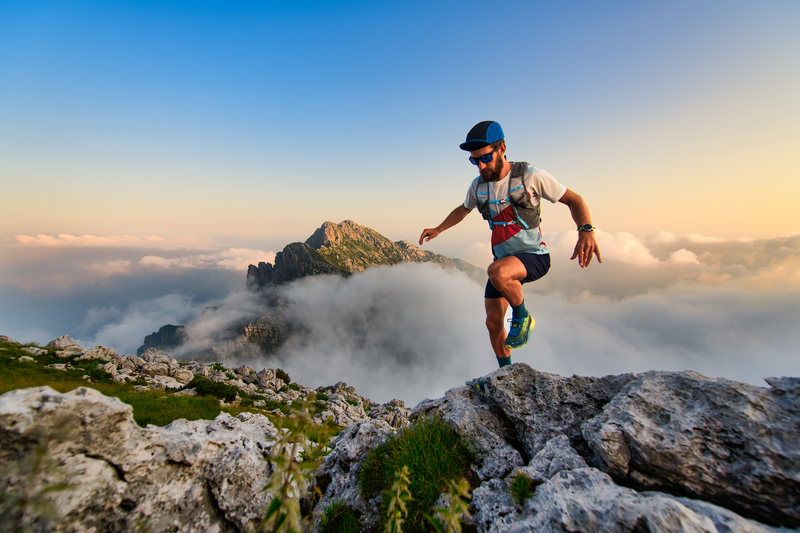
95% of researchers rate our articles as excellent or good
Learn more about the work of our research integrity team to safeguard the quality of each article we publish.
Find out more
ORIGINAL RESEARCH article
Front. Lang. Sci. , 11 December 2024
Sec. Bilingualism
Volume 3 - 2024 | https://doi.org/10.3389/flang.2024.1482861
Processing a word in a second language (L2) may be hindered or helped by the simultaneous activation of similar words present in L2 and in addition by similar words that occur in the first language (L1). Precise mechanisms for influencing variables within L2 and cross-language processing are still little understood. The current visual word recognition study explored orthographic neighborhood density (ND) effects in L2 English, replicating the effects for Dutch–English while expanding the exploration to a new language combination, Spanish–English. The within- and across-language effects were explored in a group of Dutch–English and Spanish–English bilinguals. English L2 targets were subdivided into four ND conditions: high L1 + high L2, high L1 + low L2, low L1 + high L2, and low L1 + low L2. For Experiment 1, an analysis of generalized linear mixed-effects models (GLMMs) revealed that Dutch (L1)–English (L2) bilinguals showed a facilitatory main effect of English ND on reaction times and error rates. However, an inhibitory main effect of L1 Dutch ND on L2 visual word recognition was only observed for reaction times, not error rates. Overall, no interaction was detected between L1 and L2 NDs for reaction times or error rates. Additionally, a factorial permutation test confirmed the L2 facilitatory effect on both reaction times and error rates, while it supported an L1 inhibitory effect for error rates only. In Experiment 2, a GLMM analysis replicated the L2 facilitatory effect on reaction times in Spanish (L1)–English (L2) bilinguals but did not reveal an inhibitory main effect of L1 on L2 word recognition. Instead, we found a significant interaction between English (L2) and Spanish (L1) ND. Reaction time patterns were confirmed by the non-parametric analysis, although with only a marginally significant interaction. For error rates, no effect for ND was detected using GLMMs. However, the permutation test revealed significant L2 facilitatory and L1 inhibitory effects on L2 word recognition but no significant interaction. Our data confirm a robust L2 facilitatory main effect of ND on reaction times across experiments and analyses, while L1 inhibitory main and interaction effects were less robust across experiments and analyses. The latter may be dependent on language specificity and speaker characteristics.
Across the globe, 5,000–8,000 distinct languages are spoken that differ along many dimensions (Evans and Levinson, 2009). In addition, at least half of the world's population knows and uses more than one language (Van Hell and Tanner, 2012). It is therefore unsurprising that language users are diverse, and understanding precisely how bilingual speakers navigate their two or more languages when they speak, read, write, listen, or engage in conversation remains a challenge because many more factors may influence these processes compared to a monolingual language system.
Several decades of research have provided evidence for the important role of orthographic neighborhood density (ND) in lexical decisions in monolingual readers (Andrews, 1997; Mueller Gathercole, 2010). The term orthographic neighbor was coined by Coltheart et al. (1977) and is defined as a real word (target) that differs from another word (neighbor) by one letter. Orthographic neighbors can occur by deletion of a letter (e.g., target: twin vs. neighbor: tin), by addition (target: train vs. neighbor: strain), or by substitution (e.g., target: mat vs. neighbor: cat; Davis et al., 2009). Words with many orthographic neighbors are defined as words with high ND (e.g., male has 28 English neighbors, such as pale, ale, and males; CLEARPOND, Marian et al., 2012). Words with few orthographic neighbors are defined as words with a low ND (e.g., orange, which has just three English neighbors, oranges, range, grange). Research with monolingual speakers shows that words with high NDs consistently show a facilitatory effect in written word recognition (e.g., Andrews, 1997; Sears et al., 1995). Therefore, monolinguals are more likely to be faster in a lexical decision task and make fewer errors when recognizing words with high compared to low NDs.
In bilingual individuals, visual word recognition is further complicated by the presence of cross-language neighbors from the second language (L2). For example, bear has a cross-language Spanish neighbor besar, which translates to kiss. There are only a handful of bilingual word recognition studies that explore neighborhood effects reporting varying result patterns (Dirix et al., 2017; Mulder et al., 2018; Van Heuven et al., 1998; Dijkstra et al., 1999) and only capture a small range of language combinations (Dutch–English; French–English, Norwegian–English, Welsh–English, and language-specific and language-ambiguous pseudoword stimuli; e.g., Grossi et al., 2012; Midgley et al., 2008; Oganian et al., 2015, 2016; Van Kesteren et al., 2012). The original bilingual neighborhood study by Van Heuven et al. (1998) explored, in their Experiment 4, the effects of ND on written word recognition between L1 and L2. They used an English lexical decision task in a group of Dutch (L1) and English (L2) bilinguals and English (L1) monolinguals as a control group. The authors found that the Dutch–English bilinguals were slower (but only marginally) in responding to L2 English words when there were more L1 Dutch neighbors for the target word shown, which can be interpreted as an L1-to-L2 cross-language inhibition effect. However, the authors also showed that the more orthographic neighbors the L2 English targets possessed, the faster the visual word recognition time, which can be interpreted as an L2 within-language facilitation effect. This latter effect was also shown for the L1 English monolinguals, replicating the robust L1 facilitation effect consistently reported in the literature (e.g., Andrews, 1997). However, it is of note that the interaction between Dutch L1 and English L2 NDs was not significant, and no effects were detected for error rates.
