- 1Advanced Organ Bioengineering and Therapeutics, University of Twente, Enschede, Netherlands
- 2Applied Stem Cell Technologies, University of Twente, Enschede, Netherlands
- 3Organ-on-Chip Centre Twente, MESA+ Centre of Expertise, University of Twente, Enschede, Netherlands
Organs-on-chips (OoC) are in vitro models that emulate key functionalities of tissues or organs in a miniaturized and highly controlled manner. Due to their high versatility, OoC have evolved as promising alternatives to animal testing for a more effective drug development pipeline. Additionally, OoC are revealing increased predictive power for toxicity screening applications as well as (patho-) physiology research models. It is anticipated that enabling technologies such as biofabrication, multimodality imaging, and artificial intelligence will play a critical role in the development of the next generation of OoC. These domains are expected to increase the mimicry of the human micro-physiology and functionality, enhance screening of cellular events, and generate high-content data for improved prediction. Although exponentially growing, the OoC field will strongly benefit from standardized tools to upgrade its implementational power. The complexity derived from the integration of multiple technologies and the current absence of concrete guidelines for establishing standards may be the reason for the slower adoption of OoC by industry, despite the fast progress of the field. Therefore, we argue that it is essential to consider standardization early on when using new enabling technologies, and we provide examples to illustrate how to maintain a focus on technology standards as these new technologies are used to build innovative OoC applications. Moreover, we stress the importance of informed design, use, and analysis decisions. Finally, we argue that this early focus on standards in innovation for OoC will facilitate their implementation.
1 Introduction
Organs-on-chips (OoC) are microfluid-based physiologically inspired three-dimensional (3D) in vitro models that are designed to mimic the key functionality of tissues, organs, and/or organ systems. These complex miniaturized tools offer high control of several variables, including cellular and physico-chemical micro-environments. OoC hold great promise for the improvement of drug development/screening, toxicity assessment, disease modeling, and personalized medicine, overcoming the shortcomings of traditional in vitro and in vivo models at multiple levels (Leung et al., 2022).
Meanwhile, the rise of technologies such as biofabrication, multimodality imaging, and artificial intelligence (AI) is expected to offer opportunities for expanding the relevance and impact of OoC. This will enable a higher degree of (bio)mimicry of organ micro-physiology and functionality, screening for many events with cell-level resolution, and using the derived high-content data to integrate with or train AI-based algorithms (Moreira Teixeira and Mezzanotte, 2021; Deng et al., 2023; Tabatabaei Rezaei et al., 2023). In turn, the latter will lead to further amplification and extrapolation of the results in an automated, objective, and unbiased fashion. Moreover, these technology combinations are anticipated to accelerate the validation of results, thus increasing the power and significance of these platforms. Such ever-increasing possibilities will undoubtedly constitute fundamental building blocks for the development of the next generation of OoC. However, one of the challenges facing the field is the integration of such advanced technologies with the establishment of standards and guidelines (Piergiovanni et al., 2021a). Standardization is an essential and urgent step for steering OoC toward broader industrial adoption, as regulatory acceptance and qualification will enhance their significance in pharmaceutical research and applications (Figure 1).
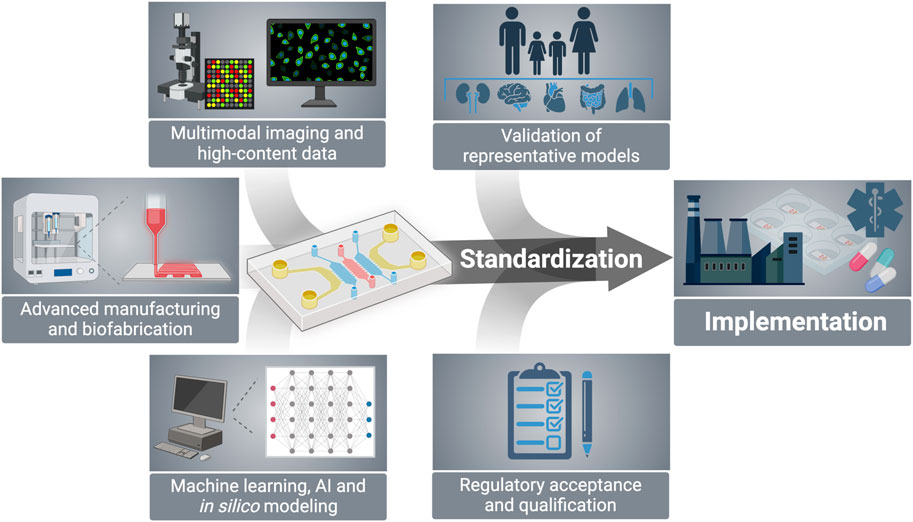
Figure 1. Emerging technologies including biofabrication, multimodal imaging, and AI modeling have the potential to expand the capabilities of OoC platforms, thus accelerating model validation and facilitating regulatory acceptance to ultimately lead to standardized tools adopted by main stakeholders in the drug development pipeline. Created with BioRender.com.
Therefore, we (i) provide an overview of recent advances in OoC design, biofabrication, and methods for output generation and automated analysis, (ii) emphasize the integration of large-scale data mining procedures coupled with AI-based algorithms as a tool that unveils a streamlined pathway to more swift and precise detection and screening protocols, and (iii) explore the balance between simplification, to drive standardization and complexification and to advance the functionalities of OoC. We thus provide a vision of the present and near-future expected developments, applications, and technologies for broader OoC implementation, facilitated by informed decisions on design, use, and analysis, rooted in guidelines and standards.
