- Language Education Center, Ritsumeikan University, Kyoto, Japan
This article fulfills the need for quantifiable, physical, common characteristics across different languages, which is needed to support the theory that humans use domain-general neurocognitive machinery to acquire, process, and produce language. It is shown that four different languages—English, German, Slovak and Japanese—contain linguistic chunks characterized by at least one redundancy, degeneracy, pluripotentiality, or modularity (R, D, P, or M, respectively) trait, following precedent from other fields of signal investigation. It is first established that language can be regarded as a set of signals between individuals within a complex adaptive system framework and that all signals in all signaling systems exhibit R, D, P, and/or M traits. It is then shown that linguistic chunks can be regarded as individual signals and that the chunks examined from the aforementioned languages express at least one R, D, P, and/or M trait. The present contribution thereby indicates the potential provision of a new source of data for quantifying some of the pressures involved in language production and processing, and the work concludes by assessing the value of the present work for further investigation in related fields.
1 Introduction
All human societies use language to communicate (see Gontier, 2022, for a more extensive treatment of what language is), and all humans use the same neurocognitive machinery to process and produce language (Del Maschio and Abutalebi, 2019; Macuch Silva et al., 2020; Malik-Moraleda et al., 2022). However, the characterization of common traits across different languages is lacking. This work presents a suggestion for classifying linguistic chunks, thereby allowing their quantitative analysis across different languages in the future. A tentative first assessment of this suggested system of classification is then made across four languages—English, German, Slovak, and Japanese.
The investigation begins by establishing whether it is possible to view language as a system of signals. This is done by assessing language as a phenomenon within a complex adaptive system (CAS) theory framework, and it is shown that it is possible to consider language as a system of signals between independent actors in a CAS. The investigation continues by identifying commonalities across all systems of signaling within CASs, establishing that it is feasible to regard linguistic “chunks” as signals, and it finishes by verifying the existence of four universal chunk traits across four different languages, all of which have been used on a routine basis by the author at one time or another.
2 Considering language a system of signals
Language has been viewed as a system of signals (Holler and Levinson, 2019; Macuch Silva et al., 2020), although this view is also contested (Reboul, 2015). To further substantiate this idea, the concept of language as a system of communication between autonomous agents (Lipowska and Lipowski, 2022) is examined within a CAS theory framework.
2.1 CASs
CAS theory seeks to understand how seemingly unconnected individual, autonomous, possibly heterogeneous components inside a chaotic assembly interact and exhibit a sustained, recognizable self-organization at the macro-level. Furthermore, the activity of these autonomous actors produces a predictable behavior of the system as an entity observable from outside the system—the behavior emerges. However, the behavior of individual actors in the system and the behavior of the system as a whole might be very different. Since the phrase “complex adaptive system” was first used in the late 1960s by Walter F. Buckley (1968) and the framework developed further in the 1990s by such as Gell-Mann (1994), Holland (1996), and Kauffman (1993), CAS theory has been applied to many areas to better facilitate an understanding of them, including immune systems (Grilo et al., 2002), the economy (Tesfatsion, 2003), the brain (Sporns et al., 2000; Singer, 2018), organized crime gangs (Magliocca et al., 2019), supply chain networks (Surana et al., 2005) and language (Larsen-Freeman, 1997; Cornish et al., 2009; Five Graces Group et al., 2009). A domain-general CAS model is presented in Figure 1.
Inside the system, (A) “Actors”—free to enter and leave a system, for example, through birth and death or migration between communities—are possibly heterogeneous, self-maximizing, self-replicating, autonomous individuals that use energy; there is no central control over their behavior, and whether consciously or not, all these individuals are doing what is best for themselves, which may, under certain conditions of reciprocity, include helping each other. (B) “Interaction between actors” is any kind of transfer between actors, including information as signals, resources, and/or collaboration. (D) “Feedback” comes from the outside environment, and it feeds down to interactions between individual actors inside the system. Feedback can be positive, which increases the activity of actors and leads to growth, chaos, and instability, or negative, which stabilizes or reduces activity by actors and leads to a steadier state. Feedback might then lead to (E) “Change of actor characteristics and behaviors,” which may or may not change the nature of the interactions of actors. Meanwhile, at the macro-level, (C) “Emergent behavior” is how the system appears to the outside world, and from the outside an observer sees a system with properties and/or behaviors that the individual actors do not possess on their own and that only emerge when the individual actors interact.
As the ideas regarding CAS theory have become more developed, commonalities across systems have been identified, thereby enabling all examples to be discussed in the same way. An early attempt is shown in Table 1. Tables 2 and 3 show different examples of CASs created to better fit the model in Figure 1.
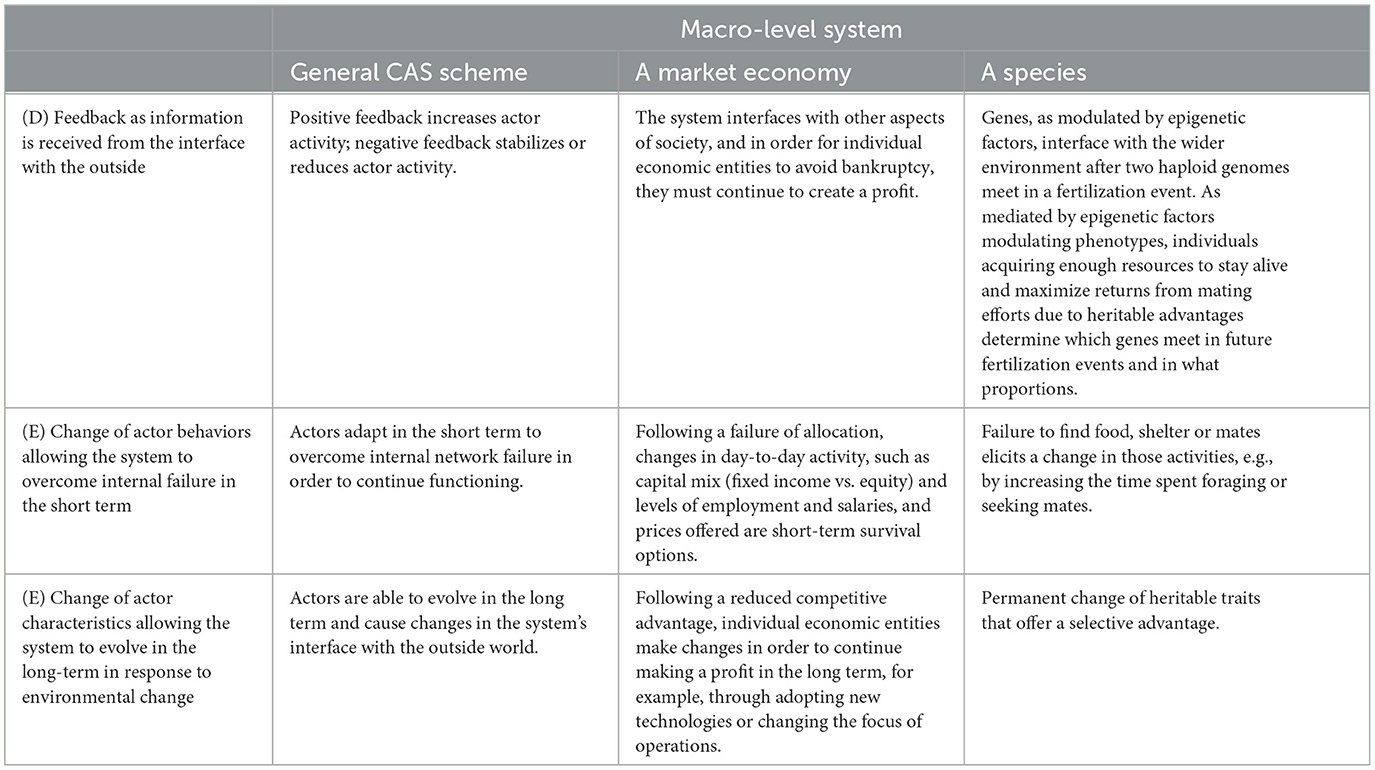
Table 3. Comparison of feedback from outside the system and changes made in different complex adaptive systems (CASs).
2.2 CASs and self-organization of autonomous individuals without central control
Another defining characteristic of a CAS is chaos inside the system as many autonomous individuals go about their activities, resulting in order and predictability of the system overall. For this reason, a bee colony, for example, cannot be viewed in a CAS framework as individuals are under the complete control of a central agent, the queen.
2.3 CASs and energy use
Pertaining to a later section about whether language is a CAS, the idea of CASs using energy is a core idea at the heart of CAS theory. Some self-organizing systems do not use energy, for example, a crystal forming, and are energetically near equilibrium. However, a central definition of a CAS is that individual actors are using energy and that the system maintains a state away from equilibrium. For this reason, almost all CASs are connected somehow with living organisms, humans or otherwise, and the conceptualization of different biological systems in a CAS framework has a long and diverse history.
2.4 CASs and transfer of information between individual actors
Signal transfer between individual actors is by no means a new idea in CAS thinking, and it was a central idea in Holland's early work (2006). At the micro-level, interactions between actors are facilitated by some kind of exchange of information.
2.5 CASs are resilient against outside disturbances and robust against internal failure
CASs are resilient and are able to recover from disturbances from the environment outside the system and adapt in response to long-term changes; they are also robust in that they can overcome partial failures inside the system, for example, when a node fails to develop a function (Klau and Weiskircher, 2005; Sha and Panchal, 2013; Chen and Crilly, 2014; Peckre et al., 2019; Davis et al., 2021; Gillett, 2021). Resilience and robustness (R&R) are important traits in network science, and they are referred to again in the Discussion.