Van Heuven et al. (1998) interpreted their findings in support of an integrated bilingual lexicon and non-selective language processing because both orthographic neighborhoods seem to be activated in parallel, which would require interactive connection within and across lexica. The Multilink model (Dijkstra et al., 2019) can accommodate both assumptions. The Multilink model is a computational interactive, localist-connectionist model for visual word recognition and translation and can explain how monolingual and bilingual language processes may interact. It is based on the previous bilingual interactive activation (BIA) and bilingual interactive activation plus (BIA+) models (Dijkstra and Van Heuven, 1998, 2002) and assumes a shared lexicon between languages. Van Heuven et al.'s reaction time (RT) findings of cross-language inhibition and within-language facilitation effects can be interpreted within an integrated lexicon account such as Multilink, which entails that words from both languages are stored together in a single, shared lexicon. This also means that when an individual tries to recognize a word, all possible word candidates across both languages are activated simultaneously. However, factors such as proficiency (how well does the bilingual master each language?), language mode (language of instruction) and dominance (everyday language context) will determine whether there is competition between words and how strong this competition may be. The Multilink model implements competition by lateral inhibition links between target and non-target neighbors (for detailed explanations, see Dijkstra et al., 2019). The stronger a bilingual's L2 proficiency is, the less effective the competition from their L1 may be. The integrated lexicon assumption therefore also implies non-selective language processing (for a similar line of theories from word production, see the Revised Hierarchical Model [RHM] account by Kroll and Stewart, 1994, and the Adaptive Control Hypothesis by Green and Abutalebi, 2013). For example, in a word recognition task, the visual presentation of a word leads to co-activation of potential word candidates similar to the target word across all languages mastered by the speaker—but the co-activation may be modulated further by proficiency, language mode, and dominance, which will moderate the activation of the non-target language. As such, the Multilink model predicts that orthographic neighbors from one language have an inhibitory effect on the visual recognition of target words in another language and therefore formed a critical theoretical baseline for our study.
A replication of Van Heuven et al.'s (1998) Experiment 3 (a generalized lexical decision task) was carried out 20 years later by Dirix et al. (2017). This replication explored within-language effects within L1 and L2 and cross-language effects from L1 to L2 and L2 to L1. Because we were particularly interested in the within-effects of L2 and the effect of L1 on L2, we focus on Dirix et al.'s (2017) L2 English replication results from Van Heuven et al.'s (1998) Experiment 3 to methodologically fine-tune our present study, which replicates Experiment 4 of Van Heuven et al. (1998). While van Heuven et al. found a significant facilitatory main effect for L2 and a marginally significant cross-language effect of L1 ND on L2 word recognition in their Experiment 3, Dirix et al. (2017). found no significant main effects for L2 facilitation in RTs (although they did for error rates) and no main effect of inhibition between L1 ND and L2 word recognition. However, a significant interaction between L1 and L2 NDs was detected: longer RTs and a higher error rate for L2 English words when the L1 Dutch neighborhood was high.
A further study by Mulder et al. (2018) replicated Van Heuven et al.'s (1998) Experiment 4 but varied the proficiency of L2 English speakers compared to the original experiment. The participants all showed high L2 English proficiency, having learned English from age 11 onwards. The authors wondered whether the very fragile original cross-language inhibitory L1 ND–to–L2 effect would become stronger or disappear because English L2 targets may be less vulnerable to the influence of cross-language activation due to the “protective” high proficiency. The authors also carried out generalized linear mixed-effects model (GLMM) analyses instead of analyses of variance (ANOVAs). Mulder et al. found that participants were faster in recognizing English L2 words compared to the original 1998 study. However, the overall pattern of results differed from the original experiment: there was no effect of L1 Dutch ND on L2 English word recognition, which challenges the predictions of the Multilink model (Dijkstra et al., 2019), whereby L1 neighbors inhibit L2 word recognition. Overall, this leaves us still with an inconsistent evidence base for neighborhood effects in bilinguals, in contrast to the relatively robust facilitatory effects of orthographic neighborhood in monolingual word recognition: The higher the ND, the faster the lexical decision time and the lower the error rate.
The present study therefore replicates Van Heuven et al.'s (1998) Experiment 4 again, exploring the facilitation effect of ND within L2 and the cross-language inhibition effect, in a high-proficient L2 speaker group (following Mulder et al., 2018), while using improved ND measures to the original 1998 study (following Dirix et al.'s, 2017, approach). We then expand the same experiment to a Spanish (L1)–English (L2) speaker group, a language combination that has not been tested yet within this experimental paradigm and adds unique language-specific aspects (Spanish is a cognate-rich language compared to English and Dutch and belongs to a different language family than English and Dutch). The current evidence base suggests that within-L2 facilitation effects are consistently at play during visual word recognition in bilinguals (e.g., Mulder et al., 2018; Van Heuven et al., 1998). Such an effect has been robustly shown for monolingual readers (e.g., Andrews, 1997). Cross-language inhibition effects, however, were less consistently observed across language combinations (e.g., Dirix et al., 2017; Mulder et al., 2018). Therefore, we investigate whether the linguistic constraints of L1 (Dutch vs. Spanish) may alter cross-language activation effects in L2 English word recognition.
The current lexical decision study took Van Heuven et al.'s (1998) Experiment 4 as a reference point and drew on the orthographic ND effect to explore the interplay between L1 and L2 languages during visual word recognition in a group of Dutch (L1)–English (L2; Experiment 1) and Spanish (L1)–English bilinguals (L2; Experiment 2). Both experiments used L2 English as the target language. English target words were selected and subdivided into four ND conditions: (a) high L1 + high L2, (b) high L1 + low L2, (c) low L1 + high L2, and (d) low L1 + low L2. We hypothesized that the ND of the L2 English target words should have a facilitatory effect on lexical decision performance in English, with faster and more accurate responses in the high compared to the low–English ND conditions. In addition, we expected to see an L1 inhibitory ND effect (inhibition increases with increasing L1 ND) on the L2 English targets, which served as a test for the cross-language influences on visual word recognition. However, we kept in mind Mulder et al.'s (2018) finding that the L2 proficiency level could potentially alter the inhibition effect. Finally, the interaction between ND in L1 and L2 was examined to test the interplay between the lexical representations of the two languages. If a main effect of L1 ND activation during L2 lexical decision can be demonstrated, evidence for parallel activation between orthographic neighborhoods between the participants' L1 (Dutch/Spanish) and L2 (English) language processing is strengthened.
The rationale for running this lexical decision task with Dutch (L1)–English (L2) and with Spanish (L1)–English (L2) speakers was, first, to replicate Van Heuven et al.'s (1998) Dutch–English Experiment 4 while improving the original item set by using updated orthographic neighborhood measures. We retrieved orthographic ND and neighborhood frequency values from CLEARPOND, a database for within- and cross-linguistic orthographic and phonological neighbors (Marian et al., 2012). The CLEARPOND database provided values for the three languages used in this present study, which ensured consistency in calculating neighborhood scores across different language combinations. The lack of control and consistency across neighborhood measures was initially raised by Dirix et al. (2017) as a potential source of the inconsistent results when comparing result patterns of the available bilingual neighborhood studies. ND categories and their boundaries were calculated following Siakaluk et al. (2002) and Baus et al. (2008). We also increased participant numbers (from the 20 and 30 participants, respectively, in previous studies to ~50 participants).
Second, the choice of Spanish–English in Experiment 2 extends Van Heuven et al.'s (1998) Experiment 4 to a language combination that includes two different language sub-families not previously tested. While Dutch and English are both Germanic languages with a high overlap of orthographic, morphological, and phonological features, Spanish is a Romance language and shares less overlap with English in orthography, phonology, morphology, and lexical stress (e.g., Borleffs et al., 2017; Van Soeren, 2023).
Third, a further difference to Van Heuven et al.'s (1998) Experiment 4 was the inclusion of bilingual Dutch–English speakers who had mastered English as their L2 at a very high proficiency level (similar to Mulder et al.'s (2018), Experiment 1 replication). However, in our Experiment 2, the bilingual Spanish–English speaker group resembled the L2 proficiency level of van Heuven et al.'s participant group more closely, who had overall a lower proficiency level compared to participants included in Experiment 1.
