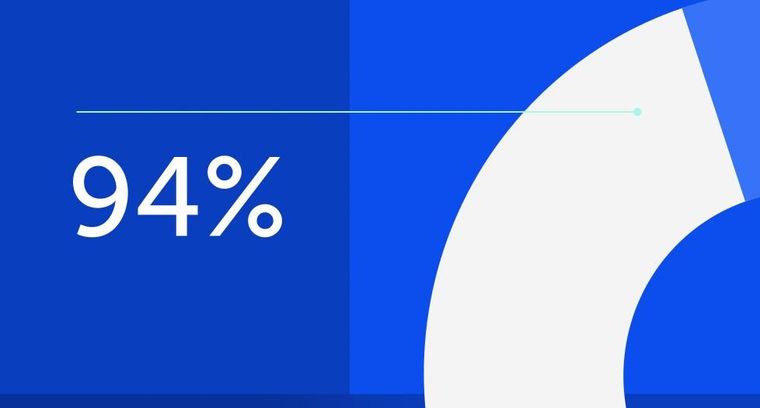
94% of researchers rate our articles as excellent or good
Learn more about the work of our research integrity team to safeguard the quality of each article we publish.
Find out more
ORIGINAL RESEARCH article
Front. Lang. Sci., 23 April 2024
Sec. Bilingualism
Volume 3 - 2024 | https://doi.org/10.3389/flang.2024.1327600
This article is part of the Research TopicFormal Approaches to Multilingual PhonologyView all 10 articles
Introduction: This paper presents a constraint-based grammar of Mandarin low vowel + nasal coda (loVN) sequences first as acquired by L1 learners, and then as transferred to L2 English.
Methods: We simulate phonological learning in Harmonic Grammar using a gradual, error-driven GLA learner, drawing on evidence from L1 Mandarin speakers' perceptual data to support our initial state assumptions. We then compare our simulation results with L2 English production (both anecdotal and ultrasound data), as well as evidence from Mandarin loanword phonology.
Results: Our results align with multiple patterns in the previous empirical literature, including an asymmetry among surface repairs for VN sequences, and we show how these emerge from our assumptions about both the L1 Mandarin grammar and the grammar's evaluation method (i.e., weighted constraints).
Discussion: We discuss the extent to which these results derive from our somewhat novel analysis of place contrasts in L1 Mandarin, and the variability in loVN outputs that we encode directly into the L1 grammar, which are then transferred to the L2 context. Ultimately we discuss how this type of modeling can make falsifiable predictions about phonological development, in both L1 and L2 contexts.
Although several decades of research has used computational simulations to investigate L1 phonological learning, comparing simulated data to observed stages of child speech (e.g., Levelt et al., 1999; Curtin and Zuraw, 2002; Hayes, 2004; Jarosz, 2010; Becker and Tessier, 2011; interalia), comparable work in the L2 acquisition literature has been rather more sparse. Often, such studies in the second language domain have focused on more phonetic questions of category learning, cue weighting and the like (starting especially with Escudero and Boersma, 2004), also including grammatical accounts of perceptual L2 learning (van Leussen and Escudero, 2015, and references therein). In the first decade after the advent of Optimality Theory, some crucial insights were investigated as to how gradual constraint re-ranking could capture stages of L2 learning and e.g., the emergence of the unmarked (e.g., Broselow et al., 1998). However, as phonological research has embraced various other brands of constraint-based grammars, such as weighted constraints in Harmonic Grammar (HG), Maximum Entropy grammars, and the like, there have been relatively few L2 acquisition analyses using these tools. One particularly interesting avenue for research, starting even with the Stochastic OT of Boersma (1998) and Boersma and Hayes (2001), is how an L1 grammar with multiple possible surface optima (i.e., grammatical variation) might be used to learn an L2 grammar built of the same constraints, and what consequences that inherent variation might bring about. Adding constraint weightings to the analytical mix also introduces the possibility that lower-weighted constraints might in the course of learning “gang up” in groups on higher-weighted constraints, providing different acquisition stages than would be predicted by a ranked constraint grammar.
This paper is an attempt to look at all of these questions, beginning with a modest but fairly detailed account of one phonotactic pattern: sequences of low vs. followed by nasal codas in Northern (Beijing-area) Mandarin, as compared with North American English. Starting with an analysis of the L1 Mandarin phonotactics and its inherent variability, we implement some simple computational learning simulations of the pattern, using a Harmonic Grammar of weighted constraints and a classic GLA learner. We then use the same simulated learner to implement a Full Transfer approach to L2 acquisition (see also Schwartz and Sprouse, 1996; as in van Leussen and Escudero, 2015). We can then compare how the L1 Mandarin transferred grammar treats the range of loVN sequences in L2 English with existing empirical data, especially from an L2 ultrasound production study (Liu, 2016) but also drawing on perceptual data and loanword phonology.
The paper is structured as follows. Section 2 compares the phonotactic restrictions of loV-N sequences in Mandarin vs. English and introduces a set of constraints to capture the inventories of both languages. Section 3 first presents how the learner can acquire an L1 Mandarin grammar of loV-N: §3.1 establishes some assumptions about ranking biases and the learner's input data over time, and §3.2 demonstrates via learning simulations in an HG-GLA model how the learner can reach the target L1 grammar successfully. We then turn to the L2 acquisition of English loV-N in Section 4, beginning with a clarification of the scope of our L2 study (§4.1). We present two key error patterns in the early stages of L2 simulations (§4.2), and show that these patterns are indeed found in previously reported literature and anecdotal reports (§4.3). After further discussion of the nature of L2 learning in our simulations (§4.4–4.5), Section 5 compares our analysis of L1 Mandarin with a standard alternative in the literature (§5.1), and discusses some of the crucial theoretical aspects of our approach (§5.2). We end with a short general discussion of L2 phonological learning and modeling.
Both English and Beijing Mandarin have three nasal consonants /m, n, ŋ/ and two low vowels which contrast for [+/–back] in their surface inventories. Standard North American English uses [æ, ɑ]1 while Mandarin uses [a, ɑ] (Duanmu, 2007). However, Beijing Mandarin (at least) has two crucial phonological restrictions on lowV-nasalC that do not apply in English: (1) only [n] and [ŋ] are allowed in coda position, but not [m] (Duanmu, 2007); (2) low vowels must agree for [+/–back] with a following coronal or dorsal nasal coda (Duanmu, 2007; Luo et al., 2020). Thus, English has 6 possible loV-N combinations while Mandarin has only two, as shown in Table 1.
In addition, the surface realizations of the legal Mandarin loV-N sequences [an] and [ɑŋ] may in fact be quite variable. Many studies demonstrate that coda nasals can be lenited (produced with no full oral closure) or deleted entirely, along with nasalization and possible compensatory lengthening of the preceding vowel (see Wang, 1993; Chen, 2000; Fang, 2004; Duanmu, 2007 inter alia; Luo et al., 2020). For example, Chen (2000) conducted acoustic measurements on the production of Mandarin coda nasals /n, ŋ/ preceded by high, mid, and low vowels (e.g., shan-ao [ʂan55.ɑʊ51]”‘hollow of the hill”). It was found that more than half of the word tokens containing the loV-N rhyme were produced without an oral closure for the nasal coda at normal speech rates, but that this oral closure deletion was less frequently observed when the nasal followed a high or mid vowel (Chen, 2000, p. 53, 54).
Thus, there are arguably three possible surface outputs for each input loV-N:
(a) faithful: the vowel is followed by nasal produced with full closure;
(b) lenited coda: the vowel is nasalized and the nasal coda is perhaps “weakened” but not fully deleted (here transcribed phonologically as [ãn] and [ŋ];
(c) deleted coda: the vowel is nasalized (and possibly lengthened) and the nasal is deleted (here transcribed as [ã] and [])2.
The three variants of the output form are not contrastive; all that remains contrastive is the distinction between [-bk] and [+bk] sequences.
Table 2 compares these three surface variants in Mandarin with the corresponding range of surface possibilities in English. Unlike in Mandarin, a nasal coda in an English loV-N sequence is crucial to meaning, since its place is not determinable from the vowel – thus, there are four rows in the English section of Table 2 compared to two rows in the Mandarin section. With respect to the vowel, we will assume that the phonological target output for these sequences in English is a simple, oral vowel – that is, that the anticipatory vowel nasalization that appears before a nasal consonant is sufficiently partial, automatic and non-contrastive that it is not part of the phonological representation (e.g., Cohn, 1993; see also Beddor et al., 2013).
Here we lay out a constraint set that can minimally capture both the Mandarin and English inventories of lowV-nasal sequences, with reference to cross-linguistic typologies. All of these constraints are fairly standard from the previous literature, so they will be introduced fairly briefly. Note that all the tableaux that illustrate constraint definitions in this section are included in Appendix A.