2.6 A definition of CASs
For the purpose of this work, the following is a definition of CASs:
• CASs exhibit innate self-organization of autonomous individuals without central control, allowing predictable order to emerge and be observable from outside;
• CASs use energy to maintain a state away from equilibrium;
• CASs are made up of individual actors that transfer information between each other, for example, as signals;
• CASs are robust to internal failure and resilient as they evolve with a changing environment.
2.7 Language per se is not a CAS
The phenomenon of language cannot be considered a CAS because if linguistic chunks were the individual actors in the system, they do not themselves metabolize energy to continue through time in a state away from equilibrium. Furthermore, linguistic chunks within a generation are not self-maximizing autonomous individuals as they need other linguistic chunks of a different type to be able to function in the system: if the system were all one type of linguistic chunk expressing the same piece of information, that system could not function.
2.8 Comparing different signaling systems and commonalities across different CASs
Signaling systems have been analyzed in different theoretical frameworks, and some kind of information transfer is seen in all CASs, including species (Maynard Smith and Harper, 2003; Rendall et al., 2009; Bradbury and Vehrencamp, 2011), central nervous systems (e.g., Fung and Vanden Berghe, 2020; Chen et al., 2021), commercial activity (e.g., Fredin and Lidén, 2020; Adamchuk et al., 2021; Arthur, 2021), developing embryos (e.g., Johnson and Toettcher, 2019; Kölle et al., 2020), genomes (Dawkins, 1976; Maynard Smith and Szathmáry, 1995; Maynard Smith and Harper, 2003, when discussing genetic replicators1), and immune systems (e.g., Tiberio et al., 2018; Dorrington and Fraser, 2019), and some examples of signals between actors in CASs are presented in Table 4.
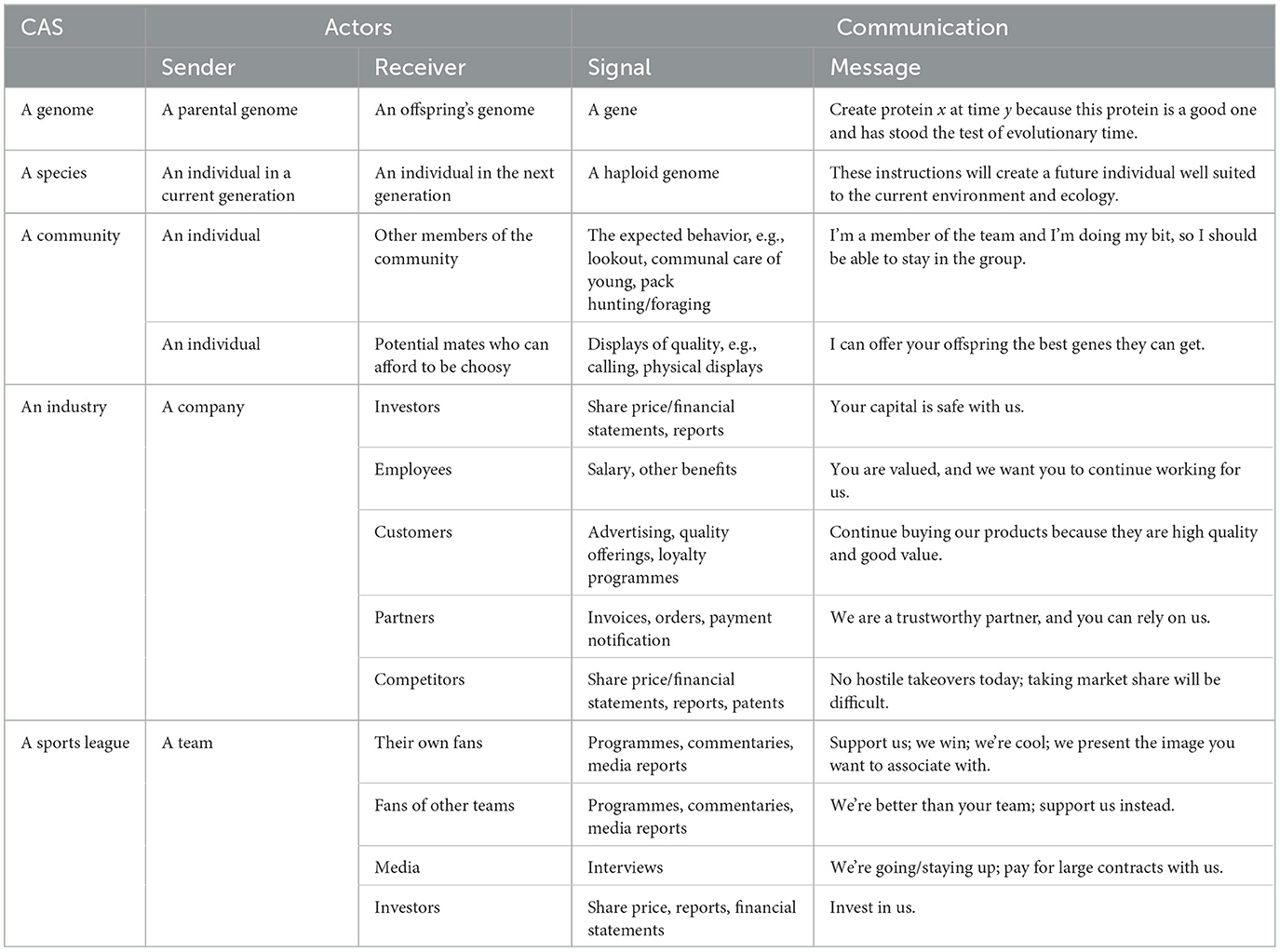
Table 4. Examples of information transferred as signals as seen in some example complex adaptive systems (CASs).
2.9 Individuals within a system who use more complex signaling are more successful
In some CASs, it is easy to perceive actors competing against each other to self-maximize, for example, in a sports league or a species. However, in other systems, such as the immune system of an individual, although actors are autonomous, it is less easy to appreciate on what level they are competing and how they would self-maximize, and such a discussion is beyond the necessary realms of the current work. However, if the actors are not competing with each other, the system is not a CAS, and in a CAS, individuals who can create and use more complex signals are more successful (Choi et al., 2022), for example, the lesser prairie chicken (Gould and Augustine, 2020) and the wolf spider (Hebets et al., 2013). Indeed, much of the returns to complexity in animal mating systems are accrued through not making mistakes between closely related species in mating situations (Tibbetts et al., 2020), which would constitute a waste of valuable resources with the creation of infertile hybrid offspring.
2.10 More complex CASs have more complex signaling systems
More complex CASs have been shown to have more complex signaling systems (Freeberg et al., 2012; Peckre et al., 2019). More complex systems are those where individual actors come into contact more often, especially the same individuals (Freeberg et al., 2012); have larger groups of individuals interacting (Knörnschild et al., 2020); have greater chances of uncertainty (Rebout et al., 2021); have a greater number of social alliances, more social competition among groups, and/or a higher number of different social roles within a group (Sewall, 2015); or have more complex mating and offspring-care systems (Peckre et al., 2019).
2.11 Why other animals do not have a signaling system as complex as human language
Proto-human societies, living hunter-gatherer lifestyles in multi-family groups of different generations, must have already been highly complex when language started to evolve, with actors exhibiting different activities and having different motivations, and no other animal society can be said to have ever been as complex. A theory has been put forward and largely corroborated saying that signaling systems are as complex as they need to be, and as no other animal society is as complex as human societies were when language started to evolve, no other signaling system is as complex as human language (Beecher, 2021; Penn and Számadó, 2021; Wacewicz, 2021).
2.12 Alone, a single signal is likely to be ambiguous and equivocal
Signals between animals of the same species appear to be ambiguous (Santana, 2014), and ambiguous signals seem to be associated with multimodal signaling (Partan and Marler, 2005; Mühlenbernd, 2021). This also appears to be true in human languages (Piantadosi et al., 2012; Gibson et al., 2019).
2.13 A definition of signals in CASs
For the purpose of this work, language as an uncountable noun is to be considered a system of signals between actors in the CAS of human communities. Signals in CASs have been studied extensively (Dawkins, 1976; Maynard Smith and Szathmáry, 1995; Maynard Smith and Harper, 2003; Scott-Phillips, 2008; Holland, 2012; Barker et al., 2019), and it is possible to make the following definition of signals in CASs:
• The primary purpose of a signal is to transfer information, that is, all signals contain information that is immediately processed as a recognized meaning;
• Alone, a single signal is likely to be ambiguous and equivocal while being the smallest constituent part that retains some meaning;
• Signals are intentional and honest;
• Signals are the same as those used by individuals in previous generations;
• Signals are reproduced each time they are used;
• Each copy of a signal contains some variation/inaccuracy.
2.14 Different signaling systems have common characteristics
It was earlier stated that within a system, individuals using more complex signaling are more successful and that across systems, more complex systems use more complex signaling systems. There are two main routes for increasing complexity that have been identified in theory and that are found to a lesser or greater extent in all signaling systems: increasing the number of signals for any given unit of information/meaning, called degeneracy (D), and; increasing the number of uses for a given signal, called modularity (Chen and Crilly, 2014; Hebets et al., 2016; Peckre et al., 2019; M). Moreover, within a signaling system, degeneracy and modularity will always arise (Newman, 2006; Chen and Crilly, 2014) as the added complexity provides benefits. Furthermore, in more complex systems, different types of degeneracy and modularity can be found, namely, redundancy (R) as a form of degeneracy with different signals used simultaneously and expressing the same meaning and pluripotentiality (P), or multifunctionality, as a form of modularity where a single signal has a number of different, related context-dependent meanings. Table 5 compares the R, D, P, and M in two systems.