In sum, our rationale for this study was to use a consistent database for cross-language neighborhood values across different language combinations, which can help shed light on the question of whether the observed neighborhood effects in the current literature are replicable across languages or are language-specific. L2 proficiency differences (although subtle) between the participants in Experiments 1 and 2 may provide additional information about the mechanisms of inhibition due to neighborhood influences across languages.
A total of 48 Dutch–English bilingual speakers (female: 35, male: 12, other: 1, age range: 18–42, M: 24.79, SD: 5.84) were recruited through the paid online participant database Prolific (Palan and Schitter, 2018). This study was approved by Curtin's Human Research Ethics Committee (HRE2017-0274). Participants gave consent when entering the project on Prolific and before opening the experiment in Inquisit 6.0 (Smith, 2021). Participants were required to speak Dutch (L1) and English (L2) and received monetary reimbursement. The participants' reading proficiency was examined using the English version of the LexTALE (https://www.lextale.com/takethetest.html), developed by Lemhöfer and Broersma (2012). The test involved 60 trials, including 40 words and 20 non-words. Participants were instructed to decide if the target items were existing English words or not. The test took ~5 min to complete. The LexTALE score consisted of the percentage of correct responses, corrected for the unequal proportion of words and nonwords in the test by averaging the percentages correct for these two item types (averaged % correct). One bilingual person did not complete the LexTALE and was therefore excluded. The remaining participants revealed high proficiency with close to 90% accuracy in the task (M = 87.26, SD = 8.20). In addition, a modified 5-min version of a qualitative self-reported questionnaire, the LEAP-Q (Language Experience and Proficiency Questionnaire) developed by Marian et al. (2007), was used to provide demographic information about individual language use, language exposure, and a measure of language proficiency (see Appendix A in the Supplemental material for the selective questions used). The results of the LEAP-Q showed that 48 speakers used Dutch (their L1) as their dominant language and English (their L2) as their non-dominant language. Among the participants, 31 had some knowledge of a third language but used it less frequently as their non-dominant language compared to Dutch and English (French: n = 21, German: n = 9, and Spanish: n = 1). Of the 48 participants, 17 indicated knowledge of a fourth language with non-dominant use in their everyday lives (German: n = 9, French: n = 6, and Spanish: n = 2). The average age of the participants' language acquisition for Dutch (L1) was before age 1 (M = 0.71, SD = 1.0) and for English (L2) before age 9 (M = 8.70, SD = 2.61) for all 48 participants. Their third language was usually also acquired before age 9 (M = 8.62, SD = 4.74), and their fourth language, at approximately 10 years of age (M = 10.01, SD = 5.71) but with minimal language use in their everyday lives.
Eighty English target words were selected and subdivided into four ND conditions: high L1 + high L2, high L1 + low L2, low L1 + high L2, and low L1 + low L2 following Van Heuven et al.'s (1998) original English target words and non-words from their Experiment 4. We improved the item sets by applying improved ND measures calculated in the CLEARPOND cross-linguistic orthographic and phonological neighborhood database (Marian et al., 2012). In addition, we controlled for neighborhood frequency (based on averaged written word frequency per million; Marian et al.) across conditions.
The values for high and low neighborhoods were retrieved from the CLEARPOND database that listed available neighbors across different frequencies. This meant that our definition of high ND consisted of English target word subsets that had a mean number of neighbors of more than five within language (English) and across language (Dutch). All English target word lists with a mean neighborhood size of five or less were classified as low ND within and across language. This change of definition turned the high–low N condition into a high–high condition; hence, we ran secondary analyses (see the Results section) with a reduced subset (excluding five items from van Heuven's original low–high N subset: butt, lion, poor, nude, and noon) that brought the high Dutch–low English neighborhood condition back within the boundaries of our low versus high neighborhood definition (mean low ND ≤ 5; mean high ND ≥5). Table 1 shows the mean item characteristics for item lists per condition. The mean characteristics of the reduced low- versus high-ND condition (n = 15) are indicated in parentheses. The original word target subsets are based on 20 target words. The full list of Dutch–English materials including nonwords can be found in Appendix B in the Supplemental material.
Table 1. Mean item characteristics for Experiment 1 (standard deviations are provided in parentheses).
Stimuli were presented in the center of a computer screen using Inquisit 6.0 (Smith, 2021). Each trial consisted of a blank black screen for 150 ms, followed by a fixation stimulus in the center of the screen for 750 ms, followed by the target letter string that was displayed in lowercase between two horizontal white lines and remained there until the participant responded “yes” (stimulus is an English word) or “no” (stimulus is not an English word) by pressing the “N” or “M” key, respectively. Feedback was provided by changing the color of the white lines to either green (correct) or red (incorrect). Participants were instructed to respond as quickly and accurately as possible. After completing the lexical decision task, participants were presented with the English version of LexTALE and the LEAP-Q questionnaire. The entire session lasted for ~45 min.
We analyzed our results using R Statistical Software (version 4.2.2) and R Studio (version 1.2.5033; R Core Team, 2021). We employed the glmer function from the lme4 package (version lme4_1.1-35.1: Bates et al., 2015) to fit GLMMs to both RT and error rate data. For the RT data, we used the same glmer function to fit a gamma GLMM. This model included Dutch (L1) and English (L2) ND as fixed factors and featured random intercepts for both participants and words. We applied the “identity” link function to this model. For error rate, we initially aimed to include both Dutch and English NDs as fixed factors and intended to use participants and words as random factors within a logistic GLMM. However, in the second experiment, the model with both random factors did not converge. Therefore, we decided to fit a simpler model for error rate data across both experiments, featuring participants as the only random factor. This assumption implies that all word stimuli exert the same effect across participants. We used the “logit” link function for these models.
In addition, to verify the robustness of our main analysis, we also analyzed the aggregated data using the ezPerm function from the ez package (version ez_4.4-0: Lawrence, 2016). This function allowed us to perform a factorial permutation test, serving as a non-parametric alternative to an ANOVA that is less sensitive to violations of parametric assumptions (e.g., normality and homogeneity of variances), which can be subtly violated without detection. The results of the ezPerm function (10,000 permutations) are presented along with those of the main analysis using the glmer function.
Non-word fillers were excluded from the analyses. Lexical decisions to English (L2) word targets were analyzed as follows: Incorrect responses were removed from the RT analysis (3.5% of all data). All correct trials were above 200 ms and below 2,500 ms (293–2,498 ms), and therefore, no additional trials were discarded based on RT values. The mean model estimates for RTs and error rates for each condition are presented in Figure 1, Table 2.
Figure 1. (A) Reaction time (ms) as a function of Dutch and English neighborhoods. (B) Error rate as a function of Dutch and English neighborhoods. The plots show the estimates from the linear mixed effects model, and error bars represent standard errors of the mean.
Table 2A. Estimated marginal means of response times by Dutch (L1) and English (L2) neighborhood density conditions (Experiment 1).
Table 2B. Estimated marginal means of error ratios of Dutch (L1) and English (L2) neighborhood density conditions (Experiment 1).
