- 1Department of Psychology, Aichi Shukutoku University, Nagakute, Japan
- 2College of Humanities and Sciences, Nihon University, Setagaya, Tokyo, Japan
1 Introduction
Several lexical databases have been developed in both English-speaking countries and other countries, leading to numerous studies using these resources. A prominent example is the English Lexicon Project (ELP; Balota et al., 2007), a large-scale database containing behavioral data on English word processing. The ELP provides data for two main tasks: the lexical decision task (LDT) and the speeded naming task. Among these, the LDT is the most commonly utilized in word-processing research, largely because (1) it is easy to implement, and (2) it can be conducted online with relative ease (Lieber et al., 2014).
In the LDT, participants are asked to decide as quickly and accurately as possible whether a visually presented string of letters forms a real word or a non-word. By analyzing the response time from when the string is presented until the participant makes a decision, researchers can evaluate the speed of word access and semantic processing. The LDT has been employed not only to assess word processing efficiency and cognitive load but also to investigate the structure of the mental lexicon and concept representation. For example, researchers have examined the relationship between LDT response times and various word properties, including the frequency effect, where more frequent words are processed faster and reexamined using the LDT data (Brysbaert et al., 2011).
Numerous psycholinguistic studies have explored the semantic properties of word recognition using LDT data. Recently, LDT databases have expanded beyond English, with resources available in languages such as Chinese (Tse et al., 2017), French (Ferrand et al., 2018, 2010), and Spanish (Aguasvivas et al., 2018), allowing for more efficient research across languages. For example, researchers have tested hypotheses involving grounded cognition and embodied cognition (Barsalou, 2008) in word recognition and explored the relationship between word recognition and sensorimotor information across various languages, e.g., English (Pexman et al., 2019; Sidhu et al., 2014), French (Lalancette et al., 2024), and Spanish (Alonso et al., 2018). They further examined theoretical predictions with large-scale survey data, often using lexical decision task (LDT) reaction times as the dependent variable in regression analyses. While earlier findings have supported these theories by showing consistent trends across languages, recent discussions have highlighted cross-linguistic variability in these effects (Alonso et al., 2018; Lalancette et al., 2024). Such hypothesis testing using a database reduces stimulus bias by incorporating many words (see Dymarska et al., 2023) and enables new discoveries through cross-linguistic comparisons.
In Japanese, several databases are available, as will be discussed later. For example, databases exist for attributes such as word imageability (Sakuma et al., 2005) and familiarity (Asahara, 2020), each containing evaluative data for tens of thousands of words. These databases have long been used in various ways, such as serving as control variables in numerous Japanese word recognition studies (e.g., Mizuno and Matsui, 2018; Mochizuki and Ota, 2020, 2024). However, no LDT database currently exists for Japanese, posing a challenge to psycholinguistic research on the Japanese language as a result of limited resources. Of course, lexical decision tasks have been widely used in Japanese word recognition studies (e.g., Kawakami, 2002; Kusunose et al., 2013). However, the number of stimulus words used in these studies is significantly smaller compared to databases such as the ELP (Balota et al., 2007). Furthermore, the data are not always publicly available, which limits their utility as resources. Given the increasing emphasis on cross-linguistic validation—particularly in studies of abstract concepts shaped by language and culture (Dove, 2018)—developing a large-scale Japanese LDT database would not only aid Japanese researchers but also contribute to the broader field. Therefore, this study aimed to construct a Japanese version of LDT database.
It is important to note that individual differences in LDT response times exist (e.g., Hawker and Ferraro, 2007; Yates and Slattery, 2019; Lim et al., 2020). To enhance the database, we collected data on participants' individual characteristics following the LDT. Specifically, participants completed the ENDCOREs, which measures interpersonal communication skills (Fujimoto and Daibo, 2007), and the Japanese version of the Plymouth Sensory Imagery Questionnaire (Psi-Q; Fukui and Aoki, 2022). The ENDCOREs assesses six dimensions of communication: self-control, expressiveness, comprehension, assertiveness, acceptance of others, and relational adjustment. The Psi-Q evaluates the vividness of mental imagery across sensory modalities (i.e., vision, sound, smell, taste, touch, body, and emotion), capturing individual differences in multisensory imagery.