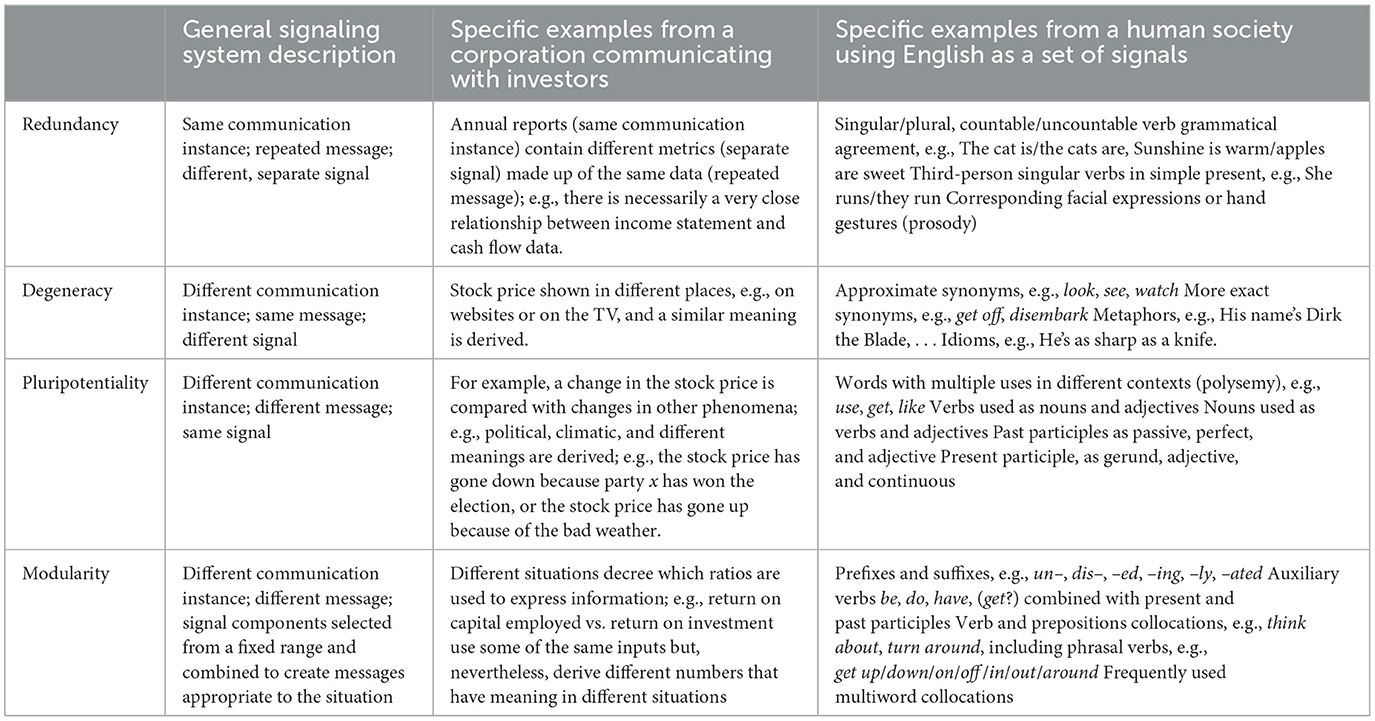
Table 5. Redundancy, degeneracy, pluripotentiality, and modularity in two different signaling systems.
For example, a degenerate system of communication between actors in a CAS that appears to be functionally redundant with respect to particular outcomes in a particular context but that may perform differently in a different context was discussed in work by Tononi et al. (1999), who worked on the functioning of the human brain. They also stated that “redundant systems in which many elements can affect the output in a similar way but do not have independent effects.” The relationship and distinction between redundancy and degeneracy were also later discussed by Friston and Price (2003)—again with respect to brain function—who said that “degeneracy refers to a structure-function relationship…. Redundancy refers to the function of a necessarily degenerate set of structures,” and that, “degeneracy is necessary for redundancy.” Chen and Crilly (2014), in discussing the matter, make the distinction that redundancy is spare capacity, whereas degeneracy is different structures performing the same function in certain situations.
Additional modularity in CASs has been shown to be another route to increasing complexity, for example, in engineering systems (Sinha and Suh, 2018) and the power, propulsion, and cooling systems of naval ships (Paparistodimou et al., 2020). In signaling systems, modular signals have been shown to increase signal complexity in some species of monkeys (Snowdon and Ziegler, 2021) and bacteria (Hengge, 2021).
2.15 Increasing systems stability as modularity balances degeneracy
There is a huge lack of consensus across the network science literature as to what redundancy and resilience are, and most descriptions are not mutually exclusive. In this work, the signal characteristic of degeneracy is said to endow a network of signals with the network characteristic of robustness, and likewise, the signal characteristic of modularity confers the network characteristic of resilience. A networked system of signals that has a number of different linkages within it derived either from degeneracy and/or modularity can now be envisaged. This “and/or” situation is of great importance, and a single signal would have a mix of R, D, P, and M traits that is different to the mix exhibited by another signal. Therefore, for example, the more overlapping R and D traits of a signal, the more sound and meaning nodes and edges in a network would be shared by that signal with other signals, and the more likely the network would be able to overcome any internal problems: a phenomenon in network science called network buffering (Whitacre and Bender, 2010). Nevertheless, network buffering has complications because while system-wide connections—or connectedness—offer advantages, this same measure in excess—or over-connectedness—can increase the risk of cascades of potentially catastrophic systemic failure after an external disturbance, when one failure leads to more because all the failing components are interlinked. However, this risk is mitigated by modularity (Carpenter et al., 2012; Clune et al., 2013; Walker, 2020). Therefore, a system with some degenerate signals, some modular signals, and some signals with both traits would have a more reliable functionality in an unpredictable environment.
3 Linguistic “chunks” as the signals in human language
Table 5 presents a scheme depicting the characteristics of signals between actors in two different CASs. If human society is a CAS, and human language in all its forms—German, Persian, Hindi, Russian, and others—is a signaling system between actors in that CAS, the actual signals themselves must be identified.
3.1 Identifying the signals between actors in the CAS of human society
Returning to the definition of a signal that was given earlier, the first requirement is that a signal must have information that is immediately processed as a recognized meaning, which relates to the processing that has been carried out previously or something that has been learnt previously; the second requirement is that although a single signal is likely to be small, ambiguous, and equivocal, it must still contain some meaning. Nevertheless, when deciding how to discuss signals in languages, there is a huge problem with terminology and with what certain specific terms have become associated with through time. A term that relates both to processing and meaning is chunk. Here, it is proposed that linguistic chunks and signals in language are synonymous, as both must be recognized immediately to allow reflex-like processing and both must contain a meaning.
The term chunk is not new, and the idea of working memory having a limited capacity has a long history (Miller, 1956). It is also theorized that the capacity of working memory is limited by the number of chunks that can be stored, not the number of items or the amount of information (Gobet et al., 2016). It is postulated that chunking is a neurocognitive mechanism carried out when humans use language (Christiansen and Arnon, 2017; McCauley and Christiansen, 2017), and a “linguistic chunk,” to paraphrase an early scholar in the field (Abney, 1995), describes an encoded, or known, sound pattern with a concomitant meaning that is processed simultaneously. Furthermore, it is postulated that this processing is instantaneous and carried out in a reflex-like manner because the processing function has been proceduralised (O'Grady, 2015; Ellis and Ogden, 2017; DeKeyser, 2018) after successive repetition of the same processing routine.
It must therefore be asked how multiword sound patterns that constitute a chunk are to be differentiated from those multiword sound patterns that do not. To answer this question, the ideas of variability in working memory and the process of proceduralisation must be considered. Across individuals, there is variation in the capacity of working memory and, therefore, it is presumed here, the size of chunks that can be processed in a single routine, including variation in individuals of the same age (Daneman and Carpenter, 1980) and older individuals usually being able to accommodate larger chunks (Cowan, 2016). This difference is also augmented with training (Titz and Karbach, 2014; Schwaighofer et al., 2015) and related to language use and learning (Huettig and Janse, 2016; Kidd et al., 2018). However, proceduralisation occurs through neuroplasticity, which is a neurological growth process and during which neurones repeatedly used in a certain process reinforce their connectivity to enable rapid reactions (Lillard and Erisir, 2011; Gallistel and Matzel, 2013). This idea is enshrined in the wellworn axiom “neurones that fire together, wire together” (Hebb, 1949). Taken together, these ideas would predict that what constitutes a chunk would be different for people of different ages and in topics for which different people have had different amounts of prior exposure. Therefore, older people with more knowledge and experience in a certain topic would be able to process larger and more specific multiword sound patterns than younger people with less experience in the same topic. Moreover, of course, what constitutes a chunk to a monolingual speaker of, for example, German, would be different to what constitutes a chunk of, for example, Japanese. Furthermore, it is suggested here that what constitutes a signal in any given language might also contain collocations, constructions, words, morphemes, phonemes, and any other unit in that same language.