As depicted in Figure 1A, there was a main facilitatory effect of L2 ND on response times (χ2 = 8.28, df = 1, p = 0.004). We observed faster response times for words with high-L2 ND when recognizing words in L2. There was also a main inhibitory effect of L1 ND on L2 response times (χ2 = 4.17, df = 1, p = 0.041), with slower latencies observed when high-density L2 words also had high-density L1 neighbors. However, the interaction between L2 (English) and L1 (Dutch) was not statistically reliable (χ2 = 1.65, df = 1, p = 0.20). The non-parametric permutation analysis partially supported these results: The effect of L2 ND was significant (p = 0.0002), while the effect of L1 ND (p = 0.26) was not. As for the primary analysis, the interaction (p = 0.28) between L2 and L1 NDs was also not significant. This indicates that the facilitatory effect of L2 ND is robust, whereas the inhibitory effect of L1 ND on L2 word recognition is less reliable.
For the error rate analysis, the main facilitatory effect of L2 ND on error rate was statistically significant (χ2 = 17.21, df = 1, p < 0.0001), with higher error rates for words with low (L2) ND as shown in Figure 1B. However, there was no main inhibitory effect of L1 ND on the error rate (χ2 = 1.74, df = 1, p = 0.19). As for the latency analysis, we did not detect an interaction between L2 (English) and L1 (Dutch) NDs (χ2 = 0.028, df = 1, p = 0.866). The non-parametric analysis also provided support for the facilitatory effect of L2 ND on error rate (p < 0.0001) but in contrast to the GLMM analysis provided evidence for an inhibitory effect of L1 ND on error rate (p = 0.0073). The interaction between L1 and L2 NDs was again not significant (p = 0.2291). This indicates that both L2 and L1 NDs affect error rates, with the within-language facilitatory effect of L2 ND being more robust, whereas the inhibitory effect of L1 ND on L2 word recognition seems less robust given that only the non-parametric (but more conservative) analysis picked up a significant effect.
A secondary analysis was conducted with the reduced set of 15 items for the high-ND (L1) and low-ND (L2) condition (excluding poor, noon, nude, butt, and lion) to improve low- and high-ND boundaries using our improved ND criteria (mean low ND ≤ 5; mean high ND ≥5), which was not met when using the van Heuven original word list for this particular subset (here the ND boundary cut-off was 3 but only for this subset). This modification improved the high–Dutch ND and low–English ND condition.
This secondary analysis of RTs yielded results consistent with our primary findings: a facilitatory within-language L2 effect (English) of ND on RT, whereby the higher the neighborhood, the faster the lexical decision performance (χ2 = 20.19, df = 1, p < 0.0001; non-parametric: p < 0.0001). There was also an inhibitory effect of the L1 (Dutch) neighborhood on lexical decision performance in L2 (χ2 = 4.74, df = 1, p = 0.03): the higher the L1 neighborhood, the slower the L2 lexical decision, especially for the L2 high-density condition. However, the non-parametric analysis did not reach significance (p = 0.0983). No interaction between L2 (English) and L1 (Dutch) ND was observed (χ2 = 0.09, df = 1, p ≤ 0.76; non-parametric: p = 0.71). While there is less evidence for the effect of L1 ND on L2 word recognition RT in the non-parametric analysis, both analyses are consistent in highlighting the robust facilitatory effect of L2 ND on latency.
The analysis for error rate also provided consistent results with the primary analysis. A main facilitatory effect of L2 (English) neighborhood on error rate was evident (χ2 = 24.52, df = 1, p < 0.0001), with a higher error rate for words with a lower neighborhood size. the inhibitory effect of L1 (Dutch) neighborhood on lexical decision responses in L2 was also not significant for error rates (χ2 = 1.73, df = 1, p =0.19), and no interaction was detected between L2 (English) and L1 (Dutch) (χ2 = 0.52, df = 1, p = 0.47). However, while the non-parametric analysis supported the effect of L2 ND on error rates (p < 0.0001), it also revealed an inhibitory effect of L1 neighborhood on L2 word recognition (p = 0.0005) and an interaction between L1 and L2 NDs (p = 0.0040), which was not detected with the GLMM analysis. This indicates that while the facilitatory effect of L2 ND is reliable, the non-parametric analysis suggests additional influences of L1 ND and its interaction with L2 density, aligning closely with the visual inspection of the results in Figure 1B.
We found faster RTs and lower error rates for L2 English target words with high L2 ND which translated to a facilitatory within-neighborhood effect for the L2 target language. Such facilitation effect of high-L1 orthographic ND on L2 lexical decision matches with findings from the monolingual literature, where a higher ND benefits word recognition times (e.g., Andrews, 1997). We also observed an inhibitory effect of L1 (Dutch) ND on L2 latencies; however, this effect was less robustly detected across all analyses, with the clearest inhibition effect shown for the non-parametric error analysis. However, a weaker L1-to-L2 cross-language inhibition effect compared to the L2 facilitation effect is in line with Van Heuven et al.'s (1998) original Experiment 4 findings, where only a marginal cross-linguistic effect was detected in the L2 word recognition task but a strong L2 facilitatory effect was found. We also could not confirm a significant interaction between Dutch ND and English ND for RTs and error rates, which is also in line with van Heuven's original Experiment 4 results. The L2 within-language facilitation effect clearly replicates Van Heuven et al.'s (1998) Experiment 4 findings and confirms the pattern found consistently for monolingual visual word recognition, with more neighbors helping process the word target (e.g., Andrews, 1997). This effect can be explained well in the Multilink model (Dijkstra et al., 2019), with links between L2 neighbors and the L2 target language being more strongly activated. The stronger the activation, the faster the response time and the lower the error rate.
In Experiment 2, we explore the same effects of cross-language ND in a different language combination, Spanish–English, two languages that have not been tested for bilingual orthographic neighborhood influences. Spanish (Romance) and English belong to different sub-families, while Dutch and English belong to one sub-family (Germanic). Expanding to a different language population was the main purpose of Experiment 1. However, we need to acknowledge that participants in Experiment 2 also had slightly lower proficiency levels compared to Experiment 1. An even slightly lower L2 proficiency level may bring out a stronger cross-language inhibition effect because weaker inhibitory links are at play that can “protect” the influence from cross-linguistic orthographic neighbors from L1.
The target population were 49 native Spanish speakers (22 females, 27 males), fluent in English as their second language (age range: 19–54, mean age 28.73, SD = 8.16) with no cognitive or vision impairment. Participants were recruited via the paid online participant recruitment database Prolific (Palan and Schitter, 2018) and provided consent upon entering the online project in Inquisit 6.0 (Smith, 2021). Experiment 2 was approved by Curtin's Human Research Ethics Committee (HRE2017-0274). As in Experiment 1, participants' reading proficiency was examined using the English version of the LexTALE (Lemhöfer and Broersma, 2012; https://www.lextale.com/takethetest.html). Participants revealed high proficiency, with an average of 80% accuracy in this task (M = 80.33%, SD = 9.86). In addition, a modified 5-min version of a qualitative self-reported questionnaire, LEAP-Q (Marian et al., 2007) was used to provide demographic information about individual language use, language exposure, and language proficiency. The results of the modified LEAP-Q showed that out of the 49 participants, 49 indicated Spanish as their dominant L1, 47 participants indicated English as their second but non-dominant language (L2), while 2 participants indicated Catalan and Italian. Among participants, 22 indicated French (n = 13), Portuguese (n = 2), English (n = 2), Italian (n = 2), Korean (n = 1), German (n = 1) and Valencian (n = 1) as their third language, and 6 participants stated that they spoke German (n = 3), French (n = 2), Swedish (n = 1), and Catalan (n = 1) as their fourth frequently used language. Participants acquired their L1 and L2 as follows: Spanish as L1 was acquired on average at approximately age 1 (M = 1.22, SD = 1.55), while the majority (n = 47) acquired their L2 English on average before age 8 (M = 7.67, SD = 4.84). Their third language was acquired at approximately age 13 (M = 13.21, SD = 5.84), and their fourth language, at approximately age 17 (M = 17.25, SD = 7.9).
