the analysis with MACS2 (Zhang et al., 2008), and the differentially accessible peaks resulting from the analysis with DiffBind (Stark and Brown, 2011).
The relationship between the gene expression and chromatin structure has been examined in a few model organisms (Luco et al., 2011). These previous studies showed that various epigenetic mechanisms such as nucleosome positioning, post-transcriptional modifications (Luco et al., 2011), or the modification of chromatin accessibility (Li et al., 2020; Nussinov et al., 2021) alter or correlate with AS dynamics. In A. gambiae, our earlier work investigated the relationship between chromatin accessibility and gene expression regulation at the gene level (Ruiz et al., 2021), while here we focused on the expression and potential gene regulation occurring at the isoform level via alternative splicing.
Our hypothesis was to find a positive relationship between chromatin accessibility differences and the differential expression and/or usage of the isoforms. For the differential isoform expression (DEMG), we expected to find increased accessibility in the promoter of one or more isoforms in the tissue where the expression of the isoform/s is higher (Figure 2A). For the differential isoform usage (DUI), we expected to find changes in chromatin accessibility at regulatory regions coinciding or near alternative splicing sites in the isoform more used in each tissue, as shown in Figure 2B—for example, for an exon skipping event, we would expect more accessibility in the surroundings of the exon to be added to the transcript at the condition with more usage of the isoform.
Figure 2 Model for the relationship between isoform expression, isoform usage, and chromatin accessibility. The ATAC-seq peaks are shown in red. (A) For the DEMG group, the expectation is that the accessibility of the promoter will increase in the tissue where the expression of the isoform is higher, i.e., the RA isoform in midguts display a higher ATAC-seq peak. For the RB isoform, the expression is higher in salivary glands, and accordingly the accessibility peak is higher in this tissue. (B) For the DUI group, we expect to find chromatin accessibility changes near the splicing sites coinciding with the different usage of the isoform/s. The ATAC-seq peaks are around the differential exons and are higher in the tissues where the isoforms are more used. The RA isoform is less expressed in midguts and becomes more expressed in salivary glands, displaying a higher ATAC-seq peak in this tissue.
To test the possible relationship between the differential expression of isoforms and chromatin accessibility, we first discarded genes that overlap by more than 25% with other genes. We used Spearman’s correlation test to analyze the possible association between isoform expression and chromatin accessibility at various regions where the AS mechanisms would operate. These include the isoform promoter, which we considered to comprise 1 kbp from the TSS (the end of the 5′ UTR), or the isoform body, which includes all exons and introns. For those isoforms that lack annotated TSS, we considered the ATG as the reference point. A density plot of the ATAC peaks downloaded from Ruiz et al. (2021) was elaborated using R package ggplot2 v3.4.4 (Wickham, 2016) and confirmed that the majority of chromatin accessibility would be located at the promoter of genes (Supplementary Figure S2).
We used R script (see data and code availability section) to classify the multisoform genes into three groups—high, medium, and low—depending on the expression level of the isoforms. The threshold values for this classification were determined by dividing the signal into three quantile groups based on their means using the cut2 function from the R package Hmisc. The software ngsplot was used to visualize as average profile plots the chromatin accessibility (nucleosome-free region signal) at the TSS in the different groups of isoforms classified by their expression level (high, medium, and low) (Shen et al., 2014).
In the case of differentially used isoforms, we analyzed the association between isoform usage and chromatin accessibility focusing on alternative splicing sites. To do this, we first searched for significant differential peaks between conditions, previously obtained by DiffBind in Ruiz et al. (2021), that coincide with A. gambiae known splicing sites (SS), adding a small distance to see what is happening around these sites. We set a restrictive window of ±100 bp, and we counted how many of those peaks annotate to isoforms classified as differentially used (DUI).
On those peaks, we then performed a motif analysis using HOMER software v4.11 (Heinz et al., 2010). We used findMotifGenome.pl to determine known and de novo motifs overrepresented in our sequences and then used annotatePeaks.pl to link the motifs back to the differentially used isoforms and the splicing sites. Only significant motifs with P-values <1e-20 were considered. HOMER employs two distinct sets of sequences during motif discovery. The first set comprises target sequences of interest, and the second set consists of background sequences, typically mostly not subjected to regulation. Subsequently, it uses a cumulative hypergeometric distribution to detect over-representation in the target set relative to the background set. In our analysis, the background sequences were automatically and randomly chosen from the genome (HOMER attempts to select background regions that match the GC-content distribution of the input sequences).
We used the set of A. gambiae enhancers that was characterized in our previous integrative analysis of ATAC-seq and RNA-seq data (Ruiz et al., 2021). We aimed to investigate whether these regulatory sequences could be involved in AS events and also compared the occurrence of enhancers in multisoform vs. non-multisoform genes. The list included 1,402 A. gambiae enhancers predicted by homology with Drosophila enhancers and 2,866 Anopheles enhancers identified either computationally or experimentally by different studies (Ruiz et al., 2021). We selected only the enhancers that appeared to be active in at least one of our conditions—that is, enhancers that were reported in Ruiz et al. (2021) to have a MACS2 chromatin accessibility peak and appeared to be enriched in H3K27ac. As a result, we obtained a list of 807 active enhancers which we overlapped with our differentially expressed (DEMG) and differentially used (DUI) isoforms using BEDtools intersect v2.30.0 (Quinlan and Hall, 2010). We also used this tool to classify these enhancers as intragenic or intergenic and intersect them with A. gambiae splicing sites.
Finally, we quantified which portion of the active enhancers that are annotated to DEMG and DUI genes display a change in accessibility—that is, which display differential accessibility between tissues (DiffBind region) coinciding with the change in the expression of the isoform or isoforms.
Considering the 1,263 multisoform genes detected in the A. gambiae genome (see “Experimental design“), we aimed to identify alternative splicing events and their role in gene expression regulation in different tissues and infection conditions. For this, we performed two types of analysis: one to identify differentially expressed isoforms belonging to multisoform genes (DEMG) and another one to detect isoforms that were differentially used (DUI), which are isoforms that are altered in their proportion relative to the total expression of the gene. First, we performed replicability analyses to verify that our samples, which are inherently heterogeneous (different mosquito tissues and Plasmodium infections), could be used as biological replicates. In the case of the comparison between tissues, there was a clear grouping pointing to the differences between tissues (Figure 1). In the case of the other two comparisons (non-infected vs. infected at each tissue independently), the samples displayed more variability, but their clustering still supported the differential analyses (Supplementary Figure S1).
