- 1Department of Mechanical and Industrial Engineering, Northeastern University, Boston, MA, United States
- 2Department of Bioengineering, Northeastern University, Boston, MA, United States
- 3Institute for Mechanobiology, Northeastern University, Boston, MA, United States
Chemotherapy alone or in conjunction with surgery and radiation is often used to treat various cancer types. While effective at treating some tumors, the response varies across patients with different malignancies. For some cancers, such as glioblastoma, ovarian cancer, and soft tissue sarcoma, 85%–100% of patients experience cancer recurrence and develop chemotherapy resistance, which often leads to worse prognoses. These alarming statistics highlight an urgent need to better understand the landscape of therapy resistance in cancer, in order to develop improved treatment strategies and prevent recurrence. A central focus has been the investigation of resistant tumor subclones and whether the use of different alkylating agents and/or immune checkpoint inhibitors can ablate different clones. However, very little effort has been directed towards studies of the tumor microenvironment, a complex ecosystem of blood vessels, fibroblasts, immune cells, signaling molecules, and extracellular matrix, in the context of therapy resistance. In this perspective, we provide an overview of different platforms, tools, and techniques that have been developed and used to identify tumor microenvironment alterations due to therapy resistance. We also address potential therapeutic strategies that involve components of the tumor milieu and have been identified and tested to overcome treatment-induced resistance. Identifying microenvironmental changes post-resistance presents opportunities for new targeted treatment strategies. The current state of the literature suggests a dire need for more engineered models that probe specific microenvironment contributors to therapy resistance or ways in which the tumor tissue can be harnessed to mitigate resistance.
1 Introduction
Therapy efficacy and resistance remain barriers to effective cancer treatment. While chemotherapy is widely used in treating most cancer types, approximately 90% of patients undergoing chemotherapy treatment fail to achieve positive outcomes, predominantly due to acquired treatment resistance (Imai and Takaoka, 2006). It is estimated that 80%–90% of patient deaths are due to drug resistance (Ramos et al., 2021). While the vast majority of studies that address therapy resistance have focused on the emergence of resistant subclones in tumors, very few groups have focused on tumor microenvironment (TME)-induced clinical resistance following therapy. Although the TME is one of the first barriers encountered by therapeutic agents targeting tumors, its role in therapy efficacy and resistance remains poorly understood.
The TME is a highly complex architecture, physiologically characterized by hypoxia, low pH, and high interstitial fluid pressure (Zhong et al., 2020). One of the many cells that populate the TME are cancer-associated fibroblasts (CAFs) (Tilsed et al., 2022). CAFs have been shown to secrete transforming growth factor-beta (TGF-beta), vascular endothelial growth factor (VEGF), and fibroblast growth factor-2 (FGF2) to promote metastasis in vitro models of colorectal cancer. CAFs also contribute to fibrosis in the TME which reduces the availability of therapies through blood vessel compression and subsequently leads to increased interstitial pressure and poor drug penetration (Tilsed et al., 2022; Agosti et al., 2023). In patients with colorectal adenocarcinomas, CAFs have been shown to transfer exosomes to tumor cells containing miR-92a-3p, resulting in chemoresistance and metastasis (Bruch-Oms et al., 2023). CAFs have also been shown to promote chemotherapy and immunotherapy resistance through the Snail1-dependent polarization of M2 macrophages which reduces their cytotoxic behavior towards tumor cells (Agosti et al., 2023; Eckford and Sharom, 2009). Potential treatment strategies that target Snail1 in combination with standard alkylating agents could prevent the pro-tumorigenic behavior of macrophages. Other relevant tumor-supportive immune cells in the TME include monocytic myeloid-derived suppressor cells (m-MDSCs) and polymorphonuclear MDSCs (PMN-MDSCs). MDSCs, in particular, have been shown to contribute to angiogenesis via VEGF secretion as well as regulate aerobic glycolysis in breast cancer cells, resulting in worse prognoses for patients (Lei et al., 2020).
Recently, there has been more emphasis on investigating the role of cell surface transporters in the context of treatment resistance. Efflux pump proteins, such as multidrug resistance associated protein 1 (MDR-1), also called P-glycoprotein (P-gp), and ATP-Binding Cassette protein 2 (ABCG-2) have consistently been demonstrated to play a role in mechanisms of drug resistance in several cancer cell lines (Eckford and Sharom, 2009). P-gp was initially discovered in 1976 when researchers were investigating colchicine resistance in CHO cells (Juliano and Ling, 1976). However, later work with MCF-7 breast cancer cells revealed that P-gp-deficient MCF-7 were resistant to several compounds that P-gp usually confers resistance to (Eckford and Sharom, 2009). This resulted in the discovery of the ATP-dependent breast cancer resistance protein (BCRP/ABCG-2), involved in chemotherapy resistance in several tumor types (Eckford and Sharom, 2009). While these efflux transporters have been shown to contribute to drug efflux in cancer cells, their role in the TME and in vascular barriers remain understudied.
