- Nanodelivery Systems and Devices Branch, National Cancer Institute, National Institutes of Health, Bethesda, MD, United States
Despite significant improvements in methodologies behind the development of new anticancer therapies, the path from early-stage drug development through preclinical and clinical development pipeline is still arduous. Further refinement of the current preclinical models and the development of complementing alternative techniques that enable more reliable studies are paramount. The emerging organ-on-a-chip (OoC) technologies are physiological-like organ biomimetic systems built on a microfluidic chip, capable of enabling precise control over various physicochemical and biomechanical parameters and helping recreate the natural physiology and mechanical forces that cells experience in the human body. In oncology research, especially, since cancer has been understood to be a dynamic disease featured by complex interactions between cancer cells and their environment, this technology offers added advantage as it is able to provide a dynamic platform to simulate cancer-on-a-chip emulating the biological context of tumor microenvironment (TME); demonstrating progression to metastases to multiorgans; and helping to unravel complex information that other current in vitro methods are otherwise not able to provide. In this contribution, we provide a biological perspective on the recent advances in the field of OoC models in cancer biology studies from a cancer hallmark perspective. We also discuss current prospects and funding opportunities in this space, as well as a possible future outlook from a biology perspective, including major challenges and new opportunities as a way forward to OoC technologies.
Introduction
Conventional preclinical models for cancer biology studies and anticancer drug development include computational cancer models; in vitro cell models such as 2D cell/monolayers; 3D cell models such as tumor spheroids; and traditional in vivo animal models, while organoids and non-traditional animal models such as patient-derived xenografts (PDXs) and genetically engineered mouse models (GEMMs) constitute the relatively complex contemporary ones. Such models have and continue to provide an invaluable insight into many aspects such as tumor pathogenesis; host tumor interaction; role of tumor microenvironment (TME); and tumor heterogeneity in metastasis. These models are also of great utility in predicting novel cancer markers and targeted therapies, as well as in drug screening and therapeutic discoveries (Sajjad et al., 2021). However, about 90% of drugs under development could not pass the clinical trials, even though they have passed the above-mentioned preclinical model studies (Sun et al., 2022). For many years 2D cell/monolayers and 3D cancer models have been widely employed as initial screening models to provide simple, fast, cost-effective, as well as versatile and easily reproducible method to elucidate the mechanisms of cancer biology or to identify the efficacy and safety of a drug candidate. However, they are unable to offer great translational value as they often fail to recapitulate complex biochemical and biophysical factors of the human TME; the in vivo heterogeneity of human cancer cells; the stromal compartment; as well as the tissue-tissue interfaces, fluid flows and mechanical cues that living cells experience, thus leading to weak predictive ability (Sajjad et al., 2021; Langhans, 2018; Loewa et al., 2023).
Further, current in vitro models are not always suitable for at predicting non-organ specific toxicity; obscure mechanisms; non-linear dose-toxicity relationships; as well as conflicting downstream (side) effects (Lynch et al., 2024; Atkins et al., 2020).
Along the same lines, animal models offer an assortment of opportunities to address a wide variety of scientific questions, including tumor biology and growth, tumor response to drug compounds in vivo, for the study of the pharmacokinetics (PK), pharmacodynamics (PD) and mechanism of action after drug treatment, and to determine if a compound is effective and safe enough to move into the clinic (Ireson et al., 2019). However, besides ethical considerations, many times they fail to predict the human response because of numerous physiological and interspecies metabolic capacity and toxicity-related differences compared to humans, and biological processes not identical to humans. Although animal models often exhibit phenotypes that appear like those of a human disease, they are known to not exactly replicate human physiology or pathophysiology as the underlying molecular and cellular mechanisms can be quite different. Further, limitations such as time-consuming low-throughput in vivo animal research often lead to the extension of drug development life cycle and the increase of development cost. Moreover, in most cases, the results of animal experiments cannot directly verify the toxicity and side effects of drugs in humans due to disease adaptations, differences in physiology and interspecies metabolic capacities. In addition, factors such as the specificity for human target sequences, or usage of immunocompromised/humanized animal models that do not offer the same stroma-tumor interaction as humans, lacking key features of the native tissue microenvironments due to mix human and non-human cells, prevents the efficient translation of novel research to clinical settings. What’s more in the same lines is that conventional preclinical animal models are often discordant with predicting some cancer immunotherapy-mediated adverse events in patients owing to immunological response differences, and newer cancer immunotherapies such as T-cell-engaging bispecific antibody (TCB) therapies that target human-specific antigens lack expression in animals, rendering preclinical animal studies incomprehensible and uninformative for safety and efficacy evaluations (Hegde and Chen, 2020). Similarly, vaccine responses are often not accurately interpretated in animal models, suggesting careful consideration of animal model data for more accurate predictions towards clinical trials (Ireson et al., 2019; Atkins et al., 2020; Horejs, 2021; Loewa et al., 2023). Surveys by several teams demonstrated that the average rate of concordance between animal model findings and clinical trials has been barely touching 9%, and despite proven safety and efficacy in animal model settings, most drugs fail in clinical stages (Herati and Wherry, 2018; Atkins et al., 2020; Loewa et al., 2023). Nonetheless, it is an acknowledged fact that compelling preclinical evidence is a pre-requisite for progression into clinical trials. Development of more reliable and predictable complex human disease models that can offer high clinical biomimicry, and that can additively complement animal studies findings, are urgently needed to help further understand the complex nature of cancer and to develop effective therapeutic anticancer agents. Given the recent passing of the 2022 FDA Modernization Act 2.0 by the United States Congress, pointing towards New Alternative Methods (NAMs) program to reduce animal testing through the development of qualified alternative methods, this landscape of conventional preclinical models is changing and will provide drug sponsors with the capacity to use alternative complex in vitro models, where suitable (FDA, 2023a).
Cancer processes such as tumor initiation, growth, dissemination and metastasis, have been long identified as being biological outcomes of mutations, owing to changes within the neoplastic malignant cell population. In addition to this knowledge, recent evidence suggests extensive involvement of the biological context surrounding these neoplastic cells, such as TME, taking active part in complex interactions with tumor cells leading to histopathological modifications, where TME is a specialized, dynamic, constantly evolving and interactive environment (de Visser and Joyce, 2023). This evolving knowledge calls for the move from the traditional way of studying cancer cells in isolation and to come up with better models that can assist capturing cancer cell interactions with associated TME and extracellular matrix (ECM) changes, for improved pathology and anticancer therapeutics studies. However, reproducing all (or most) of the complex cancerous and non-cancerous cell interactions remains highly challenging as TME possesses human-specific biochemical and biophysical features that are difficult to be representatively recapitulated in typical in vitro and in vivo animal models. The development of alternate and optimal representative platforms that can captivate the complex features of cancer cells can greatly help in the translation of fundamental research findings to clinical applications.
The integrated arrangement of bioengineered or natural 2D or 3D cell/tissue miniature culture systems with microfluidics has resulted in the development of microphysiological system (MPS) platforms called organ-on-a-chip or organ-on-chips (OoC). OoC models help recreate/mimic the complex structure and function of human organs, including the TME, allowing studies on how various parameters, such as cell-cell interactions, biochemical gradients and mechanical forces contribute to cancer development and progression. OoC models can be used to assess how cancer responds to therapy and how therapy affects adjacent organs, including evaluating the effects of drugs on cells collected directly from a patient. OoCs are designed to enable controlled fluid transport and controlled cell microenvironments to maintain tissue-specific functions, to reproducibly create an environment in which 2D/3D cell-culture models can grow, develop, and interact within their own specified microenvironment, as such mimicking (parts of) human organs and tissues, allowing them to evolve as a next-generation experimental platform to study the effect of therapeutics in the body, as well as to understand (patho)physiology (Imparato et al., 2022; Liu et al., 2021; Liu et al., 2022; Zhang et al., 2022; Nolan et al., 2023; Singh et al., 2024). OoC models can recreate cell-cell or cell-ECM interactions, tissue barriers, vascular perfusion, fluid shear forces, spatiotemporal chemical/physical gradients as well as the (hydro)dynamic properties of the microenvironment into a biochemical response. OoCs thus appear in the list of top 10 powerful emerging technologies that offer a possible solution to some of the above-mentioned limitations of current 2D/3D in vitro models, as well as preclinical animal models. The possibility of developing personalized chips similar to that of the patient on which different drugs can be tested in a high-throughput automated manner offers opportunities to identify the correct drug(s) with a minimal amount of patient tissue sample. Today, there exist several OoCs such as lung, liver, skin, gut, brain chips (Imparato et al., 2022; Liu et al., 2021; Liu et al., 2022; Zhang et al., 2022; Nolan et al., 2023; Singh et al., 2024). Cells employed in OoC come from multiple sources, such as animal and human cell lines, primary cells from human donors, human induced pluripotent stem cells (hiPSCs), and PDX tumor biopsies. The advantages and limitations of different cell sources has been reviewed elsewhere (Singh et al., 2024). When it comes to cancer, OoC technology has fortunately enabled researchers to replicate and ideally reproduce several of the key aspects of the in vivo TME, with ECM and specific cells, allowing for the manipulation of the TME for studying cell and molecular behavior under specific/variable metabolic gradients conditions (Strelez et al., 2023). Figure 1 presents the comparative features of preclinical models used in contemporary cancer research and emphasizes the added value that OoC technology brings. Organ-on-a-chip platform is thus projected to bridge the gap between traditional in vitro and in vivo preclinical translation studies, as well as between animal studies and clinical trials for the pharmaceutical industry (Ewart et al., 2022; Casanova et al., 2024; Gil et al., 2023).
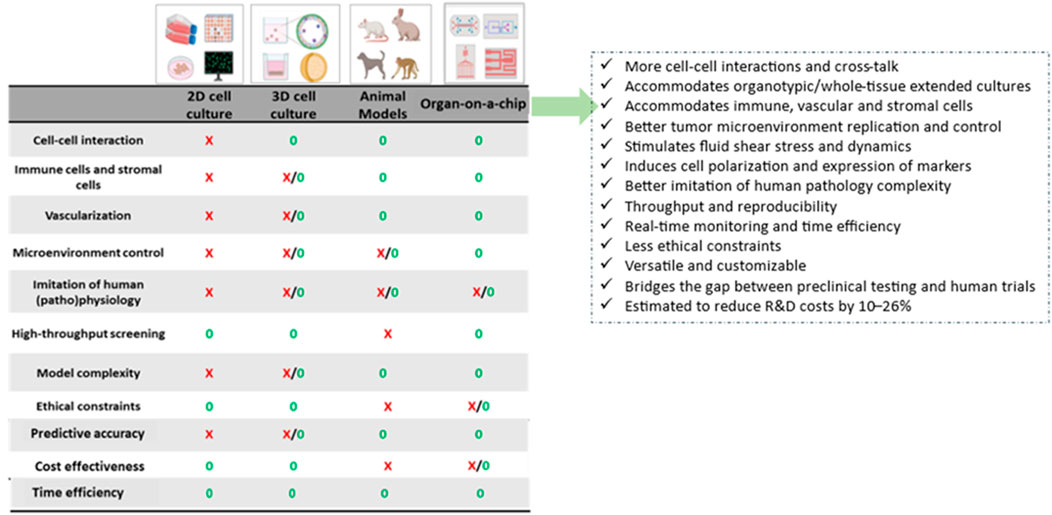
Figure 1. Schematic showing comparative features of currently used preclinical models for cancer research, and the added value that organ-on-a-chip technology can offer (Sokolowska et al., 2022; Li et al., 2023).
To provide a perspective on the current status of OoC research, we performed a pubmed search with key terms “organ-on-a-chip” in Title/Abstract field and discovered that research employing OoC technologies has been increasing sharply (9 papers in 2013 to 213 papers in 2023) in the last decade because of its applicability in human life. As oncology is one of the most important targets of drug discovery, it is in this area that a number of advances in the creation of more (patho)physiologically relevant approaches of OoCs are taking place. OoCs can be classified into multiple types based on design, such as single channel, multi-compartment, template-based model, self-assembly model and the porous membrane chips-based system. The complete chips are typically a few cm in size and made of optically transparent plastic, glass, or flexible polymers. OoCs are typically provided with culture compartments, often separated by a porous membrane, in which customarily 3D tissues, often consisting of several cell types, can be cultured, while microchannels feeding the compartments secure nutrient supply. Some of the biological complexity of organ-on-a-chip culture systems is depicted in Figure 2. OoC takes advantage of the recent development of microfabrication techniques and can be “custom-designed” to better mimic tissue-specific function. By ensuring the right choice of the cell sourcing; the design of materials in the chips; and the introduction of electrodes to deliver electrical/mechanical stimuli, it is possible to recapitulate the tissue-specific microenvironment and control the behavior of cells. At the same time, the microchannels in OoC provide the cells with the necessary nutrients, while removing accumulated waste, and can be precisely engineered to ascertain the 3D tissues with the correct (bio)chemical environment, to mimic the microenvironment as within the body. Measuring parameters and read-out methods can vary among chip types, but the read-outs are commonly based on cell viability and invasive lesion tracking; metabolic activity/physiological barrier integrity/mechanical activity measuring, gradient sensing, staining, and gene expression quantification (Zhu et al., 2021b). For more detailed information on OoC design and manufacturing, as well as on advances in 3D bioprinting, please refer to Leung et al. (2022); Ma et al. (2021); Wu et al. (2024); Gil et al. (2023)).
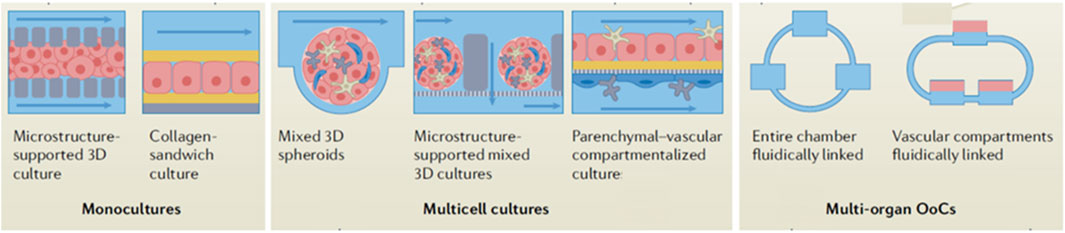
Figure 2. Schematic showing biological complexity of organ-on-a-chip culture systems. Adapted from Leung et al. (2022).