4 Methods
4.1 Examining different languages for linguistic chunks with r, d, p, and m traits
Having identified chunks as being individual signals in the signaling system of the CAS of human communities, an initial assessment of different languages for the presence of sound-meaning pairs that have R, D, P, and M traits was made. Examples are presented from four languages for R in Table 6, D in Table 7, P in Table 8, and M in Table 9.
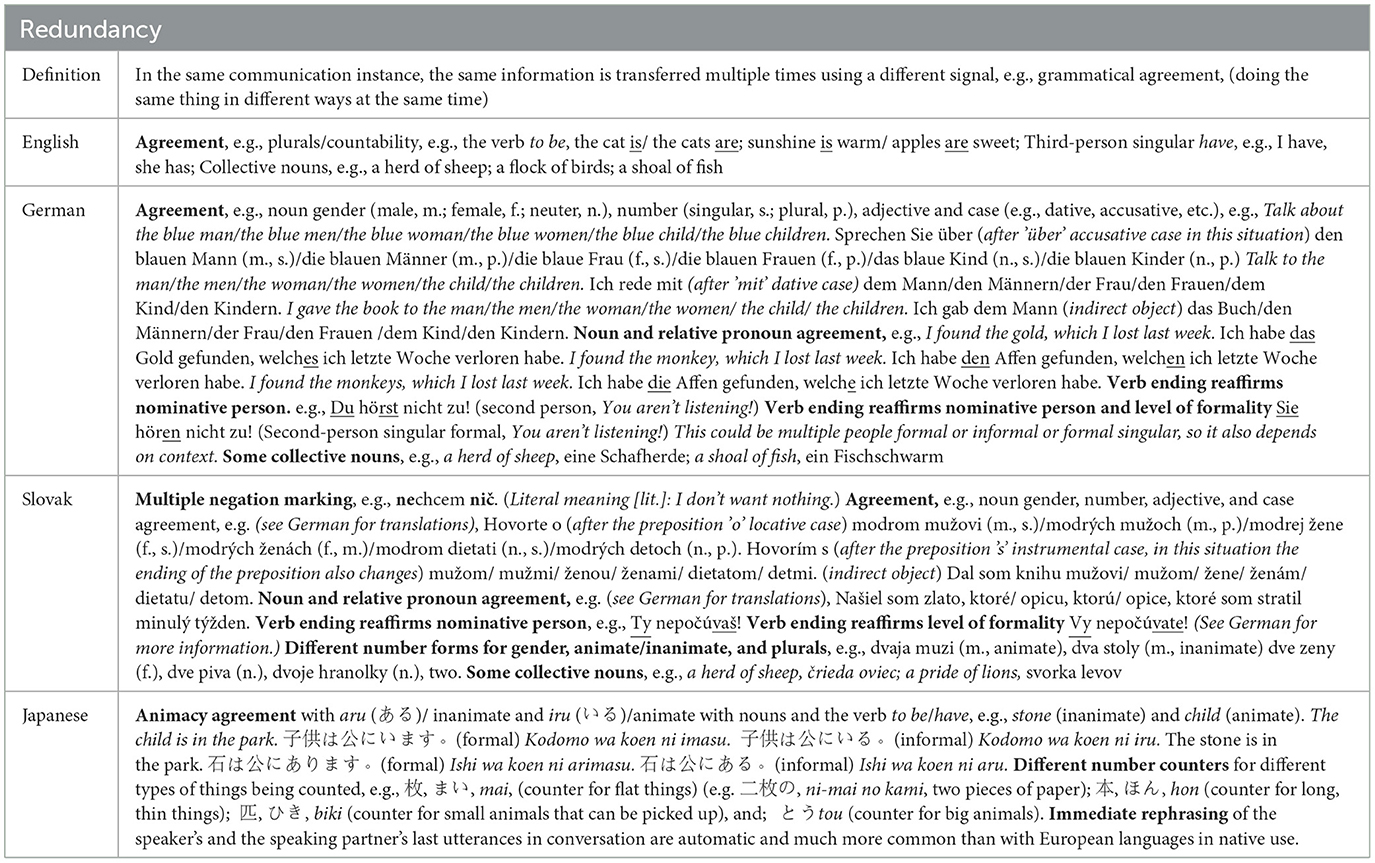
Table 6. Comparisons of constructions exhibiting the trait of redundancy in English, German, Slovak, and Japanese.
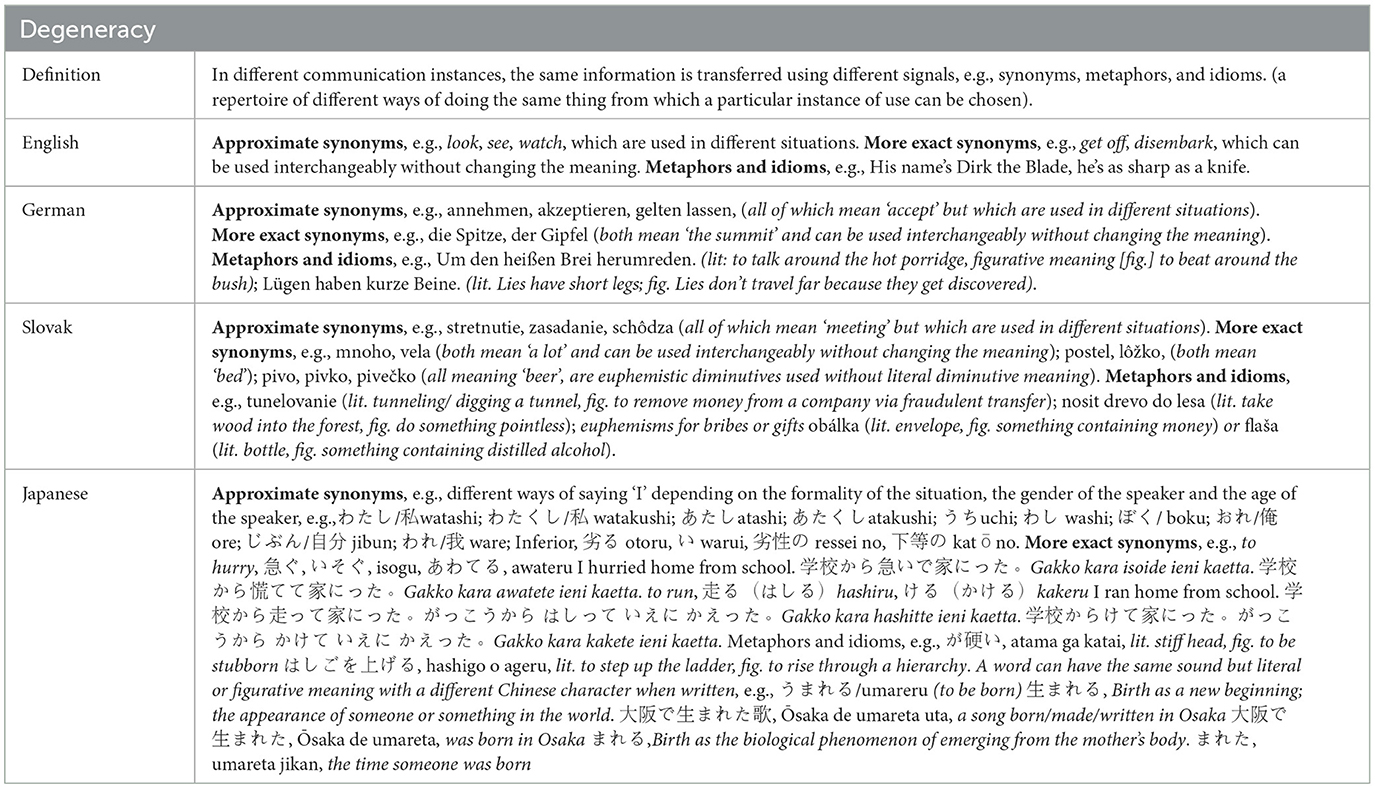
Table 7. Comparisons of constructions exhibiting the trait of degeneracy in English, German, Slovak, and Japanese.