The markedness constraints that we will use are in (1) and (2) below. First, there are the two constraints in (1) which are violated by those structures that are banned outright in Mandarin compared to English. Tableau A1 provides a few example outputs to illustrate how RHYME HARMONY and NOCODA [m] work.
(1) RHYME Assign a violation mark to every
HARMONY sequence of two segments, a low
vowel + nasal consonant, where one is
[+back] and the other [–back]
NOCODA[m] Assign a violation mark to every labial
nasal consonant associated with the
Coda position of a syllable
Then, there are the constraints violated by some subsets of the surface variants for loV-N in Mandarin – some of which are also ultimately relevant to the English inventory. Tableau A2 presents how the constraints in (2) work in getting different surface outputs in Mandarin.
(2) *VN Assign a violation mark for every oral
vowel followed by a nasal
consonant
*NASALV Assign a violation mark for every
nasalized vowel
NOCODA Assign a violation mark to every
segment associated with the Coda
position of a syllable
The key types of unfaithfulness that our grammars will need to consider are changes in place, changes in nasalization, and segmental deletion. Thus, we begin with the four Faithfulness constraints in (3), using the framework of McCarthy and Prince (1995). Note that in this system, changes in [+/–back] violate one of two Ident constraints, whereas [+nasal] is protected by a Max constraint. Tableau A3 provides some examples showing how these faithfulness constraints work.
(3) IDENT Assign a violation mark for every pair
[+/–BACK] of input and output vowels in
-V correspondence which disagree in
specification for [+/–back]
IDENT Assign a violation mark for every pair
[+/–BACK] of input and output nasal
-N consonants in correspondence which
disagree in specification for
[+/–back]
MAX Assign a violation mark for every input
segment without an output
correspondent
MAX[NASAL] Assign a violation mark for every input
[nasal] feature without an
output correspondent
As can be seen by comparing the third to fifth candidates in Tableau A3, we are interpreting the primitive [nasal] feature on both consonants and vowels – even adjacent ones – to be separate features, each protected by faithfulness. Thus, we assume that deleting a nasal consonant incurs a violation of MAX[NASAL] even if the preceding vowel is already nasalized. In Tableau A4, we see that an underlying nasal consonant which is deleted but triggers nasalization on the preceding vowel does not violate MAX[NASAL]; the underlying and surface forms both have one instantiation of [nasal], in correspondence with each other directly (just not associated with segments that are in correspondence)3.
In addition, we include two DEP constraints into our constraint set: one that penalizes segmental insertion, and one that specifically penalizes inserting a [nasal] feature onto a vowel, as in (4). Epenthesis of either nasalization onto a vowel or a full nasal consonant after a nasal vowel can both be compelled by one or more of the markedness co-occurrence constraints above in Tableau A1. Tableau A5 demonstrates how the two DEP constraints work.
(4) DEP Assign a violation mark for every
output segment without an input
correspondent
DEP[NASAL] Assign a violation mark for every
output [nasal] feature associated with a
[+vocalic] segment without an input
segment associated with
an input [nasal] feature
Our learner's initial state consists of two weighting biases. The first is to weight all markedness constraints above faithfulness constraints, as is well established in decades of literature. This general bias captures both the fact that children's production grammars begin with highly unmarked outputs on the whole, and that any alternative starting point is more prone to subset/superset traps, in the sense of Angluin (1980) and Berwick (1985). In OT, discussion of this general bias begins with Smolensky (1996), see also extensive discussion in e.g., Gnanadesikan (2004), Hayes (2004), Tessier (2016).
The second bias deals with the relative ranking of faithfulness constraints, and adopts Steriade (2001)'s proposal of a P-Map, whereby faithfulness constraints that militate against more perceptually salient changes are higher ranked (or weighted) than those which ban less salient changes. In particular, we include a bias for weighting Ident-Vplace above Ident-Nplace, reflecting the result that changes to vowel place of articulation are relatively more salient. The most immediately relevant such results come from Zhang (2023), in which native speakers of Mandarin gave similarity ratings of loV-N pairs, and they perceived [æn]/[æŋ] as more similar than [æn]/[ɑn] or [æŋ]/[ɑŋ]. We discuss this study further in Section 4.5.
We combine these two biases into the initial state of a Harmonic Grammar below. The first – M>>F – is simply a starting point, which evidence can easily overturn. The second, however, is taken to be as universal a fixed weighting as possible, and therefore will be implemented in our simulations so as to persist as much as possible, regardless of errors and learning data.
In Section 2.1, Table 2 provided six possible Mandarin outputs. In the earliest stage of phonotactic learning, without any firm knowledge of meanings associated with these surface forms, the learner's assumption is that all six such outputs are faithfully mapped. This “Identity Map” or purely phonotactic learning grammar, will be our first simulated learning task. Later, the L1 Mandarin learner must determine – via semantic word learning and associated reasoning4 – that not all of these strings are uniquely mapped, and that in fact the grammar must instead produce surface allophonic variation from input loV-N sequences. In our Mandarin learning simulations, we model this two-stage process by first letting the learner reach a stable grammar that produces the mappings in Table 3A, then feeding it the input-output mappings in Table 3B and learning again.
Our phonotactic grammar is formalized as a weighted Harmonic Grammar, in which the candidate with the highest harmony value is the optimum (Legendre et al., 1990; Smolensky and Géraldine, 2006; Potts et al., 2010). A violation score is assigned to each constraint, which is a negative number corresponding to the number of violations of that constraint. The harmony value of a candidate is calculated as multiplying each constraint's violation score by its weight, and then summing up. In addition, a small amount of noise drawn from a Gaussian distribution (SD = 2.0) is added to the constraint weights on each iteration of Eval.
The learner we adopt is error-driven and gradual, using the HG version of the Gradual Learning Algorithm (Boersma and Hayes, 2001). On each trial, the HG-GLA learner feeds an input to the current grammar and maps it to its currently-optimal output candidate. If that optimal candidate is the target grammar's intended winner for that input, no learning occurs. If that optimum is not identical to the intended winner, however, then that loser form is used to create an error. Recalling that at first, our learner assumes that the observed surface winners are identical to their input forms, some sample errors in the L1 Mandarin purely phonotactic learning stage are below shown in Table 4.
When an error is made (i.e., there exists a mismatch between the winner target output form and the loser output form chosen by the current grammar), the learner increases the weight of the constraints that prefer the winner, and decreases the weight of constraints that prefer the loser. For instance, given the error that occurs in Table 4(i), the learner will make an update by decreasing the weight of *VN and increasing the weights of MAX and MAX[NASAL]. The HG-GLA learner adjusts the weights on each learning trial until the target form matches with the optimum produced by the current grammar of the learner. Learning occurs only in this type of mismatch scenario based on positive evidence – that is to say, it only learns when it has been prompted by an observed target form (e.g., Hayes, 2004; Prince and Tesar, 2004). In the constraint-based literature on phonological learning, this approach is in contrast to learners which use Bayesian-style reasoning to consider unobserved surface forms and decrease their predicted likelihood in the grammar (as in e.g., Jarosz, 2006; Hayes and Wilson, 2008 and many others).
The learning simulations were implemented in Praat (Boersma and Weenink, 2016). In order to impose the bias Markedness >> Faithfulness in a Harmonic Grammar, we initialized the weight of all the markedness constraints at 100 with the plasticity of 1, including *VN, *NASALV, NOCODA, NOCODA[M], and RHYMEHARMONY. All faithfulness constraints but one (see next paragraph), were initialized with a weight of 1 and plasticity of 1, including IDENT[BK]-N, MAX, MAX[NASAL], DEP and DEP[NASAL].
We implemented an initial bias between IDENT[BK]-V and IDENT[BK]-N such that IDENT[BK]-V had a weight 10 higher than IDENT[BK]-N (IDENT[BK]-V = 11). In order to retain the relative weighting between the two Ident constraints, we set the plasticity of IDENT[BK]-N at 0.1, a much smaller value than that of IDENT[BK]-V and all other constraints (= 1). The difference in the plasticity between the two IDENT constraints allows IDENT[BK]-N move at a slower rate compared to IDENT[BK]-V, so that the bias IDENT[BK]-V >> IDENT[BK]-N can be persistently imposed throughout learning (see esp. Jesney and Tessier, 2011).
We used the update rule symmetric all, which is defined such that the weight of all constraints that are violated more in the target output than in the learner's output is lowered, and the weight of all constraints that are violated more in the learner's output than in the target output is raised (Boersma and Hayes, 2001). The learning proceeded at a constant plasticity at 1 (number of plasticities = 1, initial plasticity = 1), with an evaluation noise set at 25. The learning strategy was set to LinearOT (Keller, 2006), so that no constraint weights could drop below zero on evaluation, and any negative one-time disharmonies were treated as zero.