2 Current studies and models of treatment resistance
2.1 Computational models of the TME
Recently, several computational models have been designed to conduct theoretical studies on complex factors that regulate TME dynamics in the context of therapy resistance. These models have guided future experimental work while also enabling the validation of results from clinical trials. Nikmaneshi et al., for instance, developed a transport and cancer apoptosis model to study the effect of glycemia on drug delivery to generalizable tumor models with ECM components (Nikmaneshi et al., 2021). They found that hyperglycemia improves drug delivery to tumors and that neoadjuvant combination therapy is the most effective to ablate tumors (Figure 1A). In a subsequent study, the authors refined their model to capture the 3D architecture of tumor tissues and investigate low concentration-high frequency treatment schedules. They showed that these treatment regimens normalize tumor vasculature, improve drug delivery, and decrease cancer cell invasion (Nikmaneshi et al., 2023). Figures 1B,C highlight the effects of treatment schedules on drug delivery and cell viability from computational models. These theoretical results provide key insights into optimal dosage strategies to prevent chemotherapy resistance in the TME and can help guide clinicians to model treatment response. Future studies in the clinic can be done to validate the claim that metronomic treatment schedules increase tumor cell death while preserving non-cancerous tissue. Other computational agent-based studies have focused on correlating tumor spatial progression and patient outcomes. One group found that clustered adenocarcinoma tumors were strongly associated with more favorable patient outcomes in terms of recurrence, compared to tumors with random geographic diversification (Wu et al., 2022). Another group developed an agent-based pharmacokinetic model to optimize the treatment regimen of glioblastoma (GBM) based on the dynamics of the perivascular niche of its TME (Randles et al., 2021). The model predicted treatment timing strategies of chemotherapy delivery 1 h prior to radiation, in order to achieve optimal tumor ablation. The authors validated their computational results with in vivo experiments where PDGF-driven GBM mice were administered Temozolomide 1 h prior to radiation. These animals did not show evidence of acquired resistance and exhibited improved survival rates, validating the use of in silico models to predict tumor-TME-therapy interactions (Randles et al., 2021). Figure 1D highlights the efficacy of the predicted optimal schedule on tumor volume fraction compared to a suboptimal schedule. In their computational model of perivascular dynamics for optimized treatment schedules, Randles et al. validated their simulations with in vivo experiments. Figures 1E,F highlight these results, validating that the predicted improved response to the optimal schedule results in improved survival rates in mouse models of glioblastoma (ref). These results highlight the use of computational models as validation tools for animal experiments. Further comparisons to clinical data would confirm the value of these models as a tool for developing optimized treatment plans that improve overall survival.
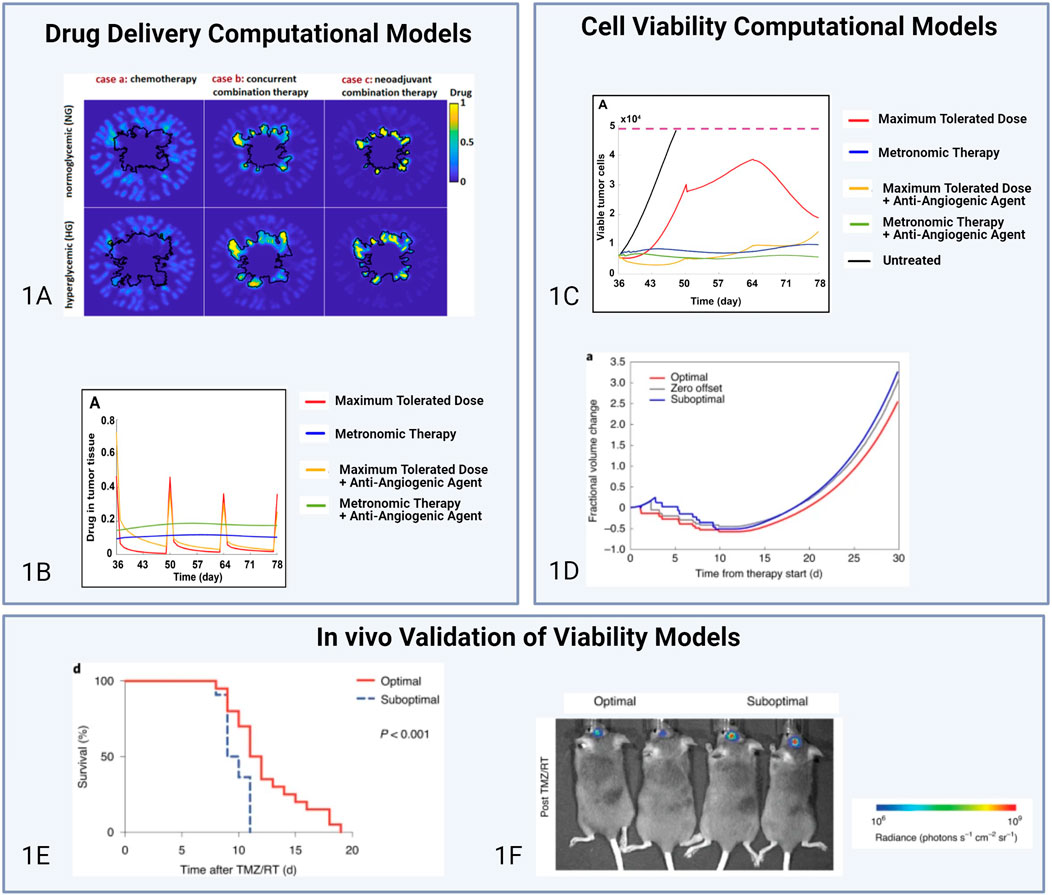
Figure 1. Comparisons of computational models of drug delivery, cell viability, and respective in vivo validations. The models from Nikmaneshi et al., (A) and Randles et al., (B, C) are both based on similar assumptions regarding cell proliferation behavior and the diffusivity of key molecules in the TME. However, the Nikmaneshi et al. model relies on cubic lattices to model the tumor and represent cellular dynamic behavior whereas the Randles model was implemented as a massively parallel simulation framework. (A) Impacts of hyperglycemic and normoglycemic conditions on drug delivery in chemotherapy treatment (case a), concurrent combination treatment (case b), and neoadjuvant combination therapy (case c) (Juliano and Ling, 1976). (B) Drug concentration in the tumor under various treatment regimens (Nikmaneshi et al., 2023). (C) Effect of various treatment regimens on cancer cell viability (Nikmaneshi et al., 2023). (D) Prediction plots of tumor volume fraction under different treatment schedules (Randles et al., 2021). (E, F) In vivo validation of computational model in (D). Post-treatment survival of mice treated with the optimized schedule (n = 20) versus the suboptimal schedule (n = 22) (n = 2 chemoradiation schedules) (Randles et al., 2021). Licensed Under Creative Commons Attribute 4.0.
Created with Biorender.com.