In the comparison between P. falciparum-infected and non-infected mosquito midguts, 11 isoforms appeared to be differentially regulated out of the 848 multisoform genes present in this dataset (see “Experimental design“). Of these, four (0.4%) corresponded to differentially expressed (DEMG) and seven (0.8%) to differentially used isoforms (DUI). In the comparison of infected and non-infected salivary glands, we detected 977 to be expressed, of which 24 isoforms appear differentially regulated: two (0.2%) were DEMG and 22 (2.2%) DUI. We then examined differences in AS regulation between tissues (Inf MG vs. Inf SG), and we detect 920 multisoform genes present in this dataset: 392 isoforms (42.6%) DEMG and 247 isoforms (26.8%) DUI. We also report multisoform genes in which one or more isoforms were at the same time DEMG and DUI (Figure 3A). In our results, this overlap between AS mechanisms involved 7.5% (86 isoforms belonging to 69 multisoform genes) of the total 920 multisoform genes that display AS when comparing Inf MG vs. Inf SG (Figure 3B) and less than 1% (one for the midguts and one for the salivary glands) when comparing non-infected vs. infected mosquito tissues. Supplementary Tables S2, S3 present the results of the DEMG and DUI analyses, respectively.
Figure 3 Patterns of differentially expressed isoforms belonging to multisoform genes (DEMG) and differentially used isoforms between tissues. (A) Diagram illustrating possible DEMG and DUI patterns expected in our data. The gene can be differentially expressed and the isoform differentially expressed but not differentially used; only one more of the isoforms differentially used and the isoforms can be differentially expressed and used at the same time. (B) Volcano plot showing total usage change (i.e., expression redistribution between isoforms) versus the log-transformed values of isoform expression fold change between tissues. The right-hand side of the graph corresponds to logFC values >0 and therefore isoforms differentially expressed in salivary glands. The left part represents isoforms differentially expressed in midguts with logFC <0. Isoforms that are DUI and DEMG are shown in red, whereas black dots correspond to differentially expressed isoforms. Labels identify isoforms corresponding to A. gambiae genes with immune functions. (C) IGV representation of the AGAP005203 (PGRPLC) gene. Red lines highlight differential expression and accessibility at RA and RE differential exons. The red box on the left points to a higher expression of the RA isoform in midguts, while the box on the right points to a higher expression in salivary glands.
To test for the differential functionality of DEMG and DUI isoforms between tissues, we performed GO enrichment analysis. For the differentially expressed isoforms that appear more expressed in salivary glands, the 10 first GO terms (ordered by P-value) include metabolic processes, regulation of response to stimulus, and signaling. On the contrary, differentially expressed isoform genes that are more expressed in midguts showed enriched functions to be involved in fiber and cytoskeleton organization, ion transport, and muscle contraction (Supplementary Table S4). In the case of differentially used isoforms that appear overused in midguts, the 10 first GO terms correspond to functions related to homeostatic and metabolic processes. In salivary glands, overused isoforms displayed functions like metabolism, homeostasis, and inorganic ion transmembrane transport (Supplementary Table S4).
Next, we described the types of events that lead to differential isoform expression (DEMG) and/or isoform usage (DUI) in our set of A. gambiae multisoform genes. In our data, the alternative splicing event more frequently appeared to be exon skipping (ES) (Li et al., 2020), followed by alternative star site (ATSS). For DEMG, we report differences in the frequency of these events between conditions only for ES: 56 ES events in Inf MG vs. 83 ES events in Inf SG. For DUI, only the frequency of ES is moderately altered when comparing Inf MG vs. Inf SG (43 ATSS events in MG compared to 56 events in SG). These results are summarized in Supplementary Table S5. Conforming to the more frequent ES events, we would expect chromatin accessibility enrichment (ATAC peaks) to be located in the body of the isoform/s involved in the ES event (see the next section). Alternatively, this association could also be evident in the promoter of isoforms that result from other AS events such as ATTS or intron retention (IR) (Li et al., 2020).
Finally, we interrogated whether our DEMG and DUI coincided with genes known to be involved in mosquito immunity (Sreenivasamurthy et al., 2013). We found eight genes among our differentially expressed and used isoforms that encode for proteins involved in anti-Plasmodium mosquito responses (for example, STAT, PGRPLC, and OXR1). Supplementary Table S6 shows the information of the overlapping with the ImmunoDb database (Sreenivasamurthy et al., 2013). A relevant example includes the AGAP005203-RA and AGAP005203-RD isoforms of the PGRPLC gene, identified in the present work as differentially expressed (RA) and used (RA and RD). Both isoforms appeared to be overexpressed during midgut infection, while RC and RE appeared to be similarly expressed. RB and RF isoforms displayed values below the expression threshold, so they were considered as non-expressed (Figure 3C).
First, we tested if a higher expression of the isoforms was associated with higher levels of chromatin accessibility in the isoform promoter (1 kb upstream of the TSS/ATG of the isoform) or the isoform body.
The results for the DEMG group show a positive and significant correlation between isoform expression and chromatin accessibility for the isoform body, both in salivary glands (rho = 0.30, p-value = 3.94e-05) and midguts (rho = 0.348, p-value = 1.42e-05), but not for the promoter region (Supplementary Table S7; Figure 4). Next, we examined whether the relationship was quantitative, that is, if the levels of expression of isoforms differentially expressed (categorized as high, medium, or low; see “Experimental design”) correlated with the chromatin accessibility in the promoter of the isoforms. This relationship did not appear to be significant either in midguts nor salivary glands (rho < 0.15, p-value > 0.3 for all levels and tissues; Supplementary Table S7) (Figure 5).
Figure 4 Differential expression of the isoforms correlates with changes in chromatin accessibility between tissues in the isoform body. Heat map showing ATAC-seq nucleosome-free enrichment at promoters or in the body of DEMG isoforms and their expression levels. Genes are ordered by normalized RNA-seq isoform expression (RPKM). Changes tend to occur in the same direction, and there is a positive and significant correlation between the magnitude of changes in accessibility and expression at the isoform body in both tissues.
Figure 5 Profile plots (up) and violin plots (down) showing changes in ATAC-seq nucleosome-free signal enrichment at each tissue with respect to the TSSs (± 1 kb). Genes are divided into groups and ranked by their mRNA levels (high, medium, or low). In the violin plots, the width of the graph takes into account the density of repeated values in the interval. The mean values are marked with a black dot.
For the analysis of chromatin accessibility in relation to the DUI group, we used the processed data of differential accessibility peaks previously published by us (Ruiz et al., 2021). We selected DiffBind peaks that were located at the splicing site or in the region nearby (± 100 bp) and that annotated to differentially used isoforms. We reported a clear association between differential usage and accessibility, with 98 differentially used isoforms (39.6% of the total number of isoforms differentially used) displaying a DiffBind peak located at or nearby splicing sites (see the list in Supplementary Table S8).
We performed a motif analysis using HOMER on the set of DiffBind peaks that annotated to differentially used isoforms and located at or nearby the splicing sites. The purpose of this analysis was to detect overrepresented motifs that point to transcription factors that may be involved in mosquito AS regulation.
Using the hierarchical clustering of MEME de novo motif discovery option, several motif hits with distinct similarities with Drosophila sequences appeared to be overrepresented (Supplementary Table S9). A summary of the de novo motifs can be found in Table 1. For the subset of differentially used isoforms, we found de novo motifs including brachyentron (byn), which is implicated in midgut development, zeste (z), which helps to recruit the BRM nucleosome-remodeling complex, or dorsal (dl), which encodes a transcription factor that works downstream of the Toll pathway or chromatin remodelers like the chromatin-linked adaptor for MSL proteins (CLAMP). Analyzing the known motifs, we found only one motif to be overrepresented, NTCAGTYG, which matched with an initiator of the Drosophila promoters (Supplementary Table S9).