Although we do not hypothesize a direct relationship between these individual difference variables and simple LDT response times (e.g., the higher/lower a score, the slower/faster the response time), they may serve as possible predictors for validating certain content. For instance, the grounded or embodied cognition framework (Barsalou, 2020, 2008) posits that processing words or concepts involves simulating the sensory modalities through which they are acquired. Consistent with this, processing words rich in sensorimotor information tends to be more efficient (Lynott et al., 2020; Siakaluk et al., 2008; Sidhu et al., 2014; Sidhu and Pexman, 2016; Tillotson et al., 2008). Individual differences in sensitivity to sensory and motor modalities may interact with word characteristics and influence LDT performance. Furthermore, the “Words as Social Tools” (WAT) perspective (Borghi and Binkofski, 2014) posits that simulating social and linguistic information is crucial for understanding abstract concepts (Borghi et al., 2019). Therefore, words with a stronger social nature may be processed more efficiently (Diveica et al., 2023), and the interaction between verbal sociality and individual sociality may affect LDT response times. Since ENDCOREs reflect an individual's communication skills, individuals with high social interaction skills may find it easier to simulate socially relevant words. Consequently, they might be more efficient in processing abstract words with strong social characteristics. While the present study did not specifically examine the relationship between individual differences and LDT response times, future research could benefit from incorporating these variables into the database.
This report introduces the Japanese LDT database (JALEX), which incorporates individual differences among participants. The response time and accuracy data can be used for future psycholinguistic studies involving Japanese participants. Additionally, while no hypotheses were tested, future research may explore the role of individual differences as needed.
2 Method
2.1 Participants
In the development of psycholinguistic norms, ~30–40 observations per word are typically required (Balota et al., 2007; Ferrand et al., 2018). However, we recruited a relatively large number of participants to account for potential dropouts, as this was an online study, and to develop more reliable norms.
Participants were recruited through a crowdsourcing service Yahoo! Crowdsourcing (https://crowdsourcing.yahoo.co.jp/). A total of 2,689 individuals accessed the task. However, 1,037 either did not start, failed to complete the task, or provided no responses. Ultimately, 1,652 participants completed the task. All participants self-reported as native Japanese speakers. Among them, 1,226 were men, 407 were women, two identified as other genders, and 17 chose not to respond. The mean age was 51.07 years (SD = 11.91), with a range from 18 to 85 years. The participants' highest levels of education were as follows: 26 had completed doctoral programs, 119 had master's degrees, 1,069 were college graduates, 21 had finished high school, 28 had completed junior high school, and 26 chose not to respond. As detailed below, the words were divided into 38 lists. With 1,652 participants, this resulted in ~43 participants per list.1
2.2 Stimuli
To develop JALEX databases, we selected words with semantic properties listed in multiple extant databases (DBs). This approach ensured consistency with previous word recognition studies and supported continuity in future research. We followed a specific selection procedure. First, we used the Word List by Semantic Principles, revised and enlarged edition (WLSP, National Institute for Japanese Language Linguistics, 2004) as the master list. From this, we selected words that appeared in all eight of the following DBs: the word familiarity DB (Asahara, 2020), an alternate word familiarity DB (Fujita and Kobayashi, 2020), the word frequency DB (Amano and Kondo, 2000), the NINJAL-LWP for TWC word frequency DB (University of Tsukuba, 2013), the word difficulty DB (Kajiwara et al., 2020), the imageability DB for visual words (Sakuma et al., 2005), the semantic orientations DB (Takamura et al., 2005), and the abstractness DB for Japanese words (The Social Computing Laboratory, 2021). Following this procedure, we selected 5,736 Japanese words as stimuli. These included 4,977 nouns, 648 verbs, and 111 adjectives.
For each word, linguistic characteristics such as orthographic neighborhood size (ONS), phonological neighborhood size (PNS), orthographic Levenshtein distance 20 (OLD20, Yarkoni et al., 2008), the number of letters, and the number of morae (a rhythmic unit of sound) were calculated. The PNS was computed by decomposing the “phonetic” (読み) variable in the WLSP (National Institute for Japanese Language Linguistics, 2004) by mora and calculating how many words in the WLSP had one mora replaced. Similarly, the ONS was calculated by decomposing the “letter (見出し本体)” variable in the WLSP into individual characters and determining how many words had one letter replaced. OLD20 was calculated using the old20 function in the vwr package (Keuleers, 2013) in R (R Core Team, 2022), based on the “letter (見出し本体)” variable in the WLSP.
In addition, non-words were constructed as filler items for the LDT. First, from the WLSP, we excluded words with one mora, words containing spaces, symbols, or particles, homophones, and items with repetitive morae (e.g., ha-ha-ha [ha/ha/ha]), as these could not be transformed into non-words using the procedure described below. The remaining items were then decomposed into morae, and each mora was randomly shuffled. If the resulting item was not found in the WLSP, it was considered a non-word candidate. This process yielded 63,305 non-word candidates, from which we randomly selected 5,736 to serve as fillers for the LDT. The authors reviewed these candidates, and those deemed too similar to real words were replaced with different non-word candidates. All non-word stimuli are available for reference on Open Science Framework (OSF).