Over the years, several innovative and promising OoC platforms of single organs have been created, but the combination of two or more interconnected organs in the same device allows getting results closer to reality. Therefore, the development of multi-organ-on-chips, combined with advanced 3D culture systems such as spheroids and organoids, are of great utility for evaluating, not only the side effects of particular drugs and drug nanocarriers in other organs, but also the metastasis processes, which can provide venues for the identification of possible targets for intervention (Zuchowska and Skorupska, 2022; Zhu et al., 2023). Multi-OoC platforms are therefore a promising tool for drug screening. They also enable investigating interactions between cancer and other organs related to the biotransformation of the drug, drug accumulation and delivery, as well as direct or indirect effects on other organs in the human body, in addition to studying the adverse effects of drugs in multiple organs. Further, to make OoCs fully functional, the physical parameters of the OoCs environment generated in microfluidic chambers need to be monitored accurately and longitudinally at different time intervals. In this regard, organ-on-a-chip devices offer an appreciable opportunity of integration with imagers, sensors, genomic analyses and/or artificial intelligence (AI) for in situ-and beyond analyses towards cancer research and management. OoCs have engineered physical environments under variable testing conditions where cells are exposed to changes of oxygen levels, pH, nutrient content as well as gradients of secreted metabolites (i.e., glucose and lactate) from adjacent cells (Ferrari et al., 2020). Demand for biocompatible, non-invasive, and continuous real-time monitoring in OoCs has driven the development of a variety of novel sensor approaches. The highest monitoring accuracy and sensitivity can arguably be achieved by integrated biosensing, which enables in situ monitoring of the in vitro microenvironment and dynamic responses of tissues and miniature organs recapitulated in OoCs. Several biosensing methodologies have been tested in OoC devices to monitor the microenvironment (pH, dissolved oxygen, and temperature), cell metabolism and function (metabolic parameters, secreted biomarkers, organ activity, and barrier integrity), and response to external stimuli (electrical, mechanical, and drugs) (Zhu et al., 2021a). Due to their high sensitivity, smooth integration with microfluidics, label-free and non-invasive operation, optical biosensors represent an appealing choice for OoC technology. These sensors pick up changes in optical absorption, illumination, refractive index, or scattering, among other optical properties. Having the ability to retrieve the signal without the sensing device making direct contact with the biological substrate is a crucial benefit of optical sensors, enabling long-term analysis and microenvironmental monitoring without disturbing the cells (Tawade and Mastrangeli, 2024). Electrical and electrochemical sensors have been also finding their way into OoC technology. Direct measurement of transepithelial/transendothelial electrical resistance (TEER) across cell generated barriers is used to assess barrier integrity and transport phenomena. Electrochemistry-based sensors have been used to measure secreted analytes via antigen-, aptamer-, enzyme-, and bead-based binding assays. Recently, also mechanical sensing allowing for the evaluation of cell mechanics have been studied. These methods are designed to measure both cell-generated forces and mechanical properties of cells and tissues (Morales et al., 2022). Anticancer drug response can be evaluated in OoCs using variety of sensors. Optical measurements combined with staining are used to evaluate cell viability, while electrochemical means can assess cellular metabolic activity. Despite the advances in the fields of OoC systems and sensors, efforts to integrate sensors with OoCs are only beginning to emerge. Physical sensors monitoring pH, oxygen, and temperature have been demonstrated in OoCs for real-time monitoring of the cellular microenvironment. In contrast, cell-secreted molecules, used to monitor cellular functionality, are often monitored off-line at single point measurements, which does not allow the dynamic longitudinal characterization of cell behavior (Zhu et al., 2021a).
Further development of OoC functionality combining improving of their biological reliability with methods capable of repeated and robust measures of on-chip cell microenvironments is strongly needed. Both areas of development need to move forward hand-in-hand. The combination of OoC platforms with automation and AI/machine learning (ML) algorithms seems to have a great potential utility in overcoming the complexity and limitations of traditional methods of TME studies and analyses, which can ultimately break the current state of drug evaluation (Deng et al., 2023). This multi-technology approach can help in multiple ways, such as exploiting the identification and delineation of novel TME features; constructing live real-time data collection from human cells; and analyzing/interpreting multiomics data in an objective, reproducible, and efficient manner. The use of OoC technology combined with AI/ML allows for the integration of complex assays and non-invasive real-time high-throughput monitoring via advanced imaging technologies at multiple time points, to offer various advantages, such as to uncover important cellular and extracellular parameters for online drug design, biomarker discovery, drug-target interactions, prediction of therapy response and to modify the drug design on-the-go collectively for the next-generation of drug design and development, ultimately to enrich clinical decision-making (Elmusrati and Ashammakhi, 2018; Deng et al., 2023). The combination of ML models with OoC studies can yield huge quantities of reproducible data, to help maximize value of output information.
Herein, in this review we provide a snapshot on the latest advances in the field of organ-on-a-chip models in cancer biology studies, especially in the context of cancer hallmarks (Hanahan, 2022; Hanahan and Weinberg, 2011). We arrange the sections according to the “Hallmarks of Cancer” integrative concept proposed by Hanahan and Weinberg, which help in fully understanding the mechanisms of cancer development and malignant progression (Figure 3). Embedded in the cancer hallmarks sections we also discuss integration of OoCs with advanced imaging, screening and biosensing technologies, from a biological perspective for future precision medicine applications. Further in the same manner, multi-organ-on-chip models, that are able to replicate the connectivity between the dynamic microenvironment of the tumor and other organs, and that allow for the elucidation of the mechanisms and changes taking place in cancer metastasis, as well as for recognizing novel treatment opportunities, are also briefly discussed. Additionally, we present an analysis of National Institutes of Health (NIH)’s efforts in support of development of OoCs to improve clinical trials’ development and design. We will also pose a possible future outlook, including major challenges and new opportunities for OoC studies. Our snapshot thus focuses on how OoCs create new tool-set to enable discovery research in cancer; allow for improved preclinical drug testing; and enable further development of precision medicine, while at the same time pointing to the challenges that need to be overcome by this newly emerging technology to play its part in improving cancer care.
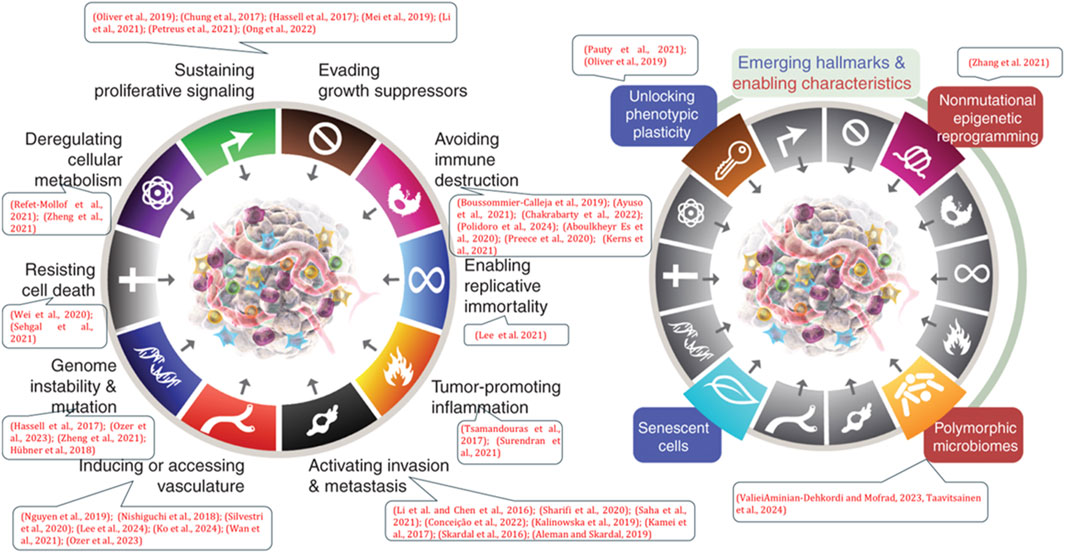
Figure 3. Figure showing the examples of referenced works of organ-on-a-chip (OoC) models (as listed in the dialogue boxes) in the various hallmarks of cancer (cancer mechanisms). Adapted from Hanahan (2022).
Organ-on-a-chip in hallmarks of cancer
Organ-on-a-chip studies focusing on sustaining proliferative signaling and evading growth suppressors
Cancer cells harbor genetic mutations and have the unique ability to reprogram their cellular activities to assist their rapid uncontrolled proliferation and migration as well as to counteract metabolic and genotoxic stress during cancer progression. Altered expression/activity of cell cycle related proteins and constitutive activation of multiple signal transduction pathways also stimulate tumor cell growth followed by alterations in cell metabolism (Feitelson et al., 2015). On the other hand, tumor suppressor genes play an inverse role in cell growth management, normally acting to diminish cell proliferation and tumor development. Cancer cells typically resist inhibitory signals of autophagy and apoptosis, death receptor signaling pathways that might otherwise stop their growth.
TME is a specialized entity which is dynamic, interactive, and constantly changing and recently, the importance and relevance of TME in uncontrolled tumor cell growth/proliferation and metastasis has been increasingly realized due to the histopathological modification observations found at the interface between putative malignant cells and the surrounding non-neoplastic tissues during the development of cancer. It is also being recognized that cancer cells can respond to environmental cues provided by TME and these identifications sustained a drastic shift in the way of conceiving cancer, from a gene-centric to a dynamically interactive disease featured by complex reciprocations between tumor cells and their surrounding environment (Neophytou et al., 2021; Bukhari et al., 2022; Imparato et al., 2022). This new vision of cancer calls for a relative change in the models used to study cancer pathophysiology and assess anticancer therapeutics. Conventional in vitro cultures employing cancer cell lines and tumor spheroids pass over noncancerous parenchymal cells and the surrounding tumor stroma, which is composed of ECM, fibroblasts, immune and endothelial cells and can make up the bulk of the total tumor mass. For, e.g., cancer-associated fibroblasts (CAFs) are abundantly recruited and activated within the tumor stroma by cancer cells and these changes push proliferation, ECM remodeling, neo-angiogenesis, metastatic cascade, and also affect drug resistance mechanism by releasing cytokines and growth factors. Besides, the soluble factors in the interstitial fluid, signaling molecules, cell–ECM, or cell–cell adhesion interactions, niche factors, and mechanical cues (such as ECM stiffness, and ECM composition, fluid strength, interstitial flow and shear stress) trigger bi-directional/multi-directional cell signaling in the TME (Feitelson et al., 2015). Given such cellular/non-cellular and structural complexity within tumor tissues, it has not been easy to reproduce/replicate all the complex tumoral and non-tumoral cell interactions, and hence, the development of optimal surrogate platforms to investigate the complex features of cancer cells, such as migration, proliferation, and chemoresistance has proven very challenging. Unlike in healthy tissue, in many tumors the TME is characterized by increased ECM stiffening and alignment, irregular/leaky vasculature, tumor-related-inflammation, and suppressed-tumor-cell killing (de Visser and Joyce, 2023). Dissecting the role of individual components of the TME for cancer and metastasis studies involving the above features benefits from 2D/3D multicell analysis in a controlled, tumor-type-specific environment, for, e.g., by systematically analyzing the behavior of tumor cells individually or collectively in the form of 3D structures; within an ECM with specific stiffness or morphology; in the presence of other cells such as fibroblasts or immune cells and vasculature; and when exposed to biochemical gradients. Fortunately, OoC platforms have the ability to recreate many cardinal TME features. Several OoC models have tapped into TME in the last years allowing for the recapitulation and manipulation of TME for cancer studies, including those featuring tumor proliferation studies (Ayuso et al., 2021; Sontheimer-Phelps et al., 2019; Giannitelli et al., 2024; Cauli et al., 2023; Seaman et al., 2023).
ECM components embedded in the in vitro microenvironment provide a 3D structural framework to offer biochemical and structural support to the cells. Moreover, ECM plays a role in simulating the development of cancer cells in vitro, playing an important part of the TME. Several OoC studies strived to recreate the ECM on chip to closely resemble natural ECM. Most of the current 3D culture systems use synthetic polymers or selected components of the ECM and are insufficient and defective in reflecting the biophysical and biochemical properties of the native ECM and are far from optimally supporting the signaling cue-oriented cell survival and growth. Rijal et al. developed a reconstitutable tissue matrix scaffold (TMS) system which was fabricated using native tissue ECM with structural and compositional features that promote robust cell proliferation, survival, migration, and invasion in culture (Rijal and Li, 2017). The porous and hydrogel combination in TMS allowed compartmental culture of tumor and stromal cells that can be distinguishable by biomarkers, and drug responses on this platform reflected animal and clinical observations making it a prospectively suitable tool for preclinical drug screening.
Oliver et al. introduced a microfluidic blood-brain niche (μBBN) chip to characterize the proliferative and migratory phenotypes of cancer cells possessing varying degrees of brain metastatic potential as well as tumor cells from cancer patient samples with known metastatic potential (Oliver et al., 2019). Their study provides an example of combination of advanced confocal tomography live cell imaging algorithm and AI with OoC technology. In another work, Chung et al. examined tumor cell-stromal cell interaction with a 3D ECM in their TME OoC platform which consisted of an array of microposts that enabled straightforward micropatterning of the hydrogel and flexible experimental configurations (Chung et al., 2017). They used ovarian adenocarcinoma cells, stomach cancer cells, and colorectal cancer cells as well as normal human lung fibroblasts to investigate both the role of how the ECM composition affects these changes as well as how co-culture with fibroblasts induces morphological changes in cancer cells. Their results demonstrated interactions between cancer cells and fibroblasts in the OoC platform, and that the ECM composition could markedly affect the cellular behavior of cancer cells, including proliferation, in response to these interactions. Fibroblasts were also affected by cancer cells when cocultured, indicating the synergistic effects of fibroblasts and ECM composition on cancer proliferation and in regulating their aggressiveness. One other excellent example of compartmentalized OoC device that may be used to investigate the complex interactions between multiple cancer-associated cell types and ECM within the TME with high resolution is the study by Choi et al. to replicate the early stages of breast cancer (Choi et al., 2015). Their device replicated 3D microarchitecture of multicellular ductal carcinoma in situ (DCIS) lesions in tissue-specific microenvironment of the human mammary duct. The DCIS spheroids were found to successfully adhere to the epithelial cell surface and gradually became flattened and integrated into the epithelium, while their prolonged culture in the OoC device indicated the ability of the model to support proliferation of DCIS cells. The team established a physiological dynamic fluid flow of paclitaxel solution which resulted in the inhibition of the growth of DCIS spheroids, without any toxic effects on normal mammary epithelium. In another study also using a similar compartmentalized chip, Hassell et al. from Ingber’s research group were able to recapitulate TME-specific cancer behaviors, including rapid growth and proliferation in the lung alveolus microenvironment compared to relative tumor dormancy in the lung airways, as well as tumor responses to tyrosine kinase inhibitor therapy previously only observed in vivo (Hassell et al., 2017). They did this by orthotopically injecting a human non-small-cell lung cancer (NSCLC) line within the primary alveolus and small airway organ-on-a-chips. The rapid proliferation of NSCLC cells and therapy response were correlated with physical cues, which appeared to be mediated by changes in specific local microenvironmental signaling factors released by normal lung endothelial and epithelial cells communicating across a porous ECM-coated synthetic membrane. Interestingly, these proliferation changes were not observed under 2D conditions with the same medium demonstrating that OoC studies demonstrate feasibility of engineering in vitro human orthotopic cancer models with clinical relevance, and that they can be resourceful for identifying cues to therapy resistance. Addition of tumor stromal cells or immune cells to such models in the future would offer improved pathophysiological relevance.
Mei and group developed an OoC model to investigate the role of osteocytes in the mechanical regulation of breast cancer bone metastasis (Mei et al., 2019). The device consisted of a simulated blood vessel with 3D lumen structure through which cancer cells can extravasate and generate oscillatory fluid flow through which bone cells can produce physiologically relevant mechanical forces on the cancer cells. The presence of osteocytes and mechanical stimulation were found to enhance cancer cell proliferation, migration, and invasion. OoC models such as this can be used to study other cancer types that typically metastasize to the bone. Further, an interesting study by Li et al. for the first time replicated extremely early-stage (EES) tumor behavior on-a-chip and visually presented how this behavior is affected by TME, in the context of angiogenesis and fibroblasts (Li et al., 2021). The proliferation/progression of a single solid tumor at the EES, was demonstrated using a cervical cancer cell spheroid cultured on the chip surrounded by a microvascular network to reveal not only growth patterns and cell behaviors but also the effect of tumor progression on peritumoral angiogenesis. Smaller tumors were found to be more aggressive, while endotheliocytes and fibroblasts aggravated proliferation and migration of tumor cells. This study also exemplified the dynamic tumor cell epithelial-mesenchymal transition (EMT) process and the formation of vasculogenic mimicry at the EES, exemplifying formation of vessel-like structures without endothelial cells as an alternative pathway for tumor cells to gain nutrients. OoC studies such as these contribute opportunities for new ideas for early-stage tumor therapy. One exemplary tumor-on-a-chip OoC innovative model was developed by Petreus et al. that was able to guide real physiological drug exposure, drug dose and schedules for optimal efficacy and pharmacodynamic assessment (Petreus et al., 2021). Response of colorectal cancer (CRC) spheroids to chemotherapy drug topoisomerase I inhibitor irinotecan alone, and in combination with an ATM inhibitor, were tested using concentrations mimicking mouse plasma exposure profiles of both agents, as the OoC platform allowed for varying drug concentrations typical of a plasma PK profile. The spheroid volume and viability were measured for cancer cell response and changes in mechanistically relevant pharmacodynamic biomarkers were evaluated as well. OoC platforms such as these can aid in successfully predicting the efficacy from in vivo studies while reducing the need for animal studies.