Table 1 Ten first de novo motifs identified by HOMER that appear overrepresented in the splicing sites of differentially expressed and used isoforms that display differential accessibility between tissues (additional information in Supplementary Table S9).
We aimed to study if the previously identified A. gambiae transcriptional enhancers participate in the regulation of AS. Such a relationship has been shown in human and mouse, in which 5% of the enhancers appear associated to AS changes (Shiau et al., 2021). For this analysis, we used A. gambiae enhancers from our previous study (Ruiz et al., 2021). This dataset included 4,268 enhancers and the corresponding annotated genes. These were identified computationally through homology with Drosophila enhancers or experimentally discovered by others in mosquitoes and validated by our transcriptomic and epigenomic data (gene expression changes between tissues, chromatin accessibility, and histone modification marking) (Ruiz et al., 2021). Of these mosquito enhancers, 807 appeared to be active in our dataset, that is, annotate to one or more differentially expressed or used A. gambiae multisoform genes.
Considering the group of differentially expressed isoforms, we found a total of 109 accessible (active) enhancers that annotate to 61 genes (19.8% of the 307 multisoform genes present in the DEMG group) (Supplementary Table S10). Out of these, 22 enhancers (21.94%) overlap with a Diffbind peak, thus being differentially accessible between infected midguts and salivary glands.
For the group of differentially used isoforms, we identified 46 accessible enhancer regions that annotate to 26 genes (17.9% of the 145 multisoform genes present in the DUI group) (Supplementary Table S10), of which 12 exhibit Diffbind peaks, indicating differential accessibility between infected midguts and salivary glands. Lastly, we examined the location of the accessible enhancers that annotate to both differentially expressed and used isoforms. For the DEMG group, the number of these enhancers that were intragenic almost doubled the number of intergenic (38 intergenic and 71 intragenic enhancers), while in the DUI group the numbers were more similar (21 intergenic and 25 intragenic enhancers). More importantly, 19 enhancers out of the 807 (2.3% of the total) overlapped by at least 25% of the region of the splicing sites of our DEMG and DUI.
Next, to further validate the existence of an association between enhancers and differentially expressed isoforms, we analyzed the occurrence of enhancers in non-multisoform genes that are not subjected to alternative splicing. We identified 707 accessible enhancers associated with 510 genes (4.61% of the 11,060 non-multisoform genes). In this set, the number of intragenic enhancers was very similar to the intergenic (341 and 366, respectively). When examining the presence of DiffBind peaks within these enhancers, we observed that 17.68% (125 out of the 707 active enhancers) exhibited such peaks. In addition, 23 out of the 707 enhancers (2.8% of the total) overlapped, by at least 25% of the region, with the predicted splicing sites of our genes. Considering the number of genes corresponding to these enhancers, only 0.3% of them showed the overlap (30 out of 11,060 genes), whereas the percentage is much higher, 5.21% in DEMG and 4.8% in DUI genes (16 out of 307 DEMG genes and seven out of 145 in DUI).
The above-mentioned results altogether suggest that in the groups of isoforms mentioned above, enhancers, particularly intragenic and affecting differential expression rather than use, appear to be more prevalent than in non-multisoform genes. Furthermore, a fraction of enhancers also overlapped known splicing sites, and almost 20% of our isoforms displayed association with a previously described enhancer.
One example is the gene AGAP005234 that encodes for copper–zinc superoxide dismutase. This protein has functions involved in mosquito immunity, including anti-Plasmodium activity, as well as the catalysis of oxygen radicals (Marikovsky et al., 2003; Molina-Cruz et al., 2008). The gene contains two different isoforms (AGAP005234-RA and AGAP005234-RB). The RB isoform was more differentially expressed and used in salivary glands, and RA was more differentially used in midguts. The enhancer associated to this gene was intergenic, located 2 kbp upstream from the start of the gene, and its accessibility was higher in salivary glands, coinciding with a higher expression and use in salivary glands (Figure 6).
Figure 6 Enhancer function and alternative splicing of the gene AGAP005234. (A) Scheme summarizing gene and isoform expression between tissues. (B) IGV screenshot showing the enhancer annotated to the AGAP005234 gene which is differentially accessible between tissues coinciding with the differential expression and usage of their isoforms. RB isoform is differentially expressed and used in salivary glands, and the enhancer is more accessible in this tissue. All tracks are shown at equal scale.
Alternative splicing has been little studied in mosquitoes, and the relationship of this process with chromatin dynamics remains mostly unknown. In this study, we investigated alternative splicing in A. gambiae and the role of chromatin structure in its regulation. We compared patterns of AS in different conditions: first, between two mosquito tissues (midguts and salivary glands) and, second, between P. falciparum-infected and non-infected (control) mosquito midguts and salivary glands.
The first part of this work aimed at identifying how prevalent is AS in mosquitoes and how AS patterns change between tissues and infection status. From a total of 1,263 A. gambiae genes susceptible of AS, we detected around 30% of them being differentially expressed or used at the level of isoforms. Here it is important to note that we detected some coverage biases in our sequencing data, specifically for the mean coverage at the 5′ region in the comparison between tissues. In the total RNA library preparation approach, a certain degree of read-density bias toward the 3′ end of transcripts would be expected (Menéndez-Arias et al., 2017), so the coverage biases that we report could be interpreted as an inherent technical limitation. Even so, the lower coverage that we obtained in certain regions could lead to false positives in the detection of AS events, such as those based on exon skipping or alternative transcription start site detections, but we expect them to be minimal. Another potential limitation is that the level of transcription could affect the sensitivity of AS detection—for example, previous studies have pointed out that alignment-free computational methods, such as salmon, may introduce noisiness in the differential splicing detection, more particularly in transcripts expressed at low levels (Varabyou et al., 2021). Similarly, in pre-mRNA splicing studies, longer or more expressed transcripts would be sampled with higher frequency, which may distort the statistical power of enrichment or differential tests (Dwyer and Pleiss, 2023). These issues, which unfortunately remain unsolved in the field, must be taken into account in any quantitative study of AS.
In this study, the reported differences appeared to be largely due to AS events at the tissue level. When comparing the two infected tissues, 42.6% of isoforms (392) appeared to be differentially expressed (DEMG) and 26.8% (247) differentially used (DUI), suggesting that AS plays an important role in regulating tissue-specific expression in A. gambiae. Indeed the role of AS in tissue-specific processes has been reported in different organisms like humans, D. melanogaster, and C. elegans (Wang et al., 2008; Telonis-Scott et al., 2009; Venables et al., 2012; Ragle et al., 2015) or even in the mosquito species Anopheles stephensi (Sreenivasamurthy et al., 2017). While in this study the AS patterns varied largely between tissues, differences were minimal when comparing infected and non-infected tissues for both differentially expressed and differentially used isoforms (only 11 DEMG and DUI isoforms detected in the case of midgut infections and 24 DEMG and DUI isoforms detected in the case of salivary gland infections). These results could be explained due to the larger inter-sample variability in these comparisons as revealed by our replicability analyses. This intrinsic heterogeneity may hinder the statistical power of our approach, decreasing the number of detected DUI and DEMG; so, our estimates could therefore be considered conservative. Alternatively, our results could be also due to a low involvement of AS events in the context of a Plasmodium infection in the mosquito.