Recall from Section 3.1.2 that at Stage 1, the learning data is fully-faithful – that is, all three surface variants of the loV-N outputs [an, ãn, ã] or [ɑŋ, ŋ, ] are assumed to come from identical corresponding inputs. On each learning trial, the learner is fed one such mapping from the six possible pairs (e.g., /ãn/ → [ãn]). After 10000 such learning trials, the end state grammar typically look like the example in (5) below, which shows one set of precise constraint weights from a simulation. This grammar produces all six of the required fully-faithful mappings:
(5) RH, NOCODA[M] >> MAX[NASAL] >> *VN >>
100, 100 82.6 72
*NASALV >> MAX, NOCODA >>
62 52, 48
IDENT[BK]-V >> DEP, DEP[NASAL], IDENT[BK]-N
11 2, 1, 1
Compared to the initial stage, the weights of RHYMEHARMONY (=100) and NOCODA[M] (=100) in (5) have not changed; no L1 Mandarin surface form violates either constraint, so there is no pressure for demotion or promotion. The two IDENT[BK] constraints IDENT[BK]-V (=11) and IDENT[BK]-N (=1) have also not changed, since there is no markedness pressure that could be better satisfied in any of the input learning data by changing [+/–back]. Thus, we remove these four constraints temporarily from our analysis, and explain in the remainder of this section how the remaining constraint weights are updated in this first stage of learning. Together, these constraints determine the optimal candidate among the four possible outputs – [an], [ã], [ãn], and [a] – given one of the three inputs (Here we illustrate for the [–back] pairs, but all of the below also applies to the [+back] pairs). Note that the tableaux that illustrate these crucial weightings are all included in Appendix B.
Tableau B1 shows how the /ã/ input is mapped faithfully; the winning candidate violates only *NASALV. In (6), we provide the two weighting conditions that explain how the winning candidate's violation of *NASALV can be optimal – the third candidate in Tableau B1 is harmonically bounded (see shading on candidate iii), so any weighting conditions will rule it out.
(6) For /ã/ to map faithfully to [ã]:
- w(*VN + NOCODA) > w(*NASALV) ruling out [an]6
- w(MAX[NASAL]) > w(*NASALV) ruling out [a]
The second of these weighting conditions is in bold because it represents a simple trading relation between two constraints (akin to a ranking argument in classic OT).
The next input /an/'s fully-faithful output violates two markedness constraints: *VN, and NOCODA, shown in Tableau B2. For the faithful candidate to win, the weights of these two constraints must sum to less than the weighted violations of the other options, and these conditions are listed in (7).
(7) For /an/ to map
faithfully to [an]:
- w(*NASALV+ > w(*VN + ruling out [ã]
MAX) NOCODA)
- w(*NASALV) > w(* VN) ruling out [ãn]7
- w(MAX[NASAL] > w(*VN + ruling out [a]
+ MAX) NOCODA)
Again, the bolded weighting condition is a straight competition between two constraints.
Finally, we consider the fully-faithful mapping of /ãn/, which violates both *NASALV and NOCODA [see Tableau B3 and (8) below].
(8) For /ãn/ to map
faithfully to [ãn]:
- w(MAX[NASAL] > w(NOCODA) ruling out [ã]
+ MAX)
- w(MAX[NASAL] > w(*NASALV) ruling out [an]
+ *VN)
- w(2* > w(*NASALV ruling out [a]
MAX[NASAL] + + NOCODA)
MAX)
Note that one of these weighting conditions (shown in italics) is a specific case of a more general weighting condition already established [see (6)'s second line].
To summarize this section, this fully-faithful (or purely phonotactic) grammar is one in which deleting nasalization is not allowed, regardless of whether it is in a marked context or not, and in which vowel nasalization is also not added just to avoid oralV-nasalC sequences. These two statements summarize the two simple weightings above: w(MAX[NASAL]) > w(*NASALV) > w(*VN). Somewhat lower weighted but still relevant are MAX and NOCODA, in that order, so that segmental deletion to avoid codas is not possible – and also, as the more complex weightings above show, that deletion is in fact never optimal, due to the constellation of higher-weighted M and F constraints. At the bottom of this grammar are DEP and DEP[NASAL], which will play more of a role later.
It is important to notice that this grammar, while fully-faithful to all the inputs it has seen, does not accomplish this pattern by simply ranking all F >> M. The majority of the faith constraints are weighted at the bottom – only MAX[NASAL] or MAX have risen significantly above their initial state values. Because the learner is biased to start with M constraints weighted high, any errors which can be attributed to the competition between markedness pressures will result in the reordering of those constraints as well as the promotion of F. Thus, this learner's initial biases plus this particular set of somewhat antagonistic markedness constraints results in a grammar which maps these inputs faithfully.
The second stage of Mandarin learning in our simulation occurs once the learner has discovered that the six surface outputs learned in stage 1 correspond to only two underlying contrasts. As discussed in Section 3.1.2, this learner now knows that output variants [an, ãn, ã] are all derived from a single [–back] input, and [ɑŋ, ŋ, ] are all surface realizations of a [+back] input. In the learning data given in 3.1.2, the underlying forms are /an/ and /ɑŋ/.
The Stage 2 learner begins with the end-state grammar from stage 1 from 3.2.3 above, and is now given its new, frequently unfaithful mappings as learning data, with each of the three variants given equal probability (0.33) as the correct output. Here too our learner is successful – after 10,000 trials, the learner is able to generate the three output variants with the frequency shown in the input-output pair distribution plot (Figure 1). One sample of such a grammar is given in Table 5; which illustrates how the weightings change between the two stages.
From the comparison above, it is fairly clear that the change at Stage 2 has come from evening out the weightings of pairs of markedness constraints – *VN and *NASALV are now very similar in weight, as are MAX and NOCODA – and most other weights have remained the same. This is shown in the two comparison tableaux B4 and B5. To choose the unfaithful candidate in Tableau B4 we need:
w(*VN + NOCODA) > w(*NASALV + MAX)
Comparing the Stage 2 grammar in Table 5 with this weighting condition, we see that in each bracket there is one constraint weighted around 67-68, and another around 50. Similarly, to choose the unfaithful candidate in Tableau B5 we need:
w(*VN) > w(*NASALV + DEP[NASAL])
Since DEP[NASAL] is still very low-weighted, this again makes the choice very variable.
Changing the number of learning trials (10000 ± 1000) results in slightly different proportions of the output variants (e.g., [an] or [ãn] as the most frequent output form rather than [ã] as shown in Figure 1) – but the overall results is roughly equivalent variation, which we will take to be a successful Mandarin end-state grammar.
To re-iterate and also foreshadow, this end state creates variability through similar weightings of a set of antagonistic markedness constraints, such as *VN and *NASALV, which make opposite demands when a vowel is followed by a nasal. In the next section, we will see how this variability impacts L2 acquisition, when additional loVN sequences are introduced.
At the outset, we wish to clarify the general purpose of our modeling L2 development and its context. Our goal is not to make specific predictions about individual grammars of L2 English learners, such as absolute rates of acquisition, ultimate attainment, and the like. As a reviewer rightly points out, if the HG-GLA learner we adopt is given the right constraint set and an informative set of input/output mappings to learn from, it will eventually learn a correct end state grammar, mimicking “perfect” L2 acquisition. The speed with which this is achieved will be a consequence of the plasticity parameter settings, as well as the relative frequency of different mappings the learner is fed, and we do not have any insights as to these aspects of development.
Instead our goal is to spell out the consequences of our assumptions about the L1 grammar and learning biases, as they make predictions about L2 learning. In particular, we will demonstrate that our proposals from Section 3 of how to capture inherent variation in L1 Mandarin loV-N sequences make two clear predictions when that grammar is applied to an L2 like English, and then discuss the extent to which these predictions align with observed data. For the sake of completeness, a later Section 4.4 demonstrates that this simulated learner can indeed reach a target-like English end-state – but we do not intend to imply that any or all L2 learners of English from L1 Mandarin backgrounds necessarily acquire grammars that are identical to L1 English ones (see more in Section 4.4 below).