One other great example proof of concept OoC study that led to insights in cancer biology and (human) drug efficacy relevance in the context of sustaining tumor heterogeneity was from Ong et al. (2022). Their group performed side-by-side comparison of the results of PDX animal models and the corresponding paired tumor spheroids on OoCs to evaluate the efficacy response of 5 standard-of-care (SOC) chemotherapies in 3 CRC patients from which the specimens were obtained. The agreement between two methodologies (in vivo animals and in vitro tumor spheroid OoCs) stood from very good to moderate (Pearson’s correlation coefficient 0.40, 0.90 and 0.70 respectively for the 3 PDX samples). This study exemplified that OoC platforms can enable parallel perfusion culture of primary PDX-derived tumor spheroids, in a manner that such tumor spheroids are able to maintain the heterogeneity and patient-specific tumor characteristics of PDX cells, and associated drug responses. Their platform enabled multiple spheroid cultures to be simultaneously and uniformly evaluated as an array for drug efficacy testing, and this is an example of a first of a kind study showing a comparative framework to quantitatively correlate the drug response predictions made by a microfluidic OoC model against that of matched PDX models in a patient-specific manner. Future improvements in such platforms can include accommodation of tumor’s native ECM for better reproducibility and drug efficacy predictability, and incorporation of immune effector cells such as natural killer (NK) and CAR-T-cells for immunotherapy efficacy testing.
Organ-on-a-chip studies focusing on inducing or accessing vasculature
Tumor vasculature is very different from that supplying the healthy tissues, and neovascularization and angiogenesis play a crucial role during cancer progression and tumor maintenance (de Visser and Joyce, 2023; Neophytou et al., 2021). In TME, pathological disbalance between pro- and anti-angiogenic factors trigger endothelial cells to sprout and initiate angiogenesis in a process called the “angiogenic switch”. This angiogenic switch contributes to malignant progression of benign tumors by releasing tumors from dormancy and sparking rapid growth of malignant cells in association with new blood vessel formation. Establishing successfully vascularized functional tumor in vitro models has always been a major challenge. However, during the last years several OoC models demonstrating tumor vasculature/angiogenesis have been successfully developed providing valuable information on supply of oxygen, nutrients, and therapeutics, as well as clearing metabolic wastes. Vasculature also provides an important route for tumor metastasis and several vascularized OoC models have been customized to elucidate the mechanisms of the cancer metastasis cascade. Such vascularized OoC models also provide useful tools to improve engineered immune cell trafficking or drug delivery to the tumor. The designs can be matched to the 96- or 384-well format and integrated with high-throughput machinery, making it an attractive tool for therapeutic testing. For complete details on advances in vascularized OoC models, please refer to Huang and Tu (2023); Wang et al. (2022); Kim et al. (2017).
Nguyen et al., demonstrated the use of organotypic endothelium-lined channels as a model to recapitulate angiogenic sprouting in vitro (Nguyen et al., 2013). The system allowed them to screen combinations of pro- and antiangiogenic agents and identify cocktails that reconstitute or alter the morphogenetic steps of angiogenic invasion into a surrounding ECM, mimicking key morphological aspects of in vivo angiogenesis that are not depicted by other in vitro models. The same group later studied the interaction between biomimetic pancreatic ductal adenocarcinoma (PDAC) cells and a blood vessel positioned 500 µm apart, emulating vascular invasion and tumor-blood vessel interaction (Nguyen et al., 2019). PDAC cells were observed to migrate as a group toward blood vessels and induce contact-dependent endothelial cell apoptosis and this phenomenon was found to be mediated by activin and its receptor-like kinase 7 (ALK7) expressed by PDAC cells. Their findings were validated and corroborated in GEMMs as well as mouse ectopic tumor model, also explaining why despite high vascular invasiveness PDAC tissue is hypo-vascularized and why the high circulating tumor cells (CTCs) load in these patients. Since pancreatic cancers are highly invasive solid tumors with hypovascular structures and dense fibrosis, developing advanced pancreatic cancer-on-a-chip systems suitable for in vitro experiments is highly beneficial for enhancing pancreatic drug screening efficiency prior to animal model testing and clinical trials. Nishiguchi et al. built a 3D human stromal pancreatic cancer model employing a specialized layer-by-layer technique with an integrated blood capillary structure, to better mimic the TME and study metastasis progression (Nishiguchi et al., 2018). This in vitro 3D layer-by-layer model finds itself to be useful in representing the death of endothelial cells during metastasis and assessing the efficacies of different drugs against varieties of pancreatic cancer cells, targeting the hematogenous metastases or the lymphogenous metastases. Such OoC platforms can offer powerful tools for predicting cancer cell responses and time-lapse-throughput screening of secreted molecules. Another tissue-engineered model study using mouse mammary tumor organoids cocultured with physiologically realistic microvessel showcased the formation of endothelial cell +tumor cell-mosaic vessels following the cell interaction (Silvestri et al., 2020). Real-time imaging and quantitative assessment of these tumor-vessel interactions nicely demonstrated the detachment of cancer cells from the fused organoids traversing to the vascular lumen and constricting or displacing the vessel causing vascular defect. Such models enable future investigations into targetable mechanisms of intravasation and vascular recruitment.
More recently, Lee et al. developed an angiogenesis-on-a-chip platform coupled with single-cell RNA sequencing to study the effects of anti-cancer drugs resulting in an anti-angiogenic effect accompanied by activation of autophagy (Lee et al., 2024). This model recapitulated human 3D angiogenic sprouting containing a co-culture of human umbilical vein endothelial cells (HUVECs) and fibroblasts, where in the fibroblasts release pro-angiogenic factors to activate the HUVECs to cause angiogenic sprouting. Single-cell RNA sequencing was performed to determine the heterogeneity of autophagy in endothelial cells along elongating sprouts. Such models can help improvise strategies for developing autophagy-based anti-angiogenic therapeutics. Also recently, Ko et al. developed an innovative patient-derived tumor spheroid (PDTS) preclinical model obtained from gastric cancer clinical samples, including ascites and primary tumor specimens (Ko et al., 2024). This model reconstructs the TME and is particularly driven towards angiogenesis enabling one to examine unique patient-specific angiogenesis patterns, facilitating the exploration of therapeutic vulnerabilities. The high-throughput testing system allows close monitoring of the micro changes in both angiogenesis and tumor proliferation, which can assist in the rapid evaluation of combination drug therapy efficacy focusing on the interplay between cancer and the vascular system and making precise quantitative assessments. Furthermore, Chung et al.’s OoC model mentioned earlier in “sustaining proliferation” section allows direct observation of angiogenesis induced by the tumor-stroma interaction, and as well enables reconstituting simultaneous angiogenesis and lymphangiogenesis induced by such an interaction with TME mimicking extrinsic factors (Chung et al., 2017). With a slight difference to this, Wan et al. developed a novel approach of 3D collagen endothelial vasculature-mimetic tumor-on-a-chip for dynamic combinatorial drug screening (Wan et al., 2021). This system mimics drug transport through the ECM and tumor vasculature and was employed to study viability of tumor pieces and dynamic drug diffusion profiles compared to traditional in vitro 2D methods in response to three different drug combinations. Their 3D ECM OoC produced data that was reliable on viability, combination indeces and drug ratios, enabling higher throughput and better drug sensitivity prediction algorithms.
One other recent example of an interesting effort driving specific biological discoveries in cancer research is from Ozer et al., who devised a cancer metastasis-on-a-chip assay for high-throughput drug screening that included GFP-labelled triple-negative breast cancer (TNBC) cells, stromal fibroblasts mixed coculture on one side and a perfused microvessel on the other, separated by collagen gel representing ECM in the middle (Ozer et al., 2024). This system accommodated high-content imaging with automated quantification methods that allowed testing of a vast targeted anti-cancer therapy drug library and evaluating cancer cells propagating into collagen area and eventually intravasating into the formed microvessel. One of the important findings was with drugs Imatinib (tyrosine kinase inhibitor), which, compared to the control, was found to reduce cancer cell intravasation into the formed microvessel, owing to its identified effects on enhancing endothelial barrier stability and significantly reducing the trans-endothelial invasion activity of tumor cells. OoC systems such as this offer powerful tools for high content screening of targeted anti-cancer therapies as well as for studying cancer metastasis biological mechanisms, which traditional preclinical platforms are otherwise not able to model.
Organ-on-a-chip studies focusing on deregulating cellular energetics/metabolism and modeling tumor hypoxia
Changes in oxygen levels are commonly prevalent in cancerous tissue due to the irregularities or abnormalities in vascular supply, which may lead to normoxia, limited hypoxia, or even almost complete hypoxia in some areas. 50%–60% of solid tumors may show hypoxic regions, and lack of oxygen supply has long been recognized as one of the main causes of metabolism-related cellular (mis)adaptations and phenotypic shifts leading to therapy resistance, changes in proliferation, metastasis stimulation and ultimately poor prognosis. This explains why mimicking the hypoxia situation accurately similar to in vivo situation by artificial induction of hypoxia in in vitro systems becomes complex, implying the challenges in controlling oxygen tension and mimicking changes in cellular phenotype in the in vitro tumor model design. Thus, so far, no user-friendly traditional in vitro preclinical tools are available to study natural chronic hypoxia. Refet-Mollof et al. developed a hypoxic jumbo spheroids on-a-chip (HOnAChip) for easy culture and analysis of naturally hypoxic sarcoma spheroids (Refet-Mollof et al., 2021). The response of oxygen-dependent combined radiotherapy and the hypoxic prodrug tirapazine (TPZ) were investigated, to find out that TPZ preferentially targets the jumbo spheroids’ hypoxic core, and that that core was found to harbor just as much DNA damage 24 h after irradiation as normoxic spheroid cells. This model demonstrated itself as a powerful preclinical tool to study hypoxia and how it impacts the therapeutic efficacy response. Zheng et al. developed a very resourceful oxygen-controllable 3D-culture multiorgan microfluidic (CMOM) model providing a platform for exploring the hypoxia-induced tumor metastasis mechanism in lung cancer liver metastasis, and for studying hypoxia-related targeted anticancer drug effect (Zheng et al., 2021). The platform consisted of a multiorgan level lung cancer and liver linkage model that precisely controls dissolved oxygen concentration wherein the effects of cancer cell fibroblast cocultures in cancer metastasis were analyzed by transcriptomics and protein expression detection, followed by treatment effects of hypoxia-induced factor (HIF)-1α inhibitors. A deeper characterization and understanding of the multilayered structure of the 3D cancer cells with emphasis on the presence of quiescent cell, senescent, and/or necrotic cells and gene expression profiling of hypoxia-responsive element genes, and of the hypoxia OoC models as exemplified above would offer great application potential for screening hypoxia-related target anticancer drugs and patient-stratification studies.
Organ-on-a-chip studies focusing on avoiding immune destruction
Immune system constitutes an important part of the TME playing a major role in the growth and metastasis of cancer. More in this direction, the recent surge of focus on cancer immunotherapies urges the incorporation on OoC studies modelling the host immunological setting and the dynamic tumor-immune cell interaction. 3D tumor-chip models mimicking spatial aspects of tumor architecture so far nicely demonstrated immunosuppressive microenvironment with profound effects on immune cell exhaustion. As a step up, the dawn of immunocompetent cancer-on-a-chip models has been adding immense scope to assess immuno-oncology therapy. Such models allow for translation to human immunity and an ability to predict clinical efficacy and safety outcomes. For more detailed information on these aspects, please refer to Seaman et al. (2023); Sun et al. (2019); Mattei et al. (2021); Maulana et al. (2021).
Boussomier-Calleja et al. quantified the variable effects of monocyte differentiation on tumor cell extravasation and migration in an all-human OoC model, and such knowledge can help in developing new cancer immunotherapies targeting various immune cell types across the several stages of immune cell differentiation and metastasis (Boussommier-Calleja et al., 2019). This is also an example of a model that allowed for high spatiotemporal resolution imaging of monocyte transmigration. Ayuso et al. developed a tumor-OoC model that contained an endothelial cell-patterned channel to mimic a functioning vascular tube, while hydrogel was used to construct metabolite gradients and nutrition. Their study illustrated the play of tumor environmental stress on NK cell exhaustion by demonstrating that NK cells from the OoC device exhibited erosion of their cytotoxic capacity (indicating NK cell exhaustion), which even persisted for an extended period of time after removal of the NK cells from the OoC platform. Treatment with checkpoint inhibitors and immunomodulatory agents partially alleviated the NK cell exhaustion phenomenon in the OoC, wherein tumor cells in the necrotic region were identified to be distinctly resistant to treatment. Such platforms not only allow for the identification of specific molecular mechanisms driving immunosuppression, as well as the adaptation of immune cells to such suppressive environment in a physiologically relevant platform, and also additionally highlight the spatial dependence on cancer cell response to immunotherapies.