Among relevant examples of multisoform genes with tissue-specific AS, we reported several genes with immune functions. An example is the AGAP005203 gene that encodes for a peptidoglycan recognition protein (PGRPLC) and is involved in the Imd pathway (Meister et al., 2009). This protein has been reported to be implicated in anti-Plasmodium responses at the level of the mosquito midgut, but without information about the functionality of their isoforms (Sreenivasamurthy et al., 2013). This gene consists of six isoforms corresponding to three different variants. The PGRPLC gene produces three primary protein isoforms, each with a distinct PGRP domain and an optional 75-nucleotide cassette located at the 3′ end of the shared exon 3. Consequently, each isoform is present in two forms: the longer version is 25 amino acids longer than the shorter version. In our results, AGAP005203 had two isoforms differentially expressed and used: RA corresponding to the shorter version of the variants and RD corresponding to the longer version. We reported the RA form to be overexpressed and overused during midgut infection, and this conforms with previous studies where its gene was proposed to be involved in the mosquito immune response against Plasmodium (Meister et al., 2009). The RD isoform was also overused in midguts, and although its function in this tissue is still unknown, a previous work reported that the RD and RE isoforms are positively regulated in response to stress and antimicrobial responses (Lin et al., 2007; Chen et al., 2009; Meister et al., 2009).
Chromatin dynamics and transcriptional regulation are intimately related, but its role in the regulation of alternative splicing remains little known. There are very few studies that have investigated how epigenetic mechanisms, such as histone modifications or nucleosome positioning, would contribute to changes in the AS patterns. This is despite the functional consequences that AS events can have during development and disease (Simon et al., 2014; Petrova et al., 2022). Previous evidence in model organisms reported local changes in nucleosome positioning, histone modifications, and chromatin accessibility near exons undergoing splicing (Naftelberg et al., n.d.; Chodavarapu et al., 2010; Li et al., 2020), but whether these mechanisms are conserved in non-model organisms, such as mosquitoes, remains obscure.
We proposed a model to describe the association between isoform expression and/or usage and chromatin structure dynamics in A. gambiae. For differentially expressed isoforms, we expected increased promoter accessibility in the tissue where the isoform was more expressed (Li et al., 2020; Ruiz et al., 2021). For differentially used isoforms, we expected accessibility changes associate to the differential use, but localized at or nearby AS regulatory regions (splicing sites). In agreement with this model, our results indicate that there is a relationship between the differential expression and the use of isoforms and the changes in chromatin accessibility enrichment, suggesting that chromatin structure plays a conserved role in AS-mediated gene expression regulation in A. gambiae. However, this relationship was supported by a significant correlation only in the region of the isoform body. These observations conform to previous studies in mammals and plants, which reported higher chromatin accessibility coinciding with the region of intron retention (Ullah et al., 2018; Petrova et al., 2022). In addition, changes in accessibility along an isoform body have been also shown to alter the speed of RNA polymerase II (RNA pol II) (Saldi et al., 2016) or, alternatively, to impact the recruitment of splicing factors through direct or indirect recognition of different chromatin marks (Schor et al., 2013). An increase in chromatin accessibility in histone-depleted genes was shown to alter the elongation rate of Pol II, resulting in several transcripts with increased intron retention and changes in alternative splicing (Jimeno-González et al., 2015).
For differentially used isoforms, our results conformed with our hypothesis that changes at the level of the splicing sites would be more important. We confirmed the existence of differential accessibility peaks at splicing sites for around 46.6% of the isoforms. What we cannot predict from our results, however, are the precise mechanism and the functional consequences of this change, that is, whether a local increase in chromatin accessibility at the splicing site would result in one or another alternatively spliced isoform due to the selective binding of splicing repressor or an activator. Indeed, according to a recent study, the type of event and the choice of a particular isoform would be multifactorial and depend on a trade-off between chromatin accessibility at regulatory sequences (such as splicing sites) and variable RNA pol II elongation rates (Pai and Luca, 2019).
In this sense, motif analyses can give some insights on the underlying mechanisms of AS regulation. Looking at the de novo motifs identified in the differentially expressed and used isoforms, we found overrepresented motifs that resemble several transcription and splicing factors of Drosophila, such as vismay (vis), zeste (z), chromatin-linked adaptor for MSL proteins (CLAMP), and dorsal (dl). In the case of CLAMP, this is a chromatin-remodeling protein with many different functions in regulating RNA Pol II dynamics, chromatin architecture, and alternative splicing (Schuettengruber et al., 2009; Urban et al., 2017). It has been described, for example, that the interaction of CLAMP with the proteins Squid (Sqd) and Syncrip (Syp), members of the heterogeneous nuclear ribonucleoproteins (hnRNPs), has a role in the regulation of the AS in Drosophila (Blanchette et al., 2009). Another aspect that we approached in this study was the relationship between the function of A. gambiae enhancers, defined in previous publications based on histone marks and chromatin accessibility patterns, and AS events. Previous evidence suggest that enhancers could mediate isoform-specific expression by regulating the production and binding of antisense transcripts as well as by regulating messenger RNA 3′ end processing (Onodera et al., 2012; Shiau et al., 2021; Kwon et al., 2022). In such cases, enhancers were expected to be intragenic, located near the exons or introns that undergo AS (Lam and Hertel, 2002; Matlin et al., 2005).
In this study, when we look at the presence of enhancers in isoforms with detected AS events (DEMG and DUI), we discovered that approximately 19% and 23% of differentially expressed and used isoforms, respectively, have an enhancer annotated, with ~20% of these being active in our study (accessible). This observation suggests a relationship between the enhancers and the expression and use of the isoforms. We also report that indeed 20% of the active enhancers in the DEMG group and 26% in the DUI group corresponded to differentially accessible enhancers (they have a differential activation status between tissues marked by a DiffBind region). Furthermore, most of our accessible enhancers (84.4% of the total of both groups) appeared to be intragenic, and 17.4% intersected with known splicing sites.
To validate this observation, we investigated whether such an association would also exist for non-multisoform genes. In this case, we reported that 707 accessible enhancers were annotated to 510 genes (only 4.61% of the total 11,060 non-multisoform genes). We also observed that the numbers of intragenic and intergenic enhancers were very similar (341 and 366, respectively) and that ~18% of the active enhancers (125 out of 707) displayed DiffBind peaks. Compared with our results in the much-restricted set of multisoform genes, clear differences can be observed—the not only the much higher percentage of annotated enhancers; but also preference of intragenic rather than intergenic enhancers in multisoform genes, which does not seem to be the case for the non-multisoform genes.