2.2 In Vivo models
Immunocompetent mouse models have enabled a greater understanding of the effect of the immune system on cancer progression and metastasis (Lei et al., 2016). Maniati et al. characterized the TME of six syngeneic murine high-grade-serous ovarian cancer (HGSOC) lines and identified several similarities to biopsies of the human HGSOC TME, notably in the transcriptome, host cell infiltrates, vasculature, and tissue modulus (Maniati et al., 2020). Transcriptional profiles of mouse tumors were analyzed and machine learning was utilized to classify and correlate chemotherapy sensitivity in mouse and patient tumors. Another group developed a transgenic mouse model of mammary carcinomas to study the role of the TME on Doxorubicin resistance (Nakasone et al., 2012). Their model identified vascular barrier disruption, which was influenced by tumor stage, and a lack of matrix metalloprotease (MMP)-9 secretion in Doxorubicin-sensitive tumors. MMP-9 was validated to contribute to barrier disruption in Doxorubicin-resistant tumors, which was also associated with CCR2+ monocyte recruitment and tumor relapse (Nakasone et al., 2012).
More recently, animal models have been used to identify the contributions of the immune system on resistance to immune blockade inhibitors. Using a combination of human biopsies and murine models of triple negative breast cancer, Huseni et al. showed that interleukin-6 (IL-6) is associated with poor prognosis and response to anti-PD-L1 inhibitors. A combination of anti-IL-6 receptor and anti-PD-L1 treatments was able to re-sensitize resistant murine models and improve anti-tumor cytotoxic T lymphocyte responses, compared to monotherapy with anti-PD-L1 alone (Huseni et al., 2023). This highlights the effect of cytokine contributions from the TME on therapy resistance and how alterations in the TME in resistant vs sensitive tumors can be harnessed to re-sensitize tumors to standard agents.
Animal models have also been used to look at the tumor vasculature. A study by Parrish et al. investigated the role of the blood-brain barrier and efflux transporters in orthotopic xenograft models treated with palbociclib, a cyclin-dependent kinase 4/6 inhibitor. Post-treatment, animals with subcutaneous GBM22 tumors had improved survival compared to those with intracranial tumors (Parrish et al., 2015). The authors found that combinations of palbociclib with efflux inhibitor elacridar lead to significant improvement in brain delivery, suggesting that BBB efflux transporters play a significant role in barrier and resistance to treatment (Parrish et al., 2015). These findings highlight the role of the vasculature in drug shuttling, resistance to treatment, and prognoses, suggesting a potential for TME targeting in combination with standard tumor-directed agents to improve treatment outcomes.
2.3 In Vitro models
In vitro models enable complex modeling of the TME using human cells to recapitulate patient response. These platforms also allow for low-cost and high-throughput experiments where components of the TME can be probed individually in the context of therapy resistance and effects on tumor cells. Several classes of in vitro models have been developed, including 2D cultures, 3D in vitro models using organoid systems with commercial cell lines or recently, patient-derived tissues, and tissue engineered 3D models developed using bioprinting or microfluidic technologies.
2.3.1 2D in vitro models
2D in vitro models enable rapid assessment of the contributions of the TME on therapy resistance and cancer cell invasion. Munoz et al. developed a 2D model of GBM and identified that overexpression of efflux transporter P-gp results in temozolomide resistance (Munoz et al., 2015). Using a Transwell model of ovarcian cancer, another group investigate the role of inflammatory cytokines in IgA transcytosis. They showed that elevated TNF and IL7 cytokines in the TME contribute to IgA transport and improved responses to cytolytic killing by T cells in ovarian cancer (Moon et al., 2014; Biswas et al., 2021). Gilmore et al., developed a 2D in vitro restricted exchange environment chamber (REEC) to study oxygen and nutrient gradients of the TME. They observed that cells form oxygen gradients within hours and after several days of culture, form disk like patterns based on hypoxic gradients. In Linville et al., studies of the blood-tumor barrier for metastatic breast cancer were conducted. The study mostly focuses on a 3D model to recapitulate the interaction, but separate 2D models were also studied and they found that the barrier dysfunction in the 2D model differed from findings in the 3D model. While these models provide rapid insights into the role of TME components in drug delivery and resistance, their architecture fails to mimic appropriate TME organization, and several groups have opted for 3D models to circumvent these challenges.
2.3.2 3D in vitro models using commercial cell lines
3D in vitro models provide a better recapitulation of the tumor microenvironment through the addition of other cell types and acellular components to mimic the 3D structure of tissues with the presence of an ECM, vasculature, and immune cells (Hajal et al., 2021). Various studies (Hajal et al., 2022; Jubelin et al., 2022) have shown that gene expressions of 2D cell cultures differ from 3D cultures, and 3D organoids cultured with other TME components exhibit genomic and phenotypic profiles similar to those seen in original patient tissues. These similarities to patient genomic profiles allow 3D in vitro models to provide results that more closely resemble results from clinical or in vivo environments.
In vitro 3D models using commercially available cells lines enable the study of 3D TME interactions in the context of therapy resistance for several tumor types. In a co-cultured model of human umbilical vein endothelial cells (HUVECs) with A549 adenocarcinoma cells and T24 bladder carcinoma cells, Bai et al. identified that only the A549 co-coculture inhibited HGF-and-FGF2-dependent HUVEC cell dispersion in the presence of therapy AZD0530 (Src inhibitor originally developed for metastatic colon cancer) (Bai et al., 2015). This suggests that therapies targeting the epithelial-to-mesenchymal transition may offer clinical benefit to control vascularization, through HGF and FGF-2, and its effects on cancer spread in adenocarcinomas. In a different model of 3D tumor spheroids using H460 lung cancer cells, Meng et al. demonstrated enhanced activity of the hypoxia-activated prodrug TH-302 compared to monolayer cultures of H460 (Meng et al., 2012). This highlights the contributions of the 3D tumor space in assessing TME contributors to drug resistance and cancer spread.