As an advanced effort to probe intrinsic chemotherapeutic sensitivity and resistance of tumors directly, Chakrabarty et al. developed a multi-sample parallel cancer-tissue-on-a-chip (CoC) platform to assess cisplatin and apalutamide therapy response in breast and prostate tumor xenograft models, respectively (Chakrabarty et al., 2022). Their OoC system accommodated ex vivo organotypic whole tumor slice culture that can be extended for up to 14 days, wherein the tissue slices specifically retained all tumor cells, including immune cells, while maintaining tissue architecture, and allowing for investigation of chemotherapy responses, as well as for future detailed mechanistic studies, under precisely controlled conditions. Such platforms allow for investigation into therapy responses, such as therapy resistance development or clonal outgrowths, wherein these processes require extended incubation times (beyond 1 week). In a more research effort Polidoro et al. developed a human 3D cholangiocarcinoma-on-a-chip (CCA) platform, that was able to integrate the major non-immune components of the TME and the T-cell infiltrate, thus reflecting the in vivo CCA niche (that is otherwise not possible to be replicated by traditional in vitro setups), and replicating tumor drug response (Polidoro et al., 2024). Such platforms can provide substantial benefit in understanding the so far less-understood mechanism by which some cancers such as CCA act at the cellular level and to improve patient-specific therapies and overcome drug resistance in such cancer diseases. Mi et al. devised a novel OoC platform that can be used for real-time invasion studies in the context of bidirectional breast tumor-macrophage crosstalk (Mi et al., 2019). This OoC model exhibited a side-by-side 3D ECM arrangement with a blood vessel monolayer mimicking the in vivo transendothelial transport, where in the authors analyzed the real-time relationship between breast cancer cells and macrophages, including tumor-associated macrophages (TAMs), to find out that invasive breast cancer cells that were co-cultured with TAMs resulted in significantly higher cancer cell migration distance compared to those co-cultured with normal macrophages. Moreover, effect of drugs such as paclitaxel on the viability of breast cancer cells was the highest when co-cultured with TAMs, suggesting that resistance to anti-cancer therapies may owe to transformed immune cells. Such OoC models exemplify the antagonism of tumor–macrophage bidirectional crosstalk on anticancer drugs. Regarding targeting cytokines, Es et al. examined the effects of a fibrosis-inhibiting drug named pirfenidone (PFD), on CAFs in a breast cancer OoC model (Aboulkheyr Es et al., 2020). Their findings demonstrated that PFD inhibits the production of immunosuppressive cytokines, causing a reduction in both CAF and cancer cell migration and invasiveness. Preece et al. used a three-lane liver cancer-on-a-chip model to evaluate the efficacy of TCR-engineered T lymphocytes against hepatoma cells (Preece et al., 2020). Their findings demonstrated a promising potential of these altered T-cells as implicated by an upsurge in the production of cytokines and the ability of these modified T-cells to destroy tumor cells. Kerns et al. investigated immune response to foreign cells and confirmed the validity of human immunocompetent lung- and intestine-on-a-chip models for evaluating the on-target, off-tumor safety liabilities of target-specific T-cell bispecific antibodies (Kerns et al., 2021). Their OoC studies, when compared with in vivo in animal models, accurately predicted the optimal time frames for effectively destroying cancer cells while minimizing injury to healthy tissues. To address the issue of alloreactivity, they employed period and components that reduce this danger, however, future studies should ensure compatibility between human leukocyte antigen/major histocompatibility complex (HLA/MHC) or use autologous cells produced by the patient. As a very recent development in this context, Veith and Nurmik et al. presented an OoC approach to evaluate personalized responses to anti-protein programmed death 1 (PD-1) employing autologous patient-derived primary cells from lung cancers and automated cell-tracking (Veith et al., 2024). At the same time, they were also able to recapitulate stroma-dependent resistance mechanisms, opening avenues and increasing scope for both fundamental and translational research in immuno-oncology. In order to further validate the predictive power of such platforms, larger patient cohorts and biopsy-compatible chips, as well as direct comparison between OoC and in vivo data are imperative. A few more OoC systems have been used for drug/therapy investigations in immunooncology and reviewed in detail by Mattei et al. (2021); Chernyavska et al. (2023). As a future direction, incorporation of immunosuppressive immune cell types such as Tregs and myeloid-derived suppressor cells, as well as region-specific resident cell types could help unravel the immunomodulatory role that these cells play in cancer within that specific region (Kerns et al., 2021; Maulana et al., 2021).
Recapitulation of an anti-tumoral antibody-dependent cell-mediated cytotoxicity was made possible through a tumor immune-OoC platform designed by Ngyuen et al. consisting of 3D cancer, immune, endothelial cells, and fibroblasts (Nguyen et al., 2018). This chip design made possible to reconstitute an ex vivo human immunocompetent tumor ecosystem of HER2+ breast cancer and to track cancer-immune cell interactions using a deep learning (DL)-based CellHunter method. More recently, other studies by Mencattini et al. employed the CellHunter algorithm to high-resolution video analysis to localize and track cancer cell-immune interactions, as well as to measure immune kinematics (Mencattini et al., 2020; Mencattini et al., 2022). This study revealed cooperative antitumoral activity of immune cells and an emerging immunotherapy agent oncolytic vaccinia virus by direct imaging and automatic analysis. Several such analytical tools integrated with immunoonco chips have been emerging to study the cancer cell-immune cell interactions in OoC platforms, which help track immune cell migratory (toward or outward) behavior toward cancer cells and also to capture the ability of the immune cell to “sense” cancer cells inside the device upon drug exposure (Mattei et al., 2021). Other ML tools such as Generative Adversarial Networks (GANs), along with the integrated automated tracking plugins and image-assisted analysis, have also been evolving to evaluate cancer cell-immune cell interactions and to generate an in silico OoC simulation prior to optimizing OoC experiments reducing effort, time and experimental costs. Following the training procedure called “Input Tracks”, GANs are able to beneficially predict cell trajectories from the real-cell tracking captured by a time-lapse studies, even of poorly identifiable immune cells. Tracking of trajectories relative to immune cell motion in OoCs can recapitulate the cancer and immune cell interactions in the TME and assist in exploiting hidden data that is not readily accessible by traditional in vitro or in vivo TME methodologies.
Organ-on-a-chip studies focusing on unlocking phenotypic plasticity
Phenotypic plasticity of somatic cells and switch from hierarchy to plasticity relates directly to the origination of cancer and to cancer progression and resistance to therapy response. Shin et al. reported about an in vitro breast tumor OoC model with ECM scaffolds that mimics intratumoral phenotypic heterogeneity and demonstrated that this phenomenon encourages cancer invasion (Shin et al., 2014). In this system they co-cultured two breast cancer cell types with distinct phenotypes, specifically, highly invasive breast cancer cells with a capacity of proteolytic ECM remodeling (MDA-MB-231), and epithelial-like cancer cells (MCF-7) possessing a non-aggressive low-invasive phenotype with strong cell-cell junctions. Their results showed the ability of cells of more invasive phenotype to promote the ones with less invasive potential in the heterogeneous tumor mass was strongly dependent on the ECM type. Pauty and colleagues investigated senescence-associated secretory phenotype-induced angiogenesis and drug responses in a 3D tissue model-on-a-chip (Pauty et al., 2021). In a study conducted by Oliver and colleagues, as mentioned above in the proliferation section, a microfluidic blood-brain niche coupled with confocal tomography for live-cell 3D imaging and ML algorithms were combinedly employed to predict the metastatic potential of aggressive TNBC cell lines and PDX across the blood brain barrier (BBB) (Oliver et al., 2019). This low cost and quick turnaround time platform uniquely, in combination with AI, aided in distinctively discerning the metastatic signatures of cancer subclones, such as the migratory and proliferative phenotypes, that are too subtle for manual identification. The cytoskeletal plasticity observed in the cells enabled them to assume specific shape(s) during extravasation and colonization. Such studies aid quicker and more accurate clinical decisions, while future studies will need to better recapitulate the BBB in the OoC devices through the introduction of fluid flow and additional cell types.
Organ-on-a-chip studies focusing on nonmutational epigenetic reprogramming
Carcinogenesis involves a complex process in which normal cells undergo genetic and epigenetic modifications/abberations leading to the development of cancer. OoC studies addressing epigenetic reprogramming have not been pursued to date. Zhang and colleagues expanded CTCs extracted from early-stage lung cancer patients along with fibroblasts on a co-culture chip (Zhang et al., 2014). Next-generation sequencing of 124 onco-related genes revealed matched mutations between the primary tumor and cultured CTCs in 3/8 paired-CTC-tumor samples. Such studies indicate potential of combination of OoC with next-generation sequencing to help uncover the genetic and epigenetic underpinnings of cancer, which are the putative drivers of metastasis, and for redefining treatment paradigms.
Organ-on-a-chip studies focusing on enabling replicative immortality
It is a well-known and accepted fact that cell lines are not completely representative models of in vivo tumors, since indeterminate phenotypic, transcriptomic, genetic and epigenetic changes may keep occurring during the process of cell immortalization. In contrast to cell lines, alternative sources such as iPSCs achieve immortality by inducing pluripotency rather than by transformation. For OoC development, iPSCs and iPSCs-derived organoids offer an unprecedented opportunity to conduct patient-specific treatment studies centering patient diversity. Lee et al. introduced a heart-breast cancer-on-a-chip (CoC) platform to monitor cancer chemotherapy-induced cardiotoxicity (CIC) (Lee et al., 2020). This platform is versatile in that it accommodated a nanoparticle-based doxorubicin (DOX)-delivery system, and such platforms show promise in early detection and prediction of CIC in susceptible patients in the future. Future opportunities of iPSCs in immune research include the generation of agnostic tissues using a common HLA-null iPSC line and combining with the patient’s immune cells to offer renewable sources of both the innate and the adaptive immune cells and fill the gap to access functional immune cells for immunooncology studies (Leung et al., 2022). iPSCs offer the ability to be personalized from patient-specific donor cells and to be reprogrammed into different types of tissues, but technical issues, such as (in)efficiency of iPSCs to be reprogrammed into malignant cells, limit the possibility of routine integration of iPSC-derived organoids with OoCs.
Organ-on-a-chip studies focusing on resisting cell death
Cancer cells undergo reprogramming and phenotypic changes that allow them evade apoptosis as well as the immune system attack long enough to grow into a tumor. Nanoparticles are gaining more and more attraction in medicine, especially for cancer, because their surfaces can be chemically manipulated for targeting specific disease cells. Availability of an in vivo-like reproducible platform is crucial and beneficial to evaluate the biological behaviors of nanoparticles, more so in the contexts such as therapy resistant cells that evade cell killing. Wei et al. developed an OoC device that can culture 2 cell lines in parallel in in vivo-like fluidic microenvironments that is dynamic, uniform and stable (Wei et al., 2020). This device was used for testing the tumor targeting of nanoparticles such as folic acid - cholesterol - chitosan (FACC) and the results were found to be comparable with in vivo imaging of HeLa cells grown in nude mice models. Further, a 384-well format multiplexed hanging drop culture plate of spheroids was used by Tung et al. to compare the anti-cancer effect of different drugs on prostate cancer cells and to provide biological insights that are often missed in 2D platforms. They showed that drugs with different modes of action produce distinct responses in the physiological 3D spheroids compared to traditional 2D cell monolayers. 3D-cultured cells were found to accumulate and be more resistant to proliferation inhibitor 5-fluorouracil, whereas 2D cultures were more resistant to tirapazamine, which tends to be toxic in hypoxic conditions (Tung et al., 2011). For detailed information on OoC models for cancer efficacy and toxicity and therapy resistance studies, and those recapitulating physiological cues of TME for nanomedicine applications, please refer to Oh et al. (2021); Stavrou et al. (2023); Kang et al. (2021); Chen et al. (2021); Tian et al. (2022). Sehgal et al. demonstrated the efficacy of an anti-PD1 antibody and targeted inflammatory cytokines in collagen-embedded murine colon cancer spheroids generated from ex vivo tissue. Using a commercial colon cancer sphero-chip compatible with immunofluorescence staining, extraction of cellular material for RNA analysis, and manual exchange of media, Sehgal and team identified a subpopulation of immunotherapy-resistant cells that avoided T-cell-mediated killing (Sehgal et al., 2020). They also demonstrated the effectiveness of PD-1 blockade combined with inhibitor of apoptosis members such as Birc 2/3 antagonism to enhance cancer cell elimination.
Organ-on-a-chip studies focusing on activating invasion and metastasis
Patients with metastatic tumors are often unresponsive to existing therapies, and consequently, metastatic cancer is responsible for about 90% of cancer deaths in the United States each year. Once malignant cells acquire the capability to disseminate and penetrate the adjacent tissues, the competent and motile cells pierce the basement membrane and ECM; intravasate through the vasculature or lymphatics instigating the process of invasion into the vessels; followed by extravasation from the circulatory system where malignant cells adhere at the new location to colonize/proliferate and produce the (pre)metastatic niche and secondary tumor(s) (Jiang et al., 2015). Metastasis progression involves several discrete cellular processes where the specifics of actual trigger(s) of metastatic process are not well unknown. This emphasizes the need for development of a new generation of highly predictive cancer models with advanced capabilities, and therefore, the development of OoC models comes to use for its capability to replicate the TME and to study the activation factors in the TME that lead to metastasis. OoC models of cancer metastasis provide a unique biomimetic environment to recapitulate the metastatic events, as well those of the metastatic niche, and can be used to better elucidate the behavior of new drugs in the context of vasculature and immune support. OoCs also allow for the replication of the patho-physiological separation existing between cancer mass and stroma allowing to perform advanced invasion assays to see how cancer cells cross the barrier and interact with the stromal population. Research efforts concentrating on developing better in vitro platforms as OoCs for identifying and understanding the mechanisms concerned in tumor metastasis cascade have so far been stemming, striving to lead to treatments limiting tumor progression and, as a result, to a reduction in mortality for many cancer patients (Jouybar et al., 2024). OoC progress in immune system involvement and immune barriers were discussed elsewhere in “avoiding immune destruction”, while angiogenesis and lymphangiogenesis were discussed in “accessing vasculature” sections above. In this section, we attempt to summarize some of the recent progress in OoCs in the areas of metastasis such as cell heterogeneity, extracellular stimulator(s) as well as other signaling mechanisms, cell adhesion, crosstalk between cells, and organ specific cancer metastasis and metastatic foci.
Tumor heterogeneity and metastatic dissemination pose a positive link between each other, which dictate invasion patterns identified in vivo, where in a small proportion of stronger cells are able to lead the escape into the surrounding stroma by migrating directionally toward the blood vessel, while the rest of the cells provide coordination efforts (Hallou et al., 2017). This subpopulation of cells is indeed crucial to understand the mechanism at work of metastasis and to develop novel therapeutic strategies targeting this subpopulation of cells. Screening of such highly metastatic heterogeneous malignant cells involved in tumor invasion was made possible by Chen et al. in the OoC platform which worked via differential resolution of cell invasiveness (Chen et al., 2016). Using the Petri dish-based liquid supply pattern, a long-term malignant cell invasion driving force was created based on a chemotactic factor gradient, and the invasive cells were collected for round-by-round selection. The human gastric cancer SGC-7901 cell line was exploited to establish an SGC-7901/B2 subline, which was superior to the parental cells in proliferation and invasiveness, as well as validated in mouse model for in vivo tumorigenicity. Additionally, the protein expression differences such as E-cadherin and Smad3 between the highly metastatic subline and the parental cells were unraveled. Such OoC systems could serve as a potential base model for further exploring tumor cell heterogeneity and aggressiveness in tumor metastasis research. Incorporation of stromal cells to assess the cross-talk between cells, as well as of endothelial cells and immune cells, may provide additional information on the migration capabilities of the different cell lines and the penetration capability.
Further, Lei et al. set out to assess the correlation between nasopharyngeal carcinoma cell invasiveness and extracellular stimulation, which is a critical step for the inhabitation of metastatic dissemination (Lei et al., 2016). To overcome some of the limitations of conventional cell invasion Boyden chamber assays, such as the pore size of the membrane and gravity, Lei and team used a microfluidic device containing two reservoirs connected by a microchannel filled with methyl cellulose hydrogel mimicking ECM. IL-6 was added as an extracellular stimulator to stimulate promotion of migration of cells toward the channel containing the measuring electrode. The entire system was made to locate on top of impedance electrodes to allow impedance measurement and real-time quantitative measurement of cancer cell invasion. This work provides a good example of a non-invasive method to quantify cell invasion by associating the impedance magnitude with cell density. Adding capacitive-based sensors to assess the degradation of the ECM and its impact on the impedance variations will add value to such devices. More so, Sharifi et al. described an in vivo imaging platform for modeling and tracking hepatocellular carcinoma (HCC) - bone metastasis and for analyzing the inhibitory effect of a herb-based compound thymoquinone (TQ) on the this process (Sharifi et al., 2020). The bioreactor design consisted of two chambers, one containing encapsulated HepG2 cells and another bone-mimetic niche with hydroxyapatite (HAp). A microporous membrane was placed above these chambers to mimic the vascular barrier, where medium was circulated over the membrane. Their work showed that cancer cells in their primary chamber sense calcium ions released from the chamber containing the bone module and then begin to migrate across the vascular barrier to settle in a secondary site. The presence of HAp in the hydrogel enhanced metastatic cells migration to the bone compartment, and TQ (more so when encapsulated with nanoparticles) caused a longer period of inhibitory effect on HCC metastasis. Such platforms enable understanding of the key aspects of cancer metastasis-associated biology as well as for improved anti-metastatic drug screening.