The words and non-words were randomly divided into 38 lists, each containing 150 or 151 words (150 × 2 + 151 × 36 = 5,736) with an equal number of non-words.
2.3 Procedure
The LDT task was conducted online, and participants accessed the LDT program via their own PCs. The program was created using PsychoPy (Peirce et al., 2019) and hosted on Pavlovia (https://pavlovia.org/). After obtaining informed consent from the participants, they were instructed to begin the task. In the LDT, a blank screen appeared for 200 ms, followed by a fixation point in the center of the screen for 300 ms. A string of characters was then presented, and participants had to decide as quickly and accurately as possible whether the string represented a real Japanese word. The string remained on the screen until a response was made or for up to 2,000 ms. Participants pressed the “L” key for words and the “S” key for non-words. If the response was correct, the task proceeded to the next trial; if incorrect, a feedback message (“Wrong”) appeared in red. If no response was given within 2,000 ms, the feedback message (“Too late”) was displayed in red for 300 ms. Words and non-words were presented in random order. Participants completed 20 practice trials before starting the actual task. The practice trials used different stimuli from those in the actual task.
During the task, participants were allowed to take a break for a maximum of 60 s between the 100 and 200th trials. During the break, their percentage of correct answers was displayed to encourage them to continue. Upon completing the LDT, participants answered the ENDCOREs (Fujimoto and Daibo, 2007) and Psi-Q (Fukui and Aoki, 2022) questionnaires.2 Additionally, they provided demographic information, including gender, age, dominant hand, highest level of education, and native language. Data were collected on May 20 and May 21, 2024.
3 Data processing
We calculated the accuracy rate for each participant, and the lowest percentage of correct responses exceeded 75%. Since no participants demonstrated an exceptionally low accuracy rate, data from all participants were retained for analysis.
The procedure for processing the response time data followed that employed in ELP (Balota et al., 2007). First, we extracted only correct trials, where the “L” key was pressed for word stimuli, and excluded any trials with response times below 200 ms. Second, we removed trials that deviated by ±3 SD from the participant's mean response time. This resulted in the exclusion of 1.96% of trials as outliers.
4 Dataset overview
The distribution of response times averaged by item is showed in Figure 1. We presented the partial correlations with existing DB variables referenced in stimulus selection to examine the convergent validity of the response time data (Figure 2). These findings confirmed the phenomena predicted in prior studies. Specifically, we confirmed the frequency effect (Rubenstein et al., 1971) and familiarity effect (Connine et al., 1990), where lexical decision times decrease as word frequency and familiarity increase. We also observed the imageability effect (Balota et al., 2004), where higher imageability leads to faster lexical decisions, and the orthographic similarity effect (Yarkoni et al., 2008), where greater Levenshtein distance results in longer response times. While few studies have reported simple or partial correlations with these variables in Japanese, several experimental studies using Japanese words as stimuli have observed effects similar to those identified in the present study. For instance, Japanese word recognition research has reported faster word processing for words with higher imageability (Ogawa and Nittono, 2018) and higher frequency (Mizuno and Matsui, 2015). The relationship between response time and difficulty rating (Kajiwara et al., 2020) has yet to be investigated. However, it is reasonable to predict that more difficult words would require longer processing times for comprehension. The current analysis identified a slight positive correlation between word difficulty and response time. These findings suggest that JALEX is valid to a considerable extent.