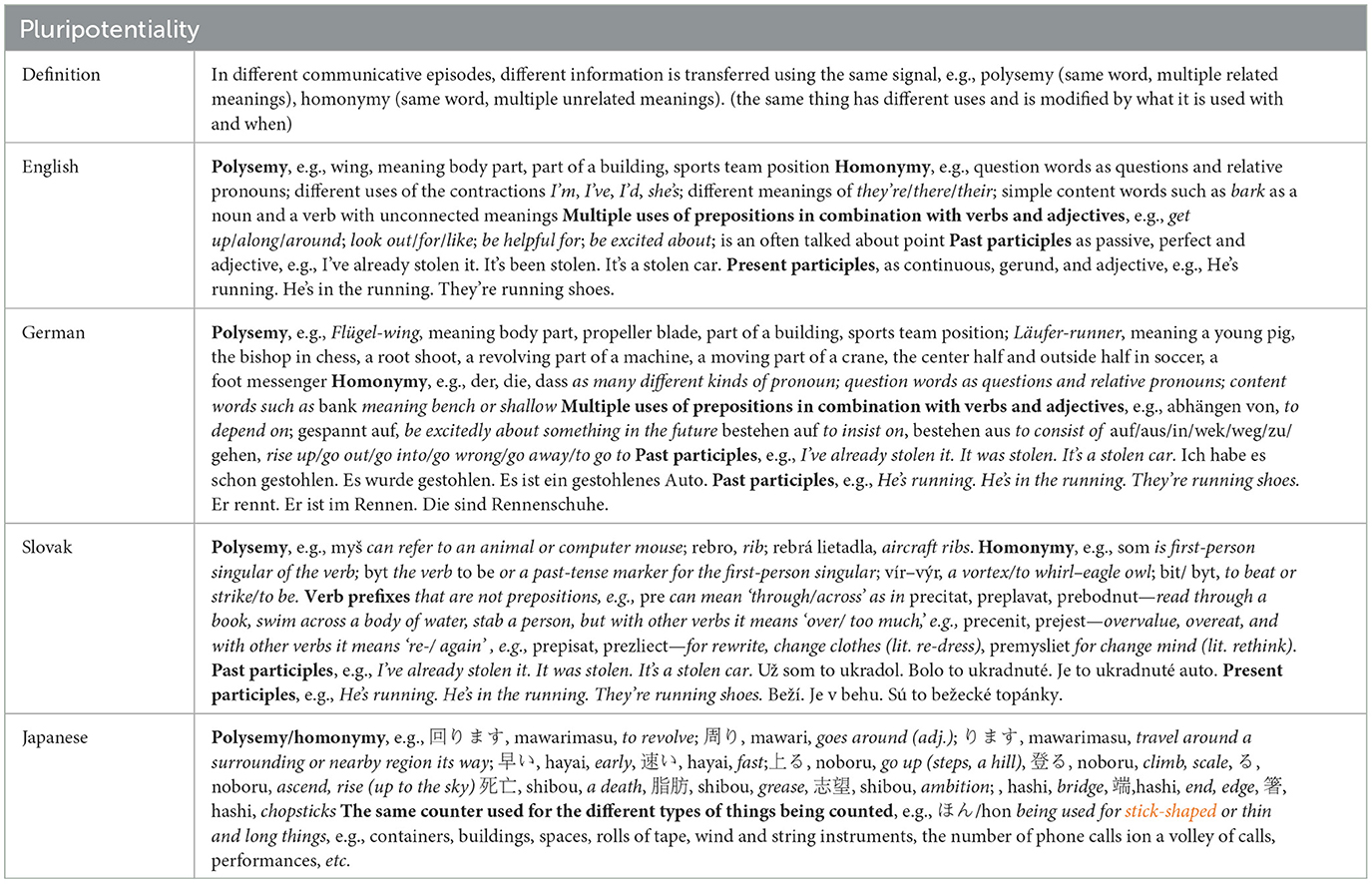
Table 8. Comparisons of constructions exhibiting the trait of pluripotentiality in English, German, Slovak, and Japanese.
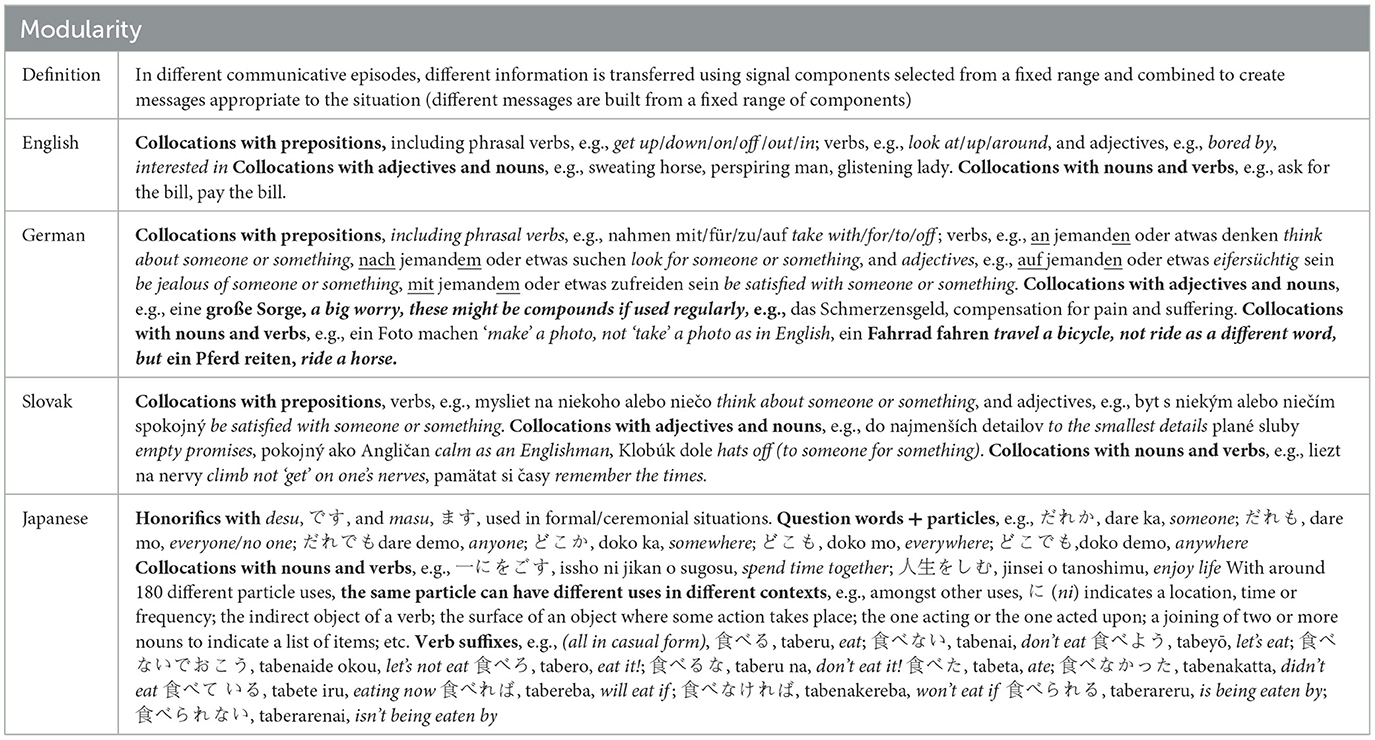
Table 9. Comparisons of constructions exhibiting the trait of modularity in English, German, Slovak, and Japanese.
5 Results
As can be clearly seen, all four languages express R, D, P, and M traits in their common chunks.
6 Discussion
It has been shown that four human languages—English, German, Slovak, and Japanese—contain chunks with at least one R, D, P, or M trait. This is the first time commonalities across different languages have been demonstrated, which is something other approaches have never done. It has also been demonstrated that R, D, P, and M traits are closely related to R&R traits in language when it is viewed as a signaling system in the CAS of the human community.
Again, as stated in the Introduction, this is only a tentative first assessment, and these four languages were chosen because they were well known to the author, not because of the degrees to which any R, D, P or M traits are expressed. Phylogenetic analysis of languages would place English and German as being closely related, Slovak as a close relative of the two, and Japanese as being only distantly related to those three (Greenhill et al., 2010).
Five main avenues of further research are opened up by the current work, namely, the existence of R, D, P, and M traits in the chunks of other languages; the quantification of R, D, P, and M in chunks; the role of chunks in foreign language teaching; the structure in CASs and system stability; and the evolution of different languages. These are discussed in more detail in the following subsections.
6.1 Quantifying r, d, p, and m in chunks
There are a number of reasons why it might be desirable to quantify R, D, P, and/or M in a chunk. For example, we might wish to compare R, D, P, and M traits in chunks from different languages or different chunks in the same language to investigate language processability or learnability. There are two sources of precedential work that can be drawn on to provide direction: corpus linguistics and network science in the natural sciences and engineering. Measures from corpus linguistics can be further divided into syntactic association measures (e.g., Gries and Ellis, 2015; Gablasova et al., 2017; Ellis and Wulff, 2019) and semantic association measures (e.g., Glynn and Robinson, 2014; Gries, 2015; Katz, 2019), while measures from network sciences can be further divided into robustness estimation (e.g., Peng et al., 2018; Dong et al., 2019), resilience estimation (e.g., Klau and Weiskircher, 2005; Turnquist and Vugrin, 2013), network connectivity (assortivity coefficient; e.g., Newman, 2003; Peel et al., 2018), and the potential for cascades of failure leading to collapse (e.g., Gutfraind, 2012; Liu et al., 2014).
6.2 The role of chunks in foreign language teaching
Prior work has shown R, D, P, and M traits improve learnability and usability, and examples of experimental evidence supporting (1) statistical and relational learning of language and/or (2) processing and production in use can be found for redundancy (e.g., Wang and Mintz, 2018; Bahrick et al., 2019; Brehm et al., 2020; Lany and Shoaib, 2020; Tal and Arnon, 2022), degeneracy (e.g., Vulchanova et al., 2015, 2019; Gentner and Asmuth, 2017; Thibodeau et al., 2019; Starr et al., 2021), pluripotentiality (e.g., Brocher et al., 2018; Srinivasan et al., 2019; Floyd and Goldberg, 2021), and modularity (e.g., Boers and Lindstromberg, 2012; Conklin and Schmitt, 2012; Christiansen and Arnon, 2017). However, since an individual construction can have multiple overlapping R, D, P, and/or M characteristics, meaning those traits amenable to learning and use are also overlapping, it might be difficult to separate out individually the effects of any particular trait of a certain chunk.
6.3 Structure in CASs and system stability
R, D, P and M and R&R add extra levels of structural complexity to language when viewed as a signaling system in a CAS. Parallels between structure in language and proteins have been drawn before (Lackova, 2018), and the signaling systems of other CASs also have extra levels of structural complexity; for example, DNA has supercoiling, and some animal mating systems take place in special groupings called leks. The function and origin of these extra levels of complexity are of great interest for system stability when the environment is unchanging and for evolvability/survival when the environment is changing.