As mentioned in the introduction, we adopt a Full Copying model of L2 acquisition (also following Schwartz and Sprouse, 1996; in this context, see especially Escudero, 2005; van Leussen and Escudero, 2015). This means that the initial state of our L2 grammar is precisely the end state of the L1 grammar. We note, however, that we focus only on the acquisition of a production grammar; while van Leussen and Escudero (2015)'s model (the L2LP model revised) concerns the full copying of an L1 perception grammar to apply initially in perceiving L2 surface forms (see Escudero, 2005's Optimal Perception Hypothesis; see also Boersma, 2011 and other work on bidirectional learning of perception and production). In this work, we assume that the learner has progressed to the point of relatively accurate input perception at the point where we begin our L2 production learning scenarios. We return to our learner's perception/production assumptions in Section 4.5.
The target English grammar – the idealized goal end state of L2 learning – maps all of the six output loV-N sequences as faithful to their input forms (Recall from Section 2 that we assume that no vowel nasalization at this phonological level of English – that is, whatever degree of anticipatory nasalization is produced on these vowels, it is consistent and non-contrastive, so it does not form part of these input/output mappings). So with this fully-faithful English grammar as its target, what does a Full Copying L1 Mandarin learner look like? There are two crucial properties of this L2 learner revealed by our simulations that we will focus on.
Table 6a shows the winning output candidates produced by the L1 end-state grammar described in the previous section (i.e., Table 5b), now given English inputs. From now on, we restrict ourselves to the [–back] vowels and their possible loV-N outputs – since nothing in our grammar distinguishes between front and back vowels, beyond whether or not they harmonize. Thus, the input form in bold /æn/ represents those which are also found in the L1 Mandarin input lexicon (i.e., it will also describe the treatment of /ɑŋ/ ceteris paribus), and in the initial L2 English grammar these existing input forms are mapped to the same three output options in the L1 system. The other four inputs in Table 6 are novel, and they all raise potential violations of the two undominated markedness constraints in the L1 Mandarin grammar: the first input violates NOCODA[m], /æm/, and the bottom row input contains place mismatch that violates RHYMEHARMONY, /æŋ/.
Table 6. Output proportions generated from L2 learning at the initial state (L1 end-state grammar), and after 5 and 15 trials.
The first result of the simulation that we highlight is that all of the output candidates for the two types of novel English inputs are in some way unfaithful to the input nasal – that is, either it is deleted, or it is unfaithful to nasal place, while vowel place is kept faithful. Two such options are illustrated in the tableaux of Tables 7, 8. The reason that this initial state grammar satisfies Rhyme Harmony by repairing nasal place, rather than vowel place, is our built-in bias for IDENT[BK]-V to be weighted above, and with greater plasticity than, IDENT[BK]-N. In other words, this learner prefers to be unfaithful to nasal place rather than vowel place without evidence, despite their L1 experience having provided no overt alternations toward this choice of repair.
The second simulation result that we want to examine is a skew in the relative frequency of nasal deletion vs. the other two output options, when comparing the L1 legal input vs. the novel ones. To see this in Table 6, we have bolded the proportion of deletion candidates for each input. Here just focusing on the initial L2 grammar, in the first (6a) column, we can see that the /æn/ input that does not violate RHYMEHARMONY surfaces with deletion 44% of the time, whereas the other two inputs generate deletion candidates 53 and 56% of the time.
In the tableaus of Table 9, we see how this asymmetry between deletion and non-deletion candidates comes about. The Tableau in 9 (a) compares the winners [] and [æn] given an input which does not violate RHYME HARMONY, /æn/. Here the choice between these two possible outputs comes down to a fight bettesween similarly-weighted constraints, such as MAX and NOCODA. In comparison, Tableau 9 (b) compares the same two possible output types, but given an input like /æŋ/ that violates undominated RHYMEHARMONY. Since the fully-faithful option is ruled out (iii), the resulting competition between (i) and (ii) involves the same closely-weighted constraint set, but also the crucial violation of IDENT[BK]-N.
The result will be that whatever the distribution of probabilities for the two potential outputs (i) and (ii) in (9a), the probability of deletion will be slightly higher in (9b). Specifically, deletion in (9bii) will be relatively more harmonic than (9aii), by virtue of the additional violation of IDENT[BK]-N accrued by (9bi). Given the precise weightings of our L1 end-state grammar – where IDENT[BK]-N is weighted just at 1 – this adds a few percentage points in favor of the deletion candidate in Tableau 9 (bi), producing the skew in Table 6a's italicized proportions. We emphasize that this result at the beginning of L2 production comes in large part from the L1 already being variable in its outputs for loVN sequences, so that these multiple output options transfer even to the L2 novel inputs which are L1-illegal. Since L2 learning is initialized as varying between possible output candidates, we can observe this skew toward deletion over nasal place substitution for mismatched VN.
Using the learning parameters described in Section 3 above, the L1 Mandarin initial state grammar can quickly be re-arranged to replicate some aspects of the L2 English system. Table 10 illustrates some typical constraint weightings during these learning stages: moving on from the initial state (a) to its next stages after a few learning trials (given our previous parameters, after five learning cycles in Table 10b and 15 learning cycles in Table 10c). Those constraints which are been overall promoted or demoted are indicated with an up or down arrow. While only small changes in constraint values have occurred after so few learning trials, the overall effect on output probability distributions is significant, as the later columns of Table 6 demonstrates.
The first overall change in distributions shown in Table 6 (b, c) is a sharp proportional increase in output candidates with the goal output shape, namely oral vowel followed by nasal consonant. After only 15 trials, this output shape accounts for more than 90% of each of the three output types above. It is not surprising that the first aspect of the L1 grammar to be overturned in L2 learning is precisely the delicate balance between three potential output winners – a very small nudge to those constraints (e.g., MAX up and NOCODA down) is enough to focus the grammar on one of these three variants.
With respect to second feature of our simulation, the skew in deletion rates depending on input nasal place, we continue to see the preference for deletion when the input contains a [+/–back] mismatch at all three stages – the input /æn/ has a lower percentage of [] outputs than the other two inputs, and this is also illustrated graphically in Figure 1.
To what extent do these two simulation properties align with the L2 acquisition of English by Mandarin speakers? In support of the first simulation result, it is certainly reported anecdotally that nasal place and not vowel place is the feature that surfaces unfaithfully in L2 English errors by L1 Mandarin speakers. For example, the first author (a lifelong speaker of Beijing Mandarin) reports that English words such as bang and gang are frequently produced as [bæn] and [gæn] by L1 Mandarin speakers (or as [b] and [g]), and similarly that gone surfaces as [gɑŋ] (or as [g]) – all with nasal coda place replaced to match vowel backness.
Another piece of data comes from the systematic adaptation patterns of English loanwords with mismatches in vowel and nasal place into Mandarin (Hsieh et al., 2009 and references therein). Consistent with the above anecdotal reports, loanword adaptations also suggest that the L1 Mandarin grammar, when faced with a [+/– back] loVN mismatch, will alter the place of the nasal rather than the vowel. Thus, Hsieh et al. (2009) report borrowings such as tango [æŋ] → tan.ge [an] and Wisconsin [ɑn] → wei.si.kang.xing [ɑŋ]. Similarly, when English words with coda [m] are borrowed into Mandarin, [m] tends to be replaced by [n] or [ŋ] to match the [+/–back] of the low vowel, e.g., Nottingham[æm] -> nuo.ding.han[an]. Of course, we acknowledge the long-standing debate as to the extent to which a language's loanword phonology is equivalent to or divergent from its L1 grammar, which we have assumed here as the initial L2 state (for example, compare Yip, 1993; Paradis and LaCharité, 1997; Peperkamp et al., 2008). In the present case, we point to recent work by Huang and Lin (2023), which presents evidence that L1 Mandarin learners of L2 English produce English nonce words and adapt English loanwords equivalently (and see also Broselow, 2023 on the shared uses of probabilistic cue weighting in loanword and native grammars.) We therefore take this evidence as at least suggestive of convergence between Mandarin loanword repairs and the L1 grammar, which here is transferred to an L2 scenario.
With respect to the second simulation result, here we turn to an articulatory result from Liu (2016)'s ultrasound study of L2 English words produced by adult L1 Beijing Mandarin speakers, comparing vowel + oral stop coda /d, g/ (e.g., bad) and vowel + nasal stop coda /n, ŋ/ (e.g., ban). Speakers were asked to read a list of monosyllabic words containing the target sequence in a carrier sentence while their lingual gesture and movement were imaged.
To analyze the production of nasal coda closure, the shortest distance between the tongue contour and the region of interest along the palate (either alveolar or velar region) was calculated for each word. This shortest distance (also referred to as the smallest aperture) was normalized across speakers, by taking into account the full range of tongue positions that an individual speaker can achieve when producing any coda (oral or nasal). Specifically, percent lingual aperture refers to the smallest aperture produced during a particular coda, compared to the smallest aperture measurement overall. The higher the percent lingual aperature, the greater the distance between the tongue contour and the corresponding palate region (alveolar or velar region), and hence more coda gestural reduction. The key result in Liu (2016)'s data is that, for loV-N sequences, the nasal coda was produced with a more reduced gesture (i.e., higher percent lingual aperture) when the low vowel and the nasal had mismatched backness compared to that with matched backness. In other words, the closure of [ŋ] was more reduced than [n] following [æ], and [n] is more reduced than [ŋ] following the back vowel [ɑ] (Liu, 2016, p. 29, Figure 4.2).