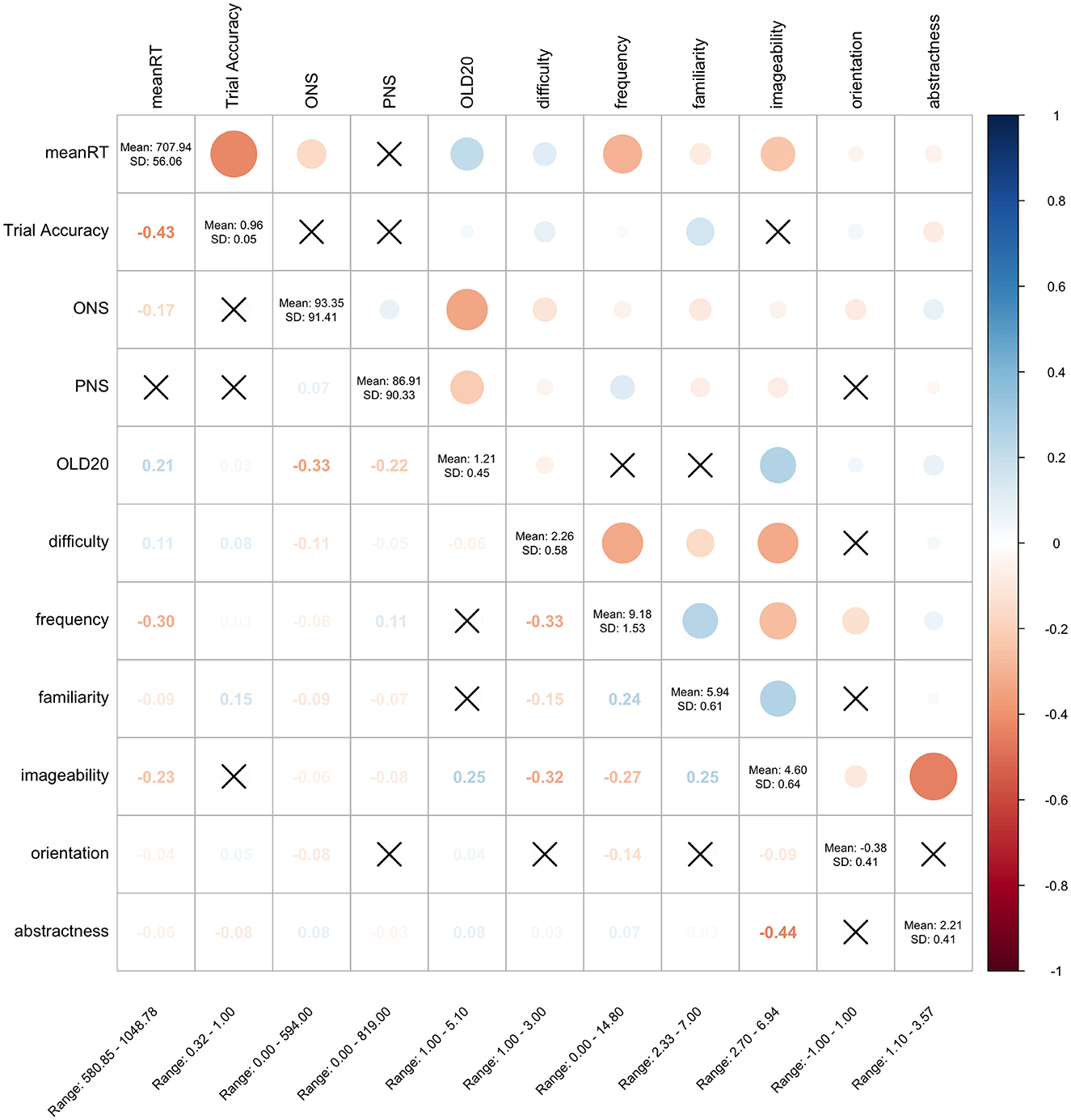
Figure 2. Partial correlation between measures in LDT and each variable. Crosses in the figure indicate non-significant correlations. LDT, Lexical Decision Task; meanRT, Mean response time in the LDT; ACC, Mean accuracy in the LDT; ONS, Orthographic Neighborhood Size; PNS, Phonological Neighborhood Size; OLD20. Orthographic Levenshtein Distance 20; were calculated by the authors. The following variables are derived from previous studies: difficulty (Kajiwara et al., 2020), frequency (log-transformed, University of Tsukuba, 2013), familiarity (Fujita and Kobayashi, 2020), imageability (Sakuma et al., 2005), orientation (semantic orientations, Takamura et al., 2005), and abstractness (The Social Computing Laboratory, 2021). The diagonal elements in the matrix shown in the figure represent the mean and standard deviation for each variable, while the values at the bottom indicate the range of each variable.
The partial correlations between familiarity, semantic orientation, abstractness, and response time were significant, but the effects were small. Of these, the zero-order correlation for familiarity was r = −0.42, suggesting that higher familiarity facilitates responses when not adjusted for covariates. Zero-order correlations for abstractness revealed a small effect (r = 0.11), indicating that processing was slightly suppressed for more abstract words, consistent with the representativeness effect (Cortese and Balota, 2012). This study also found that words with high ONS had shorter lexical decision times. The results showed that high ONS words took less time to judge than low ONS words when using Kanji words (Mizuno and Matsui, 2014), which is consistent with the current results. However, when using Katakana words, the inhibitory effect was observed, indicating that low ONS words took less time to judge than high ONS words (Kawakami, 2002). Furthermore, an interaction between ONS and PNS has also been observed in lexical decision performance for katakana words (Hino et al., 2011). The difference in these results may be caused by the limited number of words used in the experiment and the factors of the orthographic form. In studies using word norms, it is particularly important to consider the extent of word coverage and the absence of bias (Dymarska et al., 2023). The failure to replicate the effects observed in previous studies in the present analysis of a relatively large database may be attributed to biases in the stimulus sets used in those studies, which could have significantly influenced their results. Future research should assess the reproducibility of findings from previous studies by leveraging large databases, such as JALEX, and conducting comprehensive analyses.