Another line of evidence supporting the role of enhancers in AS-mediated isoform usage and expression regulation in A. gambiae is the observation that their activation status changed according to the corresponding expression and use of the isoforms.
One example of an AS gene with an associated enhancer that is differentially accessible between conditions is the immune gene AGAP005234. In A. gambiae, the function of this gene is associated with the production of reactive oxygen species (ROS), which have been implicated in immune response to pathogens (Marikovsky et al., 2003), including Plasmodium (Molina-Cruz et al., 2008). Our data shows that the RB isoform is more used and expressed in salivary glands, whereas the RA isoform is only used in midguts. Accordingly, there is an upstream enhancer annotated to the AGAP005234 gene that is more accessible in salivary glands, suggesting a possible regulatory function of this enhancer in determining the choice of isoform that should be used (RA or RB) or differentially expressed (RB).
To conclude, this is, to our knowledge, the first study that comprehensively analyzes alternative splicing and its regulation in A. gambiae. We report genome-wide alterations in isoform expression and usage between different tissues and, to a lesser extent, linked to the Plasmodium infection status. The study of the relationship between AS and chromatin also points to the importance of chromatin structure dynamics at splicing sites in tissue-specific gene expression regulation. Finally, the identification of active enhancers associated to differentially expressed and used isoforms suggests a possible role of these regulatory elements on the splicing process. Our study lays the foundation for further research on the mechanisms of alternative splicing in A. gambiae, including the functional characterization of regulatory elements like enhancers and splicing factors as potential candidates for vector control.
RNA-seq data new to this study are deposited in the GEO database under accession number GSE243215. All code related to these analyses is available on GitHub with the name trn30/Agam_AlternativeSplicing project (https://github.com/trn30/Agam_AlternativeSplicing).
The studies involving humans were approved by Centre Muraz Institutional Ethics Committee (A003-2012/CE-CM). The studies were conducted in accordance with the local legislation and institutional requirements. Written informed consent for participation in this study was provided by the participants’ legal guardians/next of kin. The animal study was approved by Centre Muraz Institutional Ethics Committee (A003-2012/CE-CM). The study was conducted in accordance with the local legislation and institutional requirements.
BD-T: Data curation, Formal analysis, Investigation, Methodology, Software, Visualization, Writing – original draft. JLR: Writing – review & editing, Data curation, Methodology, Formal analysis, Investigation, Software. EG-D: Conceptualization, Funding acquisition, Investigation, Project administration, Resources, Supervision, Writing – review & editing, Data curation.
The author(s) declare financial support was received for the research, authorship, and/or publication of this article. This work was supported by the Spanish Ministry of Science and Innovation (grant no. PID2019-111109RB-I00). BD-T is funded by a doctoral training fellowship (grant no. PRE2020-095076). JR was the recipient of a technical support staff grant from the Ministry of Science and Innovation of the Spanish Government (grant no. PTA2021-019864-I).
We are grateful to Dr. Lisa Ranford-Cartwright. We thank the Bioinformatics Unit of the IPBLN-CSIC for computing facilities. This manuscript is available in preprint format at biorxiv. It is also part of the doctoral thesis of Bárbara Díaz Terenti in the Ph.D. program of molecular biology and biochemistry of the University of Granada.
The authors declare that the research was conducted in the absence of any commercial or financial relationships that could be construed as a potential conflict of interest.
The author(s) declared that they were an editorial board member of Frontiers, at the time of submission. This had no impact on the peer review process and the final decision.
All claims expressed in this article are solely those of the authors and do not necessarily represent those of their affiliated organizations, or those of the publisher, the editors and the reviewers. Any product that may be evaluated in this article, or claim that may be made by its manufacturer, is not guaranteed or endorsed by the publisher.
The Supplementary Material for this article can be found online at: https://www.frontiersin.org/articles/10.3389/fmala.2024.1347790/full#supplementary-material
DEMG, differential isoform expression of multisoform genes; DUI, differential isoform usage of multisoform genes; SS, splicing sites
Babraham Bioinformatics (n.d) FastQC A Quality Control tool for High Throughput Sequence Data. Available online at: https://www.bioinformatics.babraham.ac.uk/projects/fastqc/ (Accessed November 11, 2022).
Blanchette M., Green R. E., MacArthur S., Brooks A. N., Brenner S. E., Eisen M. B., et al. (2009). Genome-wide analysis of alternative pre-mRNA splicing and RNA binding specificities of the Drosophila hnRNP A/B family members. Mol. Cell 33, 438. doi: 10.1016/j.molcel.2009.01.022
Bush S. J., Chen L., Tovar-Corona J. M., Urrutia A. O. (2017). Alternative splicing and the evolution of phenotypic novelty. Philos. Trans. R. Soc. B: Biol. Sci. 372, 20150474. doi: 10.1098/rstb.2015.0474
Bushnell B. (2014). BBMap: a fast, accurate, splice-aware aligner. Lawrence Berkeley National Laboratory. LBNL Report #: LBNL-7065E. Retrieved from. https://escholarship.org/uc/item/1h3515gn.
Calkins T. L., Woods-Acevedo M. A., Hildebrandt O., Piermarini P. M. (2015). The molecular and immunochemical expression of innexins in the yellow fever mosquito, Aedes aEgypti: insights into putative life stage- and tissue-specific functions of gap junctions. Comp. Biochem. Physiol. B Biochem. Mol. Biol. 183, 11. doi: 10.1016/j.cbpb.2014.11.013
Chen L., Chen K., Hong Y., Xing L., Zhang J., Zhang K., et al. (2022). The landscape of isoform switches in sepsis: a multicenter cohort study. Sci. Rep. 12, 1–16. doi: 10.1038/s41598-022-14231-9
Chen Y., Ling E., Weng Z. (2009). Functional characterization of PGRP-LC1 of Anopheles Gambiae through deletion and RNA interference. Insect Sci. 16, 443–453. doi: 10.1111/j.1744-7917.2009.01296.x
Chodavarapu R. K., Feng S., Bernatavichute Y. V., Chen P. Y., Stroud H., Yu Y., et al. (2010). Relationship between nucleosome positioning and DNA methylation. Nature 466, 388–392. doi: 10.1038/nature09147
Davuluri R. V., Suzuki Y., Sugano S., Plass C., Huang T. H. M. (2008). The functional consequences of alternative promoter use in mammalian genomes. Trends Genet. 24, 167–177. doi: 10.1016/j.tig.2008.01.008
De La Fuente L., Arzalluz-Luque Á., Tardáguila M., Del Risco H., Martí C., Tarazona S., et al. (2020). TappAS: A comprehensive computational framework for the analysis of the functional impact of differential splicing. Genome Biol. 21, 1–32. doi: 10.1186/S13059-020-02028-W
Dixit R., Sharma A., Mourya D. T., Kamaraju R., Patole M. S., Shouche Y. S. (2009). Salivary gland transcriptome analysis during Plasmodium infection in malaria vector Anopheles stephensi. Int. J. Infect. Dis. 13, 636–646. doi: 10.1016/j.ijid.2008.07.027
Djihinto O., Saizonou H. D. M., Djogbenou L. S., Nolan T., Lee Y. (2022). Single nucleotide polymorphism (SNP) in the doublesex (dsx) gene splice sites and relevance for its alternative splicing in the malaria vector Anopheles Gambiae [version 1; peer review: 1 approved with reservations, 1 not approved]. Wellcome Open Res 7. doi: 10.12688/wellcomeopenres.17572.1
Dobin A., Davis C. A., Schlesinger F., Drenkow J., Zaleski C., Jha S., et al. (2013). STAR: ultrafast universal RNA-seq aligner. Bioinformatics 29, 15–21. doi: 10.1093/bioinformatics/bts635
Dwyer Z. W., Pleiss J. A. (2023). The problem of selection bias in studies of pre-mRNA splicing. Nat. Commun. 14, 1–5. doi: 10.1038/s41467-023-37650-2
EZlab | Computational Evolutionary Genomics group (n.d). Available online at: https://www.ezlab.org/ (Accessed November 14, 2022).