2 Next generation of organs-on-chips based on the convergence of advanced technologies
Despite increased investment in research and development over the past decade, drug development success rates reached a 10-year low of 6.3% in 2022 (Aitken et al., 2023). Such a low success rate can be partially explained by failure to replicate human disease features in pre-clinical animal models, which often lead to approved clinical trials with harmful or ineffective drug candidates (Loewa et al., 2023). Thus, the need for models with improved biomimicry and predictive value has shifted attention from conventional animal studies to complex human-based in vitro models. To reproduce the inherent complexity of the human body, the convergence of current OoC models with emerging technologies provides high-content data for better informed decisions in the drug development pipeline. In this section, we will highlight recent advances and efforts in the combination of bioprinting and multimodal imaging with OoC.
2.1 Bioprinting strategies and increased cellular complexity
Bioprinting generally refers to the controlled, layer-by-layer deposition of cells, biomaterials, scaffolds, and growth factors to form complex structures with a desired pore size and interconnectivity. Different biomaterials and cells can be simultaneously combined and printed to replicate the native distribution of distinct cell types and biochemical cues across three-dimensional (3D) space (Tabatabaei Rezaei et al., 2023). Additional control over the construct structure can be achieved by the inclusion of stimuli-responsive biomaterials and/or cells to induce conformational changes in the printed tissue, even after the printing process is finished, in the process of four-dimensional (4D) bioprinting (Osouli-Bostanabad et al., 2022). Scientific and industrial interest in 3D/4D bioprinting has increased considerably in recent years: the 3D bioprinting market size reached $2 billion in 2022 and is expected to grow at an annual growth rate of 12.5% until 2030 (Research G. V, 2023). Nevertheless, traditional 3D/4D bioprinting still has limitations. Current bioprinted constructs are not suited for high-content screening applications due to their relative complexity. In addition, most bioprinted structures are cultured in static conditions which result in uneven nutrient and oxygen diffusion throughout the entire construct.
By converging bioprinting technology with OoC platforms, the precise distribution of different cell types on physiologically relevant extracellular matrices can be achieved to maximize biomimicry, while allowing for fine control of biophysical culture parameters (Chliara et al., 2022). Bioprinting and microfluidic devices have been combined to establish a variety of humanized models, including tracheal (Park et al., 2018), liver (Lee et al., 2020), tumor (Yi et al., 2019), vascular (Zhang et al., 2016a), myocardium (Zhang et al., 2016b), kidney (Homan et al., 2016), lung (Kim et al., 2023), and placenta (Mandt et al., 1970) models. In addition to adjusted biomaterial complexity and spatial organization for the reproduction of organ features, the use of patient-specific primary cells is advantageous for capturing the heterogeneity of disease progression, as often observed in clinical settings (Loewa et al., 2023). These exciting possibilities have interested both academia and industry in applications ranging from disease modeling to personalized medicine and drug screening (Tabatabaei Rezaei et al., 2023). Nonetheless, key issues still need to be addressed before these methods will find widespread adoption. Developing bioinks with appropriate mechanical properties for printability while also keeping adequate biochemical cues for cell survival and differentiation, specific to each organ, is not trivial. In addition, bioprinting hardware and processes need to be adapted to increase compatibility with OoC devices, and printing resolution is not sufficient for the development of complex vascular networks similar in vivo observation. Another significant challenge concerns scalability and throughput since the manufacture of bioprinted, biomimetic structures in OoC is still costly and time-consuming. To that end, improving device monitoring and maintenance by coupling advanced imaging techniques with sensors could be crucial to achieving meticulous control of the bioprinting process. For instance, real-time monitoring would allow the detection of any irregularity or deviation from the desired bioprinted structure. Simultaneously, integrating sensors could provide optimal conditions over extended periods, ensuring the reproducibility and reliability of bioprinted tissues. Overall, the synergy of advanced imaging techniques and sensor technologies may be fundamental to paving the way for high-throughput applications, which will be the focus of the following section.
2.2 Resolving high-content data from multi-sensors and imaging modalities
In the last decade, there has been significant progress in multidisciplinary research on manufacturing, microelectronics, and their application in the life sciences (Zhou et al., 2023). These have had a remarkable impact on OoC development since the design flexibility of microfluidic chips can now be further complemented with analytical methods capable of probing small sample volumes or even single-cell information (Pal et al., 2023). The integration of a multitude of detection devices, such as electrodes and optics, in microfluidic chips allows time-resolved analysis of environmental factors for accurately monitoring cell culture state.
Imaging is one of the main outputs of OoC models, owing to the outstanding optical access to cell culture chambers and perfusion channels. The on-chip quantification of cell dynamics and interactions is thus facilitated and could be instrumental for progressing the state of the art in disease modeling and drug development. Several imaging modalities have been successfully applied to microfluidic systems, ranging from standard brightfield and fluorescent imaging (Mencattini et al., 2022; Habibey, 2023), multiphoton imaging (Mazzarda et al., 2020; Lohasz et al., 2021), calcium signaling detection (Mazzarda et al., 2020), bioluminescence imaging (Araújo-Gomes et al., 2023), and photoacoustic (Jin et al., 2021) and light sheet microscopy (Fan et al., 2021). In addition, the miniaturization of imaging chambers and channels presents significant advantages: i) precise molecule manipulation, ii) control over the physicochemical properties of the microenvironment, iii) higher sample concentration (Miyake et al., 2008), and iv) increased signal-to-noise ratio (Campos et al., 2011). Microfluidic systems can thus be made suitable for single-molecule imaging modalities such as fluorescent resonance energy transfer (FRET) and total internal reflection fluorescence (TIRF) (Colson et al., 2023).