One other interesting example of advancement in this direction is an ovarian TME OoC (OTME-chip) engineered by Saha et al. which incorporates tumors interfacing platelet-perfused vascular endothelial channel alongside collagen-based ECM microenvironment (Saha et al., 2021). This design facilitates precise visualization of the dynamics of cancer cell invasion as a consequence of the biological and biophysical effects of platelet extravasation through the endothelial tissue into the TME. A good advantage that this OTME-chip offers over the traditional in vitro and in vivo models is that it allows the complete recapitulation of tumor cell-platelet interaction in ovarian cancer metastasis. The OTME-chip design permits time-lapse isolation of cancer cells depending on the degree of platelet perfusion thus allowing to study the platelet-mediated alteration mechanisms, such as of glycoprotein Ⅵ and tumor gelactin-3, in cancer cell cycle and proliferation. More importantly, as proof of principle of a clinical trial, Revecept (an anthrombotic inhibitor) was tested for impairment of metastatic potential and improvisation of chemotherapy. Such OoC studies provide good example towards aiding the discovery of novel antiplatelet therapeutics that target tumor metastasis and chemoresistance, as well as show how integration of such next-generation sequencing and gene editing tools have the potential to level up OoC technology.
With progress in biomedical technologies, multiple efforts have being ongoing to create relevant multi-organ-on-chip models, i.e., the combination of various organs in one single complex microfluidic OoC, for cancer and metastasis studies (Zuchowska and Skorupska, 2022; Zhu et al., 2023). Based on recent knowledge that the sympathetic nervous system can modulate the breast cancer - bone metastasis, and to explore treatment strategies in this direction, Conceicao et al. developed a 3D bioprinting-based human metastasis-on-a-chip to recapitulate neuro-breast cancer crosstalk in a bone metastatic context (Conceição et al., 2022). The platform comprised three compartments representing the breast cancer organoids embedded in Matrigel, bone osteoclasts were seeded in bone slices and neuronal cells seeded in the compartment, to demonstrate the selective multicellular crosstalk in combination with microscopic, biochemical and proteomic profiling. In addition to static diffusion to induce bidirectional communication, their platform also allowed the manipulation of intercompartmental communication through the use of incorporated pressure actuating valves. Synergistic paracrine signaling from sympathetic neurons and osteoclasts tended to increase breast cancer aggressiveness as demonstrated by augmented levels of pro-inflammatory cytokines IL-6 and MIF-1α. Advanced microfluidic OoC chips such as this holds potential to assess new intracellular communication mechanisms involved at the metastatic niche.
Kalinowska et al. employed a microfluidic multi-organ microsystem chip to test the effectiveness of photothermal therapy (PTT) on 3D multicellular spheroid tumor model using aptamer-modified nanoshells (Kalinowska et al., 2019). Two strategies of conducting PTT therapy (single or double irradiation) were compared on human breast cancer and human glioblastoma lines alongside normal controls, where double irradiation was found to provide better efficiency of PTT-induced cytotoxicity towards tumor spheroids. The therapy did not seem to affect viability of normal cells to such an extent as tumor cell. Kamei et al. developed an integrated heart/cancer-on-a-chip (iHCC)-using human healthy heart cells and liver cancer cells to recapitulate the side effects of doxorubicin (DOX/DXR) (Kamei et al., 2017). Their device allowed individual cell cultures from different tissues on the same single device with three sets of artificial blood circulation loops, as well as accurate fluid operation and precision fluid flow. As the drug travels through tissues via the circulation loop producing tissue-specific metabolites such as doxorubicinol; (DXRol), modelling of the side effects of these metabolites on heart cells, was made possible. Their study proposes a proof of concept for recapitulation of the cardiotoxic side effects of anti-cancer drugs in vitro using their simple design, and modelling this cannot be achieved using conventional cell culture studies. The circulation loop to connect different tissues is the main advantage of such a system, and thus overtakes conventional cell culture studies that cannot offer this opportunity, and so, this study was reported to be the first of its kind in recapitulating the side effects of a (cancer) drug using in vitro platforms. For more such OoC platforms studies for cancer drug screening and delivery please refer to review by Gonçalves et al. (2022).
Skardal et al. describe a metastasis-on-a-chip system that introduced the feature of tumor foci within host tissue constructs, thus distinguishing between the primary tumors and the distant metastatic foci and results in a powerful investigative and diagnostic tool (Skardal et al., 2016). The system allows real-time tracking of fluorescent colon cancer cells, that upon response to environmental manipulation and drug treatment, migrate from hydrogel-fabricated gut organoid constructs to downstream liver constructs bio-fabricated by encapsulating cells in hyaluronic acid (HA)-based hydrogels within a circulatory fluidic device system. Customization within areas of host cells allowed formation and growth metastatic phenotype of colon carcinoma tumor foci, verified by the losing of membrane-bound adhesion markers, and expressing mesenchymal and proliferative markers. Such metastatic foci eventually disseminated from the intestine construct and entered circulation, subsequently reaching in the liver construct, thus mimicking some of the migratory events observed during metastasis. The authors also studied the effects of modifying the hydrogel mechanical properties and delivering a chemotherapeutic agent on invasive tumor migration. The advantages of this model system lie in its simplicity, customizability, and the ability to mimic vascular network. However, more work in terms of improving throughput; better control on tumor structure or growth; incorporation of endothelial and stromal cells and of alternative metastatic sites will offer significant benefit as future development. As an extension to the above study, another metastatic process assay was performed by Aleman and Skardal where they describe a metastasis-on-a-chip device that employs ECM-derived hydrogel biomaterials, houses multiple bioengineered 3D organoids, and was established by a 3D photopatterning technique (Aleman and Skardal, 2019). This metastasis-on-a-chip platform is designed such that CRC cells reside in a single microfluidic chamber connected to multiple downstream chambers in which liver, lung, and endothelial constructs are accommodated, to beautifully show CRC metastasis to liver and lung, just as occurs in human patients. Such multi-OoC models offer great tools for identification of targets for intervention.
Organ-on-a-chip studies focusing on genome instability and mutation
Although OoC studies have an immense potential towards pursuing genomic analysis, such studies seem to be rare so far. Hassell et al. performed a study to reproduce human orthotopic models of NSCLC in vitro by recapitulating the in vivo-like TME (Hassell et al., 2017). They investigated tumor growth on a lung-on-a-chip device made of two parallel channels (epithelial cells and a low density of NSCLC cells in one channel human and lung microvascular endothelial cells on the other) separated by a porous membrane, while mechanical suction was applied to mimic normal breathing. This orthotopic device successfully reproduced resistance to tyrosine kinase inhibitor therapy of patients showing specific mutations, a mechanism that was never observed in the conventional 2D in vitro models. As mentioned above in the vasculature section, Ozer et al. developed a cancer metastasis-on-a-chip bioassay towards high-content screening of a targeted anti-cancer therapy drug library, and their study included drugs such as Olaparib and Talazoparib, both of which are approved clinically as monotherapies for TNBC with germline BRCA mutations (Ozer et al., 2024). They also tested Lapatinib and Neratinib which are FDA-approved drugs for treating the subtype of HER2+ breast cancer patients, and which have differential effects on breast cancer cells with HER2 K753E mutation. Through their OoC platform the team was able to screen/evaluate drug response(s) and qualitatively assess drug effects on metastatic cascade processes such as tumor cell invasion and intravasation. Further, the effect of lung cancer metastasis to the liver was investigated in the afore-mentioned CMOM hypoxic microenvironment platform by Zheng et al. (2021). RNA-seq and protein expression results from this study indicated differentially expressed genes induced by hypoxia, of which several elevate EMT transcription factor expression levels, thereby regulating expression of the downstream factors resulting in the advancement of cancer invasion and metastasis. Furthermore, coculture of lung cancer cells with fibroblasts further promoted gene changes leading to lung cancer metastasis to liver cells, especially in hypoxic conditions. Such data can be envisioned for their wide application in future investigations of cancer metastasis and therapy screening under hypoxic conditions with the potential to replace/reduce animal experiments.
Further, from among the several commercial OoC devices that are nowadays available; Hubner et al. adapted a multi-organ-on-chip platform linking two-organ culture compartments for co-culture of human lung cancer microtissue and healthy human full-thickness skin equivalents (which is the site of target-mediated adverse effects in patients) to evaluate the effect of anti-EGFR antibodies (cetuximab) on both tumor and human skin tissue (Hübner et al., 2018). Their findings showed that repeated dose treatment of the cetuximab increased the pro-apoptotic related gene expression in the tumor lung microtissues. At the same time, they noticed crucial inhibitory effects on the physiological epidermal cell turnover in the skin and modulations in the release of certain chemokine ligands, reflecting the pattern changes seen in antibody-treated patients. Such OoC devices that combine metastatic tumor environment with a normal organotypic metastatic site equivalent provide ideal tools for therapeutic window identification and “safficacy” test assay, which is the simultaneous generation of efficacy and safety data. Integration of vasculature to such devices would further enhance the co-culture data due to organ and tumor conditioning.
Organ-on-a-chip studies focusing on tumor-promoting inflammation
Activation of immune-inflammatory signaling pathways is a hallmark of cancer as well and has been relatively less explored in the context of OoCs. Tsamandouras et al. used an interesting integrative multi-OoC platform comprising of human gut and liver muti-cell mixtures which were in continuous communication, to parallelly investigate different PK processes taking place after oral drug administration in humans (Tsamandouras et al., 2017). These processes were studied under normal and inflammatory contexts and can be relevantly applied to cancer-related inflammation as well. Other gut-on-chip devices modeling inflammatory disorders that can accommodate cancer cells, and that can configurate the coculture of the microbiome, also pose immense potential to be explored further in the context of cancer (Valiei et al., 2023; Taavitsainen et al., 2024). Surendran and team developed an OoC which is a novel and microfluidics-integrated 3D tumor spheroid-immune microenvironment (TIME)-on-a-chip device (Surendran et al., 2021). This device mimics tumor vasculature and recreates neutrophil exotosis and neutrophil extracellular traps (NET)osis functions in vivo in a reproducible and rapid way and could thus be used to study the interactional dynamics of neutrophils and ovarian tumor aggregates during the inception of their collective 3D invasion. Such OoC platforms are advantageous to increase analytical throughput for performing complex in vitro biomimetic assays, as well as for unravelling rationale for new anti-tumor therapeutics research.
Organ-on-a-chip studies focusing on senescent cells
In adults, senescence is thought to be a primary defense mechanism against cancer. Senescent cells exhibiting the senescence-associated secretory phenotype (SASPs) promote tumorigenesis by inducing EMT, and guiding cancer-cell migration and invasion in skin cancer. Pauty and colleagues developed a 3D tissue model-on-a-chip consisting of a blood vessel embedded in a collagen gel with young or senescent skin fibroblasts (Pauty et al., 2021). This chip enabled the team to investigate (SASP)-induced angiogenesis, and to evaluate the effects of SASP inhibitors, a class of drugs useful in cancer research.
Organ-on-a-chip studies focusing on polymorphic microbiomes
Alterations of the gut microbial composition and the accompanying dysregulation of the mucosal immune response underlay chronic inflammation and cancer. To create a platform for studying the underlying mechanisms, Maurer et al. established a 3D OoC model of the human intestine composed of endothelial and epithelial cell layers forming organotypic microanatomical villus- and crypt-like structures (Maurer et al., 2019). Through this platform, they demonstrated physiological interactions between epithelial and endothelial cells in an immunocompetent environment. Furthermore, they colonized the intestinal OoC model with non-damaging living bacteria which makes their intestinal model a valuable tool to systematically explore the underlying mechanisms of host-microbe interactions, immune cell activation and microbial pathogenicity mechanisms under physiologically relevant conditions in vitro. Thus, it represents a powerful platform for the investigation of disease mechanisms driven by pathogens, as well as for the screening and development of novel treatment strategies for diseases such as cancer.
Analysis of National Institutes of Health (NIH)/National Cancer Institute (NCI) funding in support of OoC technologies
Being part of NIH, and with an interest in the topic of OoC (in cancer), we used iSearch–an NIH grant database tool to analyze grants and contracts that investigated/employed OoC technologies and were submitted to/funded in the past 10 years (2013–2023) by the National Cancer Institute (NCI) and across all the National Institutes of Health (NIH) institute centers (ICs) as a whole. Key phrases included “tissue-on-chip”, “tissue-on-a-chip”, “organ-on-chip”, “organ-on-a-chip”, “cancer-on-chip”, “cancer-on-a-chip” “tumor-on-chip” or “tumor-on-a-chip”. As shown in Figure 4, a total number of 839 competing applications were received across NIH, of which 118 were funded, and the numbers of incoming applications grew over the years and reached 122 in FY 2023. NCI granted 20 OoC awards over the past 10 years, which were originally submitted to Notice of funding opportunities (NOFOs) such as PAR-15-021, RFA-CA20-054, PAR-19-056, PAR-19-113, PAR-19-354, PA-20-185 and PA-21-268. NCI’s Cancer Tissue Engineering Collaborative (TEC) (led by NCI/Division of cancer Biology (DCB)), through the afore-mentioned PAR19-113, called for the development of biomimetic tissue-engineered systems and funded 4 OoC-related cancer grants, wherein this NOFO mainly addresses fundamental research. In addition, to improve the rate of success of new therapeutics in drug development, National Center for Advancing Translational Sciences (NCATS)@NIH has awarded 10 inaugural grants, in response to RFA-TR-19-014“Clinical trials-on-a-chip: Tissue chips to inform clinical trial design and implementation in precision medicine (UG3/UH3 - Clinical Trial Not Allowed)”, to support researchers’ efforts in creating microphysiological, bioengineered models of human tissues and organ systems to inform clinical trial design for both common and rare diseases. This RFA has since expired in October 2019. In addition to helping inform clinical trial design, these projects also will support the planning and execution of clinical trials, assist in patient stratification, help identify reliable clinical trial endpoints, and ultimately develop tools for more informative and efficient clinical trials for both common and rare diseases. One of the awarded 10 grants focused on cancer. Appending to the above mentioned NOFOs, an SBIR contract call was issued recently by NCI (NIH/NCI 462 – Organ-on-a-chip for preclinical and translational radiobiological studies) focusing on radiobiology and drug-radiation combination studies.
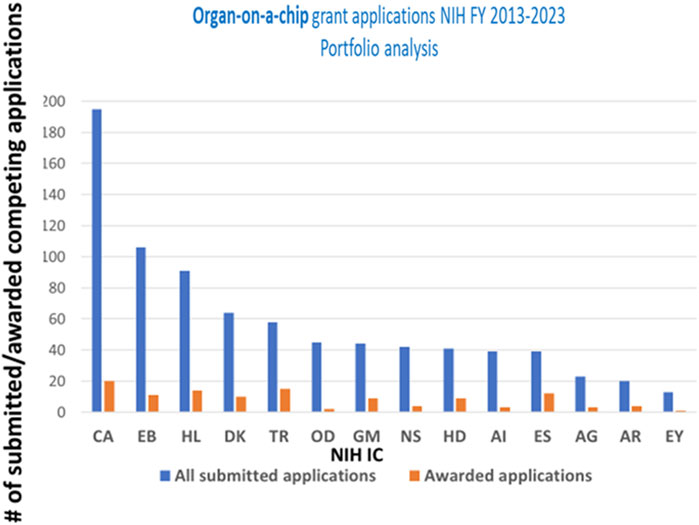
Figure 4. Organ-on-a-chip (OoC) grant applications submitted and awarded across the different NIH Institute Centers (ICs) over the last 10 fiscal years (FY). Acronyms on X-axis for NIH Institute Centers (IC): CA National Cancer Institute (NCI); EB National Institute of Biomedical Imaging and Bioengineering (NIBIB); HL National Heart, Lung, and Blood Institute (NHLBI); DK National Institute of Diabetes and Digestive and Kidney Diseases (NIDDK); TR National Center for Advancing Translational Sciences (NCATS); OD Office of the Director; GM National Institute of General Medical Sciences (NIGMS); NS National Institute of Neurological Disorders and Stroke (NINDS); HD Eunice Kennedy Shriver National Institute of Child Health and Human Development (NICHD); AI National Institute of Allergy and Infectious Diseases (NIAID); ES National Institute of Environmental Health Sciences (NIEHS); AG National Institute on Aging (NIA); AR National Institute of Arthritis and Musculoskeletal and Skin Diseases (NIAMS); EY National Human Genome Research Institute (NHGRI).