2.3.3 3D in vitro models using patient-derived cell lines
Patient-derived explant (PDE) models are ex vivo models that allow for individualized characterization of tumor treatments from resected human tumor and tissue samples. These personalized medicine platforms enable high-throughput drug screening to identify amenable therapies that achieve desired outcomes while avoiding treatment resistance. These platforms can also be used to probe the contributions of individual components of the TME that were maintained from the original tumor in the context of therapy response. One group was able to develop a well characterized cohort of PDEs from prostate cancer patients which accurately captured the TME integrity, tissue morphology, and androgen receptor (AR) signaling found in vivo for these patients (Shafi et al., 2018). In particular, the authors found that high levels of phosphorylated focal adhesion kinase (pFAK) and activation of alpha-5 integrin in the TME were associated with a tumor permissive state in two of the PDE samples and de novo resistance to enzalutamide. In a different 3D model of patient-derived GBM in hydrogels, Wang et al. demonstrated that 3D cultured GBM tumors exhibited increased temozolomide resistance compared to 2D controls and increasing the stiffness of the hydrogels in which GBM spheroids were embedded resulted in greater treatment resistance (Wang et al., 2021).
PDEs have also enabled studies of the tumor-immune microenvironment and contributions to resistance. In mismatch repair-deficient glioma explands, Touat and Li et al. observed poor CD3+ T-cell infiltrates, no substantial intratumoral heterogeneity, and poor response to PD-1 blockade. These PDEs were also resistant to temozolomide. These findings contradict previous findings in other tumors such as colorectal carcinomas where mismatch repair deficiency improves response to PD-1 inhibitors (Touat et al., 2020). In another study of GBM patient brain slices, spatially resolved transcriptomic sequencing was used to study the immunosuppressive TME following JAK1/2 inhibitor treatment. Interleukin-10 was identified to be linked to the transformation of T-cells from effectors to dysfunctional and a decrease in myeloid cells (Ravi et al., 2022). The authors then performed a case study on JAK/STAT inhibition in a single GBM patient with recurrence post-radiation after treatment with alkylating agents lomustine and temozolomide and TTField therapy (Ravi et al., 2022). This resulted in an increase in CD8+ and CD4+ T cell infiltration with stable CD68+ expression compared to pre-treatment tissue staining. Importantly, disease progression was stable for approximately 8.5 months after treatment (Ravi et al., 2022). These results highlight ways in which the immune tumor landscape can be targeted to improve survival and response to treatment, following resistance to standard alkylating agents.
In addition to tumor explants, groups have also focused on the development of patient-derived organoids (PDOs) to study clinical response in a physiologically relevant context. Unlike PDEs which consist in tumor sections or slices directly obtained from biopsies or surgeries with minimal intervention, PDOs are generated from dissociating the tumor tissue and growing the mixture of cells in amenable 3D hydrogels composed of Matrigel or Collagen (Neal et al., 2018). Neal et al. designed PDO models of melanoma, renal cell carcinoma, and non-small cell lung cancer, which they later treated with PD-1 immune inhibitors, following validation of the presence of T lymphocytes, among other components of the immune system. Their study identified that their PDO model and treatment regimen was able to activate tumor-antigen specific tumor-infiltrating lymphocytes to illicit an immune response post-therapy (Neal et al., 2018). Another study using PDOs of esophageal squamous cell carcinomas found that CD44 expression was strongly correlated with enriched organoid formation and resistance to 5-fluorouracil treatment (Kijima et al., 2019). Other studies have validated CD44 as a therapeutic target in the TME for esophageal squamous cell carcinomas, specifically with tumor associated macrophages regulating the P13K-4EBP1-Sox2 pathway, suggesting a potential use of CD44 inhibitors in the treatment of resistant models (Kijima et al., 2019).
High-throughput screening in organoids has also been used to identify inhibitors of breast cancer resistance protein (BCRP) in intestinal tumors derived from mouse tissue (Zhang et al., 2017). The development of a BCRP biosensor was also established to detect the function of BCRP in the organoid model (Zhang et al., 2017). This study provides a significant advancement in the study of efflux transporters and their role in therapy resistance and cancer progression, as well as the development of biomarkers of resistance targeting components of the TME that may be responsible for poor response to treatment (Hadj Bachir et al., 2022; Meier et al., 2022; Harada and Sakamoto, 2022).
While current research into 3D in vitro models enables high-throughput and targeted studies of the TME in cancer, very few studies have focused on using 3D in vitro models to probe individual components of the TME in the context of therapy resistance, particularly non-immune components. One explanation lies in the lack of available tissue from patients with recurrent and therapy-resistant tumors in order to properly model several components of the TME of resistant tissues, such as vasculature, stromal compartments, and ECM. PDOs are also a relatively small subset of the organoid model space as most organoid systems are derived from animal tissue, which validates the low availability of patient tissue models for therapy resistance studies. While patient derived models are valuable for precision medicine, they also have limitations such as loss of cells and genomic background during culture over time, study selection bias in choosing initial tissues, and the inability to fully recapitulate the tumor microenvironment in 2D cases and native immune responses in 3D cases (Hou et al., 2022). The use of patient derived models must also adhere to strict regulatory conditions to protect private patient information. Despite these limitations, patient derived models still prove to be extremely valuable in progressing cancer research, owing to their ability to recapitulate genomic and phenotypic profiles found in a specific patient for personalized medicine and rapid drug testing.