Our interpretation of Liu (2016)'s data is that these Mandarin-speakers L2 developing grammar produced relatively more deletion for the mismatched loV-N sequences than the matched ones. This is of course something of a translation, from one type of behavioral data to a grammatical abstraction across symbolic variants. One way to understand this might be that each phonological output candidate can be implemented with a range of coda closure gestures (and associated percent lingual apertures), and that implementing a “deletion” candidate more often for a particular type of input will result in an average higher percent aperture. In the terms of our simulation, their production grammar mapped the input /æŋ/ relatively more often to [] the deletion candidate than they did with /æn/, and similarly /ɑŋ/ generated more [] outputs than /ɑn/. And this is indeed what our simulated learner does (recall Table 6).
Over time, the L2 learner will accrue evidence that supports faithfulness to the two types of ungrammatical Mandarin surface forms in their new lexicon: coda [m]s, and backness mismatches. In the six forms given to the learner at equal proportions, two inputs violate NOCODA[m] and two others violate RHYMEHARMONY, so it is roughly the case that these two markedness constraints are demoted at the same speed. The last two columns of Table 10 show this, comparing typical values for the L2 grammar at 15 and 1,000 trials, with a visual re-organization that reflects the main constraint re-orderings.
The final state grammar after 1,000 learning trials encodes all the relevant English constraint weightings, and thus is faithful to all six of the English inputs. To illustrate, the tableaux in Table 11 compare the same two mappings from the L2 initial state of Tables 7, 8 above – here we see that violations of NOCODA[M] (11a) and of RHYMEHARMONY (11b) are now both tolerated.
As mentioned in Section 4.1, just because the simulation can reach “perfect” L2 acquisition does not mean that any human learner can or will; we acknowledge that much more must be considered to make predictions about ultimate attainment. As a sidenote from our main arguments, we note that one approach to capturing incomplete L2 acquisition in this kind of grammatical simulation might be to impose limits on the frequency with which a learner uses errors to update their current constraint weights, and/or the number of errors that the learner is willing to process before it “fossilizes” at some particular state. For a learning approach that bears some resemblance to this view, though in an L1 context, see Tessier (2016). Another relevant simulated learning approach is found in Zhao and Li (2022), in which an unsupervised, connectionist model is given training lexicons of varying sizes, to observe something akin to progressives stages of learning and the different types and frequencies of errors that each resulting learner makes. In this work, too, the goal is to compare model-predicted errors with production data (from learner corpora), but not to determine how and when an L2 target-like end-state is achieved.
As pointed out in Section 4.1, the initial L2 learning state in the grammar that we model here is not the end state of an L1 production grammar. Our assumption is that by this point, our learner's L2 perception is fairly accurate. To support this claim, we return to the perceptual evidence from Zhang (2023). In Section 3.1.1, we reported the difference in participants' accuracy in perceiving L2 English place contrasts in nasals vs. vowels. Nevertheless, though nasal contrasts were less well discriminated, her participants still had high accuracy overall in perceiving both of these contrasts – even between those English loVN sequences which are illegal in their L1. Even for the least salient contrast, the AX discrimination task, L2 listeners had an average accuracy over 93.46% in discriminating loVN sequences that differ only in the nasal (e.g., [æn]-[æŋ]). This degree of accuracy was not significantly different from the average accuracy rate of 96.24% for L1 English listeners.
However, since Zhang (2023) did not test production data along with perception, we should ask how reasonable it is to aim our simulations at the behavior of an L2 English learner whose L2 perceptual abilities are quite advanced but whose L2 production remains highly L1-influenced? In fact, we see fairly good support for this view in the two groups of experimental participants whose data we have extracted, comparing Zhang (2023) and Liu (2016). Both of these L2 groups are L1 Beijing Mandarin-speaking educated adults, who at the time of the study were living and studying in an English-speaking country or region [Hong Kong for those speakers in Liu (2016), and Vancouver, Canada for Zhang (2023)]. Most had moved to an English-speaking environment after the age of 18, before which they were exposed to English in classroom settings, and they had all achieved sufficient English proficiency to gain acceptance to an English-speaking university (i.e., TOFEL or IELTS). Overall, we believe that the amount of L2 knowledge and experience was relatively similar for the speakers in these two studies. Thus, the learning context that we have attempted to simulate above seems relatively aligned with the L2 populations that we observe from these two studies – i.e., learners with fairly target-like perception of English loVN in place, yet still with atypical L2 production strategies.
A reviewer points out an alternative interpretation – namely, that L2 English learners are so proficient in perceiving these novel contrasts not found in their L1 Mandarin simply because they are easy enough to perceive without L1 experience. In other words: perhaps it is not that Zhang (2023)'s participants had already completed significant L2 perceptual learning about loV-N sequences, but rather that their L1 production grammar's restrictions on loV-N sequences had not dampened their abilities to perceive them (In support of this possibility, see Kabak and Idsardi, 2007 for an example of English coda-onset contrasts which are ungrammatical in Korean but do not result in perceptual distortion among L1 Korean L2 English listeners). In the present case, we do not have direct evidence about nasal place perception among monolingual Mandarin listeners (i.e., those who have never been exposed to additional English contrasts), so we are not in a position to choose definitively between these two views. We do note, however, that nasal place contrasts have been reported in both L1 adult and infant studies to be more challenging to discriminate than most (Narayan, 2008; Narayan et al., 2010), and see especially Harnsberger (2001) on the crosslinguistic perceptual difficulties presented by novel L2 nasal place contrasts.
In the following final section, we discuss the consequences of our simulations' crucial assumptions, a comparison with alternative understandings of the L1 Mandarin phonology, and the bigger picture for this type of L1/L2 learning simulation.
Previous literature on Mandarin phonology often assumes that the nasal coda in VN sequences is the bearer of the place contrast (Duanmu, 2007; Hsieh et al., 2009 interalia; Luo et al., 2020). In these analyses, the backness of the low vowel is underspecified in the underlying form or else explicitly described as non-contrastive; on the surface the vowel is driven by RHYMEHARMONY to agree with the nasal's underlying specification for [+/–back]. To show how the target output is generated from the input loV-N with underspecified [+/–back] of the vowel (indicated by the archiphoneme), an example in Table 12 is adapted from Luo et al. (2020, p. 19), using a constraint set similar to the one adopted in this paper8.
Table 12. The grammar proposed by Luo et al. (2020).
In the analysis of Luo et al. (2020), changing vowel place is the only option under consideration, and there is no motivation to alter nasal place from its UR value. A slightly different approach is adopted in Hsieh et al. (2009), though they also assume that Mandarin inputs have an unspecified low vowel that matches its backness with the nasal coda's underlying place. However, given the OT premise of Richness of the Base, Hsieh et al. (2009) also consider inputs with specified vowel place and input sequences that violate Rhyme Harmony, making the change of either the vowel place or the nasal place possible. Thus, Hsieh et al. (2009) proposes that IDENT-CPL-CODA is crucially ranked above IDENT[BK] (which targets vowels), so that here the low vowel is unfaithful to place.
Since we have adopted the opposite assumptions in this paper, we must now consider what sources of evidence there are for the underlying representation of Mandarin low vowels or coda nasals? One such piece of evidence is the romanized orthographic system Pinyin for Mandarin. The nasal codas [n] and [ŋ] are transcribed differently in Pinyin as n and ng, whereas the low vowel is uniformly transcribed as ɑ; this could well be interpreted as the latter's under-specification for place in Mandarin speakers' minds.
If we expand our view and consider the status of [+/–back] contrasts in the full Mandarin inventory of vowels and nasal codas, the picture is considerably complicated; here we rely especially on the descriptions and discussion of Xu (1980) and Duanmu (2007). With respect to high vowels, the [+/–back] contrast is maintained in open syllables, where /i/, /y/ and /u/ are all contrastive, but highV-nasal sequences are restricted to a subset which may in part obey RHYMEHARMONY (cf. [yn] and [ʊŋ], *[yŋ] and *[ʊn]). Among mid vowels there is only one contrastive vowel category, all of whose surface allophones' features including [+/–back] are dictated by surrounding segments. When followed by either nasal coda, this mid vowel surfaces invariantly as schwa (transcribed in different sources as [ə] or [ʌ]), apparently not obeying RHYMEHARMONY. Finally, the two surface low vowels are not otherwise contrastive except in the pre-nasal environment we have discussed at length in this paper.