Conclusions and perspective on the future of OoC in cancer
While animal studies are still highly valued and required by regulators before a drug can move into clinical studies, alternative methods for preclinical drug evaluation are being pursued. In this scenario, developing and evaluating improved/new in vitro model(s) for human clinical mimicry will be of value. As explained above in this review article, a compelling avenue that is recently coming into its own age and fitting in this space is the use of OoC technology, as it allows researchers to intricately replicate the (patho)physiological processes of tissues and organs, extending capabilities of 2D/3D cell/tissue/organ cultures and providing to bridge the gap between current in vitro and in vivo preclinical studies and even to back-translate possibly from human systems (Ewart et al., 2022; Gil et al., 2023).
OoC platforms, by mimicking the main in vivo TME features, relatively better reflect the complex internal affairs of human tumors in easily accessible and controllable dynamic model systems, while also offering high-throughput potential. These systems are mainly used to screen/test anticancer drugs and to perform fundamental research towards understanding the biological basis of cancer by adding an adjustable degree of complexity through a fit-for-purpose approach. Cancer OoC platforms are being considered as candidates for contemporary preclinical models, and a large number of cancer OoC platforms have been designed and established to simulate tumors in the lung, liver, breast, brain, gut and kidney, and are considered to be useful tools for the development of effective anticancer therapies. OoC also offer to gain insights in immune-cancer interaction dynamics in an immunocompetent environment as mentioned in the sections above. Researchers are currently focusing on integrating organoids and OoC systems to narrow the gap between existing state of organoid technology and the actual development and function of organs in the human body (Zhao et al., 2024). Research on OoC technology has grown exponentially over the past decade with dramatic advances in the sophistication of biology and engineering; in the demonstration of physiological relevance; and in the range of applications.
OoC remaining challenges
Despite a number of proven examples that demonstrate the added value and potential of OoCs in cancer research, there is yet much more that is to be understood and explored to realize full potential of OoCs, as well as their limitations. Some issues that remain to be better addressed include the complexity of device manufacturing and its robustness; selection of optimal materials and conditions for preclinical drug screening studies; comparison of OoC test outcomes with existing preclinical methods; and clinical relevance of OoC tests. Given the complexity of the physiological structure and cancer microenvironment in vivo, there are still many challenges to be overcome in the development of cancer OoCs before they can be widely applied in practical pharmaceutical industrial and clinical applications. For example, cancer OoCs are more difficult to use than many other 3D culture systems, hence the need for highly specialized personnel and microfabrication facilities that can result in increased experimental costs. To circumvent this situation, development of user-friendly OoC systems and simultaneous standardization of data from different laboratories for the easy use of these emerging research models can help in obtaining meaningful data for clinical translation. One other main challenge that has remained in the field of OoCs in cancer includes the task of obtaining patient-specific cells, including primary cells and iPSCs, as the sample size to be housed in the OoC chip is small and can easily be damaged. Similarly, recapitulating cellular heterogeneity, which is a fundamental feature of tumor behavior, is another challenge in this small sample size, given the cellular range of 106 cells in OoCs, as opposed to the real size (typically > 109 cells) of tumor cells (Yu et al., 2017).
Accurate replication of complex human responses and achieving technical reliability and robustness remain to be fully demonstrated as well. For example, the small scale and complexity of microfluidic systems that provide controlled-fluid flow require the interplay of many factors to achieve optimal functionality, and simple factors, such as bubble formation, can cause major hurdles during experimentation. For long-term studies, there is the challenge of maintaining batch-to-batch variability, cell viability, functionality and structural integrity of multiple tissues as well as different cell types using a common media and consistent fluid flow. Issues associated with device design and choice of fabrication materials need to be also further addressed. Polydimethylsiloxane (PDMS), the most widely used material in the fabrication of cancer OoC devices is known to easily adsorb hydrophobic compounds, such as drugs and proteins, which may reduce drug concentrations and drug activity and can cause experimental errors in drug efficacy testing. A variety of new candidate materials have been developed, but the need for these devices to be optically transparent, mechanically adjustable, easily moldable, nonreactive, and economical, limits the available candidates. Given all the above, there is a need to rationally design microfluidic devices for the precision of the biophysicochemical properties of chips. Use of automation and set up for up-to-date functional readouts can help avoid experimental errors.
There is great potential for AI combined with high-content imaging/sensing and ML/deep learning to delineate the TME by identifying novel features, as well as to support experimental findings. OoC technology offers an opportunity for such combinations, and for the interpretation of multiomics data in an objective, reproducible, and efficient manner. As a step forward, the development of high-throughput whole-slide imaging technologies has enabled ML analyses of immunohistochemical (IHC) source data to yield large datasets for precision oncology, and for a more holistic analysis of the TME at the whole-slide level. As explained in the text above in the “immune destruction” section, AI tied with OoC technology offers the opportunity to predict the metastatic potential of cancer cells, as well as for the rapid and accurate detection of some kinds of cancer in clinical practice, in addition to differentiating between normal and tumor tissues, potentially enabling automated screening of tissues in cancer OoCs under different therapeutic regimes (Deng et al., 2023). Some of the limitations though of AI in the analysis of tumor models that must be overcome include the lack of sufficient, high-quality datasets. Given the vast quantity and complexity of information that is stored within the intricacies of the TME, further research is warranted to enable the extraction and maximization of data collection in these models.
Translational and regulatory context of OoC systems
FDA has shown strong interest in OoC technology and has partnered with industries and academic groups which are working on OoCs. As a measure to address ethical concerns employing animals for research, FDA has called for the inclusion of data from alternative models, such as OoC models, in IND applications, and suggested that in vitro models could be useful if they produce comparable results to animal models, and not necessarily better, provided they are equally robust and validated (Mahalmani et al., 2023). OoC technology thus comes as a promising fit for drug development, as an exciting in vitro alternative to assess not only the safety, but also the efficacy and mechanisms of action of drugs.
OoC models are thought to be cost-effective because they use a less time-consuming in vitro procedure and offer scope for scalability. The technology is supposed to bridge the gap within and between preclinical testing and human trials through better predictive models, thus significantly impacting R&D costs. Experts estimate the potential of OoCs to reduce R&D costs by 10%–26% (Franzen et al., 2019). In regard to systematic and quantitative evaluations of OoCs predictive value in cancer research, one good real-world example is that from the group of Ewart and Ingber et al. where they determined liver OoC ability to predict drug-induced liver injury caused by benchmark hepatotoxic and non-toxic small-molecules (Ewart et al., 2022). Their study aimed at assessing OoC utility in supporting toxicity-related decisions and was able to determine that their liver OoCs outperformed conventional models by predicting drug-induced liver injury, which is a common cause of drug failure, with a sensitivity of 87% and a specificity of 100%. This level of OoC performance, through increased small-molecule R&D productivity, is predicted to generate revenue over $3 billion annually for the pharmaceutical industry, in addition to the advantage of cutting down animal usage (Ewart et al., 2022). As far as commercialization is concerned, a small and diverse array of OoC devices and protocols in cancer space are slowly beginning to emerge, for, e.g., OoCs demonstrating CAR-T workflow (Emulate, 2024).
Despite advances in OoC technology, drug developers have not yet demanded that they be incorporated into efficacy and safety studies of newly tested compounds. Part of this is due to the dearth of performance validations of these OoC technologies that could potentially convince drug companies and contract research organizations (CROs) of their reliability. On the other end, the FDA Modernization Act 2.0 passed by the US Congress, could accelerate adoption of OoC technology in pharma and spark FDA to embody and embrace newer technologies (Mahalmani et al., 2023; Hargrove-Grimes et al., 2021; Hargrove-Grimes et al., 2022). European regulators are also, similar to the FDA, looking at developing data standards to be put in place to manage OoC-derived data. More recently, the FDA has also set up initiatives such as the “Innovative Science and Technology Approaches for New Drugs (iSTAND) pilot program” where submissions include the use of AI and tissue/organ-on-a-chips (i.e., microphysiological systems) to assess safety or efficacy questions towards promoting tools for drug discovery (FDA, 2023b). More in that direction, NIH-funded Centers have just started looking into projects for making organ/tissue-on-chips FDA-ready tools (NCATS, 2024).
Summary
This review discussed the applications of OoC technologies in advancing cancer research and oncology drug development from a cancer hallmarks’ perspective, which will help to promote the broader adoption of this platform in the missing cancer hallmark areas. From the extensive compilation of information provided in this review, we ought to recognize that OoC technology, as a resource, has so far offered great value in the fields of several cancer hallmarks, such as, sustaining proliferative signaling; inducing or accessing vasculature; modeling metabolism; avoiding immune destruction; and activating invasion and metastasis. However, there is still dearth of OoC employment/utility in several other cancer hallmark categories such as, in unlocking phenotypic plasticity; nonmutational epigenetic reprogramming; enabling replicative immortality; resisting cell death; genome instability and mutation; tumor-promoting inflammation; and senescent cells. Future research efforts employing OoCs focusing on tapping into the latter less-explored-cancer hallmark areas will help to answer several unanswered cancer questions and identify better treatment opportunities.
Further side-by-side validation in context of animal studies could also accelerate OoC data transfer from research to applications in cancer space (Caballero et al., 2022).
OoC technology does not aim to replace in vivo animal models but enables to provide a more complete picture and strengthen information by filling the gaps in the drug/therapy assessment and development. Several potential barriers still remain that need to be crossed including the need to achieve certain regulatory milestones before OoC technology reduces animal studies, as well as to provide comparable scores to previous animal studies showing drug efficacy and safety evidence that is significant enough for preclinical therapy studies to advance to human clinical trials. The continuous development of OoC technology will open new pathways for drug development, model building, and precision therapy, thereby enabling the development of the most beneficial treatment plan for cancer patients.
Author contributions
LA: Conceptualization, Data curation, Formal Analysis, Investigation, Methodology, Project administration, Resources, Validation, Writing–original draft, Writing–review and editing. PG: Conceptualization, Resources, Supervision, Writing–review and editing.
Funding
The author(s) declare that no financial support was received for the research, authorship, and/or publication of this article.
Acknowledgments
The authors would like to thank Dr. Micheal Espey and Dr. Yicong Wu for proof-reading the manuscript.
Conflict of interest
The authors declare that the research was conducted in the absence of any commercial or financial relationships that could be construed as a potential conflict of interest.
Publisher’s note
All claims expressed in this article are solely those of the authors and do not necessarily represent those of their affiliated organizations, or those of the publisher, the editors and the reviewers. Any product that may be evaluated in this article, or claim that may be made by its manufacturer, is not guaranteed or endorsed by the publisher.
References
Aboulkheyr Es, H., Zhand, S., Thiery, J. P., and Warkiani, M. E. (2020). Pirfenidone reduces immune-suppressive capacity of cancer-associated fibroblasts through targeting CCL17 and TNF-beta. Integr. Biol. 12, 188–197. doi:10.1093/intbio/zyaa014
Aleman, J., and Skardal, A. (2019). A multi-site metastasis-on-a-chip microphysiological system for assessing metastatic preference of cancer cells. Biotechnol. Bioeng. 116, 936–944. doi:10.1002/bit.26871
Atkins, J. T., George, G. C., Hess, K., Marcelo-Lewis, K. L., Yuan, Y., Borthakur, G., et al. (2020). Pre-clinical animal models are poor predictors of human toxicities in phase 1 oncology clinical trials. Br. J. Cancer 123, 1496–1501. doi:10.1038/s41416-020-01033-x
Ayuso, J. M., Rehman, S., Virumbrales-Munoz, M., Mcminn, P. H., Geiger, P., Fitzgerald, C., et al. (2021). Microfluidic tumor-on-a-chip model to evaluate the role of tumor environmental stress on NK cell exhaustion. Sci. Adv. 7, eabc2331. doi:10.1126/sciadv.abc2331
Boussommier-Calleja, A., Atiyas, Y., Haase, K., Headley, M., Lewis, C., and Kamm, R. D. (2019). The effects of monocytes on tumor cell extravasation in a 3D vascularized microfluidic model. Biomaterials 198, 180–193. doi:10.1016/j.biomaterials.2018.03.005
Bukhari, I., Zhang, Y., Thorne, R. F., and Mi, Y. (2022). Editorial: complexity of tumor microenvironment: a major culprit in cancer development. Front. Endocrinol. (Lausanne) 13, 1059885. doi:10.3389/fendo.2022.1059885
Caballero, D., Reis, R. L., and Kundu, S. C. (2022). Boosting the clinical translation of organ-on-a-chip technology. Bioeng. (Basel) 9, 549. doi:10.3390/bioengineering9100549
Casanova, C. R., Casanova, M. R., Reis, R. L., and Oliveira, J. M. (2024). “Advancing diagnostics and disease modeling: current concepts in biofabrication of soft microfluidic systems,” in In vitro models.
Cauli, E., Polidoro, M. A., Marzorati, S., Bernardi, C., Rasponi, M., and Lleo, A. (2023). Cancer-on-chip: a 3D model for the study of the tumor microenvironment. J. Biol. Eng. 17, 53. doi:10.1186/s13036-023-00372-6
Chakrabarty, S., Quiros-Solano, W. F., Kuijten, M. M. P., Haspels, B., Mallya, S., Lo, C. S. Y., et al. (2022). A microfluidic cancer-on-chip platform predicts drug response using organotypic tumor slice culture. Cancer Res. 82, 510–520. doi:10.1158/0008-5472.can-21-0799
Chen, Z.-Z., Li, W.-M., Zhang, Y., Yu, M., Shan, L.-F., Yuan, D.-Z., et al. (2016). Establishment of a gastric cancer subline with high metastatic potential using a novel microfluidic system. Sci. Rep. 6, 38376. doi:10.1038/srep38376
Chen, X., Zhang, Y. S., Zhang, X., and Liu, C. (2021). Organ-on-a-chip platforms for accelerating the evaluation of nanomedicine. Bioact. Mater. 6, 1012–1027. doi:10.1016/j.bioactmat.2020.09.022
Chernyavska, M., Masoudnia, M., Valerius, T., and Verdurmen, W. P. R. (2023). Organ-on-a-chip models for development of cancer immunotherapies. Cancer Immunol. Immunother. 72, 3971–3983. doi:10.1007/s00262-023-03572-7
Choi, Y., Hyun, E., Seo, J., Blundell, C., Kim, H. C., Lee, E., et al. (2015). A microengineered pathophysiological model of early-stage breast cancer. Lab. Chip 15, 3350–3357. doi:10.1039/c5lc00514k
Chung, M., Ahn, J., Son, K., Kim, S., and Jeon, N. L. (2017). Biomimetic model of tumor microenvironment on microfluidic platform. Adv. Healthc. Mater. 6. doi:10.1002/adhm.201700196
Conceição, F., Sousa, D. M., Loessberg-Zahl, J., Vollertsen, A. R., Neto, E., Søe, K., et al. (2022). A metastasis-on-a-chip approach to explore the sympathetic modulation of breast cancer bone metastasis. Mater. Today Bio 13, 100219. doi:10.1016/j.mtbio.2022.100219
Deng, S., Li, C., Cao, J., Cui, Z., Du, J., Fu, Z., et al. (2023). Organ-on-a-chip meets artificial intelligence in drug evaluation. Theranostics 13, 4526–4558. doi:10.7150/thno.87266
De Visser, K. E., and Joyce, J. A. (2023). The evolving tumor microenvironment: from cancer initiation to metastatic outgrowth. Cancer Cell 41, 374–403. doi:10.1016/j.ccell.2023.02.016
Elmusrati, M., and Ashammakhi, N. (2018). Cancer-on-a-Chip and artificial intelligence: tomorrow's cancer management. J. Craniofacial Surg. 29, 1682–1683. doi:10.1097/scs.0000000000004703
EMULATE (2024). Emulate organ-on-a-chip for cancer applications. Available at: https://emulatebio.com/cancer-application/.