2.3.4 Tissue engineered models
The ability to include several components of the TME, including cells, ECM, relevant fluid flow profiles, and hypoxic conditions in a three-dimensional space makes tissue engineered models highly amenable to studies of drug-tissue interactions in physiologically relevant multi-organ systems. Herland et al. developed vascularized organ chips of the gut, liver, and kidneys to successfully model the pharmacokinetics of orally administered nicotine and intravenously administered alkylating agent cisplatin (Herland et al., 2020). We have previously designed microfluidic models of tissue engineered BBB vasculatures that closely recapitulated BBB protein expression in 3D endothelial cells and reliable molecular permeabilities previously only observed in vivo (Hajal et al., 2022). In a subsequent study, this vascular platform was combined with patient-derived glioma tumor spheroids to show that lipoprotein receptor (LRP-1) expression was improved at the tumor vasculature. We harnessed this finding to develop cisplatin-encapsulated liposomes for targeted delivery to GBM through LRP1 transport at the BBB (Straehla et al., 2022). These results highlight the potential of tissue engineered platforms to be employed to validate mechanisms of therapy resistance at the scale of the tumor tissue, and not simply tumor cells. Another group developed a microfluidic model of the BBB, with GBM spheroids cultured in an adjacent channel, and showed that combination of doxorubicin with mannitol and gintonin enables a temporary increase in BBB permeability to mitigate chemotherapy resistance. Accelerated delivery of doxorubicin was also associated with accelerated uptake of doxorubicin by the GBM tumor spheroids in the device (Seo et al., 2022). Ayuso et al. used a microfluidic model to recapitulate the hypoxic microenvironment of ductal carcinoma in situ and test the efficacy of Tirapazamine (Ayuso et al., 2018). They observed that ductal carcinoma models cultured in the presence of Tirapazamine developed necrotic regions within the tumor core where hypoxia was measured to be the highest.
Other groups have also pushed the boundaries of tissue engineered systems, with the development of entirely patient-derived models of the GBM TME, using patient-derived glial stem cells, astrocytes, microglia, and GBM cells. With this patient-specific model, the authors showed that astrocyte and microglia activation and reactivity in the TME strongly correlated with glioma cell stemness and invasion (Cornelison et al., 2022). This was validated in animal models of GBM stem cells that were used for therapy screening. By understanding the mechanisms stromal cell activation through cancer stem cell progression, researchers can develop targeted therapies to stromal compartments involved in therapy resistance. Although these models suggest important advances in the realm of tissue engineered models of the TME for drug resistance, further studies need to be performed to dissect individual contributions of TME components in therapy resistance. These findings would enable the development of preventative treatment combinations with standard agents and inhibitors to TME components involved in resistance, to potentially mitigate future resistance and achieve improved response to standard first-line agents (Figure 2).
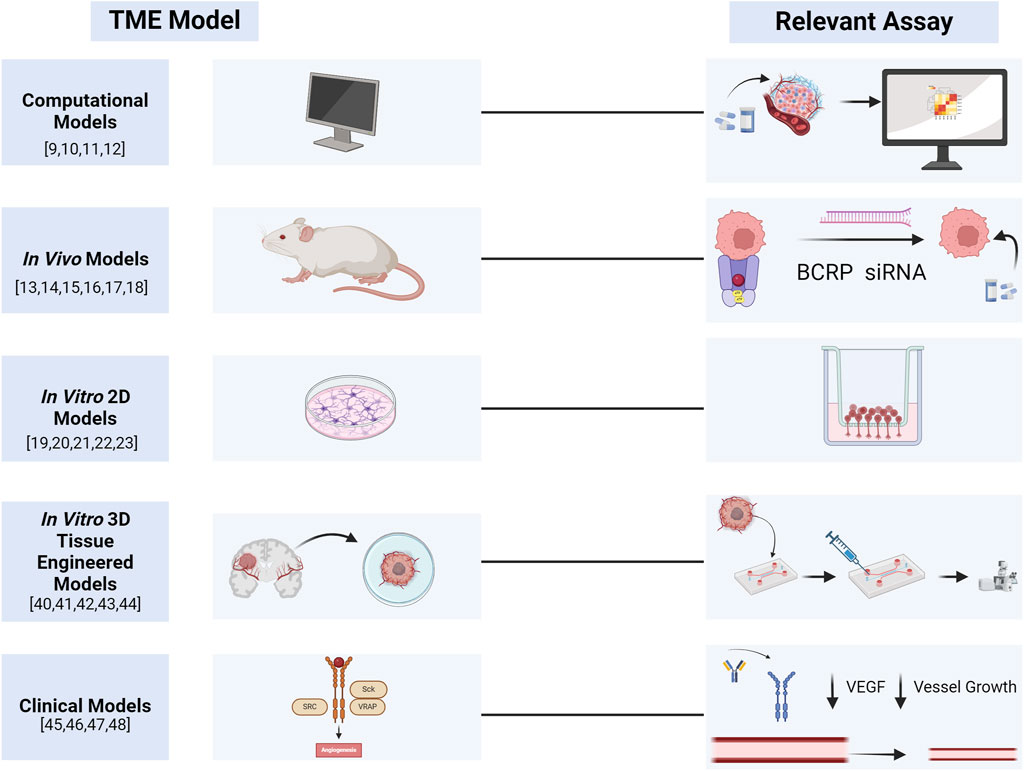
Figure 2. Graphical summary of TME models and their associated assays for assessing drug delivery and treatment resistance. Created with Biorender.com.
3 Discussion
Cancer recurrence is largely attributed to resistance to treatment in tumors. While emphasis has been placed on identifying mechanisms of resistance due to genomic alterations in tumor subclones, the role of the evolving TME in treatment resistance and cancer invasion remains poorly understood. This perspective identified studies that set the foundation for further assays and models that need to be developed to understand the role of complex components of the microenvironment in treatment resistance. Identifying these TME changes is essential in developing TME-targeting therapies to combat resistance based on cancer-stroma-immune interactions in post-treatment tumors.
The tumor vasculature, for instance, has been a target in cancer treatment for several years with the development of FDA-approved anti-angiogenesis agents that target VEGF (Meadows and Hurwitz, 2012; Hirata and Sahai, 2017) (Figure 2). Current FDA-approved anti-angiogenesis agents such as sunitinib, sorafenib, and pazopanib were approved as monotherapy treatments for renal cell cancer, while bevacizumab was approved for use with chemotherapy to treat colorectal, non-small cell lung, breast, and renal cancers. Bevacizumab is approved for use in glioblastoma as a monotherapy (Meadows and Hurwitz, 2012). While anti-angiogenic agents looked promising when they were first introduced, improved humanized models, imaging tools, and genomic capabilities have quickly shown that targeting VEGF may lead to more aggressive tumors, harboring resistance to other agents (Meadows and Hurwitz, 2012). Additional work is needed to understand the evolving TME in resistance and develop better treatment strategies that target the vasculature, but also other understudied components of the TME.