5 Theoretical implications and future studies
In the present study, the imageability effect (Balota et al., 2004) was replicated even after controlling for linguistic statistical variables, such as the frequency of neighboring words. The effects of psycholinguistic variables, such as the imageability effect, are often discussed in relation to semantic richness (Pexman et al., 2013). Semantic richness refers to the idea that words associated with more semantic information have richer semantic representations, enabling them to be processed more quickly and accurately. In other words, our study replicates in Japanese the finding that the ease of forming a mental image is an important semantic variable in word representations. As discussed in the introduction, the relationship between sensorimotor information and word recognition is explained by the concept of semantic richness—specifically, the richness of the semantic dimension of sensorimotor information facilitates word recognition. In future studies, it will be important to investigate the nature of concept representations by examining psycholinguistic variables influencing word recognition beyond imageability.
Furthermore, this study is the first DB of LDT to include individual difference variables for respondents, paving the way for future research on individual differences using JALEX. In word recognition research, it has been observed that certain words exhibit significant individual differences and high variability in ratings of psychological variables (Paisios et al., 2023). A key limitation of the previous DB of LDT is that they did not provide individual difference data for participants, making them unsuitable for studying individual differences in words with high variability in ratings among individuals. Future research using JALEX is expected to refine further grounded cognition theory (Barsalou, 2020, 2008) and advance WAT theory (Borghi and Binkofski, 2014), particularly by promoting individual difference studies on the simulation of sensorimotor information and those related to social communication.
6 Strengths and limitations
This database represents the most comprehensive dataset on the efficiency of Japanese visual word processing and stands as a powerful resource for future research in psychology and linguistics. A unique feature of this database is its inclusion of individual difference variables for participants, allowing researchers to analyze these differences in future studies.
However, it is important to note that some words in the dataset had lower accuracy rates. For example, at least 15 items had a correct response rate below 70%, with fewer than 20 observations. Items with fewer observations may exhibit lower reliability and reproducibility compared to others. While we did not exclude these items in the current analysis, researchers should be mindful of their presence when using the database.
Data availability statement
The datasets presented in this study can be found in online repositories. The names of the repository/repositories and accession number(s) can be found at: https://osf.io/qr2sg, Open Science Framework.
Ethics statement
The studies involving humans were approved by Ethics Committee of College of Humanities and Sciences, Nihon University. The studies were conducted in accordance with the local legislation and institutional requirements. The participants provided their written informed consent to participate in this study.
Author contributions
NO: Conceptualization, Formal analysis, Validation, Visualization, Writing – original draft. MM: Conceptualization, Data curation, Funding acquisition, Investigation, Methodology, Project administration, Resources, Software, Writing – review & editing.
Funding
The author(s) declare financial support was received for the research, authorship, and/or publication of this article. This work was supported by JSPS KAKENHI Grant Number: JP24K15684.
Acknowledgments
The authors would like to thank all the participants of the study.
Conflict of interest
The authors declare that the research was conducted in the absence of any commercial or financial relationships that could be construed as a potential conflict of interest.
Generative AI statement
The author(s) declare that no Gen AI was used in the creation of this manuscript.
Publisher's note
All claims expressed in this article are solely those of the authors and do not necessarily represent those of their affiliated organizations, or those of the publisher, the editors and the reviewers. Any product that may be evaluated in this article, or claim that may be made by its manufacturer, is not guaranteed or endorsed by the publisher.
Footnotes
1. ^Note that, due to program constraints, not exactly the same number of participants per list was allocated.
2. ^As previously mentioned, we do not assume a direct relationship between individual difference variables and reaction time. Consequently, we did not investigate the association between these variables in this study.
References
Aguasvivas, J. A., Carreiras, M., Brysbaert, M., Mandera, P., Keuleers, E., and Duñabeitia, J. A. (2018). SPALEX: a Spanish lexical decision database from a massive online data collection. Front. Psychol. 9:2156. doi: 10.3389/fpsyg.2018.02156
Alonso, M. Á., Díez, E., Díez-Álamo, A. M., and Fernandez, A. (2018). Body-object interaction ratings for 750 Spanish words. Appl. Psycholingust. 39, 1239–1252. doi: 10.1017/S0142716418000309
Amano, S., and Kondo, K. (2000). NTT Database Series. Lexical Properties of Japanese, No. 7. Frequency (Tokyo: Sanseido).