Ewart, L., Apostolou, A., Briggs, S. A., Carman, C. V., Chaff, J. T., Heng, A. R., et al. (2022). Performance assessment and economic analysis of a human Liver-Chip for predictive toxicology. Commun. Med. (Lond) 2, 154. doi:10.1038/s43856-022-00209-1
FDA (2023a). About advancing alternative methods. Available at: https://www.fda.gov/science-research/advancing-alternative-methods-fda/about-alternative-methods.
Feitelson, M. A., Arzumanyan, A., Kulathinal, R. J., Blain, S. W., Holcombe, R. F., Mahajna, J., et al. (2015). Sustained proliferation in cancer: mechanisms and novel therapeutic targets. Seminars Cancer Biol. 35 (Suppl. l), S25–s54. doi:10.1016/j.semcancer.2015.02.006
Ferrari, E., Palma, C., Vesentini, S., Occhetta, P., and Rasponi, M. (2020). Integrating biosensors in organs-on-chip devices: a perspective on current strategies to monitor microphysiological systems. Biosensors 10, 110. doi:10.3390/bios10090110
Franzen, N., Van Harten, W. H., Retèl, V. P., Loskill, P., Van Den Eijnden-Van Raaij, J., and Ijzerman, M. (2019). Impact of organ-on-a-chip technology on pharmaceutical R&D costs. Drug Discov. Today 24, 1720–1724. doi:10.1016/j.drudis.2019.06.003
Giannitelli, S. M., Peluzzi, V., Raniolo, S., Roscilli, G., Trombetta, M., Mozetic, P., et al. (2024). On-chip recapitulation of the tumor microenvironment: a decade of progress. Biomaterials 306, 122482. doi:10.1016/j.biomaterials.2024.122482
Gil, J. F., Moura, C. S., Silverio, V., Gonçalves, G., and Santos, H. A. (2023). Cancer models on chip: paving the way to large-scale trial applications. Adv. Mater. 35, e2300692. doi:10.1002/adma.202300692
Gonçalves, I. M., Carvalho, V., Rodrigues, R. O., Pinho, D., Teixeira, S., Moita, A., et al. (2022). Organ-on-a-Chip platforms for drug screening and delivery in tumor cells: a systematic review. Cancers (Basel) 14, 935. doi:10.3390/cancers14040935
Hallou, A., Jennings, J., and Kabla, A. J. (2017). Tumour heterogeneity promotes collective invasion and cancer metastatic dissemination. R. Soc. Open Sci. 4, 161007. doi:10.1098/rsos.161007
Hanahan, D. (2022). Hallmarks of cancer: new dimensions. Cancer Discov. 12, 31–46. doi:10.1158/2159-8290.cd-21-1059
Hanahan, D., and Weinberg, R. A. (2011). Hallmarks of cancer: the next generation. Cell 144, 646–674. doi:10.1016/j.cell.2011.02.013
Hargrove-Grimes, P., Low, L. A., and Tagle, D. A. (2021). Microphysiological systems: what it takes for community adoption. Exp. Biol. Med. (Maywood) 246, 1435–1446. doi:10.1177/15353702211008872
Hargrove-Grimes, P., Low, L. A., and Tagle, D. A. (2022). Microphysiological systems: stakeholder challenges to adoption in drug development. Cells Tissues Organs 211, 269–281. doi:10.1159/000517422
Hassell, B. A., Goyal, G., Lee, E., Sontheimer-Phelps, A., Levy, O., Chen, C. S., et al. (2017). Human organ chip models recapitulate orthotopic lung cancer growth, therapeutic responses, and tumor dormancy in vitro. Cell Rep. 21, 508–516. doi:10.1016/j.celrep.2017.09.043
Hegde, P. S., and Chen, D. S. (2020). Top 10 challenges in cancer immunotherapy. Immunity 52, 17–35. doi:10.1016/j.immuni.2019.12.011
Herati, R. S., and Wherry, E. J. (2018). What is the predictive value of animal models for vaccine efficacy in humans? Consideration of strategies to improve the value of animal models. Cold Spring Harb. Perspect. Biol. 10, a031583. doi:10.1101/cshperspect.a031583
Horejs, C. (2021). Organ chips, organoids and the animal testing conundrum. Nat. Rev. Mater. 6, 372–373. doi:10.1038/s41578-021-00313-z
Huang, C. B. X., and Tu, T. Y. (2023). Recent advances in vascularized tumor-on-a-chip. Front. Oncol. 13, 1150332. doi:10.3389/fonc.2023.1150332
Hübner, J., Raschke, M., Rütschle, I., Gräßle, S., Hasenberg, T., Schirrmann, K., et al. (2018). Simultaneous evaluation of anti-EGFR-induced tumour and adverse skin effects in a microfluidic human 3D co-culture model. Sci. Rep. 8, 15010. doi:10.1038/s41598-018-33462-3
Imparato, G., Urciuolo, F., and Netti, P. A. (2022). Organ on chip technology to model cancer growth and metastasis. Bioeng. (Basel) 9, 28. doi:10.3390/bioengineering9010028
Ireson, C. R., Alavijeh, M. S., Palmer, A. M., Fowler, E. R., and Jones, H. J. (2019). The role of mouse tumour models in the discovery and development of anticancer drugs. Br. J. Cancer 121, 101–108. doi:10.1038/s41416-019-0495-5
Jiang, W. G., Sanders, A. J., Katoh, M., Ungefroren, H., Gieseler, F., Prince, M., et al. (2015). Tissue invasion and metastasis: molecular, biological and clinical perspectives. Seminars Cancer Biol. 35, S244–S275. doi:10.1016/j.semcancer.2015.03.008
Jouybar, M., De Winde, C. M., Wolf, K., Friedl, P., Mebius, R. E., and Den Toonder, J. M. J. (2024). Cancer-on-chip models for metastasis: importance of the tumor microenvironment. Trends Biotechnol. 42, 431–448. doi:10.1016/j.tibtech.2023.10.001
Kalinowska, D., Grabowska-Jadach, I., Liwinska, M., Drozd, M., Pietrzak, M., Dybko, A., et al. (2019). Studies on effectiveness of PTT on 3D tumor model under microfluidic conditions using aptamer-modified nanoshells. Biosens. Bioelectron. 126, 214–221. doi:10.1016/j.bios.2018.10.069
Kamei, K.-I., Kato, Y., Hirai, Y., Ito, S., Satoh, J., Oka, A., et al. (2017). Integrated heart/cancer on a chip to reproduce the side effects of anti-cancer drugs in vitro. RSC Adv. 7, 36777–36786. doi:10.1039/c7ra07716e
Kang, S., Park, S. E., and Huh, D. D. (2021). Organ-on-a-chip technology for nanoparticle research. Nano Converg. 8, 20. doi:10.1186/s40580-021-00270-x
Kerns, S. J., Belgur, C., Petropolis, D., Kanellias, M., Barrile, R., Sam, J., et al. (2021). Human immunocompetent Organ-on-Chip platforms allow safety profiling of tumor-targeted T-cell bispecific antibodies. Elife 10, e67106. doi:10.7554/elife.67106
Kim, S., Kim, W., Lim, S., and Jeon, J. S. (2017). Vasculature-on-A-chip for in vitro disease models. Bioengineering 4, 8. doi:10.3390/bioengineering4010008
Ko, J., Hyung, S., Heo, Y. J., Jung, S., Kim, S. T., Park, S. H., et al. (2024). Patient-derived tumor spheroid-induced angiogenesis preclinical platform for exploring therapeutic vulnerabilities in cancer. Biomaterials 306, 122504. doi:10.1016/j.biomaterials.2024.122504
Langhans, S. A. (2018). Three-Dimensional in vitro cell culture models in drug discovery and drug repositioning. Front. Pharmacol. 9, 6. doi:10.3389/fphar.2018.00006
Lee, J., Mehrotra, S., Zare-Eelanjegh, E., Rodrigues, R. O., Akbarinejad, A., Ge, D., et al. (2021). A heart-breast cancer-on-a-chip platform for disease modeling and monitoring of cardiotoxicity induced by cancer chemotherapy. Small 17, 2004258. doi:10.1002/smll.202004258
Lee, S., Kim, H., Kim, B. S., Chae, S., Jung, S., Lee, J. S., et al. (2024). Angiogenesis-on-a-chip coupled with single-cell RNA sequencing reveals spatially differential activations of autophagy along angiogenic sprouts. Nat. Commun. 15, 230. doi:10.1038/s41467-023-44427-0
Lei, K. F., Tseng, H.-P., Lee, C.-Y., and Tsang, N.-M. (2016). Quantitative study of cell invasion process under extracellular stimulation of cytokine in a microfluidic device. Sci. Rep. 6, 25557. doi:10.1038/srep25557
Leung, C. M., De Haan, P., Ronaldson-Bouchard, K., Kim, G.-A., Ko, J., Rho, H. S., et al. (2022). A guide to the organ-on-a-chip. Nat. Rev. Methods Prim. 2, 33. doi:10.1038/s43586-022-00118-6
Li, C., Li, S., Du, K., Li, P., Qiu, B., and Ding, W. (2021). On-chip replication of extremely early-stage tumor behavior. ACS Appl. Mater. & Interfaces 13, 19768–19777. doi:10.1021/acsami.1c03740
Li, J., Bai, H., Wang, Z., Xu, B., Peters Olson, K. N., Liu, C., et al. (2023). Advancements in organs-on-chips technology for viral disease and anti-viral research. Organs-on-a-Chip 5, 100030. doi:10.1016/j.ooc.2023.100030
Liu, X., Fang, J., Huang, S., Wu, X., Xie, X., Wang, J., et al. (2021). Tumor-on-a-chip: from bioinspired design to biomedical application. Microsystems & Nanoeng. 7, 50. doi:10.1038/s41378-021-00277-8
Liu, X., Su, Q., Zhang, X., Yang, W., Ning, J., Jia, K., et al. (2022). Recent advances of organ-on-a-chip in cancer modeling research. Biosens. (Basel) 12, 1045. doi:10.3390/bios12111045
Loewa, A., Feng, J. J., and Hedtrich, S. (2023). Human disease models in drug development. Nat. Rev. Bioeng. 1, 545–559. doi:10.1038/s44222-023-00063-3
Lynch, C., Sakamuru, S., Ooka, M., Huang, R., Klumpp-Thomas, C., Shinn, P., et al. (2024). High-throughput screening to advance in vitro toxicology: accomplishments, challenges, and future directions. Annu. Rev. Pharmacol. Toxicol. 64, 191–209. doi:10.1146/annurev-pharmtox-112122-104310
Ma, C., Peng, Y., Li, H., and Chen, W. (2021). Organ-on-a-Chip: a new paradigm for drug development. Trends Pharmacol. Sci. 42, 119–133. doi:10.1016/j.tips.2020.11.009
Mahalmani, V., Prakash, A., and Medhi, B. (2023). Do alternatives to animal experimentation replace preclinical research? Indian J. Pharmacol. 55, 71–75. doi:10.4103/ijp.ijp_223_23
Mattei, F., Andreone, S., Mencattini, A., De Ninno, A., Businaro, L., Martinelli, E., et al. (2021). Oncoimmunology meets organs-on-chip. Front. Mol. Biosci. 8, 627454. doi:10.3389/fmolb.2021.627454
Maulana, T. I., Kromidas, E., Wallstabe, L., Cipriano, M., Alb, M., Zaupa, C., et al. (2021). Immunocompetent cancer-on-chip models to assess immuno-oncology therapy. Adv. Drug Deliv. Rev. 173, 281–305. doi:10.1016/j.addr.2021.03.015
Maurer, M., Gresnigt, M. S., Last, A., Wollny, T., Berlinghof, F., Pospich, R., et al. (2019). A three-dimensional immunocompetent intestine-on-chip model as in vitro platform for functional and microbial interaction studies. Biomaterials 220, 119396. doi:10.1016/j.biomaterials.2019.119396
Mei, X., Middleton, K., Shim, D., Wan, Q., Xu, L., Ma, Y. H. V., et al. (2019). Microfluidic platform for studying osteocyte mechanoregulation of breast cancer bone metastasis. Integr. Biol. 11, 119–129. doi:10.1093/intbio/zyz008
Mencattini, A., Di Giuseppe, D., Comes, M. C., Casti, P., Corsi, F., Bertani, F. R., et al. (2020). Discovering the hidden messages within cell trajectories using a deep learning approach for in vitro evaluation of cancer drug treatments. Sci. Rep. 10, 7653. doi:10.1038/s41598-020-64246-3
Mencattini, A., Lansche, C., Veith, I., Erbs, P., Balloul, J. M., Quemeneur, E., et al. (2022). Direct imaging and automatic analysis in tumor-on-chip reveal cooperative antitumoral activity of immune cells and oncolytic vaccinia virus. Biosens. Bioelectron. 215, 114571. doi:10.1016/j.bios.2022.114571
Mi, S., Liu, Z., Du, Z., Yi, X., and Sun, W. (2019). Three-dimensional microfluidic tumor–macrophage system for breast cancer cell invasion. Biotechnol. Bioeng. 116, 1731–1741. doi:10.1002/bit.26961
Morales, I. A., Boghdady, C. M., Campbell, B. E., and Moraes, C. (2022). Integrating mechanical sensor readouts into organ-on-a-chip platforms. Front. Bioeng. Biotechnol. 10, 1060895. doi:10.3389/fbioe.2022.1060895
Ncats, N. (2024). NIH-funded centers look to make tissue chips FDA-ready tools. Available at: https://ncats.nih.gov/news-events/news/nih-funded-centers-look-to-make-tissue-chips-fda-ready.