In addition to imaging outputs, sensor units can be accommodated in OoC platforms for on-chip, online monitoring of dynamic tissue responses. In particular, fluidic integration and perfusion is not only advantageous for modeling drug bioavailability and diffusion into 3D engineered tissues, but seamless fluidic connection between cell and assay compartments offers unparalleled access to cellular states in real-time. The rapid detection of metabolite release, changes in pH and oxygen levels, and the determination of epithelial integrity is of particular importance for toxicology screening, enabling the assessment of drug response over time (Dornhof et al., 2022). Microfluidic sensors can also be potential alternatives for standard enzyme-linked immunosorbent assays (ELISA) by increasing detection limits and circumventing the need for high sample volumes (Shin et al., 2017). Potential applications of such systems include, but are not limited to, the assessment of drug-induced kidney (Kann et al., 2023), cardiac (Shin et al., 2016; Tanumihardja et al., 2021), gut (Khalid et al., 2022) and liver (Busche et al., 2022) toxicity, screening oncology drug efficacy screenings (Dornhof et al., 2022), and electrophysiology measurements (Habibey, 2023).
Despite significant advances, the integration of sensing technologies into OoC still has some challenges. For instance, the limit of detection (LOD) for some sensors may not be appropriate for low-concentration analytes, possibly affecting the sensitivity and specificity of assays (Sabaté del Río et al., 2023). Another significant challenge regards sensor stability over time, which may be compromised by chemical degradation or passivation due to biofouling (Sabaté del Río et al., 2023). Overall, these challenges highlight the continuous need for innovative solutions and approaches to balance simplification and the integration of advanced technologies in current industrial and pre-clinical applications.
3 AI as a tool for improved high content analytics
The integration of AI techniques, particularly machine learning (ML) and deep learning (DL) algorithms, has emerged as a pivotal strategy for analyzing high-throughput data derived from OoC platforms. The inherent complexity of these data requires innovative approaches for its analysis and interpretation. In this section, we discuss how AI can play a transformative role in addressing OoC-derived imaging data and offer a novel pathway toward standardization.
3.1 Integration of ML and DL models in OoC platforms
Establishing a robust OoC-AI connection is not merely a choice but a fundamental necessity for expediting the processes of drug development/screening, toxicity assessment, and disease modeling. OoC-derived imaging data serve as an illustrative case study, exemplifying the transformative power of AI in specific domains—particularly cell segmentation, tracking, and classification (Figure 2).
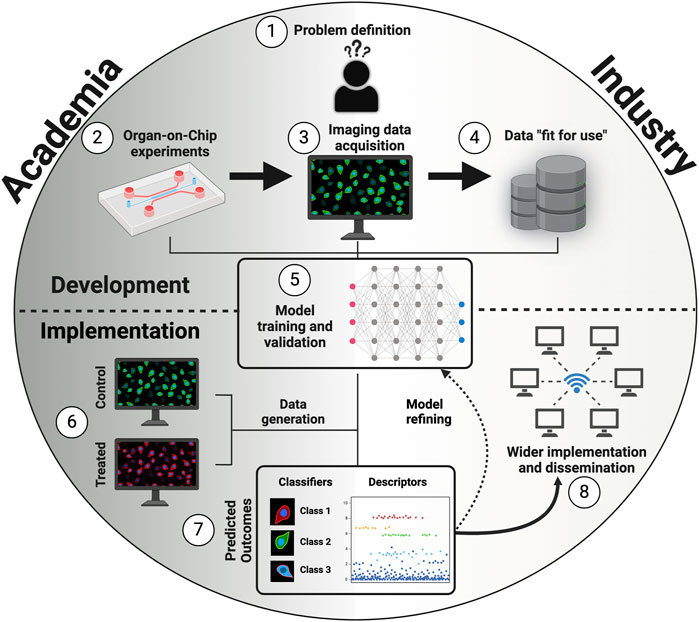
Figure 2. Building blocks to standard integration of AI-based models into OoC platforms. 1) Problem definition. 2) Optimization of OoC experiments, including OoC design, cell sources, and perfusing media. 3) Imaging data acquisition with consistent sample preparation, constant light/exposure conditions, and same magnification settings. 4) Adjust to data “fit-for-use” for AI-based models by performing data pre-processing techniques, data splitting, and adding metadata. 5) Training and validation of an explainable, interpretable, and unbiased AI-based model. 6) Generate new data of control and treated cells to test the previously developed model. 7) Analyze the predicted outcomes and refine the model according to suggestions from both academic and industry stakeholders. 8) Implement and disseminate the model. Created with BioRender.com.
Within this context, classical image segmentation techniques, such as the Otsu and Watershed methods, have long been employed for straightforward image data segmentation and are particularly useful when dealing with well-defined structures (Kumar et al., 2020). Moreover, conventional ML algorithms, such as random forest and logistic regression, can incorporate contextual information and learning from data, making it possible to automate the segmentation of images with distinguishable intensity values and textures (Oliver et al., 2019). Nonetheless, such techniques tend to decrease their performance when applied to complex image data or when faced with smooth variations in intensity and textures.
DL techniques such as convolutional neural networks (CNNs) are specifically designed for image processing, rendering them essential for interpreting imaging data in OoC experiments (Ronneberger et al., 2015). Notably, DL typically requires extensive labeled data and substantial computational resources, which can be a practical limitation. Thus, the AI technique should be carefully selected considering the interplay between data complexity and available resources.
In addition to CNNs, groundbreaking DL algorithms such as You Only Look Once (YOLO) (Jiang et al., 2022) and the Segment Anything Model (SAM) (Kirillov et al., 2023) are becoming widely used by the scientific community in object detection and segmentation, respectively. YOLO’s ability to process real-time object identification and SAM’s adaptability and versatility in segmenting diverse types of objects in slightly different image conditions might represent significant advances in the analysis of OoC-derived high-content data.
From a different point of view, the integration of Reinforcement Learning (RL) techniques (Sutton and Barto, 2018)—an algorithm that harnesses the power of interacting with the data through trial and error—might lead to a more autonomous and standalone OoC platform. For instance, an RL-based OoC platform to detect cells could reward every cell correctly identified and penalize every cell inappropriately identified, allowing for continuous improvement of the platform.