Focusing on hypoxia in the TME, small molecule inhibitors, such as carbonic anhydrase inhibitors, have also recently been enrolled in clinical trials to treat hypoxic tumors (Supuran, 2020). SLC-0111, a carbonic anhydrase inhibitor, completed a Phase I clinical trial to study antitumor effects in advanced hypoxic solid tumors (NCT02215850). In 2017, it was enrolled in an ongoing Phase Ib/II study to study the efficacy of the inhibitor in combination with other therapeutic agents for the treatment of pancreatic cancer (Supuran, 2020). In head and neck squamous carcinoma, SLC-0111 was found to boost the effect of cisplatin in inhibiting cancer invasion (Sarnella et al., 2022), suggesting potential use in combination with standard alkylating agents to target both hypoxia and replicating cancer cells.
The current literature suggests that there are several promising platforms that can be used to study the TME and treatment resistance independently. However, few have been developed to combine these aspects into cohesive and validated models. The few studies featured in this perspective highlight potential limitations in scaling and standardizing these models. Access to representative patient samples for ex vivo modeling is also challenging given the need for sufficient fresh tumor tissue from tissue biobanks and requirements for adherence to strict standards regarding patient confidentiality over the use and publication of data. Ensuring diversity in patient samples across metrics of race, gender, sexuality, and socioeconomic status, among others features of patient diversity, is also essential in developing results that can be applicable and generalizable to a broad set of patients with varying needs. These limitations can be overcome with appropriate efforts in developing personalized human models of the TME that can be used for therapy screening and resistance. The positive results from models and trials in this perspective highlight the importance of the TME in cancer treatment. Harnessing the tumor milieu in cancer treatment represents the next frontier in improving therapies and prognoses for patients with recurrent and aggressive tumors.
Data availability statement
The original contributions presented in the study are included in the article/supplementary material, further inquiries can be directed to the corresponding author.
Author contributions
GG: Data curation, Investigation, Methodology, Visualization, Writing–original draft, Writing–review and editing. CS: Data curation, Investigation, Methodology, Visualization, Writing–original draft, Writing–review and editing. CH: Conceptualization, Data curation, Investigation, Methodology, Project administration, Resources, Supervision, Validation, Visualization, Writing–original draft, Writing–review and editing.
Funding
The author(s) declare that no financial support was received for the research, authorship, and/or publication of this article.
Conflict of interest
The authors declare that the research was conducted in the absence of any commercial or financial relationships that could be construed as a potential conflict of interest.
Publisher’s note
All claims expressed in this article are solely those of the authors and do not necessarily represent those of their affiliated organizations, or those of the publisher, the editors and the reviewers. Any product that may be evaluated in this article, or claim that may be made by its manufacturer, is not guaranteed or endorsed by the publisher.
References
Agosti, E., Panciani, P. P., Zeppieri, M., De Maria, L., Pasqualetti, F., Tel, A., et al. (2023). Tumor microenvironment and glioblastoma cell Interplay as Promoters of therapeutic resistance. Biology 12 (5), 736. doi:10.3390/biology12050736
Ayuso, J. M., Gillette, A., Lugo-Cintrón, K., Acevedo-Acevedo, S., Gomez, I., Morgan, M., et al. (2018). Organotypic microfluidic breast cancer model reveals starvation-induced spatial-temporal metabolic adaptations. EBioMedicine 37, 144–157. doi:10.1016/j.ebiom.2018.10.046
Bai, J., Tu, T. Y., Kim, C., Thiery, J. P., and Kamm, R. D. (2015). Identification of drugs as single agents or in combination to prevent carcinoma dissemination in a microfluidic 3D environment. Oncotarget 6 (34), 36603–36614. doi:10.18632/oncotarget.5464
Biswas, S., Mandal, G., Payne, K. K., Anadon, C. M., Gatenbee, C. D., Chaurio, R. A., et al. (2021). IgA transcytosis and antigen recognition govern ovarian cancer immunity. Nature 591 (7850), 464–470. doi:10.1038/s41586-020-03144-0
Bruch-Oms, M., Olivera-Salguero, R., Mazzolini, R., del Valle-Pérez, B., Mayo-González, P., Beteta, Á., et al. (2023). Analyzing the role of cancer-associated fibroblast activation on macrophage polarization. Mol. Oncol. 17 (8), 1492–1513. doi:10.1002/1878-0261.13454
Cornelison, R. C., Yuan, J. X., Tate, K. M., Petrosky, A., Beeghly, G. F., Bloomfield, M., et al. (2022). A patient-designed tissue-engineered model of the infiltrative glioblastoma microenvironment. npj Precis. Oncol. 6 (1), 54. doi:10.1038/s41698-022-00290-8
Eckford, P. D. W., and Sharom, F. J. (2009). ABC efflux pump-based resistance to chemotherapy drugs. Chem. Rev. 109 (7), 2989–3011. doi:10.1021/cr9000226