Neophytou, C. M., Panagi, M., Stylianopoulos, T., and Papageorgis, P. (2021). The role of tumor microenvironment in cancer metastasis: molecular mechanisms and therapeutic opportunities. Cancers (Basel) 13, 2053. doi:10.3390/cancers13092053
Nguyen, D. H., Stapleton, S. C., Yang, M. T., Cha, S. S., Choi, C. K., Galie, P. A., et al. (2013). Biomimetic model to reconstitute angiogenic sprouting morphogenesis in vitro. Proc. Natl. Acad. Sci. U. S. A. 110, 6712–6717. doi:10.1073/pnas.1221526110
Nguyen, D. H. T., Lee, E., Alimperti, S., Norgard, R. J., Wong, A., Lee, J. J., et al. (2019). A biomimetic pancreatic cancer on-chip reveals endothelial ablation via ALK7 signaling. Sci. Adv. 5, eaav6789. doi:10.1126/sciadv.aav6789
Nguyen, M., De Ninno, A., Mencattini, A., Mermet-Meillon, F., Fornabaio, G., Evans, S. S., et al. (2018). Dissecting effects of anti-cancer drugs and cancer-associated fibroblasts by on-chip reconstitution of immunocompetent tumor microenvironments. Cell Rep. 25, 3884–3893 e3. doi:10.1016/j.celrep.2018.12.015
Nishiguchi, A., Matsusaki, M., Kano, M. R., Nishihara, H., Okano, D., Asano, Y., et al. (2018). In vitro 3D blood/lymph-vascularized human stromal tissues for preclinical assays of cancer metastasis. Biomaterials 179, 144–155. doi:10.1016/j.biomaterials.2018.06.019
Nolan, J., Pearce, O. M. T., Screen, H. R. C., Knight, M. M., and Verbruggen, S. W. (2023). Organ-on-a-Chip and microfluidic platforms for oncology in the UK. Cancers 15, 635. doi:10.3390/cancers15030635
Oh, H. J., Kim, J., Kim, H., Choi, N., and Chung, S. (2021). Microfluidic reconstitution of tumor microenvironment for nanomedical applications. Adv. Healthc. Mater. 10, e2002122. doi:10.1002/adhm.202002122
Oliver, C. R., Altemus, M. A., Westerhof, T. M., Cheriyan, H., Cheng, X., Dziubinski, M., et al. (2019). A platform for artificial intelligence based identification of the extravasation potential of cancer cells into the brain metastatic niche. Lab. Chip 19, 1162–1173. doi:10.1039/c8lc01387j
Ong, L. J. Y., Chia, S., Wong, S. Q. R., Zhang, X., Chua, H., Loo, J. M., et al. (2022). A comparative study of tumour-on-chip models with patient-derived xenografts for predicting chemotherapy efficacy in colorectal cancer patients. Front. Bioeng. Biotechnol. 10, 952726. doi:10.3389/fbioe.2022.952726
Ozer, L. Y., Fayed, H. S., Ericsson, J., and Al Haj Zen, A. (2023). Development of a cancer metastasis-on-chip assay for high throughput drug screening. Front. Oncol. 13, 1269376. doi:10.3389/fonc.2023.1269376
Pauty, J., Nakano, S., Usuba, R., Nakajima, T., Johmura, Y., Omori, S., et al. (2021). A 3D tissue model-on-a-chip for studying the effects of human senescent fibroblasts on blood vessels. Biomaterials Sci. 9, 199–211. doi:10.1039/d0bm01297a
Petreus, T., Cadogan, E., Hughes, G., Smith, A., Pilla Reddy, V., Lau, A., et al. (2021). Tumour-on-chip microfluidic platform for assessment of drug pharmacokinetics and treatment response. Commun. Biol. 4, 1001. doi:10.1038/s42003-021-02526-y
Polidoro, M. A., Ferrari, E., Soldani, C., Franceschini, B., Saladino, G., Rosina, A., et al. (2024). Cholangiocarcinoma-on-a-chip: a human 3D platform for personalised medicine. JHEP Rep. 6, 100910. doi:10.1016/j.jhepr.2023.100910
Preece, R., Pavesi, A., Gkazi, S. A., Stegmann, K. A., Georgiadis, C., Tan, Z. M., et al. (2020). CRISPR-mediated base conversion allows discriminatory depletion of endogenous T cell receptors for enhanced synthetic immunity. Mol. Ther. - Methods & Clin. Dev. 19, 149–161. doi:10.1016/j.omtm.2020.09.002
Refet-Mollof, E., Najyb, O., Chermat, R., Glory, A., Lafontaine, J., Wong, P., et al. (2021). Hypoxic jumbo spheroids on-A-chip (HOnAChip): insights into treatment efficacy. Cancers 13, 4046. doi:10.3390/cancers13164046
Rijal, G., and Li, W. (2017). A versatile 3D tissue matrix scaffold system for tumor modeling and drug screening. Sci. Adv. 3, e1700764. doi:10.1126/sciadv.1700764
Saha, B., Mathur, T., Tronolone, J. J., Chokshi, M., Lokhande, G. K., Selahi, A., et al. (2021). Human tumor microenvironment chip evaluates the consequences of platelet extravasation and combinatorial antitumor-antiplatelet therapy in ovarian cancer. Sci. Adv. 7, eabg5283. doi:10.1126/sciadv.abg5283
Sajjad, H., Imtiaz, S., Noor, T., Siddiqui, Y. H., Sajjad, A., and Zia, M. (2021). Cancer models in preclinical research: a chronicle review of advancement in effective cancer research. Animal Models Exp. Med. 4, 87–103. doi:10.1002/ame2.12165
Seaman, K., Sun, Y., and You, L. (2023). Recent advances in cancer-on-a-chip tissue models to dissect the tumour microenvironment. Med-X 1, 11. doi:10.1007/s44258-023-00011-1
Sehgal, K., Portell, A., Ivanova, E. V., Lizotte, P. H., Mahadevan, N. R., Greene, J. R., et al. (2021). Dynamic single-cell RNA sequencing identifies immunotherapy persister cells following PD-1 blockade. J. Clin. investigation 131, e135038. doi:10.1172/jci135038
Sharifi, F., Yesil-Celiktas, O., Kazan, A., Maharjan, S., Saghazadeh, S., Firoozbakhsh, K., et al. (2020). A hepatocellular carcinoma–bone metastasis-on-a-chip model for studying thymoquinone-loaded anticancer nanoparticles. Bio-Design Manuf. 3, 189–202. doi:10.1007/s42242-020-00074-8
Shin, Y., Han, S., Chung, E., and Chung, S. (2014). Intratumoral phenotypic heterogeneity as an encourager of cancer invasion. Integr. Biol. (Camb) 6, 654–661. doi:10.1039/c4ib00022f
Silvestri, V. L., Henriet, E., Linville, R. M., Wong, A. D., Searson, P. C., and Ewald, A. J. (2020). A tissue-engineered 3D microvessel model reveals the dynamics of mosaic vessel formation in breast cancer. Cancer Res. 80, 4288–4301. doi:10.1158/0008-5472.can-19-1564
Singh, G., Mishra, A., Mathur, A., Shastri, S., Nizam, A., Rizwan, A., et al. (2024). Advancement of organ-on-chip towards next generation medical technology. Biosens. Bioelectron. X 18, 100480. doi:10.1016/j.biosx.2024.100480
Skardal, A., Devarasetty, M., Forsythe, S., Atala, A., and Soker, S. (2016). A reductionist metastasis-on-a-chip platform for in vitro tumor progression modeling and drug screening. Biotechnol. Bioeng. 113, 2020–2032. doi:10.1002/bit.25950
Sokolowska, P., Zuchowska, A., and Brzozka, Z. (2022). Why can organoids improve current organ-on-chip platforms? Organoids 1, 69–84. doi:10.3390/organoids1010007
Sontheimer-Phelps, A., Hassell, B. A., and Ingber, D. E. (2019). Modelling cancer in microfluidic human organs-on-chips. Nat. Rev. Cancer 19, 65–81. doi:10.1038/s41568-018-0104-6
Stavrou, M., Phung, N., Grimm, J., and Andreou, C. (2023). Organ-on-chip systems as a model for nanomedicine. Nanoscale 15, 9927–9940. doi:10.1039/d3nr01661g
Strelez, C., Perez, R., Chlystek, J. S., Cherry, C., Yoon, A. Y., Haliday, B., et al. (2023). Integration of patient-derived organoids and organ-on-chip systems: investigating colorectal cancer invasion within the mechanical and GABAergic tumor microenvironment. bioRxiv. doi:10.1101/2023.09.14.557797
Sun, D., Gao, W., Hu, H., and Zhou, S. (2022). Why 90% of clinical drug development fails and how to improve it? Acta Pharm. Sin. B 12, 3049–3062. doi:10.1016/j.apsb.2022.02.002
Sun, W., Luo, Z., Lee, J., Kim, H., Lee, K., Tebon, P., et al. (2019). Organ-on-a-Chip for cancer and immune organs modeling. Adv. Healthc. Mater. 8, e1900754. doi:10.1002/adhm.201900754
Surendran, V., Rutledge, D., Colmon, R., and Chandrasekaran, A. (2021). A novel tumor-immune microenvironment (TIME)-on-Chip mimics three dimensional neutrophil-tumor dynamics and neutrophil extracellular traps (NETs)-mediated collective tumor invasion. Biofabrication 13, 035029. doi:10.1088/1758-5090/abe1cf
Taavitsainen, S., Juuti-Uusitalo, K., Kurppa, K., Lindfors, K., Kallio, P., and Kellomäki, M. (2024). Gut-on-chip devices as intestinal inflammation models and their future for studying multifactorial diseases. Front. Lab a Chip Technol. 2. doi:10.3389/frlct.2023.1337945
Tawade, P., and Mastrangeli, M. (2024). Integrated electrochemical and optical biosensing in organs-on-chip. Chembiochem 25, e202300560. doi:10.1002/cbic.202300560
Tian, C., Zheng, S., Liu, X., and Kamei, K.-I. (2022). Tumor-on-a-chip model for advancement of anti-cancer nano drug delivery system. J. Nanobiotechnology 20, 338. doi:10.1186/s12951-022-01552-0
Tsamandouras, N., Chen, W. L. K., Edington, C. D., Stokes, C. L., Griffith, L. G., and Cirit, M. (2017). Integrated gut and liver microphysiological systems for quantitative in vitro pharmacokinetic studies. Aaps J. 19, 1499–1512. doi:10.1208/s12248-017-0122-4
Tung, Y. C., Hsiao, A. Y., Allen, S. G., Torisawa, Y. S., Ho, M., and Takayama, S. (2011). High-throughput 3D spheroid culture and drug testing using a 384 hanging drop array. Analyst 136, 473–478. doi:10.1039/c0an00609b
Valiei, A., Aminian-Dehkordi, J., and Mofrad, M. R. K. (2023). Gut-on-a-chip models for dissecting the gut microbiology and physiology. Apl. Bioeng. 7, 011502. doi:10.1063/5.0126541
Veith, I., Nurmik, M., Mencattini, A., Damei, I., Lansche, C., Brosseau, S., et al. (2024). Assessing personalized responses to anti-PD-1 treatment using patient-derived lung tumor-on-chip. Cell Rep. Med. 5, 101549. doi:10.1016/j.xcrm.2024.101549
Wang, R., Zhang, C., Li, D., and Yao, Y. (2022). Tumor-on-a-chip: perfusable vascular incorporation brings new approach to tumor metastasis research and drug development. Front. Bioeng. Biotechnol. 10, 1057913. doi:10.3389/fbioe.2022.1057913
Wan, L., Yin, J., Skoko, J., Schwartz, R., Zhang, M., Leduc, P. R., et al. (2021). 3D collagen vascular tumor-on-a-chip mimetics for dynamic combinatorial drug screening. Mol. Cancer Ther. 20, 1210–1219. doi:10.1158/1535-7163.mct-20-0880
Wei, J., Cheng, L., Li, J., Liu, Y., Yin, S., Xu, B., et al. (2020). A microfluidic platform culturing two cell lines paralleled under in-vivo like fluidic microenvironment for testing the tumor targeting of nanoparticles. Talanta 208, 120355. doi:10.1016/j.talanta.2019.120355
Wu, X., Shi, W., Liu, X., and Gu, Z. (2024). Recent advances in 3D-printing-based organ-on-a-chip. EngMedicine 1, 100003. doi:10.1016/j.engmed.2024.100003
Yu, F., Zhuo, S., Qu, Y., Choudhury, D., Wang, Z., Iliescu, C., et al. (2017). On chip two-photon metabolic imaging for drug toxicity testing. Biomicrofluidics 11, 034108. doi:10.1063/1.4983615
Zhang, X., Karim, M., Hasan, M. M., Hooper, J., Wahab, R., Roy, S., et al. (2022). Cancer-on-a-Chip: models for studying metastasis. Cancers (Basel) 14, 648. doi:10.3390/cancers14030648
Zhang, Z., Shiratsuchi, H., Lin, J., Chen, G., Reddy, R. M., Azizi, E., et al. (2014). Expansion of CTCs from early stage lung cancer patients using a microfluidic co-culture model. Oncotarget 5, 12383–12397. doi:10.18632/oncotarget.2592
Zhao, Y., Landau, S., Okhovatian, S., Liu, C., Lu, R. X. Z., Lai, B. F. L., et al. (2024). Integrating organoids and organ-on-a-chip devices. Nat. Rev. Bioeng. 2, 588–608. doi:10.1038/s44222-024-00207-z
Zheng, L., Wang, B., Sun, Y., Dai, B., Fu, Y., Zhang, Y., et al. (2021). An oxygen-concentration-controllable multiorgan microfluidic platform for studying hypoxia-induced lung cancer-liver metastasis and screening drugs. ACS Sens. 6, 823–832. doi:10.1021/acssensors.0c01846
Zhu, J., Ji, L., Chen, Y., Li, H., Huang, M., Dai, Z., et al. (2023). Organoids and organs-on-chips: insights into predicting the efficacy of systemic treatment in colorectal cancer. Cell Death Discov. 9, 72. doi:10.1038/s41420-023-01354-9
Zhu, Y., Mandal, K., Hernandez, A. L., Kawakita, S., Huang, W., Bandaru, P., et al. (2021a). State of the art in integrated biosensors for organ-on-a-chip applications. Curr. Opin. Biomed. Eng. 19, 100309. doi:10.1016/j.cobme.2021.100309
Zhu, Y., Mandal, K., Hernandez, A. L., Kawakita, S., Huang, W., Bandaru, P., et al. (2021b). State of the art in integrated biosensors for organ-on-a-chip applications. Curr. Opin. Biomed. Eng. 19, 100309. doi:10.1016/j.cobme.2021.100309
Zuchowska, A., and Skorupska, S. (2022). Multi-organ-on-chip approach in cancer research. Organs-on-a-Chip 4, 100014. doi:10.1016/j.ooc.2021.100014
Glossary
Organ-on-a-chip (OoC): A system containing (human) cells or tissues grown inside a microfluidic chip. They mimic the structure and function of different organs/tissues and can be used for disease modeling, drug development, and personalized medicine.
Microphysiological systems: In vitro platforms/cell culture devices that simulate the activities, mechanics, and physiological response of an organ or organ system.
Tissue chips: Another name for OoC.
Cell co-culturing: A cell culture technique that uses microfabrication to create semipermeable surfaces for growing two different types of cells.
3D bioprinting: A technique that uses 3D printing to create functional tissue-like structures and organs to help develop patient-specific treatments and organ-specific treatments.
Bioassays: Analytical techniques used to detect and quantify biological molecules or activities within microfluidic devices.
Biomimetics: The study of nature inspired by biological systems to create new technologies and materials that mimic biological processes.
Keywords: cancer, organ-on-a-chip, in vitro, animal models, tumor microenvironment, metastasis, drug safety, therapy efficacy testing
Citation: Avula LR and Grodzinski P (2024) How organ-on-a-chip is advancing cancer research and oncology - a cancer hallmarks’ perspective. Front. Lab. Chip. Technol. 3:1487377. doi: 10.3389/frlct.2024.1487377
Received: 28 August 2024; Accepted: 07 November 2024;
Published: 19 November 2024.
Edited by:
Linan Jiang, University of Arizona, United StatesReviewed by:
Aleksander Skardal, The Ohio State University, United StatesSurjendu Maity, Duke University, United States
Copyright © 2024 Avula and Grodzinski. This is an open-access article distributed under the terms of the Creative Commons Attribution License (CC BY). The use, distribution or reproduction in other forums is permitted, provided the original author(s) and the copyright owner(s) are credited and that the original publication in this journal is cited, in accordance with accepted academic practice. No use, distribution or reproduction is permitted which does not comply with these terms.
*Correspondence: Leela Rani Avula, bGVlbGFyYW5pLmF2dWxhQG5paC5nb3Y=