3.2 AI-based algorithms with specific use for OoC models
One of the first remarkable approaches for applying AI-based models, specifically CNNs, to OoC-derived data was proposed by Mencattini et al. (2020). In localizing and tracking breast cancer cells (BT474 cell line) and prostate cancer cells (PC-3 cell line), they demonstrated an outstanding ability to classify the cells in categories of treated versus non-treated conditions (average accuracy of 91.5%).
Recently, Paek et al. (2023) developed a high-throughput biomimetic bone-on-chip assisted by a CNN for osteoporosis drug testing. Using this model, they took advantage of several hundred images per drug-treated and non-treated group to train and validate a CNN model, thus demonstrating an outstanding ability to categorize different cell types and groups (average accuracy of around 98%).
Therefore, the following measures are recommendations to researchers to achieve images compatible with fit-for-use AI available tools: i) develop consistent sample preparation protocols for microscopy images, ii) seek a homogeneous background, iii) reduce object density to the minimum possible (e.g., cell number per unit area), iv) use constant light or laser exposure, and, v) use the same magnification settings in all acquisitions.
4 Towards implementation of standardization modalities in OoC
Standards are norms, patterns, or models used for comparative evaluations, typically established by standards developing organizations which guarantee that products, services, and processes are fit for purpose. They ensure product functionality and interoperability and facilitate the design and enforcement of legislation by policymakers (Piergiovanni et al., 2021b). Since OoC models are fundamentally interdisciplinary, the standardization of this technology is challenging. Several initiatives, together with relevant stakeholders in the OoC field, have identified that standardization is needed to advance the OoC field and have supported regulatory acceptance and qualification. In 2019, a strategy workshop on standards from the Organ-on-Chip In Development (ORCHID) project brought together OoC developers, end-users, and regulators to formulate an outlook and recommendations (Mastrangeli et al., 2019).
Thence, a 2021 workshop organized by the European Committee for Standardization (CEN) and the European Committee for Electrotechnical Standardization (CENELEC) gathered experts from relevant stakeholder institutions and further explored the issues of the current OoC landscape, as well as proposing priorities for successful regulatory acceptance (Piergiovanni et al., 2021a; Piergiovanni et al., 2021b). For instance, a consensus on OoC definitions and classification was identified as a priority to initiate the qualification of OoC models. Device material standardization is also seen as a priority since the issue of the molecule adsorption of PDMS has still not been uniformly addressed (Piergiovanni et al., 2021a). In addition, in order to facilitate regulatory acceptance, the building of standardized lists of assays/endpoints and reference compounds for specific context of uses will help quantify the predictive value of an OoC model and its applicability domain (Piergiovanni et al., 2021a). Following the workshop, CEN/CENELEC established a focus group on OoC with the aim of developing a roadmap for standardizing OoC, identifying issues linked to European legislation, and promoting crosstalk with different innovation projects that impact the OoC field (CEN/CENELEC, 2021).
Similar initiatives are ongoing in the United States (US). The Tissue Chip for Drug Screening program has been set up by the National Institutes of Health Center for Advancing Translational Sciences, in collaboration with the US Food and Drug Administration and the Defense Advanced Research Projects Agency (NCATS, 2024). Public–private agreements were established to progress OoC development and move their acceptance and implementation forward (Fitzpatrick and Sprando, 2019). Standardization efforts should thus be coordinated internationally to avoid competition between US and European partners (Mastrangeli et al., 2019).
Previous successful examples from the electronics industry for standardizing data communication protocols and compatibility across peripherals and interfaces should be regarded as important learning experiences (Mastrangeli et al., 2019). Improved compatibility between different OoC devices is crucial since no single model can satisfy the requirements of all applications in the field. Instead, a modular approach was proposed whereby standardized and simplified OoC modules could be assembled according to end-user needs (Mastrangeli et al., 2019). Hence, a new standard specifying the requirements for the integration of different microfluidic components and systems was published (ISO 22916:2022). This standard prompted the development of a multi-institutional approach that developed the Translational Organ-on-Chip Platform (TOP). TOP employs a fluidic circuit board that serves as a microfluidic platform that connects several independent modules via standardized interfacing (Vivas et al., 2022). This approach has already been applied to cardiac and vascular OoC models (de Graaf et al., 2022; Vivas et al., 2022; de Graaf et al., 2023). Another important component of TOP is the inclusion of accessible software able to integrate control commands and sensor interfaces (de Graaf et al., 2022). Data analysis could be improved by the inclusion of AI algorithms. However, no guidelines for AI use in OoC systems are yet available.
Experts recognize that standardization must be addressed early during OoC development and should be a community-driven process to accelerate innovation. Among several standardization modalities identified are i) materials, ii) dimensions, iii) flow rates, iv) interconnections and interfaces, and v) cell sources and protocols for cell differentiation (Mastrangeli et al., 2019). These modalities can also be clustered into device characteristics and classification. Overall performance should consider technical and biological perspectives and, importantly, terminology.
5 Conclusion and prospects
The drug development pipeline is clearly suboptimal, with only 10% of newly developed drug candidates being approved for clinical use. This is highly concerning, not only for drug developers but also for regulators, and, most importantly, for patients (Hay et al., 2014). The lack of effectiveness in this process is partially due to the low predictive power of the models used in preclinical evaluation, including animal models. OoC are receiving increasing attention as they are proving their value in achieving a more robust and efficient framework for drug development and are contributing to a deeper understanding of human (patho-) physiology. The fast development of OoC has resulted in the conversion of advanced technologies, with the overall goal of mimicking native human organ or tissue functions in all their complexity with accompanying advanced methodologies for analysis or full use of the data derived. The ultimate collective aim is to increase their predictive power, thus accelerating disease research and therapeutic discovery. Nevertheless, a more extensive clinical application is hindered by the current lack of thorough qualification processes, which may include standardization and benchmarking against accepted references and/or models (Piergiovanni et al., 2021a).
