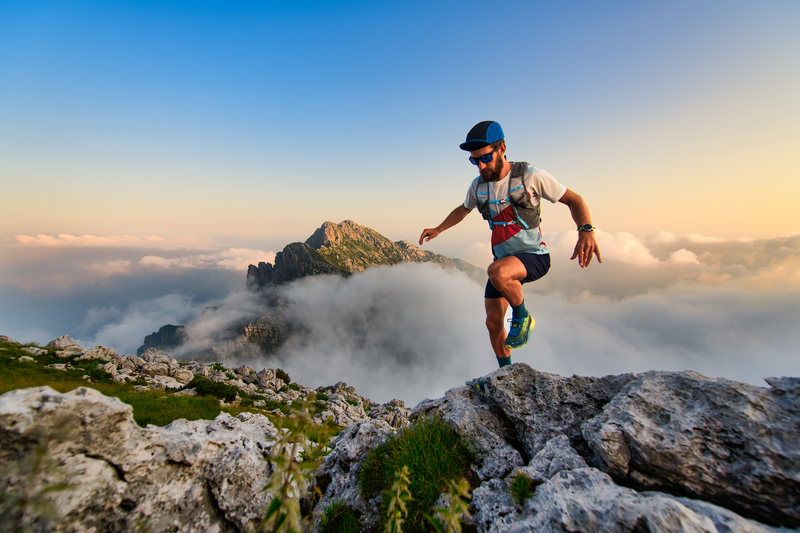
95% of researchers rate our articles as excellent or good
Learn more about the work of our research integrity team to safeguard the quality of each article we publish.
Find out more
PERSPECTIVE article
Front. Lab Chip Technol. , 18 July 2023
Sec. Imaging and Detection
Volume 2 - 2023 | https://doi.org/10.3389/frlct.2023.1230791
This article is part of the Research Topic The Future of Lab on a Chip Technologies: An Early Career Scientists’ Perspective View all 5 articles
Droplet microfluidics has evolved into a promising platform for high-throughput screening (HTS), allowing for rapid and precise analysis of thousands of samples encapsulated within droplets. Droplet microfluidic platform offers versatility, high-throughput, and the ability to compartmentalize reactions for a wide range of applications including pharmaceutics, cell analysis, and combinatorial chemical analysis. While droplet-based microfluidics has made considerable advances in automating basic laboratory tasks, for instance, manipulation, storage, and analysis, there has been comparatively little advancement toward HTS applications. The complexity of the technology, the lack of standardization, and the challenges associated with screening large numbers of samples are all factors that have contributed to the limited adoption of droplet-based microfluidics in HTS. In this perspective, we provide a comprehensive overview of the progress of droplet microfluidics as a potential platform for next-generation HTS, specifically in the domain of droplet separation and library generation. We hope that this perspective will inspire further research in relevant academic fields and contribute to the development of innovative HTS strategies based on droplet microfluidic technologies.
In the past, researchers have proposed that two-phase lab-on-a-chip devices, particularly digital microfluidic devices, have the potential to revolutionize high-throughput screening (HTS) technology. HTS platform refers to a system or technology that enables the rapid analysis and screening of a large number of individual samples. HTS is widely employed in early drug screening studies conducted by pharmaceutical companies, involving empirical testing of disease-associated targets (such as cells and proteins). In general, the majority of the HTS methods incorporated robotics to handle droplets, sensitive detectors to capture data, and software for data processing and control (Du et al., 2016). These days, there has been observed a paradigm shift in the adoption of microfluidic techniques within the field of HTS platforms (Sesen et al., 2017). Microfluidics platform enables easy generation of droplets with a wide range of volume, composition, and throughputs, often reaching speeds in the range of thousands or millions of droplets per second (Payne et al., 2020). The use of carrier fluid in the microfluidic platform grants a unique advantage by providing a protective shield for each droplet, addressing commonly faced issues such as absorption onto solid surfaces and contamination. Additional advantages of droplet microfluidic as a HTS platforms include the ability to perform large-scale screening experiments with minimal sample consumption and reduced experimental time.