Gan Q., Chepelev I., Wei G., Tarayrah L., Cui K., Zhao K., et al. (2010). Dynamic regulation of alternative splicing and chromatin structure in Drosophila gonads revealed by RNA-seq. Cell Res. 20, 763. doi: 10.1038/cr.2010.64
Giraldo-Calderón G. I., Emrich S. J., MacCallum R. M., Maslen G., Emrich S., Collins F., et al. (2015). VectorBase: an updated bioinformatics resource for invertebrate vectors and other organisms related with human diseases. Nucleic Acids Res. 43, D707–D713. doi: 10.1093/nar/gku1117
Glaus P., Honkela A., Rattray M. (2012). Identifying differentially expressed transcripts from RNA-seq data with biological variation. Bioinformatics 28, 1721–1728. doi: 10.1093/bioinformatics/bts260
Heinz S., Benner C., Spann N., Bertolino E., Lin Y. C., Laslo P., et al. (2010). Simple combinations of lineage-determining transcription factors prime cis-regulatory elements required for macrophage and B cell identities. Mol. Cell 38, 576. doi: 10.1016/j.molcel.2010.05.004
Jimeno-González S., Payán-Bravo L., Muñoz-Cabello A. M., Guijo M., Gutierrez G., Prado F., et al. (2015). Defective histone supply causes changes in RNA polymerase II elongation rate and cotranscriptional pre-mRNA splicing. Proc. Natl. Acad. Sci. U.S.A. 112, 14840–14845. doi: 10.1073/pnas.1506760112
Katz Y., Wang E. T., Airoldi E. M., Burge C. B. (2010). Analysis and design of RNA sequencing experiments for identifying isoform regulation. Nat. Methods 7, 1009–1015. doi: 10.1038/nmeth.1528
Kelemen O., Convertini P., Zhang Z., Wen Y., Shen M., Falaleeva M., et al. (2013). Function of alternative splicing. Gene 514, 1. doi: 10.1016/j.gene.2012.07.083
Klemm S. L., Shipony Z., Greenleaf W. J. (2019). Chromatin accessibility and the regulatory epigenome. Nat. Rev. Genet. 20, 207–220. doi: 10.1038/s41576-018-0089-8
Kopylova E., Noé L., Touzet H. (2012). SortMeRNA: fast and accurate filtering of ribosomal RNAs in metatranscriptomic data. Bioinformatics 28, 3211–3217. doi: 10.1093/bioinformatics/bts611
Krzywinska E., Dennison N. J., Lycett G. J., Krzywinski J. (2016). A maleness gene in the malaria mosquito Anopheles Gambiae. Sci. (1979) 353, 67–69. doi: 10.1126/science.aaf5605
Kurtz J., Armitage S. A. O. (2006). Alternative adaptive immunity in invertebrates. Trends Immunol. 27, 493–496. doi: 10.1016/j.it.2006.09.001
Kwon B., Fansler M. M., Patel N. D., Lee J., Ma W., Mayr C. (2022). Enhancers regulate 3′ end processing activity to control expression of alternative 3′UTR isoforms. Nat. Commun. 13, 2709. doi: 10.1038/s41467-022-30525-y
Lam B. J., Hertel K. J. (2002). A general role for splicing enhancers in exon definition. RNA 8, 1233. doi: 10.1017/S1355838202028030
Li Y., Liu Y., Yang H., Zhang T., Naruse K., Tu Q. (2020). Dynamic transcriptional and chromatin accessibility landscape of medaka embryogenesis. Genome Res. 30, 924–937. doi: 10.1101/gr.258871.119
Lin H., Zhang L., Luna C., Hoa N. T., Zheng L. (2007). A splice variant of PGRP-LC required for expression of antimicrobial peptides in Anopheles Gambiae. Insect Sci. 14, 185–192. doi: 10.1111/j.1744-7917.2007.00142.x
Lin X., Liu F., Meng K., Liu H., Zhao Y., Chen Y., et al. (2022). Comprehensive transcriptome analysis reveals sex-specific alternative splicing events in zebrafish gonads. Life 12, 1441. doi: 10.3390/life12091441
Love M. I., Huber W., Anders S. (2014). Moderated estimation of fold change and dispersion for RNA-seq data with DESeq2. Genome Biol. 15, 1–21. doi: 10.1186/s13059-014-0550-8
Luco R. F., Allo M., Schor I. E., Kornblihtt A. R., Misteli T. (2011). Epigenetics in alternative pre-mRNA splicing. Cell 144, 16–26. doi: 10.1016/j.cell.2010.11.056
Malko D. B., Makeev V. J., Mironov A. A., Gelfand M. S. (2006). Evolution of exon–intron structure and alternative splicing in fruit flies and malarial mosquito genomes. Genome Res. 16, 505–509. doi: 10.1101/gr.4236606
Marikovsky M., Ziv V., Nevo N., Harris-Cerruti C., Mahler O. (2003). Cu/Zn superoxide dismutase plays important role in immune response. J. Immunol. 170, 2993–3001. doi: 10.4049/jimmunol.170.6.2993
Martinez N. M., Lynch K. W. (2013). Control of alternative splicing in immune responses: many regulators, many predictions, much still to learn. Immunol. Rev. 253, 216–236. doi: 10.1111/imr.12047
Matlin A. J., Clark F., Smith C. W. J. (2005). Understanding alternative splicing: towards a cellular code. Nat. Rev. Mol. Cell Biol. 6, 386–398. doi: 10.1038/nrm1645
Meister S., Agianian B., Turlure F., Relógio A., Morlais I., Kafatos F. C., et al. (2009). Anopheles Gambiae PGRPLC-Mediated Defense against Bacteria Modulates Infections with Malaria Parasites. PloS Pathog. 5, e1000542. doi: 10.1371/journal.ppat.1000542
Meister S., Kanzok S. M., Zheng X. L., Luna C., Li T. R., Hoa N. T., et al. (2005). Immune signaling pathways regulating bacterial and malaria parasite infection of the mosquito Anopheles Gambiae. Proc. Natl. Acad. Sci. U.S.A. 102, 11420–11425. doi: 10.1073/pnas.0504950102
Menéndez-Arias L., Sebastián-Martín A., Álvarez M. (2017). Viral reverse transcriptases. Virus Res. 234, 153–176. doi: 10.1016/j.virusres.2016.12.019
Merino G. A., Conesa A., Fernández E. A. (2019). A benchmarking of workflows for detecting differential splicing and differential expression at isoform level in human RNA-seq studies. Brief Bioinform. 20, 471–481. doi: 10.1093/bib/bbx122
Molina-Cruz A., DeJong R. J., Charles B., Gupta L., Kumar S., Jaramillo-Gutierrez G., et al. (2008). Reactive oxygen species modulate Anopheles Gambiae immunity against bacteria and Plasmodium. J. Biol. Chem. 283, 3217–3223. doi: 10.1074/jbc.M705873200
Montes M., Becerra S., Sánchez-Álvarez M., Suñé C. (2012). Functional coupling of transcription and splicing. Gene 501, 104–117. doi: 10.1016/j.gene.2012.04.006