On the quest to achieve increasingly higher mimicry of human tissues and/or organs with sufficient complexity, the field of OoC is synergistically evolving with other advanced technologies. In coming years, it is expected that standardization will impact the field of bioprinting in OoC at the following levels: i) nomenclature and classification of bioprinters and bioprinted constructs; ii) standardized bioink composition, viscosity, printability and cytocompatibility for each specific application that are fit for purpose; iii) sterilization methods for bioprinting components; iv) software and workflows; v) bioprinting hardware and interfaces with OoC devices; vi) test methods and quality control. Currently, standards for test methods of bioinks for extrusion-based bioprinting are being proposed, but other bioprinting modalities still lack adequate standards (Grijalva Garces et al., 2024). Accelerating standard development should facilitate the adoption of bioprinted OoC models in both academia and industry, as well as improve regulatory acceptance of these models. With this in mind, the development of novel bioprinting constructs coupled with OoC devices should be concomitant with the ample characterization of bioinks and hardware and analysis workflows and be shared by multiple independent laboratories to confirm robustness and reproducibility (Mladenovska et al., 2023). Finally, output generation and data collection from such high-content models needs to be standardized so that the performance of the different models can be assessed and compared to currently accepted references. Although the scientific development of imaging tools and sensors for OoC is expected to rise in coming years, several shortcomings still hamper their adoption in drug development pipelines. For instance, while stage-top incubators for environmental control during image acquisition are already common in modern microscopes, most OoC models use in-house hardware setup with nonstandard dimensions and/or materials—potentially incompatible with existing industrial infrastructure (Mazzarda et al., 2020). These devices often require manual assembly processes and are likely overly complex and bulky with difficult operability. Additionally, standardized biomarkers and/or assays for sensors and imaging tools used in OoC should be selected depending on the application in a fit-for-purpose manner. As an example, urea and albumin are biomarkers that reflect the functionality of hepatocytes and are extensively characterized in both research and clinical settings (Baudy et al., 2020). Developing sensors to automatically monitor urea and albumin for liver-on-chip devices would be crucial for toxicology screenings and would help build confidence in OoC platforms.
Altogether, establishing guidelines to achieve a standard design interface for seamless integration with various analysis systems might enhance interoperability between different OoC devices and facilitate their adoption in industrial settings where reliable and consistent data collection is essential. Regarding device fabrication, polydimethylsiloxane (PDMS) is mostly used for prototyping in academic settings due to its biological compatibility, gas permeability, optical clearance, and mechanical properties. However, the adsorption of small organic molecules remains a considerable issue that limits its wide applicability in drug testing workflows (Mukhopadhyay, 2007). Additionally, although the implementation of high-content/throughput screening tools for OoC has the potential to revolutionize the pharmaceutical industry, the nature and sheer volume of generated data makes its analysis and interpretation cumbersome (Zhou et al., 2023). The application of AI algorithms in OoC, although still in its infancy, could play a role in standardizing these tools. To this end, and to seamlessly integrate AI-based tools into OoC platforms, the quality and quantity of imaging data must be prioritized. To obtain “fit-for-use” data, we recommend leveraging the findability, accessibility, interoperability, and reusability (FAIR) data principles, as consistency in imaging and experimental conditions is paramount to ensuring the effectiveness of AI-driven analysis. Regarding the training, validation, and testing of custom AI-based models, we recommend starting with a simple research question that requires a small number of training instances and then increasing the complexity as needed. Moreover, we consider it fundamental to add metadata to the experiments, ensuring that essential information accompanying the data is structured and adheres to universally understandable formats. Altogether, as OoC technology continues to advance, the collaboration between researchers from various domains will be instrumental in harnessing the full potential of AI in driving standardization and innovation within the field.
Undoubtedly, the next generation of OoC will be the result of a seamless integration of technological advancements in microfabrication, bioengineering, sensors, imaging, and digital tools. However, to live up to its promise to advance the field of drug development, adoption of these technologies will require the establishment of standardization priorities.
Author contributions
JM: writing–original draft and writing–review and editing. FC: writing–original draft and writing–review and editing. AM: writing–original draft and writing–review and editing. SW: writing–original draft and writing–review and editing. LT: conceptualization, funding acquisition, writing–original draft, and writing–review and editing.
Funding
The author(s) declare financial support was received for the research, authorship, and/or publication of this article. This work was supported by the research program Incentive Grants for Women in STEM with project number 18741, and Aspasia grant with the project number 015.017.042, financed by the Dutch Research Council (NWO, Nederlandse Organisatie voor Wetenschappelijk Onderzoek).
Conflict of interest
The authors declare that the research was conducted in the absence of any commercial or financial relationships that could be construed as a potential conflict of interest.
The author(s) declared that they were an editorial board member of Frontiers at the time of submission. This had no impact on the peer review process and the final decision.
Publisher’s note
All claims expressed in this article are solely those of the authors and do not necessarily represent those of their affiliated organizations, or those of the publisher, the editors, and the reviewers. Any product that may be evaluated in this article, or claim that may be made by its manufacturer, is not guaranteed or endorsed by the publisher.
References
Aitken, M., Connelly, N., Kleinrock, M., and Pritchett, J. (2023). Global trends in R&D 2023: activity, productivity, and enablers. Durham, North Carolina, United States: IQVIA Institute for Human Data Science.