6.4 The evolution of different languages
The genesis of different languages is a topic of contention amongst linguists and is much debated. Now having quantitative factors upon which the effects of the forces of selection can be measured might allow further resolution of some of the confounding ideas when considering why a single species, that is, Homo sapiens, uses so many different unintelligible variations of language.
Data availability statement
The original contributions presented in the study are included in the article/supplementary material, further inquiries can be directed to the corresponding author.
Author contributions
The author developed the concepts and wrote the manuscripts and is thankful for the assistance of those people mentioned in the acknowledgments for assistance with and verification of the languages other than English that are used here.
Acknowledgments
I am grateful to the following for their help with languages: Tessy Albonetti (German) and Yukiko Nakayama (Japanese) as native speakers and Richard Swales (Slovak) as a long-term resident of the Slovak Republic with near-native proficiency. I am also grateful to Andrew Plumb and Richard Swales and two reviewers for their comments on the manuscript.
Conflict of interest
The author declares that the research was conducted in the absence of any commercial or financial relationships that could be construed as a potential conflict of interest.
Publisher's note
All claims expressed in this article are solely those of the authors and do not necessarily represent those of their affiliated organizations, or those of the publisher, the editors and the reviewers. Any product that may be evaluated in this article, or claim that may be made by its manufacturer, is not guaranteed or endorsed by the publisher.
Footnotes
1. ^Epigenetic mechanisms of evolution do not have their own replicators, are not signals and cannot be considered replicators themselves.
References
Abney, S. (1995). Chunks and dependencies: Bringing processing evidence to bear on syntax. Comput. Linguist. Found. Linguistic Theory 145–164.
Adamchuk, N., Osipov, V., and Tsvetkova, L. (2021). “Insurance companies: prospective business models,” in: Financial Markets Evolution (Cham: Palgrave Macmillan), 187–198.
Adrian-Kalchhauser, I., Sultan, S. E., Shama, L. N., Spence-Jones, H., Tiso, S., Valsecchi, C. I. K., et al. (2020). Understanding'non-genetic'inheritance: insights from molecular-evolutionary crosstalk. Trends Ecol. Evol. 35, 1078–1089. doi: 10.1016/j.tree.2020.08.011
Arthur, W. B. (2021). Foundations of complexity economics. Nat. Rev. Phy. 3, 136–145. doi: 10.1038/s42254-020-00273-3
Ashe, A., Colot, V., and Oldroyd, B. P. (2021). How does epigenetics influence the course of evolution?. Philosophic. Transact. Roy. Society B 376, 0111. doi: 10.1098/rstb.2020.0111
Bahrick, L. E., McNew, M. E., Pruden, S. M., and Castellanos, I. (2019). Intersensory redundancy promotes infant detection of prosody in infant-directed speech. J. Exp. Child Psychol. 183, 295–309. doi: 10.1016/j.jecp.2019.02.008
Banta, J. A., and Richards, C. L. (2018). Quantitative epigenetics and evolution. Heredity 121, 210–224. doi: 10.1038/s41437-018-0114-x
Barker, J. L., Power, E. A., Heap, S., Puurtinen, M., and Sosis, R. (2019). Content, cost, and context: a framework for understanding human signaling systems. Evol. Anthropol. Issues News Rev. 28, 86–99. doi: 10.1002/evan.21768
Beecher, M. D. (2021) Why are no animal communication systems simple languages? Front. Psychol. 12, 602635. doi: 10.3389/fpsyg.2021.602635.
Boers, F., and Lindstromberg, S. (2012). Experimental and intervention studies on formulaic sequences in a second language. Ann. Rev. Appl. Linguist. 32, 83–110. doi: 10.1017/S0267190512000050
Bradbury, J. W., and Vehrencamp, S. L. (2011). Principles of Animal Communication (2nd ed.). Cary, NC: Sinauer Associates.
Brehm, L., Hussey, E., and Christianson, K. (2020). The role of word frequency and morpho-orthography in agreement processing. Lang. Cogn. Neurosci. 35, 58–77. doi: 10.1080/23273798.2019.1631456
Brocher, A., Koenig, J. P., Mauner, G., and Foraker, S. (2018). About sharing and commitment: the retrieval of biased and balanced irregular polysemes. Lang. Cogn. Neurosc. 33, 443–466. doi: 10.1080/23273798.2017.1381748
Buckley, W. (1968). “Society as a complex adaptive system,” in Modern Systems Research for the Behavioral Scientist. Kusterdingen: De Gruyter
Carpenter, S. R., Arrow, K. J., Barrett, S., Biggs, R., Brock, W. A., Crépin, A.-S., et al. (2012). General resilience to cope with extreme events. Sustainability 4, 3248–3259. doi: 10.3390/su4123248
Chen, C. C., and Crilly, N. (2014). “Modularity, redundancy and degeneracy: cross-domain perspectives on key design principles,” in 2014 IEEE International Systems Conference Proceedings (New York, NY: IEEE), 546–553.
Chen, W. G., Schloesser, D., Arensdorf, A. M., Simmons, J. M., Cui, C., Valentino, R., et al. (2021). The emerging science of interoception: sensing, integrating, interpreting, and regulating signals within the self. Trends Neurosci. 44, 3–16. doi: 10.1016/j.tins.2020.10.007
Choi, N., Adams, M., Fowler-Finn, K., Knowlton, E., Rosenthal, M., Rundus, A., et al. (2022). Increased signal complexity is associated with increased mating success. Biol. Lett. 18, 0052. doi: 10.1098/rsbl.2022.0052
Christiansen, M. H., and Arnon, I. (2017). More than words: the role of multiword sequences in language learning and use. Topics Cogn. Sci. 9, 542–551. doi: 10.1111/tops.12274
Clune, J., Mouret, J. B., and Lipson, H. (2013). The evolutionary origins of modularity. Proc. R. Soc. Lond. B. 280, 2863. doi: 10.1098/rspb.2012.2863
Conklin, K., and Schmitt, N. (2012). The processing of formulaic language. Ann. Rev. Appl. Linguis. 32, 45–61. doi: 10.1017/S0267190512000074
Cornish, H., Tamariz, M., and Kirby, S. (2009). Complex adaptive systems and the origins of adaptive structure: what experiments can tell us. Lang. Learn. 59, 187–205. doi: 10.1111/j.1467-9922.2009.00540.x
Cowan, N. (2016). Working memory maturation: can we get at the essence of cognitive growth?. Perspect. Psychologic. Sci. 11, 239–264. doi: 10.1177/1745691615621279
Daneman, M., and Carpenter, P. A. (1980). Individual differences in working memory and reading. J. Verb. Learn. Verb. Behav. 19, 450–466. doi: 10.1016/S0022-5371(80)90312-6
Davis, J. E., Kolozsvary, M. B., Pajerowska-Mukhtar, K. M., and Zhang, B. (2021). Toward a universal theoretical framework to understand robustness and resilience: from cells to systems. Front. Ecol. Evol. 20, 495. doi: 10.3389/fevo.2020.579098
DeKeyser, R. M. (2018). “Task repetition for language learning: a perspective from skill acquisition theory,” in Learning Language through Task Repetition, ed M. Bygate (Amsterdam: John Benjamins Publishing Company), 27–42.
Del Maschio, N., and Abutalebi, J. (2019). “Language organization in the bilingual and multilingual brain,” in The Handbook of the Neuroscience of Multilingualism, ed J. W. Schwieter (New York, NY: Wiley-Blackwell), 197–213.
Dong, S., Wang, H., Mostafavi, A., and Gao, J. (2019). Robust component: a robustness measure that incorporates access to critical facilities under disruptions. J. R. Soc. Interface. 16, 20190149. doi: 10.1098/rsif.2019.0149
Dorrington, M. G., and Fraser, I. D. C. (2019). NF-κB Signaling in macrophages: dynamics, crosstalk, and signal integration. Front. Immunol. 10, 705. doi: 10.3389/fimmu.2019.00705
Ellis, N. C., and Ogden, D. C. (2017). Thinking about multiword constructions: usage-based approaches to acquisition and processing. Topics Cogn. Sci. 9, 604–620 doi: 10.1111/tops.12256
Ellis, N. C., and Wulff, S. (2019). “Cognitive approaches to second language acquisition,” in The Cambridge Handbook of Language Learning, eds J. W. Schwieter and A. G. Benati (Cambridge: Cambridge University Press), 41–61. doi: 10.1017/9781108333603
Five Graces Group, Beckner, C., Blythe, R., Bybee, J., Christiansen, M. H., Croft, W., Nick, C., et al. (2009). Language is a complex adaptive system: Position paper. Lang. Learn. 59, 1–26. doi: 10.1111/j.1467-9922.2009.00533.x
Floyd, S., and Goldberg, A. E. (2021). Children make use of relationships across meanings in word learning. J. Experiment. Psychol. Learn. Mem. Cogn. 47, 29. doi: 10.1037/xlm0000821
Fredin, S., and Lidén, A. (2020). Entrepreneurial ecosystems: towards a systemic approach to entrepreneurship?. Geografisk Tidsskrift-Danish J. Geograph. 120, 87–97. doi: 10.1080/00167223.2020.1769491
Freeberg, T. M., Dunbar, R. I., and Ord, T. J. (2012). Social complexity as a proximate and ultimate factor in communicative complexity. Philosophic. Transact. Royal Soc. B: Biologic. Sci. 367, 1785–1801. doi: 10.1098/rstb.2011.0213
Friston, K. J., and Price, C. J. (2003). Degeneracy and redundancy in cognitive anatomy. Trends Cogn. Sci. 7, 151–152. doi: 10.1016/S1364-6613(03)00054-8
Fung, C., and Vanden Berghe, P. (2020). Functional circuits and signal processing in the enteric nervous system. Cell. Mol. Life Sci. 77, 4505–4522. doi: 10.1007/s00018-020-03543-6
Gablasova, D., Brezina, V., and McEnery, T. (2017). Collocations in corpus-based language learning research: Identifying, comparing, and interpreting the evidence. Lang. Learn. 67, 155–179. doi: 10.1111/lang.12225
Gallistel, C. R., and Matzel, L. D. (2013). The neuroscience of learning: beyond the Hebbian synapse. Ann. Rev. Psychol. 64, 169–200. doi: 10.1146/annurev-psych-113011-143807
Gell-Mann, M. (1994). “Complex adaptive systems,” in Complexity: Metaphors, Models, and Reality. Santa Fe Institute Studies in the Sciences of Complexity. No.19. Addison-Wesley, Reading, MA, 17–45.