Asahara, M. (2020). Word familiarity rate and register type estimation using a Bayesian linear mixed model. J. Nat. Lang. Process 27, 133–150. doi: 10.5715/jnlp.27.133
Balota, D. A., Cortese, M. J., Sergent-Marshall, S. D., Spieler, D. H., and Yap, M. J. (2004). Visual word recognition of single-syllable words. J. Exp. Psychol. Gen. 133, 283–316. doi: 10.1037/0096-3445.133.2.283
Balota, D. A., Yap, M. J., Cortese, M. J., Hutchison, K. A., Kessler, B., Loftis, B., et al. (2007). The English lexicon project. Behav. Res. Methods 39, 445–459. doi: 10.3758/BF03193014
Barsalou, L. W. (2008). Grounded cognition. Annu. Rev. Psychol. 59, 617–645. doi: 10.1146/annurev.psych.59.103006.093639
Barsalou, L. W. (2020). Challenges and opportunities for grounding cognition. J. Cogn. 3:31. doi: 10.5334/joc.116
Borghi, A. M., Barca, L., Binkofski, F., Castelfranchi, C., Pezzulo, G., and Tummolini, L. (2019). Words as social tools: language, sociality and inner grounding in abstract concepts. Phys. Life Rev. 29, 120–153. doi: 10.1016/j.plrev.2018.12.001
Borghi, A. M., and Binkofski, F. (2014). Words as Social Tools: an Embodied View on Abstract Concepts. New York, NY: Springer.
Brysbaert, M., Buchmeier, M., Conrad, M., Jacobs, A. M., Bölte, J., and Böhl, A. (2011). The word frequency effect: a review of recent developments and implications for the choice of frequency estimates in German. Exp. Psychol. 58, 412–424. doi: 10.1027/1618-3169/a000123
Connine, C. M., Mullennix, J. W., Shernoff, E. H., and Yelen, J. (1990). Word familiarity and frequency in visual and auditory word recognition. J. Exp. Psychol. Learn. Mem. Cogn. 16, 1084–1096. doi: 10.1037/0278-7393.16.6.1084
Cortese, M. J., and Balota, D. A. (2012). “Visual word recognition in skilled adult readers,” in The Cambridge Handbook of Psycholinguistics, eds. M. J. Spivey, K. McRae, and M. F. Joanisse (Cambridge: Cambridge University Press), 159–185.
Diveica, V., Pexman, P. M., and Binney, R. J. (2023). Quantifying social semantics: an inclusive definition of socialness and ratings for 8388 English words. Behav. Res. Methods 55, 461–473. doi: 10.3758/s13428-022-01810-x
Dove, G. (2018). Language as a disruptive technology: abstract concepts, embodiment and the flexible mind. Philos. Trans. R. Soc. Lond. B Biol. Sci. 373:20170135. doi: 10.1098/rstb.2017.0135
Dymarska, A., Connell, L., and Banks, B. (2023). Weaker than you might imagine: determining imageability effects on word recognition. J. Mem. Lang. 129, 1–25. doi: 10.1016/j.jml.2022.104398
Ferrand, L., Méot, A., Spinelli, E., New, B., Pallier, C., Bonin, P., et al. (2018). MEGALEX: a megastudy of visual and auditory word recognition. Behav. Res. 50, 1285–1307. doi: 10.3758/s13428-017-0943-1
Ferrand, L., New, B., Brysbaert, M., Keuleers, E., Bonin, P., Méot, A., et al. (2010). The French lexicon project: lexical decision data for 38,840 French words and 38,840 pseudowords. Behav. Res. Methods 42, 488–496. doi: 10.3758/BRM.42.2.488
Fujimoto, M., and Daibo, I. (2007). ENDCORE: a hierarchical structure theory of communication skills. Jpn. J. Pers. 15, 347–361. doi: 10.2132/personality.15.347
Fujita, S., and Kobayashi, T. (2020). “Resurvey of word familiarity and comparison with past data,” in Proceedings of the Twenty-Sixth Annual Meeting of the Association for Natural Language Processing (Tokyo: The Japanese Society for Artificial Intelligence), 1037–1040.
Fukui, H., and Aoki, S. (2022). The development of the Japanese version of the Plymouth sensory imagery questionnaire (Psi-Q). Jpn. J. Pers. 31, 87–99. doi: 10.2132/personality.31.2.2
Hawker, R., and Ferraro, R. F. (2007). Individual differences in lexical decision: the effect of gender and type of instruction on the number-of-meanings effects. Psychol. Educ. Interdiscip. J. 44, 13–28.