Naftelberg S., Schor I. E., Ast G., Kornblihtt A. R. (n.d). Regulation of alternative splicing through coupling with transcription and chromatin structure. Annu Rev Biochem 84, 165–198 doi: 10.1146/annurev-biochem-060614-034242
Nilsen T. W., Graveley B. R. (2010). Expansion of the eukaryotic proteome by alternative splicing. Nature 463, 457–463. doi: 10.1038/nature08909
Nussinov R., Zhang M., Maloney R., Jang H. (2021). Ras isoform-specific expression, chromatin accessibility, and signaling. Biophys. Rev. 13, 489–505. doi: 10.1007/s12551-021-00817-6
Okonechnikov K., Conesa A., García-Alcalde F. (2016). Qualimap 2: advanced multi-sample quality control for high-throughput sequencing data. Bioinformatics 32, 292–294. doi: 10.1093/bioinformatics/btv566
Onodera C. S., Underwood J. G., Katzman S., Jacobs F., Greenberg D., Salama S. R., et al. (2012). Gene isoform specificity through enhancer-associated antisense transcription. PloS One 7, 43511. doi: 10.1371/journal.pone.0043511
Pai A. A., Luca F. (2019). Environmental influences on RNA processing: biochemical, molecular and genetic regulators of cellular response. Wiley Interdiscip Rev. RNA 10, e1503. doi: 10.1002/wrna.1503
Patro R., Duggal G., Love M. I., Irizarry R. A., Kingsford C. (2017). Salmon provides fast and bias-aware quantification of transcript expression. Nat. Methods 14, 417–419. doi: 10.1038/nmeth.4197
Petrova V., Song R., Consortium D., Nordström K. J. V., Walter J., Wong J. J. L., et al. (2022). Increased chromatin accessibility facilitates intron retention in specific cell differentiation states. Nucleic Acids Res. 50, 11563. doi: 10.1093/nar/gkac994
Quinlan A. R., Hall I. M. (2010). BEDTools: a flexible suite of utilities for comparing genomic features. Bioinformatics 26, 841–842. doi: 10.1093/bioinformatics/btq033
Ragle J. M., Katzman S., Akers T. F., Barberan-Soler S., Zahler A. M. (2015). Coordinated tissue-specific regulation of adjacent alternative 3′ splice sites in C. elegans. Genome Res. 25, 982. doi: 10.1101/gr.186783.114
Ruiz J. L., Ranford-Cartwright L. C., Gómez-Díaz E. (2021). The regulatory genome of the malaria vector Anopheles Gambiae: integrating chromatin accessibility and gene expression. NAR Genom Bioinform. 3, lqaa113. doi: 10.1093/nargab/lqaa113
Ruiz J. L., Terrón-Camero L. C., Castillo-González J., Fernández-Rengel I., Delgado M., Gonzalez-Rey E., et al. (2023). reanalyzerGSE: tackling the everlasting lack of reproducibility and reanalyses in transcriptomics. bioRxiv. doi: 10.1101/2023.07.12.548663
Ruiz J. L., Yerbanga R. S., Lefèvre T., Ouedraogo J. B., Corces V. G., Gómez-Díaz E. (2019). Chromatin changes in Anopheles Gambiae induced by Plasmodium falciparum infection 06 Biological Sciences 0604 Genetics 11 Medical and Health Sciences 1108 Medical Microbiology. Epigenet. Chromatin 12, 1–18. doi: 10.1186/S13072-018-0250-9
Saldi T., Cortazar M. A., Sheridan R. M., Bentley D. L. (2016). Coupling of RNA polymerase II transcription elongation with pre-mRNA splicing. J. Mol. Biol. 428, 2623. doi: 10.1016/j.jmb.2016.04.017
Salvemini M., D’Amato R., Petrella V., Aceto S., Nimmo D., Neira M., et al. (2013). The orthologue of the fruitfly sex behaviour gene fruitless in the mosquito aedes aEgypti: evolution of genomic organisation and alternative splicing. PloS One 8, 48554. doi: 10.1371/journal.pone.0048554
Salvemini M., Mauro U., Lombardo F., Milano A., Zazzaro V., Arcà B., et al. (2011). Genomic organization and splicing evolution of the doublesex gene, a Drosophila regulator of sexual differentiation, in the dengue and yellow fever mosquito Aedes aEgypti. BMC Evol. Biol. 11, 41. doi: 10.1186/1471-2148-11-41
Schor I. E., Gómez Acuña L. I., Kornblihtt A. R. (2013). Coupling between transcription and alternative splicing. Cancer Treat Res. 158, , 1–24. doi: 10.1007/978-3-642-31659-3_1
Schuettengruber B., Ganapathi M., Leblanc B., Portoso M., Jaschek R., Tolhuis B., et al. (2009). Functional anatomy of polycomb and trithorax chromatin landscapes in drosophila embryos. PloS Biol. 7, 1000013. doi: 10.1371/journal.pbio.1000013
Shen L., Shao N., Liu X., Nestler E. (2014). Ngs.plot: Quick mining and visualization of next-generation sequencing data by integrating genomic databases. BMC Genomics 15, 1–14. doi: 10.1186/1471-2164-15-284
Shenasa H., Hertel K. J. (2019). Combinatorial regulation of alternative splicing. Biochim. Biophys. Acta (BBA) - Gene Regul. Mech. 1862, 194392. doi: 10.1016/j.bbagrm.2019.06.003
Shiau C. K., Huang J. H., Liu Y. T., Tsai H. K. (2021). Genome-wide identification of associations between enhancer and alternative splicing in human and mouse. BMC Genomics 22, 919. doi: 10.1186/s12864-022-08537-1
Simon J. M., Hacker K. E., Singh D., Brannon A. R., Parker J. S., Weiser M., et al. (2014). Variation in chromatin accessibility in human kidney cancer links H3K36 methyltransferase loss with widespread RNA processing defects. Genome Res. 24, 241–250. doi: 10.1101/gr.158253.113
Song L., Pan Z., Chen L., Dai Y., Wan J., Ye H., et al. (2020). Analysis of whole transcriptome RNA-seq data reveals many alternative splicing events in soybean roots under drought stress conditions. Genes 11, 1520. doi: 10.3390/genes11121520
Sreenivasamurthy S. K., Dey G., Ramu M., Kumar M., Gupta M. K., Mohanty A. K., et al. (2013). A compendium of molecules involved in vector-pathogen interactions pertaining to malaria. Malar J. 12, 216. doi: 10.1186/1475-2875-12-216
Sreenivasamurthy S. K., Madugundu A. K., Patil A. H., Dey G., Mohanty A. K., Kumar M., et al. (2017). Mosquito-borne diseases and omics: tissue-restricted expression and alternative splicing revealed by transcriptome profiling of anopheles stephensi. OMICS 21, 488–497. doi: 10.1089/omi.2017.0073
Stark R., Brown G. (2011). “DiffBind: differential binding analysis of ChIP-Seq peak data,” in R Package Version, vol. 100. , 4–3.