It needs to be emphasized that the Spanish–English bilinguals in Experiment 2 showed lower English L2 proficiency (80%) compared with the English L2 proficiency (90%) of the Dutch–English bilinguals in Experiment 1. We therefore monitored whether this small alteration in L2 proficiency level may already contribute to the potential interference effects of L1.
The stimuli set consisted of 48 English words and 48 non-words. As in Experiment 1, the items were selected such that they formed four ND conditions: high L1 + high L2, high L1 + low L2, low L1 + high L2, and low L1 + low L2. Neighbors were extracted from the CLEARPOND (Marian et al., 2012). As for Experiment 1, all target words were four-letter words (see Table 2 for mean item characteristics per subset). ND was closely matched across conditions. Orthographically complex words (e.g., compound words, plural words), pronouns, slang, abbreviations, and Spanish homographs were excluded; although we made all efforts to exclude Spanish cognates, we could not achieve this for the high-ND Spanish–low-ND English condition to ensure the minimum number of items (n = 12) for this condition. Overall, fewer Spanish–English item pairs that matched our inclusion criteria were available compared to the Dutch–English item sets because we wanted to keep cognate words to a minimum. The fact that we could not find even 12 non-cognate words with high-L1 ND–low-L2 ND suggests that non-cognate processing in this particular condition is a rare process. While Spanish and English span across two language types (Romance–Germanic), Dutch–English item pairs both belong to one language type (Germanic), with a greater overlap in phonological, morphological, and orthographic features, and need to be recognized as having language-specific differences that cannot be overcome in an experimental manipulation as it would potentially miss detecting whether the observed neighborhood effects may be language-dependent. As for Experiment 1, orthographic ND and frequency measures were extracted from CLEARPOND. Based on Baus et al. (2008), neighborhood frequency was defined as the mean frequency of the target word's neighborhood in the current study. A set of 48 non-words was included for the lexical decision task but was not included in the analysis. One- and two-syllable non-words were initially extracted from the Australian Research Council (ARC) non-word database (Rastle et al., 2002) and then checked for real-word orthographic neighbors for English and Spanish in CLEARPOND (Marian et al., 2012). For each neighborhood condition, 12 non-words were then chosen. A native Spanish speaker ensured that the non-words were not real words in Spanish (and English). The full list of materials is provided in Appendix C in the Supplemental material, and Table 3 shows the mean item characteristics for each item set.
Table 3. Mean item characteristics for Experiment 2 (standard deviations are provided in parentheses).
The procedure was identical to Experiment 1.
As in Experiment 1, non-word fillers were excluded from the analyses. Incorrect responses were removed from the RT analysis (8.4 % of all data). Correct trials ranged from 350 ms to 2,244 ms, and therefore, no additional trials were discarded based on RT values. Results are depicted in Figure 2, Table 4.
Figure 2. (A) Reaction time (ms) as a function of English and Spanish neighborhood. (B) Error rate as a function of English and Spanish neighborhoods. The plots show the estimates from the linear mixed effects model, and error bars represent standard errors of the mean.
Table 4A. Estimated marginal means of response times of Spanish (L1) and English (L2) neighborhood density conditions (Experiment 2).
Table 4B. Estimated marginal means of error ratios of Spanish (L1) and English (L2) neighborhood density conditions (Experiment 2).
The main facilitatory effect of L2 ND (English) on RT reached statistical significance (GLMM: χ2 = 5.79, df = 1, p = 0.016, non-parametric: p = 0.0091). As shown in Figure 2A, this result suggests that words with high NDs in L2 may lead to faster RTs. The main effect of L1 Spanish ND on L2 English word recognition did not reach statistical significance (GLMM: χ2 = 0.25, df = 1, p = 0.62; non-parametric: p = 0.938). However, the interaction between L2 and L1 NDs was statistically significant (GLMM: χ2 = 5.08, df = 1, p = 0.024; and marginally significant for the non-parametric analysis: p = 0.0589), suggesting an inhibitory effect of L1 neighborhood on low-density L2 target words but less so on high-density L2 target words.1 We further followed up on the interaction by testing whether L1 Spanish ND had a significant effect for the low-L2 English ND conditions using the emmeans package (version 1.10.2; Lenth, 2024). The contrast between low and large L1 Spanish ND and low-L2 English ND was not significant (estimate = 17.7, p = 0.2915), indicating that the L1 Spanish ND effect is not statistically significant for any condition when L2 English ND is low.
As shown in Figure 2B, error rates visually seemed to improve in the high-L2 ND condition. While this effect was not significant for the GLMM analysis (χ2 = 1.95, df = 1, p = 0.16), it was detected by the non-parametric factorial permutation analysis (p = 0.0122). Similarly, while the cross-language inhibitory effect of L1 Spanish density on L2 English word recognition did not reach statistical significance in the GLMM analysis (χ2 = 2.87, df = 1, p = 0.09), it was detected by the non-parametric analysis (p = 0.0274), suggesting a possible cross-language influence of L1 Spanish neighbors onto L2 English targets. The interaction for error rates between L1 and L2 NDs was not statistically reliable in both statistical approaches (χ2 = 0.039, df = 1, p =0.84, non-parametric: p = 0.9181).
The results of Experiment 2 replicated the main facilitatory effect for L2 lexical decision from Experiment 1. There was a main facilitatory within-L2 ND effect for word recognition RTs: Target words with a higher neighborhood showed faster word recognition. While we could not observe a main inhibitory effect of L1 ND on L2 RTs (as we observed in Experiment 1), we found a significant interaction between orthographic neighborhood sizes for L1 Spanish and L2 English, indicating that the greatest amount of cross-language interference was observed when low-ND L2 target words had high ND in L1.
For the error analysis, while GLMM analyses could not reveal significant main effects, the more conservative non-parametric analysis showed a main facilitatory effect of L2 ND on the error rate and a significant effect of L1 neighborhood on L2 word recognition. No significant interaction was observed. A significant interaction has not been previously detected either by Van Heuven et al. (1998) or in our Experiment 1. Hence, our Spanish–English data may hint at a language-dependent effect and is not necessarily bound to a bilingual group with higher proficiency in their L2 English. However, the fact that the strongest cross-language effects were found for L2 targets with high ND in their L1 is in line with the predictions made by Multilink (Dijkstra et al., 2019) of parallel activation across languages. Multilink assumes an integrated lexicon; language comprehension is non-selective. Hence, bidirectional interaction can flow freely across levels resulting in cross-language activation.