Hino, Y., Nakayama, M., Miyamura, S., and Kusunose, Y. (2011). Orthographic and phonological neighborhood size effects for Japanese Katakana words in a lexical decision task. Jpn. J. Psychol. 81, 569–576. doi: 10.4992/jjpsy.81.569
Kajiwara, T., Nishihara, D., Kodaira, T., and Komachi, M. (2020). Language resources for Japanese lexical simplification. J. Nat. Lang. Process 27, 801–824. doi: 10.5715/jnlp.27.801
Kawakami, M. (2002). Effects of neighborhood size and kanji character frequency on lexical decision of Japanese kanji compound words. Jpn. J. Psychol. 73, 346–351. doi: 10.4992/jjpsy.73.346
Keuleers, E. (2013). VWR: Useful Functions for Visual Word Recognition Research. Available at: http://cran.nexr.com/web/packages/vwr/index.html (accessed January 10, 2024).
Kusunose, Y., Yoshihara, M., Ida, K., Xue, J., Ijuin, M., and Hino, Y. (2013). Word length effects for kana and kanji words in lexical decision tasks. Jpn. J. Cog. Psychol. 11, 105–115. doi: 10.5265/jcogpsy.11.105
Lalancette, A., Garneau, É., Cochrane, A., and Wilson, M. A. (2024). Body-object interaction ratings for 3600 French nouns. Behav. Res. Method 56, 8009–8021. doi: 10.3758/s13428-024-02466-5
Lieber, R., Štekauer, P., and Baayen, H. (2014). “Experimental and psycholinguistic approaches,” in The Oxford Handbook of Derivational Morphology (Oxford: Oxford University Press), 7.
Lim, R. Y., Yap, M. J., and Tse, C.-S. (2020). Individual differences in Cantonese Chinese word recognition: insights from the Chinese lexicon project. Q. J. Exp. Psychol. 73, 504–518. doi: 10.1177/1747021820906566
Lynott, D., Connell, L., Brysbaert, M., Brand, J., and Carney, J. (2020). The Lancaster sensorimotor norms: multidimensional measures of perceptual and action strength for 40,000 English words. Behav. Res. Methods 52, 1271–1291. doi: 10.3758/s13428-019-01316-z
Mizuno, M., and Matsui, T. (2014). Orthographic similarity or semantic relation? the origin of orthographic neighborhood size effects on the lexical decision to Japanese kanji words. Jpn. J. Psychol. 85, 488–494. doi: 10.4992/jjpsy.85.13324
Mizuno, M., and Matsui, T. (2015). “The effects of word frequencies on lexical decision time: recommendation of the use of logarithms,” in The 79th Annual Convention of the Japanese Psychological Association. 1AM-072. Abstract retrieved from Proceedings of the Japanese Society for Cognitive Psychology (Tokyo: The Japanese Psychological Association).
Mizuno, R., and Matsui, T. (2018). Inhibitory effects of semantics of constituent kanji characters on semantic processing of Japanese kanji words. Jpn. J. Psychol. 89, 416–421. doi: 10.4992/jjpsy.89.17329
Mochizuki, M., and Ota, N. (2020). Relative embodiment of Japanese verbs. Int. J. Psychol. Stud. 12, 1–15. doi: 10.5539/ijps.v12n3p1
Mochizuki, M., and Ota, N. (2024). Verb processing in context: the influence of antecedent nouns on the recognition of verbs with high relative embodiment. Cogn. Stud. Bullet. Japan. Cogn. Sci. Soc. 31, 128–137. doi: 10.11225/cs.2023.068
National Institute for Japanese Language and Linguistics (2004). Word List by Semantic Principles, Revised and Enlarged Edition (Tokyo: Dainippon Tosho Co., Ltd).
Ogawa, Y., and Nittono, H. (2018). “The effects of word imageability on reaction times and event-related potentials,” in The 16th Conference of the Japanese Society for Cognitive Psychology. 101. Abstract retrieved from Proceedings of the Japanese Society for Cognitive Psychology (Fukuoka: The Japanese Society for Cognitive Psychology).
Paisios, D., Huet, N., and Labeye, E. (2023). Addressing the elephant in the middle: implications of the midscale disagreement problem through the lens of body-object interaction ratings. Collabra Psychol. 9:84564. doi: 10.1525/collabra.84564
Peirce, J., Gray, J. R., Simpson, S., MacAskill, M., Höchenberger, R., Sogo, H., et al. (2019). PsychoPy2: experiments in behavior made easy. Behav. Res. Methods 51, 195–203. doi: 10.3758/s13428-018-01193-y
Pexman, P. M., Muraki, E. J., Sidhu, D. M., Siakaluk, P. D., and Yap, M. J. (2019). Quantifying sensorimotor experience: body-object interaction ratings for more than 9,000 English words. Behav. Res. Methods 51, 453–466. doi: 10.3758/s13428-018-1171-z
Pexman, P. M., Siakaluk, P. D., and Yap, M. J. (2013). Introduction to the research topic meaning in mind: semantic richness effects in language processing. Front. Hum. Neuro., 7:723. doi: 10.3389/fnhum.2013.00723
R Core Team (2022). R: A Language and Environment for Statistical Computing. Vienna: R Foundation for Statistical Computing. Available at: https://www.R-project.org/ (accessed January 10, 2024).