In addition to phonotactic restrictions, several other studies have reported that both high and mid vowels undergo some degree of reduction or merger before nasal codas. With respect to high vowels, some varieties such as Shanghai Mandarin show loss of the /n~ŋ/ contrast before [i] in both perception and production, and an overall bias toward [iŋ] (Liu and Babel, 2023), and more mixed results among Beijing and Northern Mandarin speakers (cf. Chen and Guion-Anderson, 2011). With respect to mid vowels, loss of contrast between /ən/ and /əŋ/ is reported for some varieties such as Taiwanese Mandarin and Shanghai Mandarin (Chiu et al., 2019; Faytak et al., 2020), with a bias toward /ən/.
Regardless of this complicated landscape, the grammatical upshot is that Mandarin speakers do not get clear evidence from any language-internal data as to how vowel + nasal phonotactics are imposed, because they all represent static restrictions. To return to low vowels, we can observe that lowV-nasal sequences all match for [+/–back] on the surface, but there are no alternations to demonstrate how a multi-morphemic input like /a + ŋ/ or /ɑ + n/ would be mapped to the surface. It is overall clear from the above that across vowels and Mandarin varieties, the [+/–back] place contrast is hard to maintain in both vowels and their following nasal codas, and that many markedness constraints must outweigh faithfulness to [+/–back] in various different ways. What our analysis crucially requires, among all these restrictions on backness, is only that IDENT[BK]-V outweigh IDENT[BK]-N. Regardless of any other preservation or neutralization of place contrasts, in which IDENT[BK]-V might rank above or below many other markedness constraints, all we must predict is that if an input VN sequence is driven by markedness to change one or the other of their underlying places, the vowel's place features will win.
A separate source of data that this paper has treated centrally are the multiple surface variants of /VN/ sequences in Mandarin. Once the grammar is built to produce both [an] and [ã] from the same [–back] input, it becomes harder to analyze their place feature as determined by the underlying nasal. It is not impossible to derive a mapping like /ɑn/ → [ã] as optimal, but it requires an analysis of derived and indeed displaced contrast, whereby low nasalized vowels in the output are faithful to the place of a nasal consonant that has been deleted, but its place retained; or possibly a fusion of both input vowel and nasal segments, violating a series of other Ident constraints but not losing the input consonant's nasality.
Putting derived contrast aside, any L2 account that repairs backness mismatches in favor of the input nasal's place must assume a disconnect between L1 input/output mappings on the one hand (where nasal place is retained) and loanword/perception/L2 production data on the other. This is in fact one of the key arguments of Hsieh et al. (2009), which takes it as a given that the disconnect exists and that it reveals something special of the nature of loanword phonology. Instead: our approach has to been to assume that all sources of evidence point to the vowel as the faithful bearer of [+/–back] specification, at least in the current grammar of speakers like those of Beijing Mandarin, and to reason from there as to the learning consequences of their grammatical assumptions.
The discussion in the preceding sections provided motivations for some but not all of the properties of our grammar and our learning simulations. We explained our choice of a stochastic grammar (so that the L1 grammar could include multiple surface variants) and the rationale behind our initial values for classes of constraints: with Markedness high, Faithfulness low, and a specific relationship between IDENT[BK]-V and IDENT[BK]-N. One point that we have not addressed explicitly, however, is our choice of decision strategy – that is, adopting a Harmonic Grammar with weighted constraints, rather than a classic OT grammar of ranked constraints. But in fact using the HG-GLA system was a key to the simulation results of Section 4.
The relevant property of a weighted constraint grammar is that its choice of an optimum is affected by all the constraints that are violated by each candidate; there is no equivalent to the strict ranking that classic OT analyses rely on. In HG, low-weighted constraints can still exert their influence when other constraints might cancel each other out, contributing to what is frequently termed in the HG literature as a gang effect (see e.g., Pater, 2009; Jesney and Tessier, 2011; Breiss, 2020, among many others). This is exactly the case in the two mappings illustrated in the grammars of Table 9.
To see the importance of constraint weighting, the grammars in Table 13 reproduce those from Table 9, but now adopting ranked constraints. Here we still assume that constraints have numerical values and that these are perturbed at each use of the grammar, but that the classic OT EVAL component chooses the optimal candidate.
Table 13. Reproducing the grammar in Table 9 with ranked constraints.
In (13a), the choice of candidates is all up to the relative ranking of MAX and NOCODA; with these one-time values of MAX and NOCODA, candidate (ii) wins, but given their similar underlying values, NOCODA will often outrank MAX and candidate (i) will win. In (13b), the situation is in fact exactly the same – all that chooses between the two first candidates is that same ranking, and the fact that Tableau (13bii) violates a lower ranked faithfulness constraint does not make it any different in the grammar's eyes than Tableau 13 (aii). Since Liu (2016)'s L2 production data suggests that the (i) and (ii) candidates do differ between situations as in Table 9, we adopt Harmonic Grammar's weighted constraints to let the faithfulness constraint violated in (9bii) influence our system's overall behavior.
Our other consequential assumption concerns the implementation of our bias between IDENT[BK]-V and IDENT[BK]-N. Following Jesney and Tessier (2011) in particular, we assume that implementing “low” faithfulness in Harmonic Grammar means an initial value that is as low as possible without being zero; thus we start all F constraints at 1. To implement a “fixed” weighting between IDENT[BK]-V and IDENT[BK]-N, we initialized vowel faithfulness at 11; given our particular parameter settings regarding stochastic noise, this spread of 10 points means that effectively IDENT[BK]-V will never act as though weighted lower than IDENT[BK]-N. We also chose a plasticity value for IDENT[BK]-N that was 10 times slower than that for IDENT[BK]-V. Together, these two settings keep IDENT[BK]-V reliably weighted above IDENT[BK]-N in the early stages of learning, at least. Note, however, that they do not impose a fully-fixed relationship between the two constraints – in fact, the final L2 grammar we report in Table 10 (d) has only 1.5 points between them.
In our L1 simulations, this initial bias between the two Ident constraints ensures that the grammar resolves backness mismatches by changing nasal and not vowel place – without any evidence of alternations. Then in the L2 simulations, both Ident constraints must be promoted sufficiently to allow backness violations in English, but in the meantime the lower Ident N constraint's role is to produce the asymmetry just discussed in Table 9. The extent of that asymmetry, and how long it lasts in learning, is a function of the initial value of Ident N. In our default-type setting (IDENT[BK]-V at 11 and IDENT[BK]-N at 1), we saw that deletion is initially chosen for input with matching backness (Tableau 9a) about 10% less often than for those with mismatches (9b), and that this spread is becoming smaller and disappearing after the first 15 trials and beyond. By way of comparison, if everything else is kept the same but the initial values of IDENT[BK]-V vs. N are 30 and 20 respectively, the end state of learning is also successful, but the distributions of winning candidates along the way are quite different. Table 14 shows a typical distribution of winning candidates at and near the beginning of L2 learning, just focusing on the front vowel with coronal and velar nasals.
Compared to the distributions in Section 4.3, this is a more extreme asymmetry in Table 14; both in the difference between /æn/ and /æŋ/ and between 0 and 5 trials. Here, mismatched VN inputs are almost entirely subject to coda deletion, whereas inputs that match for [+/–back] have their nasals deleted at the usual L1 rate initially, and then almost immediately become entirely protected from deletion – so that after 5 trials, the treatment of both inputs is almost diametrically opposite. We suspect that this extreme difference in the input is not a likely trajectory, at least partly given the fairly gradient nature of Liu (2016)'s result. In any event, we thus observe that while constraint initial values may feel arbitrary, they do in fact encode substantial and falsifiable predictions about the course of learning.
The biggest picture goal of this paper has been to highlight how the details of an L1 grammatical analysis and its inherent variability can influence L2 acquisition, and how that influence can be studied formally using learning simulations.
One caveat we want to emphasize again is that the absolute numbers of learning trials, in these simulations, are merely a function of the parameter settings chosen, especially the plasticity of each constraint demoted and promoted in response to errors, and cannot reflect any direct connection with learning rates. The fact that a learner takes 15 trials to move from one qualitative stage of phonological development to another is not meaningful on its own; we only seek to show that a learner beginning at the initial state (zero trials) will first pass through one type of intermediate stage, onto another, and eventually make it to the end state with success.