In this study, two bilingual speaker groups with two different language backgrounds (Experiment 1: Dutch [L1]–English [L2]; Experiment 2: Spanish [L1]–English [L2]) were tested on their ability to recognize words in their L2 (English) while manipulating orthographic NDs within and across language(s). We used four different word conditions. Each stimulus set consisted of L2 English target words and non-words. The participants were asked to decide whether the stimulus presented on the screen was a real word. For both experiments, items were selected such that they formed four ND conditions: high L1 + high L2, high L1 + low L2, low L1 + high L2, and low L1 + low L2. The primary goal of the current study was to replicate and extend Van Heuven et al.'s (1998) Experiment 4 to another language combination that comprised a language pair that (a) belonged to two different language families and (b) for which the effect of L1 neighborhood on L2 word recognition has not yet been explored. (c) We also wanted to understand the role of proficiency in a more nuanced way (based on the findings of Mulder et al., 2018), hence we recruited participants with an overall high-L2 proficiency but with some nuanced differences between speaker groups. We addressed methodological inconsistencies of previous experimental designs (Van Heuven et al., 1998) by using updated ND and frequency measures based on CLEARPOND (Marian et al., 2012) following Dirix et al.'s (2017) instructions for their replication of Van Heuven et al.'s Experiment 3. Because the CLEARPOND database did not exist when van Heuven et al.'s work was published, van Heuven et al. only counted substitution neighbors when calculating their ND values (based on Baayen et al., 1993). However, we added addition and deletion neighbors into the ND measure for L1 and L2 (taken from CLEARPOND). Like Dirix et al. (2017), we were hoping to improve the ND measure to detect a stronger cross-linguistic effect (if present) while replicating Experiment 4.
Overall, our study revealed several findings.
The first finding is a robust within-language facilitatory effect of L2 ND on L2 target word recognition. We observed faster RTs and lower error rates in both experiments: The higher the ND within L2, the faster the RTs and the lower the error rates, which replicates the main facilitatory effect found in Van Heuven et al.'s (1998) Experiment 4 for L2 in bilinguals and L1 in monolinguals. Our study extends this finding to a new language combination: Spanish–English (Experiment 2).
The second finding is an inhibitory ND effect from L1 ND on L2 word recognition, even though less robust across both experiments and analyses. We observed for both Dutch–English and Spanish–English bilinguals a cross-language effect for RTs. We interpreted this latter finding as evidence for cross-language influences from L1 on L2, which indicates cross-language processing. This partially replicated the effect noted by Van Heuven et al.'s (1998), who found that the Dutch–English bilinguals were slower and made more errors when the English target word had a higher number of Dutch neighbors. However, this effect was only marginally significant.
Considering earlier replication studies of Van Heuven et al.'s (1998) Experiment 4, Mulder et al. (2018) only included high-proficiency L2 speakers and—what the authors interpret as a consequence—could not confirm a cross-language Dutch–English inhibition effect and only demonstrated that the RT data was overall faster compared to the original L2 RT data presented in the original van Heuven et al. Experiment 4 results. Our data could not confirm Mulder et al.'s pattern: Our highly proficient L2 Dutch–English speaker group (close to 90%) displayed a clear inhibition effect compared to van Heuven et al.'s fragile inhibition effect; hence, Mulder et al.'s finding that the inhibition effect disappears with increasing proficiency could not be confirmed in our data. Instead, our results from Experiment 2, demonstrated that there can be a significant interaction between L1 and L2 neighborhoods, even in a speaker group with lower proficiency (close to 80%).
What are the theoretical implications for these result patterns across language combinations? How do the L1 (Dutch/Spanish) neighbors influence L2 (English) lexical decisions? Dijkstra et al.'s (2019) Multilink model has the features and processing assumptions that are needed to explain inhibitory cross-language and facilitatory within-language ND effects. Multilink allows for parallel activation of two or more languages because it assumes an integrated lexicon and proposes that language comprehension is non-selective. This is in line with our findings because we found an effect of L1 neighborhood on L2 word recognition. The interactive (bidirectional) links across levels can further explain the cross-language activation since activation can flow freely.
The Multilink model implements L2 proficiency as resting level activations that can differ from L1 word entries and reflect differences in language-dependent usage. Due to higher L2 resting levels in low-proficient speakers, a stronger influence from L1 may affect and interfere with L2 word recognition. While we found L1 inhibitory links for both language groups, they manifested differently, and with a clear significant interaction found for the slightly less proficient Spanish–English bilinguals compared to Dutch–English bilinguals.
The resting level assumption proposed in the Multilink can capture the inhibition pattern we found in our data. It is possible that Spanish–English bilinguals may have been less able to suppress cross-language lexical activation during the English lexical task and therefore were more prone to be impacted by L1 ND effects when reading in their second language. This reasoning also aligns with the now classic RHM (account by Kroll and Stewart, 1994) and the Adaptive Control Hypothesis (Green and Abutalebi, 2013). The RHM provides an account for individual differences in L1 and L2 processing by considering dominance. This model predicts that speakers with weak proficiency need mediation via the translation of their L1, whereas a speaker with high proficiency can process L2 representations directly linked via the semantic system. In addition, bilingual language processing also needs to be modulated by language control (Green, 1998). Depending on the speaker's context, control mechanisms may differ among bilingual speakers. How much control is needed to resolve competition and boost facilitation when recognizing a word in a target language that is the L2 language of the speaker depends on factors such as linguistic demands (Is L2 the dominant language? What is the proficiency level of L2?) and the communicative context (What is the task? Does the word need to be produced aloud or understood silently?). Hence, interference suppression, regulation of conflict between languages, and the ability to switch to another language when necessary are needed. The Adaptive Control Hypothesis proposes that control processes change for different contexts. For example, in the context of single-word recognition in the speaker's L2, suppressing L1 is necessary if the speaker's L2 proficiency is low. This therefore explains the decreased interference from L1 in our groups of Spanish–English bilinguals, due to weaker suppression control mechanisms of L1 and the higher dominance of their L2 compared to our group of Dutch–English bilinguals.
However, our data do not align with Mulder et al.'s (2018) findings. They argued that higher proficiency in L2 (above 80%) may be the reason for the absence of an inhibition interference effect because higher proficiency “protects” from interference from the L1. We found, however, still a stronger inhibition effect in Dutch–English proficiency, with a higher proficiency compared to the original Van Heuven et al.'s (1998) Experiment 4. One interesting way to build on these findings in future research may be to more systematically vary L2 English proficiency within each speaker group, especially in the lower proficiency ranges. This would provide further insights into the interplay between L2 proficiency and the linguistic properties of L1 in cross-language orthographic neighborhood studies. We acknowledge that one of the limitations of our study is that the proficiency gap between the speaker group in Experiment 1, with an average proficiency level of close to 90%, and the speaker group in Experiment 2, with an average proficiency level of 80%, was not great enough to speak to the potential inhibition effects in the low-proficiency ranges. In addition, we observed that our Spanish–English bilinguals learned a third or fourth language, on average, later in life compared to our Dutch–English speaker group. However, it is interesting to note that, overall, the Spanish–English speaker group spoke a greater range of languages (a higher number of third and fourth languages), which also may have interfered with their L2 language use. This latter point needs to remain a speculation because we did not control for or analyze any further language influences, but it could be an interesting future research question.
A further explanation for the significant interaction between L1 and L2 orthographic ND effect for the Spanish–English language combination (Experiment 2) that was not observed in Dutch–English bilinguals (Experiment 1) may lie in the larger language-specific differences between Spanish and English compared to Dutch and English. Spanish is a language that has, on average, a higher orthographic ND than Dutch or English (Marian et al., 2012). Our subsets reflected this as our high ND group showed a higher Spanish ND (mean ND ranged between 10 and 11) compared to the high-ND Dutch and high-ND English subgroups (mean range between 5 and 8; see Tables 1, 3). A higher ND typically requires a more careful examination of the orthographic input. It is possible that speakers of orthographically dense languages like Spanish may adopt a reading strategy that involves a more fine-tuned orthographic analysis compared to speakers of orthographically less dense languages like Dutch by which a generally higher level of interference during visual word processing may be reached. This, in turn, would explain the observed interplay between the L1 and L2 ND effects in the Spanish–English bilingual participant group.