Rubenstein, H., Lewis, S. S., and Rubenstein, M. A. (1971). Homographic entries in the internal lexicon: effects of systematicity and relative frequency of meanings. J. Verb. Learn. Verb. Behav. 10, 57–62. doi: 10.1016/S0022-5371(71)80094-4
Sakuma, N., Ijuin, M., Fushimi, T., Tastumi, I., Tanaka, M., Amano, S., et al. (2005). NTT Database Series. Lexical Properties of Japanese, No. 8. Imageability. Tokyo: Sanseido.
Siakaluk, P. D., Pexman, P. M., Aguilera, L., Owen, W. J., and Sears, C. R. (2008). Evidence for the activation of sensorimotor information during visual word recognition: the body-object interaction effect. Cognition 106, 433–443. doi: 10.1016/j.cognition.2006.12.011
Sidhu, D. M., Kwan, R., Pexman, P. M., and Siakaluk, P. D. (2014). Effects of relative embodiment in lexical and semantic processing of verbs. Acta Psychol. 149, 32–39. doi: 10.1016/j.actpsy.2014.02.009
Sidhu, D. M., and Pexman, P. M. (2016). Is moving more memorable than proving? effects of embodiment and imagined enactment on verb memory. Front. Psychol. 7:1010. doi: 10.3389/fpsyg.2016.01010
Takamura, H., Inui, T., and Okumura, M. (2005). “Extracting semantic orientations of words using spin model,” in Proceedings of the 43rd Annual Meeting of the Association for Computational Linguistics (ACL'05), eds. K. Knight, H. T. Ng, and K. Oflazer (Ann Arbor, MI: Association for Computational Linguistics), 133–140.
The Social Computing Laboratory (2021). Data, in AWD-J: Abstractness of Word Database for Japanese Common Words. Nara Institute of Science and Technology. Available at: https://sociocom.naist.jp/awd-j/ (accessed January 10, 2024).
Tillotson, S. M., Siakaluk, P. D., and Pexman, P. M. (2008). Body-object interaction ratings for 1,618 monosyllabic nouns. Behav. Res. Methods 40, 1075–1078. doi: 10.3758/BRM.40.4.1075
Tse, C.-S., Yap, M. J., Chan, Y.-L., Sze, W. P., Shaoul, C., and Lin, D. (2017). The Chinese lexicon project: a megastudy of lexical decision performance for 25,000+ traditional Chinese two-character compound words. Behav. Res. Methods 49, 1503–1519. doi: 10.3758/s13428-016-0810-5
University of Tsukuba National Institute for Japanese Language and Linguistics, and Lago Institute of Language. (2013). NINJAL-LWP for TWC. University of Tsukuba (National Institute for Japanese Language and Linguistics), and Lago Institute of Language. Available at: http://corpus.tsukuba.ac.jp.
Yarkoni, T., Balota, D., and Yap, M. (2008). Moving beyond Coltheart's n: a new measure of orthographic similarity. Psychon. Bull. Rev. 15, 971–979. doi: 10.3758/PBR.15.5.971
Keywords: lexical decision task, lexical processing, lexical database, mental lexicon, visual word recognition
Citation: Ota N and Mochizuki M (2025) JALEX: Japanese version of lexical decision database. Front. Lang. Sci. 3:1506509. doi: 10.3389/flang.2024.1506509
Received: 11 October 2024; Accepted: 13 December 2024;
Published: 13 January 2025.
Edited by:
José Antonio Hinojosa, Complutense University of Madrid, SpainReviewed by:
Barbara Juhasz, Wesleyan University, United StatesHeng Chen, Guangdong University of Foreign Studies, China
Copyright © 2025 Ota and Mochizuki. This is an open-access article distributed under the terms of the Creative Commons Attribution License (CC BY). The use, distribution or reproduction in other forums is permitted, provided the original author(s) and the copyright owner(s) are credited and that the original publication in this journal is cited, in accordance with accepted academic practice. No use, distribution or reproduction is permitted which does not comply with these terms.
*Correspondence: Naoto Ota, bmFvdG8uZG90Lm90YUBnbWFpbC5jb20=