Araújo-Gomes, N., Zambito, G., Johnbosco, C., Calejo, I., Leijten, J., Löwik, C., et al. (2023). Bioluminescence imaging on-chip platforms for non-invasive high-content bioimaging. Biosens. Bioelectron. 237, 115510. doi:10.1016/j.bios.2023.115510
Baudy, A. R., Otieno, M. A., Hewitt, P., Gan, J., Roth, A., Keller, D., et al. (2020). Liver microphysiological systems development guidelines for safety risk assessment in the pharmaceutical industry. Lab a Chip 20, 215–225. doi:10.1039/C9LC00768G
Busche, M., Rabl, D., Fischer, J., Schmees, C., Mayr, T., Gebhardt, R., et al. (2022). Continous, non-invasive monitoring of oxygen consumption in a parallelized microfluidic in vitro system provides novel insight into the response to nutrients and drugs of primary human hepatocytes. EXCLI J. 21, 144–161. doi:10.17179/excli2021-4351
Campos, L. A., Liu, J., Wang, X., Ramanathan, R., English, D. S., and Muñoz, V. (2011). A photoprotection strategy for microsecond-resolution single-molecule fluorescence spectroscopy. Nat. Methods 8, 143–146. doi:10.1038/nmeth.1553
CEN/CENELEC (2021). Focus group on organ on chip. Available at: https://www.cencenelec.eu/areas-of-work/cen-cenelec-topics/organ-on-chip/.
Chliara, M. A., Elezoglou, S., and Zergioti, I. (2022). Bioprinting on organ-on-chip: development and applications. Biosensors 12, 1135. doi:10.3390/bios12121135
Colson, L., Kwon, Y., Nam, S., Bhandari, A., Maya, N. M., Lu, Y., et al. (2023). Trends in single-molecule total internal reflection fluorescence imaging and their biological applications with lab-on-a-chip technology. Sensors 23, 7691. doi:10.3390/s23187691
de Graaf, M. N. S., Vivas, A., Kasi, D. G., van den Hil, F. E., van den Berg, A., van der Meer, A. D., et al. (2023). Multiplexed fluidic circuit board for controlled perfusion of 3D blood vessels-on-a-chip. Lab a Chip 23, 168–181. doi:10.1039/D2LC00686C
de Graaf, M. N. S., Vivas, A., van der Meer, A. D., Mummery, C. L., and Orlova, V. V. (2022). Pressure-driven perfusion system to control, multiplex and recirculate cell culture medium for organs-on-chips. Micromachines 13, 1359. doi:10.3390/mi13081359
Deng, S., Li, C., Cao, J., Cui, Z., Du, J., Fu, Z., et al. (2023). Organ-on-a-chip meets artificial intelligence in drug evaluation. Theranostics 13, 4526–4558. doi:10.7150/thno.87266
Dornhof, J., Kieninger, J., Muralidharan, H., Maurer, J., Urban, G. A., and Weltin, A. (2022). Microfluidic organ-on-chip system for multi-analyte monitoring of metabolites in 3D cell cultures. Lab a Chip 22, 225–239. doi:10.1039/D1LC00689D
Fan, Y.-J., Hsieh, H. Y., Tsai, S. F., Wu, C. H., Lee, C. M., Liu, Y. T., et al. (2021). Microfluidic channel integrated with a lattice lightsheet microscopic system for continuous cell imaging. Lab a Chip 21, 344–354. doi:10.1039/D0LC01009J
Fitzpatrick, S., and Sprando, R. (2019). Advancing regulatory science through innovation: in vitro microphysiological systems. Cell. Mol. Gastroenterology Hepatology 7, 239–240. doi:10.1016/j.jcmgh.2018.08.004
Grijalva Garces, D., Strauß, S., Gretzinger, S., Schmieg, B., Jüngst, T., Groll, J., et al. (2024). On the reproducibility of extrusion-based bioprinting: round robin study on standardization in the field. Biofabrication 16, 015002. doi:10.1088/1758-5090/acfe3b
Habibey, R. (2023). Incubator-independent perfusion system integrated with microfluidic device for continuous electrophysiology and microscopy readouts. Biofabrication 15, 024102. doi:10.1088/1758-5090/acb466
Hay, M., Thomas, D. W., Craighead, J. L., Economides, C., and Rosenthal, J. (2014). Clinical development success rates for investigational drugs. Nat. Biotechnol. 32, 40–51. doi:10.1038/nbt.2786
Homan, K. A., Kolesky, D. B., Skylar-Scott, M. A., Herrmann, J., Obuobi, H., Moisan, A., et al. (2016). Bioprinting of 3D convoluted renal proximal tubules on perfusable chips. Sci. Rep. 6, 34845. doi:10.1038/srep34845
Jiang, P., Ergu, D., Liu, F., Cai, Y., and Ma, B. (2022). A review of yolo algorithm developments. Procedia Comput. Sci. 199, 1066–1073. doi:10.1016/j.procs.2022.01.135
Jin, T., Zhang, C., Liu, F., Chen, X., Liang, G., Ren, F., et al. (2021). On-chip multicolor photoacoustic imaging flow cytometry. Anal. Chem. 93, 8134–8142. doi:10.1021/acs.analchem.0c05218
Kann, S. H., Shaughnessey, E. M., Zhang, X., Charest, J. L., and Vedula, E. M. (2023). Steady-state monitoring of oxygen in a high-throughput organ-on-chip platform enables rapid and non-invasive assessment of drug-induced nephrotoxicity. Analyst 148, 3204–3216. doi:10.1039/D3AN00380A
Khalid, M. A. U., Kim, K. H., Chethikkattuveli Salih, A. R., Hyun, K., Park, S. H., Kang, B., et al. (2022). High performance inkjet printed embedded electrochemical sensors for monitoring hypoxia in a gut bilayer microfluidic chip. Lab a Chip 22, 1764–1778. doi:10.1039/D1LC01079D
Kim, W., Lee, Y., Kang, D., Kwak, T., Lee, H. R., and Jung, S. (2023). 3D inkjet-bioprinted lung-on-a-chip. ACS biomaterials Sci. Eng. 9, 2806–2815. doi:10.1021/acsbiomaterials.3c00089
Kirillov, A., Mintun, E., Ravi, N., Mao, H., Rolland, C., Gustafson, L., et al. (2023). “Segment anything,” in Proceedings of the IEEE/CVF International Conference on Computer Vision (ICCV), 4015–4026.