Gentner, D., and Asmuth, J. (2017). Metaphoric extension, relational categories, and abstraction. Lang. Cogn. Neurosci. 34, 1298–1307. doi: 10.1080/23273798.2017.1410560
Gibson, E., Futrell, R., Piantadosi, S. P., Dautriche, I., Mahowald, K., Bergen, L., et al. (2019). How efficiency shapes human language. Trends Cogn. Sci. 23, 389–407. doi: 10.1016/j.tics.2019.02.003
Gillett, A. J. (2021). Development, resilience engineering, degeneracy, and cognitive practices. Rev. Phil. Psych. 21, 550. doi: 10.1007/s13164-021-00550-9
Glynn, D., and Robinson, J. A. (2014). Corpus methods for Semantics: Quantitative Studies in Polysemy and Synonymy (Vol. 43). Amsterdam: John Benjamins Publishing Company.
Gobet, F., Lloyd-Kelly, M., and Lane, P. C. (2016). What's in a name? the multiple meanings of “Chunk” and “Chunking.” Front. Psychol. 7, 102. doi: 10.3389/fpsyg.2016.00102
Gontier, N. (2022). Defining communication and language from within a pluralistic evolutionary worldview. Topoi 41, 609–622. doi: 10.1007/s11245-022-09811-3
Gould, G. M., and Augustine, J. K. (2020). Multiple signals predict male mating success in the lek-mating lesser prairie-chicken (Tympanuchus pallidicinctus). Behav. Ecol. Sociobiol. 74, 1–17. doi: 10.1007/s00265-020-02920-2
Greenhill, S. J., Atkinson, Q. D., Meade, A., and Gray, R. D. (2010). The shape and tempo of language evolution. Proceed. Royal Soc. B Biologic. Sci. 277, 2443–2450. doi: 10.1098/rspb.2010.0051
Gries, S. T. (2015). “Polysemy,” in Handbook of cognitive linguistics, eds E. Dabrowska and D. S. Divjak (Berlin: De Gruyter Mouton), 472–490.
Gries, S. T., and Ellis, N. C. (2015). Statistical measures for usage-based linguistics. Lang. Learn. 65, 228–255. doi: 10.1111/lang.12119
Grilo, A., Caetano, A., and Rosa, A. (2002). “Immune system simulation through a complex adaptive system model,” in Soft Computing and Industry, eds R. Roy, M. Köppen, S. Ovaska, T. Furuhashi, F. Hoffmann (London: Springer), 675–698.
Gutfraind, A. (2012). “Optimizing network topology for cascade resilience,” in Handbook of Optimization in Complex Networks. Springer Optimization and Its Applications, eds Thai M., Pardalos P. (New York, NY: Springer).
Hebets, E. A., Barron, A. B., Balakrishnan, C. N., Hauber, M. E., Mason, P. H., and Hoke, K. L. (2016). A systems approach to animal communication. Proceed. Roy. Soc. B: Biologic. Sci. 283, 2889. doi: 10.1098/rspb.2015.2889
Hebets, E. A., Vink, C. J., Sullivan-Beckers, L., and Rosenthal, M. F. (2013). The dominance of seismic signaling and selection for signal complexity in Schizocosa multimodal courtship displays. Behav. Ecol. Sociobiol. 67, 1483–1498. doi: 10.1007/s00265-013-1519-4
Hengge, R. (2021). High-specificity local and global c-di-GMP signaling. Trends Microbiol. 29, 993–1003. doi: 10.1016/j.tim.2021.02.003
Holland, J. H. (1996). Hidden Order: How Adaptation Builds Complexity. Boston: Addison Wesley Longman Publishing Co., Inc.
Holland, J. H. (2006). Studying complex adaptive systems. J. Sys. Sci. Com. 19, 1–8. doi: 10.1007/s11424-006-0001-z
Holland, J. H. (2012). Signals and Boundaries: Building Blocks for Complex Adaptive Systems. Cambridge, MA, MIT Press.
Holler, J., and Levinson, S. C. (2019). Multimodal language processing in human communication. Trends Cogn. Sci. 23, 639–652. doi: 10.1016/j.tics.2019.05.006
Huettig, F., and Janse, E. (2016). Individual differences in working memory and processing speed predict anticipatory spoken language processing in the visual world. Lang. Cogn. Neurosci. 31, 80–93. doi: 10.1080/23273798.2015.1047459
Johnson, H. E., and Toettcher, J. E. (2019). Signaling dynamics control cell fate in the early Drosophila embryo. Develop. Cell 48, 361–370. doi: 10.1016/j.devcel.2019.01.009
Katz, G. (2019). “Semantics in corpus linguistics,” In Semantics—Typology, Diachrony and Processing, eds K. Heusinger, C. Maienborn, P. Portner (Berlin, Boston: De Gruyter Mouton), 409–443. doi
Kauffman, S. (1993). The Origins of Order: Self-Organization and Selection in Evolution. Oxford: Oxford University Press.
Kidd, E., Donnelly, S., and Christiansen, M. H. (2018). Individual differences in language acquisition and processing. Trends Cogn. Sci. 22, 154–169. doi: 10.1016/j.tics.2017.11.006
Klau, G. W., and Weiskircher, R. (2005). “Robustness and resilience,” in Network Analysis. Lecture Notes in Computer Science, eds U. Brandes and T. Erlebach (Berlin, Heidelberg: Springer).
Knörnschild, M., Fernandez, A. A., and Nagy, M. (2020). Vocal information and the navigation of social decisions in bats: is social complexity linked to vocal complexity?. Funct. Ecol. 34, 322–331. doi: 10.1111/1365-2435.13407
Kölle, S., Hughes, B., and Steele, H. (2020). Early embryo-maternal communication in the oviduct: a review. Mol. Reproduct. Develop. 87, 650–662. doi: 10.1002/mrd.23352
Lackova, L. (2018). A Linguistic Approach to Protein Folding. Olomouc. Dissertation Thesis. Palacký University in Olomouc.