Another language-specific difference became apparent in the Spanish high–English low ND condition. This latter condition contained a high proportion of cognates. Because, overall, Spanish had a smaller subset for each condition (12 items per condition compared to 20 items per condition for the Dutch–English in Experiment 1), we could not afford to exclude more items from any subset. Initially, we considered this difference as a potential influence on our effects found; however, an additional analysis that controlled for cognate status in Experiment 2 showed no change in results pattern (see text footnote 1). Cognate status had no significant influence on the interaction between L1 and L2 for RTs; the interaction remained significant. Therefore, while including cognates in Experiment 2 constitutes a language-specific difference from Experiment 1, the results pattern was not driven by cognate status.
The current study used tightly controlled sets of materials to examine the influence of L1 and L2 NDs on lexical decisions in Dutch–English (Experiment 1) and Spanish–English bilinguals (Experiment 2). Across both experiments, a robust facilitatory effect of ND of the target language on RT (i.e., L2 English) was observed. An inhibitory effect of ND from L1 (i.e., Dutch and Spanish, respectively) on L2 word recognition was also observed but manifested less consistently across RT and error rate measures for both experiments but provides a convincing effect for cross-lingual activation between L1 and L2 neighborhoods and L2 visual word recognition. This, in turn, supports the theoretical assumption proposed by Dijkstra et al. (2019) of an integrated lexicon and non-selective language activation. We therefore conclude that our data support that language processing in bilinguals operates in a parallel, non-selective fashion, but that the effect may be shaped by language-specific characteristics. For example, the results of Experiment 2 show that the interplay between L1 and L2 ND is particularly dynamic in Spanish–English bilinguals, suggesting that cross-language neighborhood effects do not necessarily generalize across languages, a point that will provide fertile grounds for future investigations including a greater variety of language combinations across language sub-families. The role of speakers' characteristics such as proficiency of the L2 needs further exploration but may be an additional factor for cross-language effects being present or absent. This study is the first that provides cross-linguistic neighborhood data for Spanish–English bilinguals.
The original contributions presented in the study are included in the article/Supplementary material, further inquiries can be directed to the corresponding author. Additionally, the dataset for both studies in this article can be found in Figshare: https://figshare.com/s/e4ee186670ae4097e9cc.
The studies involving humans were approved by Curtin's University Human Research Ethics Committee (approval number: HRE2017-0274). The studies were conducted in accordance with the local legislation and institutional requirements. The participants provided their written informed consent to participate in this study.
BB: Conceptualization, Funding acquisition, Investigation, Methodology, Project administration, Resources, Supervision, Validation, Writing – original draft, Writing – review & editing, Data curation. EB: Conceptualization, Formal analysis, Methodology, Supervision, Validation, Writing – original draft, Writing – review & editing. MB: Conceptualization, Methodology, Supervision, Writing – review & editing, Writing – original draft. CM: Data curation, Investigation, Software, Writing – original draft, Project administration, Writing – review & editing. AR: Data curation, Investigation, Software, Writing – original draft, Project administration, Writing – review & editing. WM: Conceptualization, Data curation, Formal analysis, Methodology, Resources, Software, Supervision, Validation, Visualization, Writing – original draft, Writing – review & editing.
The author(s) declare financial support was received for the research, authorship, and/or publication of this article. This research was conducted while Britta Biedermann and Welber Marinovic were each funded by an Australian Research Council Discovery Project (Britta Biedermann: DP190101490; Welber Marinovic: DP180100394). Elisabeth Beyersmann was supported by an ARC Discovery Early Career Researcher Award (DECRA, DE190100850).
We thank Dr. Nicolas Dirix for valuable feedback on an earlier version of this manuscript.
The authors declare that the research was conducted in the absence of any commercial or financial relationships that could be construed as a potential conflict of interest.
The author(s) declared that they were an editorial board member of Frontiers, at the time of submission. This had no impact on the peer review process and the final decision.
All claims expressed in this article are solely those of the authors and do not necessarily represent those of their affiliated organizations, or those of the publisher, the editors and the reviewers. Any product that may be evaluated in this article, or claim that may be made by its manufacturer, is not guaranteed or endorsed by the publisher.
The Supplementary Material for this article can be found online at: https://www.frontiersin.org/articles/10.3389/flang.2024.1482861/full#supplementary-material
1. ^We controlled for cognate status in Experiment 2 in an additional mixed-effects model analysis, and the results were qualitatively the same. We still found a significant interaction between L1 and L2 for RT when cognate status was added as a binary co-variable. The analysis showed that cognates were not a significant predictor of latency, χ2(1) = 1.25, p = 0.264.
Andrews, S. (1997). The effect of orthographic similarity on lexical retrieval: resolving neighborhood conflicts. Psychon. Bullet. Rev. 4, 439–461. doi: 10.3758/BF03214334
Baayen, R. H., Piepenbrock, R., and Gulikers, L. (1993). The CELEX Lexical Database (CD-ROM). Linguistic Data Consortium. Philadelphia, PA: University of Pennsylvania.
Bates, D., Mächler, M., Bolker, B., and Walker, S. (2015). Fitting linear mixed-effects models using lme4. [Version lme4_1.1-35.1]. J. Statist. Softw. 67, 1–48. doi: 10.18637/jss.v067.i01
Baus, C., Costa, A., and Carreiras, M. (2008). Neighbourhood density and frequency effects in speech production: a case for interactivity. Lang. Cognit. Proc. 23, 866–888. doi: 10.1080/01690960801962372
Borleffs, E., Maassen, B. A., Lyytinen, H., and Zwarts, F. (2017). Measuring orthographic transparency and morphological-syllabic complexity in alphabetic orthographies: a narrative review. Read. Writing 30, 1617–1638. doi: 10.1007/s11145-017-9741-5
Coltheart, M., Davelaar, E., Jonasson, J. T., and Besner, D. (1977). “Access to the internal lexicon,” in Attention and Performance VI, ed. S. Dornič (London: Routledge), 535–555.
Davis, C. J., Perea, M., and Acha, J. (2009). Re (de) fining the orthographic neighborhood: the role of addition and deletion neighbors in lexical decision and reading. J. Experim. Psychol. 35, 1550–1570. doi: 10.1037/a0014253
Dijkstra, A., and Van Heuven, W. J. B. (1998). “The BIA-model and bilingual word recognition,” in Localist Connectionist Approaches to Human Cognition, eds. J. Grainger and A. Jacobs (Mahwah, NJ: Lawrence Erlbaum Associates), 189–225.