Kumar, N., Verma, R., Anand, D., Zhou, Y., Onder, O. F., Tsougenis, E., et al. (2020). A multi-organ nucleus segmentation challenge. IEEE Trans. Med. imaging 39, 1380–1391. doi:10.1109/tmi.2019.2947628
Lee, H., Kim, J., Choi, Y., and Cho, D.-W. (2020). Application of gelatin bioinks and cell-printing technology to enhance cell delivery capability for 3D liver fibrosis-on-a-chip development. ACS biomaterials Sci. Eng. 6, 2469–2477. doi:10.1021/acsbiomaterials.9b01735
Leung, C. M., de Haan, P., Ronaldson-Bouchard, K., Kim, G. A., Ko, J., Rho, H. S., et al. (2022). A guide to the organ-on-a-chip. Nat. Rev. Methods Prim. 2, 33. doi:10.1038/s43586-022-00118-6
Loewa, A., Feng, J. J., and Hedtrich, S. (2023). Human disease models in drug development. Nat. Rev. Bioeng. 1, 545–559. doi:10.1038/s44222-023-00063-3
Lohasz, C., Loretan, J., Sterker, D., Görlach, E., Renggli, K., Argast, P., et al. (2021). A microphysiological cell-culturing system for pharmacokinetic drug exposure and high-resolution imaging of arrays of 3D microtissues. Front. Pharmacol. 12, 785851. doi:10.3389/fphar.2021.785851
Mandt, D., Gruber, P., Markovic, M., Tromayer, M., Rothbauer, M., Kratz, S. R. A., et al. (1970). Fabrication of biomimetic placental barrier structures within a microfluidic device utilizing two-photon polymerization. Int. J. bioprinting 4, 144. doi:10.18063/IJB.v4i2.144
Mastrangeli, M., Millet, S., Mummery, C., Loskill, P., Braeken, D., Eberle, W., et al. (2019). Building blocks for a European Organ-on-Chip roadmap. ALTEX - Altern. animal Exp. 36, 481–492. doi:10.14573/altex.1905221
Mazzarda, F., D'Elia, A., Massari, R., De Ninno, A., Bertani, F. R., Businaro, L., et al. (2020). Organ-on-chip model shows that ATP release through connexin hemichannels drives spontaneous Ca2+ signaling in non-sensory cells of the greater epithelial ridge in the developing cochlea. Lab a Chip 20, 3011–3023. doi:10.1039/D0LC00427H
Mencattini, A., Di Giuseppe, D., Comes, M. C., Casti, P., Corsi, F., Bertani, F. R., et al. (2020). Discovering the hidden messages within cell trajectories using a deep learning approach for in vitro evaluation of cancer drug treatments. Sci. Rep. 10, 7653. doi:10.1038/s41598-020-64246-3
Mencattini, A., Lansche, C., Veith, I., Erbs, P., Balloul, J. M., Quemeneur, E., et al. (2022). Direct imaging and automatic analysis in tumor-on-chip reveal cooperative antitumoral activity of immune cells and oncolytic vaccinia virus. Biosens. Bioelectron. 215, 114571. doi:10.1016/j.bios.2022.114571
Miyake, T., Tanii, T., Sonobe, H., Akahori, R., Shimamoto, N., Ueno, T., et al. (2008). Real-time imaging of single-molecule fluorescence with a zero-mode waveguide for the analysis of Protein−Protein interaction. Anal. Chem. 80, 6018–6022. doi:10.1021/ac800726g
Mladenovska, T., Choong, P. F., Wallace, G. G., and O'Connell, C. D. (2023). The regulatory challenge of 3D bioprinting. Regen. Med. 18, 659–674. doi:10.2217/rme-2022-0194
Moreira Teixeira, L., and Mezzanotte, L. (2021). New bioimaging avenues for organs-on-chips by integration of bioluminescence. VIEW 2, 20200177. doi:10.1002/VIW.20200177
NCATS (2024). Tissue chip for drug screening. Bethesda, Maryland: National Center for Advancing Translational Sciences.
Oliver, C. R., Altemus, M. A., Westerhof, T. M., Cheriyan, H., Cheng, X., Dziubinski, M., et al. (2019). A platform for artificial intelligence based identification of the extravasation potential of cancer cells into the brain metastatic niche. Lab a Chip 19, 1162–1173. doi:10.1039/c8lc01387j
Osouli-Bostanabad, K., Masalehdan, T., Kapsa, R. M. I., Quigley, A., Lalatsa, A., Bruggeman, K. F., et al. (2022). Traction of 3D and 4D printing in the healthcare industry: from drug delivery and analysis to regenerative medicine. ACS biomaterials Sci. Eng. 8, 2764–2797. doi:10.1021/acsbiomaterials.2c00094
Paek, K., Kim, S., Tak, S., Kim, M. K., Park, J., Chung, S., et al. (2023). A high-throughput biomimetic bone-on-a-chip platform with artificial intelligence-assisted image analysis for osteoporosis drug testing. Bioeng. Transl. Med. 8, e10313. doi:10.1002/btm2.10313
Pal, A., Kaswan, K., Barman, S. R., Lin, Y. Z., Chung, J. H., Sharma, M. K., et al. (2023). Microfluidic nanodevices for drug sensing and screening applications. Biosens. Bioelectron. 219, 114783. doi:10.1016/j.bios.2022.114783
Park, J. Y., Ryu, H., Lee, B., Ha, D. H., Ahn, M., Kim, S., et al. (2018). Development of a functional airway-on-a-chip by 3D cell printing. Biofabrication 11, 015002. doi:10.1088/1758-5090/aae545
Piergiovanni, M., Cangar, O., Leite, S. B., Mian, L., Jenet, A., Corvi, R., et al. (2021b). Putting Science into Standards workshop on standards for organ-on-chip. Stem Cell Rep. 16, 2076–2077. doi:10.1016/j.stemcr.2021.07.010
Piergiovanni, M., Leite, S. B., Corvi, R., and Whelan, M. (2021a). Standardisation needs for organ on chip devices. Lab a Chip 21, 2857–2868. doi:10.1039/D1LC00241D
Research G. V (2023). 3D bioprinting market size, share and trends analysis report by technology (magnetic levitation, inkjet-based), by application (medical, dental, biosensors, bioinks), by region, and segment forecasts, 2023 - 2030. 3D Bioprinting.