Ultimately, we would like to use this kind of learning simulation to not only capture existing data about stages and asymmetries in second language phonological acquisition, but also make novel, testable predictions about L2 perception and production. For example, Luo et al. (2020) report at length about the interaction between (i) RHYMEHARMONY in loV-N sequences and (ii) nasal place sharing with a following stop onset, across a compound boundary (e.g., /fan.kai/ -> [faŋ.kai] fan.kai “open”). One direct extension of our analyses here is to consider how the L1 Mandarin grammar might derive this pattern, especially in light of our proposal about the source of underlying [+/–back] place contrasts. We could then use simulations to predict how learners should acquire an L2 English grammar of NC clusters, within and across morphemes (e.g., the place assimilation of i[mp]ossible, i[nt]olerant and i[ŋk]capable, vs. the more gradient assimilation across word boundaries, e.g., Turnbull et al., 2018). Adding the additional dimension of nasal coda place sharing into the inventory of Mandarin surface forms ([, æn, n], plus [æŋ.k] and possibly [.k]) will create further degrees of L1 end-state variability. The specific choice of a constraint set that can make RHYMEHARMONY variably violable – but only when coda-onset place sharing is at issue – will predict the stages through which such an L2 HG-GLA learner should develop, which could then be tested experimentally. The more sources of human behavioral and perceptual data we can compare with a simulated learner, the better we may understand how grammatical and other pressures combine to create second language speech patterns.
Publicly available datasets were analyzed in this study. This data can be found at: see published data in Liu (2016) and Zhang (2023).
Ethical approval was not required for the study involving humans in accordance with the local legislation and institutional requirements. Written informed consent to participate in this study was not required from the participants or the participants' legal guardians/next of kin in accordance with the national legislation and the institutional requirements.
SZ: Data curation, Formal analysis, Writing—original draft, Writing—review & editing. A-MT: Conceptualization, Formal analysis, Writing—original draft, Writing—review & editing.
The author(s) declare financial support was received for the research, authorship, and/or publication of this article. This study was supported by SSHRC Insight Grant #435-2023-0169, awarded to A-MT.
We would like to thank the members of the UBC Child Phonology Lab especially Kaili Vesik; Molly Babel, Suyuan Liu, and Yadong Liu for their contributions to and discussion of related work; two very helpful reviewers, Baris Kabak, and audiences at Interspeech2023.
The authors declare that the research was conducted in the absence of any commercial or financial relationships that could be construed as a potential conflict of interest.
All claims expressed in this article are solely those of the authors and do not necessarily represent those of their affiliated organizations, or those of the publisher, the editors and the reviewers. Any product that may be evaluated in this article, or claim that may be made by its manufacturer, is not guaranteed or endorsed by the publisher.
The Supplementary Material for this article can be found online at: https://www.frontiersin.org/articles/10.3389/flang.2024.1327600/full#supplementary-material
1. ^This is a quite broad transcription, and abstracts away from a lot of phonetic detail between and across dialects, especially with regard to the location of [æ], e.g., its precise height and backness, as well as its status of nasalization or diphthongization. For our purposes, it is only crucial that it be a phonologically low front vowel.
2. ^We do not discuss the possible compensatory lengthening of the vowel when nasal coda is deleted in this paper, although it is reported in Duanmu (2007).
3. ^This approach to the representations of adjacent features is of course not the only or even more the common one, but it will allow us to make clear how constraints interact in the languages being learned.
4. ^For relevant work about this reasoning, see e.g., Pater et al. (2012), O'Hara (2017), and Nelson (2019); thanks to an anonymous reviewer for pointing these out.
5. ^For an argument that this noise should be assessed after evaluation, i.e. on the probabilities of candidates themselves as in MaxEnt grammars, rather than at the time of evaluation, see Kawahara (2020) and Hayes (2022). In our simulations, this distinction is not (to our knowledge) crucial.
6. ^Technically this should be w(*VN + NOCODA + DEP) > w(*NASALV), but DEP has little to do here because of its low weight.
7. ^Again, the second term should be w(*NASALV + DEP[NASAL]), but DEP[NASAL] has little to do here.
8. ^Note that the constraint IDENT-IO(NAS) is defined as “the corresponding segments in input and output have identical values for [nasal]” (Luo et al., 2020), which is not the same as our IDENT[+/–BACK]-N. IDENT-IO(BACK) is in fact a constraint targeting identical values for [+/–back].
Angluin, D. (1980). Inductive inference of formal languages from positive data. Inf. Control 45, 117–135. doi: 10.1016/S0019-9958(80)90285-5
Becker, M., and Tessier, A. M. (2011). Trajectories of faithfulness in child-specific phonology. Phonology 28, 163–196. doi: 10.1017/S0952675711000133
Beddor, P. S., McGowan, K. B., Boland, J. E., Coetzee, A. W., and Brasher, A. (2013). The time course of perception of coarticulation. The J. Acous. Soc. Am. 133, 2350–2366. doi: 10.1121/1.4794366
Boersma, P. (1998). Functional phonology: formalizing the interactions between articulatory and perceptual drives (Doctoral dissertation). University of Amsterdam.
Boersma, P. (2011). A programme for bidirectional phonology and phonetics and their acquisition and evolution. Bidirect. Optim. Theor. 180, 33–72. doi: 10.1075/la.180.02boe
Boersma, P., and Hayes, B. (2001). Empirical tests of the gradual learning algorithm. Ling. Inq. 32, 45–86. doi: 10.1162/002438901554586
Boersma, P., and Weenink, D. (2016). Praat: Doing Phonetics by Computer. Available online at: http://www.praat.org/
Breiss, C. (2020). Constraint cumulativity in phonotactics: evidence from artificial grammar learning studies. Phonology 37, 551–576. doi: 10.1017/S0952675720000275
Broselow, E. (2023). Probabilistic Cue Weighting in Native and Loanword Perception. The Annual Meeting of Phonology.
Broselow, E., Chen, S. I., and Wang, C. (1998). The emergence of the unmarked in second language phonology. Stu. Sec. Lang. Acq. 20, 261–280. doi: 10.1017/S0272263198002071
Chen, M. Y. (2000). Acoustic analysis of simple vowels preceding a nasal in Standard Chinese. J. Phonetic. 28, 43–67. doi: 10.1006/jpho.2000.0106
Chen, Y., and Guion-Anderson, S. (2011). Perceptual confusabiltiy of word-final nasals in southern min and Mandarin: implications for coda nasal mergers in Chinese. Proc. 17th Int. Cong. Phonetic. Sci. 22, 464–467. doi: 10.1121/1.3587899
Chiu, C., Lu, Y., Weng, Y., Jin, S., Weng, W., and Yang, T. (2019). “Uncovering syllable-final nasal merging in Taiwan Mandarin: an ultrasonographic investigation of tongue postures and degrees of nasalization,” in Proceedings of the 19th International Congress of Phonetic Sciences (Melbourne, VIC; Canberra, ACT: Australasian Speech Science and Technology Association), 398–402.
Cohn, A. (1993). Nasalisation in English: phonology or phonetics. Phonology 10, 43–81. doi: 10.1017/S0952675700001731
Curtin, S., and Zuraw, K. (2002). Explaining constraint demotion in a developing system. Proc. Boston Univ. Conf. Lang. Dev. 26, 118–129.
Escudero, P. (2005). Linguistic Perception and Second Language Acquisition: Explaining the Attainment of Optimal Phonological Categorization [Ph.D. thesis]. Utercht: Utercht University.
Escudero, P., and Boersma, P. (2004). Bridging the gap between L2 speech perception research and phonological theory. Stu. Sec. Lang. Acquisi. 26, 551–585. doi: 10.1017/S0272263104040021
Fang, Q. (2004). Acoustic Analysis of Nasal Codas in Connected Speech in Chinese. Beijing: Phonetic Research Report, Institute of Linguistics, CASS, 91–97.
Faytak, M., Liu, S., and Sundara, M. (2020). Nasal coda neutralization in Shanghai Mandarin: articulatory and perceptual evidence. Lab. Phonol. 11, 1–14. doi: 10.5334/labphon.269
Gnanadesikan, A. (2004). Markedness and faithfulness constraints in child phonology. Constr. Phonol. Acq. 22, 73–108. doi: 10.1017/CBO9780511486418.004
Harnsberger, J. D. (2001). On the relationship between identification and discrimination of non-native nasal consonants. The J. Acoustic. Soc. Am. 110, 489–503. doi: 10.1121/1.1371758
Hayes, B. (2004). “Phonological acquisition in optimality theory: the early stages,” in Constraints in Phonological Acquisition, eds R. Kager, J. Pater and W. Zonneveld (Cambridge: CUP), 158–203.