Dijkstra, A., and Van Heuven, W. J. B. (2002). The architecture of the bilingual word recognition system: from identification to decision. Bilingualism 5, 175–197. doi: 10.1017/S1366728902003012
Dijkstra, T., Grainger, J., and van Heuven, W. (1999). Recognition of cognates and interlingual homographs: the neglected role of phonology. J. Memory Lang. 41, 496–518. doi: 10.1006/jmla.1999.2654
Dijkstra, T., Wahl, A., Buytenhuis, F., van Halem, N., Al-jibouri, Z., de Korte, M., et al. (2019). Multilink: a computational model for bilingual word recognition and word translation. Bilingualism 22, 657–679. doi: 10.1017/S1366728918000287
Dirix, N., Cop, U., Drieghe, D., and Duyck, W. (2017). Cross-lingual neighborhood effects in generalized lexical decision and natural reading. J. Exp. Psychol. 43, 887–915. doi: 10.1037/xlm0000352
Evans, N., and Levinson, S. C. (2009). The myth of language universals: language diversity and its importance for cognitive science. Behav. Brain Sci. 32, 429–448. doi: 10.1017/S0140525X0999094X
Green, D. W. (1998). Mental control of the bilingual lexico-semantic system. Bilingualism 1, 67–81. doi: 10.1017/S1366728998000133
Green, D. W., and Abutalebi, J. (2013). Language control in bilinguals: the adaptive control hypothesis. J. Cognit. Psychol. 25, 515–530. doi: 10.1080/20445911.2013.796377
Grossi, G., Savill, N., Thomas, E., and Thierry, G. (2012). Electrophysiological cross-language neighborhood density effects in late and early English-Welsh bilinguals. Front. Psychol. 3:408. doi: 10.3389/fpsyg.2012.00408
Kroll, J. F., and Stewart, E. (1994). Category interference in translation and picture naming: Evidence for asymmetric connections between bilingual memory representations. J. Memory Lang. 33, 149–174. doi: 10.1006/jmla.1994.1008
Lawrence, M. A. (2016). ez: Easy Analysis and Visualization of Factorial Experiments (Version 4.4-0) [R package]. Available at: https://CRAN.R-project.org/package=ez (acceessed November 17, 2024).
Lemhöfer, K., and Broersma, M. (2012). Introducing LexTALE: a quick and valid lexical test for advanced learners of English. Behav. Res. Methods 44, 325–343. doi: 10.3758/s13428-011-0146-0
Lenth, R. V. (2024). emmeans: Estimated Marginal Means, aka Least-Squares Means (Version 1.10.2) [R package]. Available at: https://rvlenth.github.io/emmeans/ (acceessed November 17, 2024).
Marian, V., Bartolotti, J., Chabal, S., and Shook, A. (2012). CLEARPOND: cross-linguistic easy-access resource for phonological and orthographic neighborhood densities. PLoS ONE 7:e43230. doi: 10.1371/journal.pone.0043230
Marian, V., Blumenfeld, H. K., and Kaushanskaya, M. (2007). The language experience and proficiency questionnaire (LEAP-Q): assessing language profiles in bilinguals and multilinguals. J. Speech Lang. Hear. Res. 50, 940–967. doi: 10.1044/1092-4388(2007/067)
Midgley, K. J., Holcomb, P. J., van Heuven, W. J. B., and Grainger, J. (2008). An electrophysiological investigation of cross-language effects of orthographic neighborhood. Brain Res. 1246, 123–135. doi: 10.1016/j.brainres.2008.09.078
Mueller Gathercole, V. C. (2010). Interactive influences in bilingual processing and development. Int. J. Biling. Educ. Bilingual. 13, 481–485. doi: 10.1080/13670050.2010.488282
Mulder, K., van Heuven, W. J., and Dijkstra, T. (2018). Revisiting the neighborhood: how L2 proficiency and neighborhood manipulation affect bilingual processing. Front. Psychol. 9:1860. doi: 10.3389/fpsyg.2018.01860
Oganian, Y., Conrad, M., Aryani, A., Heekeren, H. R., and Spalek, K. (2016). Interplay of bigram frequency and orthographic neighbourhood statistics in language membership decision. Bilingualism 19, 578–596. doi: 10.1017/S1366728915000292
Oganian, Y., Conrad, M., Aryani, A., Spalek, K., and Heekeren, H. R. (2015). Activation patterns throughout the word processing network of L1-dominant bilinguals reflect language similarity and language decisions. J. Cognit. Neurosci. 27, 2197–2214. doi: 10.1162/jocn_a_00853
Palan, S., and Schitter, C. (2018). Prolific. A subject pool for online experiments. J. Behav. Exp. Finance 17, 22–27. doi: 10.1016/j.jbef.2017.12.004
R Core Team (2021). “R: A language and environment for statistical computing,” in R Foundation for Statistical Computing (Vienna: R Core Team). Available at: https://www.R-project.org/ (acceessed November 17, 2024).
Rastle, K., Harrington, J., and Coltheart, M. (2002). 358,534 nonwords: the ARC nonword database. Quart. J. Exp. Psychol. 55, 1339–1362. doi: 10.1080/02724980244000099
Sears, C., Hino, Y., and Lupker, S. (1995). Neighborhood size and neighborhood frequency effects in word recognition. J. Exp. Psychol. 21, 876–900. doi: 10.1037//0096-1523.21.4.876
Siakaluk, P. D., Sears, C. R., and Lupker, S. J. (2002). Orthographic neighborhood effects in lexical decision: The effects of nonword orthographic neighborhood size. J. Exp. Psychol. 28, 661–681. doi: 10.1037//0096-1523.28.3.661
Smith, J. (2021). “Inquisit [Computer software],” in Millisecond Software. Available at: https://www.millisecond.com/products/inquisit6/ (acceessed November 17, 2024).
Van Hell, J. G., and Tanner, D. (2012). Second language proficiency and cross-language lexical activation. Lang. Learn. 62, 148–171. doi: 10.1111/j.1467-9922.2012.00710.x
Van Heuven, W. J., Dijkstra, T., and Grainger, J. (1998). Orthographic neighborhood effects in bilingual word recognition. J. Memory Lang. 39, 458–483. doi: 10.1006/jmla.1998.2584
Van Kesteren, R., Dijkstra, T., and De Smedt, K. (2012). Markedness effects in Norwegian-English bilinguals: Task-dependent use of language-specific letters and bigrams. Quart. J. Exp. Psychol. 65, 2129–2154. doi: 10.1080/17470218.2012.679946
Keywords: visual word recognition in bilinguals, visual lexical decision, cross-language influences, orthographic neighborhood density, bilingual processing
Citation: Biedermann B, Beyersmann E, Blosfelds M, Macapagal C, Rosevear A and Marinovic W (2024) Cross-language orthographic neighborhood density effects in Dutch–English and Spanish–English bilinguals. Front. Lang. Sci. 3:1482861. doi: 10.3389/flang.2024.1482861
Received: 18 August 2024; Accepted: 18 November 2024;
Published: 11 December 2024.
Edited by:
Montserrat Comesaña, University of Minho, PortugalReviewed by:
Paz Suárez-Coalla, University of Oviedo, SpainCopyright © 2024 Biedermann, Beyersmann, Blosfelds, Macapagal, Rosevear and Marinovic. This is an open-access article distributed under the terms of the Creative Commons Attribution License (CC BY). The use, distribution or reproduction in other forums is permitted, provided the original author(s) and the copyright owner(s) are credited and that the original publication in this journal is cited, in accordance with accepted academic practice. No use, distribution or reproduction is permitted which does not comply with these terms.
*Correspondence: Britta Biedermann, Yi5iaWVkZXJtYW5uQGN1cnRpbi5lZHUuYXU=
Disclaimer: All claims expressed in this article are solely those of the authors and do not necessarily represent those of their affiliated organizations, or those of the publisher, the editors and the reviewers. Any product that may be evaluated in this article or claim that may be made by its manufacturer is not guaranteed or endorsed by the publisher.
Research integrity at Frontiers
Learn more about the work of our research integrity team to safeguard the quality of each article we publish.