Telonis-Scott M., Kopp A., Wayne M. L., Nuzhdin S. V., McIntyre L. M. (2009). Sex-specific splicing in drosophila: widespread occurrence, tissue specificity and evolutionary conservation. Genetics 181, 421. doi: 10.1534/genetics.108.096743
Thorvaldsdóttir H., Robinson J. T., Mesirov J. P. (2013). Integrative Genomics Viewer (IGV): high-performance genomics data visualization and exploration. Brief Bioinform. 14, 178–192. doi: 10.1093/bib/bbs017
Tsujimoto H., Liu K., Linser P. J., Agre P., Rasgon J. L. (2013). Organ-specific splice variants of aquaporin water channel AgAQP1 in the malaria vector anopheles Gambiae. PloS One 8, e75888. doi: 10.1371/journal.pone.0075888
Ule J., Blencowe B. J. (2019). Alternative splicing regulatory networks: functions, mechanisms, and evolution. Mol. Cell 76, 329–345. doi: 10.1016/j.molcel.2019.09.017
Ullah F., Hamilton M., Reddy A. S. N., Ben-Hur A. (2018). Exploring the relationship between intron retention and chromatin accessibility in plants. BMC Genomics 19, 21. doi: 10.1186/s12864-017-4393-z
Urban J. A., Urban J. M., Kuzu G., Larschan E. N. (2017). The Drosophila CLAMP protein associates with diverse proteins on chromatin. PloS One 12, e0189772. doi: 10.1371/journal.pone.0189772
Varabyou A., Salzberg S. L., Pertea M. (2021). Effects of transcriptional noise on estimates of gene and transcript expression in RNA sequencing experiments. Genome Res. 31, 301–308. doi: 10.1101/gr.266213.120
Venables J. P., Tazi J., Juge F. (2012). Regulated functional alternative splicing in Drosophila. Nucleic Acids Res. 40, 1–10. doi: 10.1093/nar/gkr648
Verta J. P., Jacobs A. (2022). The role of alternative splicing in adaptation and evolution. Trends Ecol. Evol. 37, 299–308. doi: 10.1016/j.tree.2021.11.010
Vitting-Seerup K., Sandelin A. (2017). The landscape of isoform switches in human cancers. Mol. Cancer Res. 15, 1206–1220. doi: 10.1158/1541-7786.MCR-16-0459/14585/AM/THE-LANDSCAPE-OF-ISOFORM-SWITCHES-IN-HUMAN
Vitting-Seerup K., Sandelin A., Berger B. (2019). IsoformSwitchAnalyzeR: analysis of changes in genome-wide patterns of alternative splicing and its functional consequences. Bioinformatics 35, 4469–4471. doi: 10.1093/bioinformatics/btz247
Wang G. S., Cooper T. A. (2007). Splicing in disease: disruption of the splicing code and the decoding machinery. Nat. Rev. Genet. 10, 749–761. doi: 10.1038/nrg2164
Wang E. T., Sandberg R., Luo S., Khrebtukova I., Zhang L., Mayr C., et al. (2008). Alternative isoform regulation in human tissue transcriptomes. Nature 456, 470–476. doi: 10.1038/nature07509
Wickham H. (2016). ggplot2: Elegant Graphics for Data Analysis (New York: Springer-Verlag), ISBN: ISBN 978-3-319-24277-4.
Wright C. J., Smith C. W. J., Jiggins C. D. (2022). Alternative splicing as a source of phenotypic diversity. Nat. Rev. Genet. 23, 697–710, 1–14. doi: 10.1038/s41576-022-00514-4
Zahler A. M. (2005). Alternative splicing in C. elegans. WormBook, 1–13. doi: 10.1895/wormbook.1.31.1
Zhang Y., Liu T., Meyer C. A., Eeckhoute J., Johnson D. S., Bernstein B. E., et al. (2008). Model-based analysis of ChIP-seq (MACS). Genome Biol. 9, R137. doi: 10.1186/gb-2008-9-9-r137
Zhang C., Zhang B., Vincent M. S., Zhao S. (2016). Bioinformatics tools for RNA-seq gene and isoform quantification. Journal of Next Generation Sequencing & Applications 03. doi: 10.4172/2469-9853.1000140
Keywords: mosquitoes, plasmodium, alternative splicing, isoform expression, isoform usage, chromatin accessibility
Citation: Díaz-Terenti B, Ruiz JL and Gómez-Díaz E (2024) Alternative splicing and its regulation in the malaria vector Anopheles gambiae. Front. Malar. 2:1347790. doi: 10.3389/fmala.2024.1347790
Received: 01 December 2023; Accepted: 05 April 2024;
Published: 26 April 2024.
Edited by:
Louisa Alexandra Messenger, University of Nevada, Las Vegas, United StatesReviewed by:
Geoffrey H. Siwo, University of Michigan, United StatesCopyright © 2024 Díaz-Terenti, Ruiz and Gómez-Díaz. This is an open-access article distributed under the terms of the Creative Commons Attribution License (CC BY). The use, distribution or reproduction in other forums is permitted, provided the original author(s) and the copyright owner(s) are credited and that the original publication in this journal is cited, in accordance with accepted academic practice. No use, distribution or reproduction is permitted which does not comply with these terms.
*Correspondence: Elena Gómez-Díaz, ZWxlbmEuZ29tZXpAY3NpYy5lcw==
Disclaimer: All claims expressed in this article are solely those of the authors and do not necessarily represent those of their affiliated organizations, or those of the publisher, the editors and the reviewers. Any product that may be evaluated in this article or claim that may be made by its manufacturer is not guaranteed or endorsed by the publisher.
Research integrity at Frontiers
Learn more about the work of our research integrity team to safeguard the quality of each article we publish.