Hadj Bachir, E., Poiraud, C., Paget, S., Stoup, N., El Moghrabi, S., Duchêne, B., et al. (2022). A new pancreatic adenocarcinoma-derived organoid model of acquired chemoresistance to FOLFIRINOX: first insight of the underlying mechanisms. Biol. Cell 114 (1), 32–55. doi:10.1111/boc.202100003
Hajal, C., Le Roi, B., Kamm, R. D., and Maoz, B. M. (2021). Biology and models of the blood-brain barrier. Annu. Rev. Biomed. Eng. 23, 359–384. doi:10.1146/annurev-bioeng-082120-042814
Hajal, C., Offeddu, G. S., Shin, Y., Zhang, S., Morozova, O., Hickman, D., et al. (2022). Engineered human blood–brain barrier microfluidic model for vascular permeability analyses. Nat. Protoc. 17 (1), 95–128. doi:10.1038/s41596-021-00635-w
Harada, K., and Sakamoto, N. (2022). Cancer organoid applications to investigate chemotherapy resistance. Front. Mol. Biosci. 9, 1067207. doi:10.3389/fmolb.2022.1067207
Herland, A., Maoz, B. M., Das, D., Somayaji, M. R., Prantil-Baun, R., Novak, R., et al. (2020). Quantitative prediction of human pharmacokinetic responses to drugs via fluidically coupled vascularized organ chips. Nat. Biomed. Eng. 4 (4), 421–436. doi:10.1038/s41551-019-0498-9
Hirata, E., and Sahai, E. (2017). Tumor microenvironment and Differential responses to therapy. Cold Spring Harb. Perspect. Med. 7 (7), a026781. doi:10.1101/cshperspect.a026781
Hou, X., Du, C., Lu, L., Yuan, S., Zhan, M., You, P., et al. (2022). Opportunities and challenges of patient-derived models in cancer research: patient-derived xenografts, patient-derived organoid and patient-derived cells. World J. Surg. Oncol. 20 (1), 37. Published 2022 Feb 17. doi:10.1186/s12957-022-02510-8
Huseni, M. A., Wang, L., Klementowicz, J. E., Yuen, K., Breart, B., Orr, C., et al. (2023). CD8+ T cell-intrinsic IL-6 signaling promotes resistance to anti-PD-L1 immunotherapy. Cell Rep. Med. 4 (1), 100878. doi:10.1016/j.xcrm.2022.100878
Imai, K., and Takaoka, A. (2006). Comparing antibody and small-molecule therapies for cancer. Nat. Rev. Cancer 6 (9), 714–727. doi:10.1038/nrc1913
Jubelin, C., Muñoz-Garcia, J., Griscom, L., Cochonneau, D., Ollivier, E., Heymann, M. F., et al. (2022). Three-dimensional in vitro culture models in oncology research. Cell Biosci. 12, 155. doi:10.1186/s13578-022-00887-3
Juliano, R. L., and Ling, V. (1976). A surface glycoprotein modulating drug permeability in Chinese hamster ovary cell mutants. Biochimica Biophysica Acta (BBA) - Biomembr. 455 (1), 152–162. doi:10.1016/0005-2736(76)90160-7
Kijima, T., Nakagawa, H., Shimonosono, M., Chandramouleeswaran, P. M., Hara, T., Sahu, V., et al. (2019). Three-dimensional organoids reveal therapy resistance of esophageal and Oropharyngeal squamous cell carcinoma cells. Cell. Mol. Gastroenterology Hepatology 7 (1), 73–91. doi:10.1016/j.jcmgh.2018.09.003
Lei, X., Lei, Y., Li, J. K., Du, W. X., Li, R. G., Yang, J., et al. (2020). Immune cells within the tumor microenvironment: Biological functions and roles in cancer immunotherapy. Cancer Lett. 470, 126–133. doi:10.1016/j.canlet.2019.11.009
Lei, Z. G., Ren, X. H., Wang, S. sha, Liang, X. hua, and Tang, Y. L. (2016). Immunocompromised and immunocompetent mouse models for head and neck squamous cell carcinoma. OncoTargets Ther. 9, 545–555. doi:10.2147/ott.s95633
Maniati, E., Berlato, C., Gopinathan, G., Heath, O., Kotantaki, P., Lakhani, A., et al. (2020). Mouse ovarian cancer models recapitulate the human tumor microenvironment and patient response to treatment. Cell Rep. 30 (2), 525–540.e7. doi:10.1016/j.celrep.2019.12.034
Meadows, K. L., and Hurwitz, H. I. (2012). Anti-VEGF therapies in the clinic. Cold Spring Harb. Perspect. Med. 2 (10), a006577. doi:10.1101/cshperspect.a006577
Meier, M. A., Nuciforo, S., Coto-Llerena, M., Gallon, J., Matter, M. S., Ercan, C., et al. (2022). Patient-derived tumor organoids for personalized medicine in a patient with rare hepatocellular carcinoma with neuroendocrine differentiation: a case report. Commun. Med. 2 (1), 80–88. doi:10.1038/s43856-022-00150-3
Meng, F., Evans, J. W., Bhupathi, D., Banica, M., Lan, L., Lorente, G., et al. (2012). Molecular and cellular Pharmacology of the hypoxia-activated prodrug TH-302. Mol. Cancer Ther. 11 (3), 740–751. doi:10.1158/1535-7163.mct-11-0634
Moon, C., VanDussen, K. L., Miyoshi, H., and Stappenbeck, T. S. (2014). Development of a primary mouse intestinal epithelial cell monolayer culture system to evaluate factors that modulate IgA transcytosis. Mucosal Immunol. 7 (4), 818–828. doi:10.1038/mi.2013.98
Munoz, J. L., Walker, N. D., Scotto, K. W., and Rameshwar, P. (2015). Temozolomide competes for P-glycoprotein and contributes to chemoresistance in glioblastoma cells. Cancer Lett. 367 (1), 69–75. doi:10.1016/j.canlet.2015.07.013
Nakasone, E. S., Askautrud, H. A., Kees, T., Park, J. H., Plaks, V., Ewald, A. J., et al. (2012). Imaging tumor-stroma interactions during chemotherapy reveals contributions of the microenvironment to resistance. Cancer Cell 21 (4), 488–503. doi:10.1016/j.ccr.2012.02.017
Neal, J. T., Li, X., Zhu, J., Giangarra, V., Grzeskowiak, C. L., Ju, J., et al. (2018). Organoid modeling of the tumor immune microenvironment. Cell 175 (7), 1972–1988.e16. doi:10.1016/j.cell.2018.11.021