Lany, J., and Shoaib, A. (2020). Individual differences in non-adjacent statistical dependency learning in infants. J. Child Lang. 47, 483–507. doi: 10.1017/S0305000919000230
Larsen-Freeman, D. (1997). Chaos/complexity science and second language acquisition. Appl. Linguist. 18, 141–165. doi: 10.1093/applin/18.2.141
Lillard, A. S., and Erisir, A. (2011). Old dogs learning new tricks: neuroplasticity beyond the juvenile period. Develop. Rev. 31, 207–239. doi: 10.1016/j.dr.2011.07.008
Lind, M. I., and Spagopoulou, F. (2018). Evolutionary consequences of epigenetic inheritance. Heredity 121, 205–209. doi: 10.1038/s41437-018-0113-y
Lipowska, D., and Lipowski, A. (2022). Emergence and evolution of language in multi-agent systems. Lingua 272, 103331. doi: 10.1016/j.lingua.2022.103331
Liu, C., Li, D., Zio, E., and Kang, R. (2014). A modeling framework for system restoration from cascading failures. PLoS ONE 9, e112363. doi: 10.1371/journal.pone.0112363
Macuch Silva, V., Holler, J., Ozyurek, A., and Roberts, S. G. (2020). Multimodality and the origin of a novel communication system in face-to-face interaction. R. Soc. Open Sci. 7, 182056. doi: 10.1098/rsos.182056
Magliocca, N. R., McSweeney, K., Sesnie, S. E., Tellman, E., Devine, J. A., Nielsen, E. A., et al. (2019). Modeling cocaine traffickers and counterdrug interdiction forces as a complex adaptive system. Proceed. Nat. Acad. Sci. 116, 7784–7792. doi: 10.1073/pnas.1812459116
Malik-Moraleda, S., Ayyash, D., Gallée, J., Affourtit, J., Hoffmann, M., Mineroff, Z., et al. (2022). An investigation across 45 languages and 12 language families reveals a universal language network. Nat. Neurosci. 22, 1145. doi: 10.1038/s41593-022-01114-5
Maynard Smith, J., and Szathmáry, E. (1995) The Major Transitions in Evolution. Oxford: W. H. Freeman
McCauley, S. M., and Christiansen, M. H. (2017). Computational investigations of multiword chunks in language learning. Topics Cogn. Sci. 9, 637–652. doi: 10.1111/tops.12258
Miller, G. A. (1956). The magical number seven, plus or minus two: some limits on our capacity for processing information. Psychologic. Rev. 63, 81–97 doi: 10.1037/h0043158
Mühlenbernd, R. (2021). Evolutionary stability of ambiguity in context signaling games. Synthese 198, 11725–11753. doi: 10.1007/s11229-020-02826-6
Newman, M. E. (2003). Mixing patterns in networks. Phys. Rev. E67, 026126. doi: 10.1103/PhysRevE.67.026126
Newman, M. E. (2006). Modularity and community structure in networks. Proceed. Nat. Acad. Sci. 103, 8577–8582. doi: 10.1073/pnas.0601602103
Paparistodimou, G., Duffy, A., Whitfield, R. I., Knight, P., and Robb, M. (2020). A network science-based assessment methodology for robust modular system architectures during early conceptual design. J. Eng. Design 31, 179–218. doi: 10.1080/09544828.2019.1686469
Partan, S. R., and Marler, P. (2005). Issues in the classification of multimodal communication signals. Am. Nat. 166, 231–245. doi: 10.1086/431246
Peckre, L., Kappeler, P. M., and Fichtel, C. (2019). Clarifying and expanding the social complexity hypothesis for communicative complexity. Behav. Ecol. Sociobiol. 73, doi: 10.1007/s00265-018-2605-4
Peel, L., Delvenne, J. C., and Lambiotte, R. (2018). Multiscale mixing patterns in networks. Proceedings of the Nat. Acad. Sci. 115, 4057–4062. doi: 10.1073/pnas.1713019115
Peng, P., Cheng, S., Chen, J., Liao, M., Wu,l, Liu, X., et al. (2018). A fine-grained perspective on the robustness of global cargo ship transportation networks. J. Geogr. Sci. 28, 881–889. doi: 10.1007/s11442-018-1511-z
Penn, D. J., and Számadó, S. (2021). Commentary: why are no animal communication systems simple languages?. Front. Psychol. 12, 685. doi: 10.3389/fpsyg.2021.722685
Piantadosi, S. T., Tily, H., and Gibson, E. (2012). The communicative function of ambiguity in language. Cognition 122, 280–291. doi: 10.1016/j.cognition.2011.10.004
Reboul, A. (2015). Why language really is not a communication system: a cognitive view of language evolution. Front. Psychol. 6, 1434. doi: 10.3389/fpsyg.2015.01434
Rebout, N., Lone, J. C., De Marco, A., Cozzolino, R., Lemasson, A., and Thierry, B. (2021). Measuring complexity in organisms and organizations. Roy. Soc. Open Sci. 8, 200895. doi: 10.1098/rsos.200895
Rendall, D., Owren, M. J., and Ryan, M. J. (2009). What do animal signals mean?. Anim. Behav. 78, 233–240. doi: 10.1016/j.anbehav.2009.06.007
Santana, C. (2014). Ambiguity in cooperative signaling. Philo. Sci. 81, 398–422. doi: 10.1086/676652
Schwaighofer, M., Fischer, F., and Bühner, M. (2015). Does working memory training transfer? a meta-analysis including training conditions as moderators. Educ. Psychol. 50, 138–166. doi: 10.1080/00461520.2015.1036274
Scott-Phillips, T. C. (2008). Defining biological communication. J. Evol. Biol. 21, 387–395. doi: 10.1111/j.1420-9101.2007.01497.x
Sewall, K. B. (2015). Social complexity as a driver of communication and cognition. Integrat. Comparat. Biol. 55, 384–395. doi: 10.1093/icb/icv064
Sha, Z., and Panchal, J. H. (2013). Towards the design of complex evolving networks with high robustness and resilience. Procedia Comput. Sci. 16, 522–531. doi: 10.1016/j.procs.2013.01.055
Singer, J. L. (2018). “Mental processes and brain architecture: confronting the complex adaptive systems of human thought (an overview),” in The mind, the brain, and complex adaptive systems, eds M. J. Morowitz and J. L. Singer (Routledge: Abingdon), 1–10.
Sinha, K., and Suh, E. S. (2018). Pareto-optimization of complex system architecture for structural complexity and modularity. Res. Eng. Design 29, 123–141. doi: 10.1007/s00163-017-0260-9
Snowdon, C. T., and Ziegler, T. E. (2021). Contextual complexity of chemical signals in callitrichids. Am. J. Primatol. 83, e23172. doi: 10.1002/ajp.23172
Sporns, O., Tononi, G., and Edelman, G. M. (2000). Connectivity and complexity: the relationship between neuroanatomy and brain dynamics. Neural Netw. 13, 909–922. doi: 10.1016/S0893-6080(00)00053-8
Srinivasan, M., Berner, C., and Rabagliati, H. (2019). Children use polysemy to structure new word meanings. J. Experiment. Psychol. General 148, 926. doi: 10.1037/xge0000454
Stajic, D., and Jansen, L. E. (2021). Empirical evidence for epigenetic inheritance driving evolutionary adaptation. Philosophic. Transact. Roy. Soc. B 376, 0121. doi: 10.1098/rstb.2020.0121
Starr, A., Cirolia, A. J., Tillman, K. A., and Srinivasan, M. (2021). Spatial metaphor facilitates word learning. Child Develop. 92, e329–e342. doi: 10.1111/cdev.13477
Surana, A., Kumara, S., Greaves, M., and Raghavan, U. N. (2005). Supply-chain networks: a complex adaptive systems perspective. Int. J. Product. Res. 43, 4235–4265. doi: 10.1080/00207540500142274
Tal, S., and Arnon, I. (2022). Redundancy can benefit learning: Evidence from word order and case marking. Cognition 224, 105055. doi: 10.1016/j.cognition.2022.105055
Tesfatsion, L. (2003). Agent-based computational economics: modelling economies as complex adaptive systems. Inform. Sci. 149, 262–268. doi: 10.1016/S0020-0255(02)00280-3
Thibodeau, P. H., Matlock, T., and Flusberg, S. J. (2019). The role of metaphor in communication and thought. Lang. Linguist. Compass 13, e12327. doi: 10.1111/lnc3.12327
Tibbetts, E. A., Liu, M., Laub, E. C., and Shen, S. F. (2020). Complex signals alter recognition accuracy and conspecific acceptance thresholds. Philosophic. Transact. Royal Soc. B 375, 20190482. doi: 10.1098/rstb.2019.0482
Tiberio, L., Del Prete, A., Schioppa, T., Sozio, F., Bosisio, D., and Sozzani, S. (2018). Chemokine and chemotactic signals in dendritic cell migration. Cell. Mol. Immunol. 15, 346–352. doi: 10.1038/s41423-018-0005-3
Titz, C., and Karbach, J. (2014). Working memory and executive functions: effects of training on academic achievement. Psychologic. Res. 78, 852–868. doi: 10.1007/s00426-013-0537-1
Tononi, G., Sporns, O., and Edelman, G. M. (1999). Measures of degeneracy and redundancy in biological networks. Proceed. Nat. Acad. Sci. 96, 3257–3262. doi: 10.1073/pnas.96.6.3257
Turnquist, M., and Vugrin, E. (2013). Design for resilience in infrastructure distribution networks. Environ. Syst. Deci. 33, 104–120. doi: 10.1007/s10669-012-9428-z
Vulchanova, M., Milburn, E., Vulchanov, V., and Baggio, G. (2019). Boon or burden? The role of compositional meaning in figurative language processing and acquisition. J. Log. Lang. Inf. 28, 359–387. doi: 10.1007/s10849-019-09282-7
Vulchanova, M., Saldaña, D., Chahboun, S., and Vulchanov, V. (2015). Figurative language processing in atypical populations: the ASD perspective. Front. Hum. Neurosci. 9, 24. doi: 10.3389/fnhum.2015.00024
Wacewicz, S. (2021). Commentary: why are no animal communication systems simple languages?. Front. Psychol. 21:4652. doi: 10.3389/fpsyg.2021.763445
Walker, B. (2020). Resilience: what it is and is not. Ecol. Soc. 25, 211. doi: 10.5751/ES-11647-250211
Wang, F. H., and Mintz, T. H. (2018). Learning nonadjacent dependencies embedded in sentences of an artificial language: when learning breaks down. J. Experiment. Psychol. Learn. Mem. Cogn. 44, 604–614. doi: 10.1037/xlm0000483
Whitacre, J., and Bender, A. (2010). Networked buffering: a basic mechanism for distributed robustness in complex adaptive systems. Theoretic. Biol. Med. Model. 7, 1–20. doi: 10.1186/1742-4682-7-20
Keywords: language acquisition, signals, degeneracy, modularity, robustness, resilience, commonality
Citation: Green K (2023) Identification of commonalities across different languages. Front. Lang. Sci. 2:1172925. doi: 10.3389/flang.2023.1172925
Received: 17 April 2023; Accepted: 18 October 2023;
Published: 20 November 2023.
Edited by:
Guillaume Thierry, Bangor University, United KingdomReviewed by:
William D O'Grady, University of Hawaii at Manoa, United StatesRichard Blythe, University of Edinburgh, United Kingdom
Copyright © 2023 Green. This is an open-access article distributed under the terms of the Creative Commons Attribution License (CC BY). The use, distribution or reproduction in other forums is permitted, provided the original author(s) and the copyright owner(s) are credited and that the original publication in this journal is cited, in accordance with accepted academic practice. No use, distribution or reproduction is permitted which does not comply with these terms.
*Correspondence: Kieran Green, a2llcmFuX2dyZWVuQGhvdG1haWwuY29t