Hayes, B. (2022). Deriving the wug-shaped curve: a criterion for assessing formal theories of linguistic variation. Ann. Rev. Ling. 8, 473–494. doi: 10.1146/annurev-linguistics-031220-013128
Hayes, B., and Wilson, C. (2008). A maximum entropy model of phonotactics and phonotactic learning. Ling. Inq. 39:3. doi: 10.1162/ling.2008.39.3.379
Hsieh, F. F., Kenstowicz, M., and Mou, X. (2009). Mandarin adaptations of coda nasals in English loanwords. Loan Phonol. 307:131. doi: 10.1075/cilt.307.05hsi
Huang, H. H., and Lin, Y. H. (2023). “English vowel quality effects on nasal germination in Mandarin Loanwords: corpus data vs. bilingual production and perception experimental results,” in [Conference Presentation] Annual Meeting of Phonology 2023.
Jarosz, G. (2006). Rich lexicons and Restrictive Grammars: Maximum Likelihood Learning in Optimality Theory [Doctoral dissertation]. Baltimore, MD: Johns Hopkins University.
Jarosz, G. (2010). Implicational markedness and frequency in constraint-based computational models of phonological learning. J. Child Lang. 37, 565–606. doi: 10.1017/S0305000910000103
Jesney, K., and Tessier, A. M. (2011). Biases in harmonic grammar: the road to restrictive learning. Nat. Lang. Ling. Theor. 22, 251–290. doi: 10.1007/s11049-010-9104-2
Kabak, B., and Idsardi, W. (2007). Perceptual distortions in the adaptation of english consonant clusters: Syllable structure or consonantal contact constraints? Lang. Speech 50:201. doi: 10.1177/00238309070500010201
Kawahara, S. (2020). A wug-shaped curve in sound symbolism: the case of Japanese Pokémon names. Phonology 37, 383–418. doi: 10.1017/S0952675720000202
Keller, F. (2006). Linear optimality theory as a model of gradience in grammar. Grad. Gram. Gen. Persp. 12, 270–287. doi: 10.1093/acprof:oso/9780199274796.003.0014
Legendre, G., Miyata, Y., and Smolensky, P. (1990). “Harmonic Grammar - a formal multilevel connectionist theory of linguistic wellformedness: an application,” in Proceedings of the Twelfth Annual Conference of the Cognitive Science Society. Cambridge: Lawrence Erlbaum, 884–891.
Levelt, C. C., Schiller, N. O., and Levelt, W. J. M. (1999). A developmental grammar for syllable structure in the production of child language. Brain Lang. 68, 291–299.
Liu, S., and Babel, M. (2023). “Production and perception of Mandarin /i/-nasal rhymes,” in Proceedings of the 20th International Congress of Phonetic Sciences, eds R. Skarnitzl and J. Volín (London: Guarant International), 142–146.
Liu, Y. (2016). Oral gestural reduction of English nasal coda produced by Mandarin English-as-a-foreign-language learners. The J. Acous. Soc. Am. 140, 3339. doi: 10.1121/1.4970656
Luo, M., Van de Weijer, J., and Sloos, M. (2020). “A perceptually-based OT approach to opacity in Mandarin Chinese nasal rhymes,” in Explorations of Chinese Theoretical and Applied Linguistics, eds D. Chen and D. Bell (Cambridge Scholar Publishing), 8–43.
McCarthy, J., and Prince, A. (1995). “Faithfulness and reduplicative identity,” in Papers in Optimality Theory. University of Massachusetts Occasional Papers in Linguistics 18, eds J. Beckman, L. Dickey, and S. Urbanczyk (Amherst, MA: University of Massachusetts; GLSA), 249–384.
Narayan, C. R. (2008). The acoustic-perceptual salience of nasal place contrasts. J. Phonetic. 36, 191–217. doi: 10.1016/j.wocn.2007.10.001
Narayan, C. R., Werker, J. F., and Beddor, P. S. (2010). The interaction between acoustic salience and language experience in developmental speech perception: evidence from nasal place discrimination. Dev. Sci. 12, 407–420. doi: 10.1111/j.1467-7687.2009.00898.x
Nelson, M. (2019). Segmentation and UR acquisition with UR constraints. Proc. Soc. Comput. Ling. 2, 60–68. doi: 10.7275/zc9d-pn56
O'Hara, C. (2017). How abstract is more abstract? Learning abstract underlying representations. Phonology 34, 325–345. doi: 10.1017/S0952675717000161
Paradis, C., and LaCharité, D. (1997). Preservation and minimality in loanword adaptation. J. Ling. 33, 379–430. doi: 10.1017/S0022226797006786
Pater, J. (2009). Weighted constraints in generative linguistics. Cognit. Sci. 33, 999–1035. doi: 10.1111/j.1551-6709.2009.01047.x
Pater, J., Staubs, R., Jesney, K., and Smith, B. C. (2012). “Learning probabilities over underlying representations,” in Proceedings of the Twelfth Meeting of the Special Interest Group on Computational Morphology and Phonology, 62–71
Peperkamp, S., Vendelin, I., and Nakamura, K. (2008). On the perceptual origins of loanword adaptations: experimental evidence from Japanese. Phonology 25, 129–164. doi: 10.1017/S0952675708001425
Potts, C., Pater, J., Jesney, K., Bhatt, R., and Becker, M. (2010). Harmonic Grammar with linear programming: from linear systems to linguistic typology. Phonology 27, 77–117. doi: 10.1017/S0952675710000047
Prince, A., and Tesar, B. (2004). “Learning phonotactic distributions,” in Constraints in Phonological Acquisition, eds R. Kager, J. Pater and W. Zonneveld (Cambridge: CUP), 245–291.
Schwartz, B., and Sprouse, R. (1996). L2 cognitive states and the Full Transfer/Full Access model. Second Lang. Res. 12, 40–72.
Smolensky, P. (1996). On the comprehension/production dilemma in child language. Ling. Inq. 27, 720–731.
Smolensky, P., and Géraldine, L. (2006). The Harmonic Mind: From Neural Computation to Optimality-Theoretic Grammar. Cambridge: MIT Press.
Steriade, D. (2001). The phonology of Perceptibility Effects: The P-map and Its Consequences for Constraint Organization. Los Angeles, CA: UCLA.
Turnbull, R., Seyfarth, S., Hume, E., and Jaeger, T. F. (2018). Nasal place assimilation trades off inferability of both target and trigger words. Lab. Phonol. J. Assoc. Lab. Phonol. 9:15. doi: 10.5334/labphon.119
van Leussen, J. W., and Escudero, P. (2015). Learning to perceive and recognize asecond language: the L2LP model revised. Front. Psychol. 6:1000. doi: 10.3389/fpsyg.2015.01000
Wang, J. Z. (1993). The Geometry of Segmental Features in Beijing Mandarin. Delaware: University of Delaware.
Xu, S. (1980). Putonghua Yuyin Zhishi [Phonology of Standard Chinese]. Beijing: WenziGaige Chubanshe.
Yip, M. (1993). Cantonese loanword phonology and optimality theory. J. East Asian Ling. 2, 261–291. doi: 10.1007/BF01739135
Zhang, S. (2023). First language effects on second language perception: evidence from english low-vowel nasal sequences perceived by l1 Mandarin Chinese listeners. Proc. Interspeech 2023, 4184–4188. doi: 10.21437/Interspeech.2023-1123
Keywords: phonological acquisition, L2 phonology, phonological variation, Mandarin, harmonic grammar, phonological learnability, gradual learning algorithm
Citation: Zhang S and Tessier A-M (2024) Modeling the consequences of an L1 grammar for L2 production: simulations, variation, and predictions. Front. Lang. Sci. 3:1327600. doi: 10.3389/flang.2024.1327600
Received: 25 October 2023; Accepted: 07 March 2024;
Published: 23 April 2024.
Edited by:
Baris Kabak, Julius Maximilian University of Würzburg, GermanyReviewed by:
Kakeru Yazawa, University of Tsukuba, JapanCopyright © 2024 Zhang and Tessier. This is an open-access article distributed under the terms of the Creative Commons Attribution License (CC BY). The use, distribution or reproduction in other forums is permitted, provided the original author(s) and the copyright owner(s) are credited and that the original publication in this journal is cited, in accordance with accepted academic practice. No use, distribution or reproduction is permitted which does not comply with these terms.
*Correspondence: Anne-Michelle Tessier, YW10ZXNzaWVyQHViYy5jYQ==
Disclaimer: All claims expressed in this article are solely those of the authors and do not necessarily represent those of their affiliated organizations, or those of the publisher, the editors and the reviewers. Any product that may be evaluated in this article or claim that may be made by its manufacturer is not guaranteed or endorsed by the publisher.
Research integrity at Frontiers
Learn more about the work of our research integrity team to safeguard the quality of each article we publish.