Nikmaneshi, M. R., Firoozabadi, B., and Mozafari, A. (2021). Chemo-mechanistic multi-scale model of a three-dimensional tumor microenvironment to quantify the chemotherapy response of cancer. Biotechnol. Bioeng. 118 (10), 3871–3887. doi:10.1002/bit.27863
Nikmaneshi, M. R., Jain, R. K., and Munn, L. L. (2023). Computational simulations of tumor growth and treatment response: Benefits of high-frequency, low-dose drug regimens and concurrent vascular normalization. PLOS Comput. Biol. 19 (6), e1011131. doi:10.1371/journal.pcbi.1011131
Parrish, K. E., Pokorny, J., Mittapalli, R. K., Bakken, K., Sarkaria, J. N., and Elmquist, W. F. (2015). Efflux transporters at the blood-brain barrier Limit delivery and efficacy of cyclin-dependent kinase 4/6 inhibitor palbociclib (PD-0332991) in an orthotopic brain tumor model. J. Pharmacol. Exp. Ther. 355 (2), 264–271. doi:10.1124/jpet.115.228213
Ramos, A., Sadeghi, S., and Tabatabaeian, H. (2021). Battling chemoresistance in cancer: Root Causes and strategies to Uproot Them. Int. J. Mol. Sci. 22 (17), 9451. Published 2021 Aug 31. doi:10.3390/ijms22179451
Randles, A., Wirsching, H. G., Dean, J. A., Cheng, Y. K., Emerson, S., Pattwell, S. S., et al. (2021). Computational modelling of perivascular-niche dynamics for the optimization of treatment schedules for glioblastoma. Nat. Biomed. Eng. 5 (4), 346–359. doi:10.1038/s41551-021-00710-3
Ravi, V. M., Neidert, N., Will, P., Joseph, K., Maier, J. P., Kückelhaus, J., et al. (2022). T-cell dysfunction in the glioblastoma microenvironment is mediated by myeloid cells releasing interleukin-10. Nat. Commun. 13 (1), 925. doi:10.1038/s41467-022-28523-1
Sarnella, A., Ferrara, Y., Auletta, L., Albanese, S., Cerchia, L., Alterio, V., et al. (2022). Inhibition of carbonic anhydrases IX/XII by SLC-0111 boosts cisplatin effects in hampering head and neck squamous carcinoma cell growth and invasion. J. Exp. and Clin. Cancer Res. 41 (1), 122. doi:10.1186/s13046-022-02345-x
Seo, S., Nah, S. Y., Lee, K., Choi, N., and Kim, H. N. (2022). Triculture model of in vitro BBB and its Application to study BBB-associated chemosensitivity and drug delivery in glioblastoma. Adv. Funct. Mater. 32 (10), 2106860. doi:10.1002/adfm.202106860
Shafi, A. A., Schiewer, M. J., de Leeuw, R., Dylgjeri, E., McCue, P. A., Shah, N., et al. (2018). Patient-derived models reveal impact of the tumor microenvironment on therapeutic response. Eur. Urol. Oncol. 1 (4), 325–337. doi:10.1016/j.euo.2018.04.019
Straehla, J. P., Hajal, C., Safford, H. C., Offeddu, G. S., Boehnke, N., Dacoba, T. G., et al. (2022). A predictive microfluidic model of human glioblastoma to assess trafficking of blood–brain barrier-penetrant nanoparticles. Proc. Natl. Acad. Sci. U. S. A. 119 (23), e2118697119. doi:10.1073/pnas.2118697119
Supuran, C. T. (2020). Experimental carbonic anhydrase inhibitors for the treatment of hypoxic tumors. J. Exp. Pharmacol. 12, 603–617. doi:10.2147/jep.s265620
Tilsed, C. M., Fisher, S. A., Nowak, A. K., Lake, R. A., and Lesterhuis, W. J. (2022). Cancer chemotherapy: insights into cellular and tumor microenvironmental mechanisms of action. Front. Oncol. 12, 960317. doi:10.3389/fonc.2022.960317Available at: https://www.frontiersin.org/journals/oncology/articles/10.3389/fonc.2022.960317.
Touat, M., Li, Y. Y., Boynton, A. N., Spurr, L. F., Iorgulescu, J. B., Bohrson, C. L., et al. (2020). Mechanisms and therapeutic implications of hypermutation in gliomas. Nature 580 (7804), 517–523. doi:10.1038/s41586-020-2209-9
Wang, C., Sinha, S., Jiang, X., Murphy, L., Fitch, S., Wilson, C., et al. (2021). Matrix stiffness modulates patient-derived glioblastoma cell fates in three-dimensional hydrogels. Tissue Eng. Part A 27 (5–6), 390–401. doi:10.1089/ten.tea.2020.0110
Wu, H. J., Temko, D., Maliga, Z., Moreira, A. L., Sei, E., Minussi, D. C., et al. (2022). Spatial intra-tumor heterogeneity is associated with survival of lung adenocarcinoma patients. Cell Genomics 2 (8), 100165. doi:10.1016/j.xgen.2022.100165
Zhang, L., Zhao, J., Liang, C., Liu, M., Xu, F., and Wang, X. (2017). A novel biosensor based on intestinal 3D organoids for detecting the function of BCRP. Drug Deliv. 24 (1), 1453–1459. doi:10.1080/10717544.2017.1381199
Keywords: cancer, tissue engineering, lab-on-a-chip, resistance, recurrence, drug delivery, microfluidics, microenvironment
Citation: Garcia GG, Schmidt CJ and Hajal C (2024) The tumor microenvironment in therapy resistance. Front. Lab. Chip. Technol. 3:1420233. doi: 10.3389/frlct.2024.1420233
Received: 19 April 2024; Accepted: 23 August 2024;
Published: 10 September 2024.
Edited by:
Yuxiang Liu, Worcester Polytechnic Institute, United StatesReviewed by:
Zhiru Zhou, Vertex Pharmaceuticals (United States), United StatesCopyright © 2024 Garcia, Schmidt and Hajal. This is an open-access article distributed under the terms of the Creative Commons Attribution License (CC BY). The use, distribution or reproduction in other forums is permitted, provided the original author(s) and the copyright owner(s) are credited and that the original publication in this journal is cited, in accordance with accepted academic practice. No use, distribution or reproduction is permitted which does not comply with these terms.
*Correspondence: Cynthia Hajal, c.hajal@northeastern.edu