Ronneberger, O., Fischer, P., and Brox, T. (2015). “Medical image computing and computer-assisted intervention–MICCAI 2015,” in 18th International Conference, Munich, Germany, October, 2015.
Sabaté del Río, J., Ro, J., Yoon, H., Park, T.-E., and Cho, Y.-K. (2023). Integrated technologies for continuous monitoring of organs-on-chips: current challenges and potential solutions. Biosens. Bioelectron. 224, 115057. doi:10.1016/j.bios.2022.115057
Shin, S. R., Kilic, T., Zhang, Y. S., Avci, H., Hu, N., Kim, D., et al. (2017). Label-Free and regenerative electrochemical microfluidic biosensors for continual monitoring of cell secretomes. Adv. Sci. Weinheim, Baden-Wurttemberg, Ger. 4, 1600522. doi:10.1002/advs.201600522
Shin, S. R., Zhang, Y. S., Kim, D. J., Manbohi, A., Avci, H., Silvestri, A., et al. (2016). Aptamer-based microfluidic electrochemical biosensor for monitoring cell-secreted trace cardiac biomarkers. Anal. Chem. 88, 10019–10027. doi:10.1021/acs.analchem.6b02028
Sutton, R. S., and Barto, A. G. (2018). Reinforcement learning: an introduction. St Bradford, PA: A Bradford Book.
Tabatabaei Rezaei, N., Kumar, H., Liu, H., Lee, S. S., Park, S. S., and Kim, K. (2023). Recent advances in organ-on-chips integrated with bioprinting technologies for drug screening. Adv. Healthc. Mater. 12, 2203172. doi:10.1002/adhm.202203172
Tanumihardja, E., Slaats, R. H., van der Meer, A. D., Passier, R., Olthuis, W., and van den Berg, A. (2021). Measuring both pH and O2 with a single on-chip sensor in cultures of human pluripotent stem cell-derived cardiomyocytes to track induced changes in cellular metabolism. ACS Sensors 6, 267–274. doi:10.1021/acssensors.0c02282
Vivas, A., van den Berg, A., Passier, R., Odijk, M., and van der Meer, A. D. (2022). Fluidic circuit board with modular sensor and valves enables stand-alone, tubeless microfluidic flow control in organs-on-chips. Lab a Chip 22, 1231–1243. doi:10.1039/D1LC00999K
Yi, H.-G., Jeong, Y. H., Kim, Y., Choi, Y. J., Moon, H. E., Park, S. H., et al. (2019). A bioprinted human-glioblastoma-on-a-chip for the identification of patient-specific responses to chemoradiotherapy. Nat. Biomed. Eng. 3, 509–519. doi:10.1038/s41551-019-0363-x
Zhang, Y. S., Arneri, A., Bersini, S., Shin, S. R., Zhu, K., Goli-Malekabadi, Z., et al. (2016b). Bioprinting 3D microfibrous scaffolds for engineering endothelialized myocardium and heart-on-a-chip. Biomaterials 110, 45–59. doi:10.1016/j.biomaterials.2016.09.003
Zhang, Y. S., Davoudi, F., Walch, P., Manbachi, A., Luo, X., Dell'Erba, V., et al. (2016a). Bioprinted thrombosis-on-a-chip. Lab a Chip 16, 4097–4105. doi:10.1039/C6LC00380J
Keywords: Organs-on-chips, advanced technologies, standardization, biofabrication, artificial intelligence, multidimensional data, pharmaceutical applications
Citation: Meneses J, Conceição F, van der Meer AD, de Wit S and Moreira Teixeira L (2024) Guiding organs-on-chips towards applications: a balancing act between integration of advanced technologies and standardization. Front. Lab. Chip. Technol. 3:1376964. doi: 10.3389/frlct.2024.1376964
Received: 26 January 2024; Accepted: 15 April 2024;
Published: 15 May 2024.
Edited by:
Josep Samitier Martí, Institute for Bioengineering of Catalonia (IBEC), SpainReviewed by:
Sarah Spitz, Massachusetts Institute of Technology, United StatesEugenio Martinelli, University of Rome Tor Vergata, Italy
Copyright © 2024 Meneses, Conceição, van der Meer, de Wit and Moreira Teixeira. This is an open-access article distributed under the terms of the Creative Commons Attribution License (CC BY). The use, distribution or reproduction in other forums is permitted, provided the original author(s) and the copyright owner(s) are credited and that the original publication in this journal is cited, in accordance with accepted academic practice. No use, distribution or reproduction is permitted which does not comply with these terms.
*Correspondence: L. Moreira Teixeira, l.s.moreirateixeira@utwente.nl
†These authors have contributed equally to this work