Multiple functionalities are associated with droplet microfluidic platform, for instance, droplet separation, splitting, merging, incubation, composition control, etc. These features enhance the efficiency and versatility of droplet-based microfluidic platforms. This perspective provides a comprehensive overview of the advancement of droplet microfluidics as a promising platform for next-generation HTS in the domains of droplet separation for encapsulated sample analysis and droplet library generation with chemical concentration control as shown in Figure 1. Our aim is to inspire further research in relevant academic disciplines and contribute to the development of innovative HTS strategies based on droplet microfluidic technologies. This overview will serve as a reference for important considerations and potential applications of droplet microfluidics in HTS, while also highlighting the significant progress the field has achieved in the past decade.
FIGURE 1. Schematic representation of a high-throughput screening process for generating droplet libraries and separating the droplets containing the desired samples.
Microfluidic devices enable the generation of uniform droplets ranging from nanoliters to microliters in an immiscible carrier liquid, facilitating comprehensive and rapid studies to be conducted successively. In a droplet-based microfluidic device, the initial step is the precise and consistent production of a stream of uniformly sized droplets. Typically, droplet formation is accomplished using passive techniques that leverage the flow dynamics to deform the interface and facilitate the natural growth of interfacial instabilities. Widely used passive approaches for droplet production are T-junctions, flow focusing devices and co-flowing devices. Additionally, there are active techniques available for droplet formation, whereby the production of droplets can be initiated, and the volume of the produced droplets can be dynamically adjusted according to specific requirements (Zhu and Wang, 2017). Generally, the formation of droplets in microfluidic devices is primarily driven by fluid instabilities that arise when an immiscible dispersed fluid is introduced into a continuous fluid.
The strength of HTS platform lies in its capacity to simultaneously screen multiple targets employing a droplet library with different compositions. Henceforward, an accurate on-chip control of chemical concentration within droplets is crucial in various applications that rely on droplet-based systems, including combinatorial chemistry in drug discovery (Liu et al., 2017), high-throughput drug screening (Tu et al., 2021), and particle and hydrogel synthesis (Marre and Jensen, 2010). Researchers can manipulate the composition of droplets by incorporating different compounds, samples, or analytes of interest. The platform allows for the precise introduction of specific reagents, chemicals, or biomolecules into droplets, tailoring their composition to match the experimental requirements. Several droplet microfluidic techniques have been developed to modulate the chemical concentration of individual droplets. Initially, these techniques involve mixing desired chemical species in a solution prior to introduction in the dispersed phase of the microfluidic device. Few passive approaches are available which rely on controlling fluid flow rates and microchannel geometries. For instance, complex microchannels such as tree-shape (Dertinger et al., 2001), Y-shape (Holden et al., 2003), and counter-flow (Atencia et al., 2009), along with controlled flow rates, can be employed to mix the sample liquids before droplet production. But these methods have certain limitations such as long processing times up to several minutes and dynamic control over droplet concentration. Alternatively, active approaches, including the use of a peristaltic pump (Cai et al., 2012), a pneumatic valve (Zeng et al., 2009), or a compartment-on-demand platform (Gielen et al., 2013), can overcome the limitations of passive techniques. Nevertheless, these active methods necessitate large and intricate off-chip systems that are incompatible with other microfluidic platforms for integration and miniaturization. Most recently, few versatile approaches have been reported to achieve droplets with adjustable chemical concentration. One approach involves utilizing induced charge electroosmosis to regulate the chemical concentration within the droplets, resulting in the generation of droplet libraries with varying concentrations (Wu et al., 2022). Yet, this method has limitations in terms of throughput, range of chemical concentrations, and high amplitude. Another method utilizes surface acoustic waves (SAW) to induce acoustic streaming flow, allowing precise control of the chemical concentration in the dispersed phase liquid (Park et al., 2020). However, this method is highly limited by low throughput and irreversible bonding between the substrate and the microchannel. Most recently, a parallel-type acoustofluidic device was introduced to address the limitations of aforementioned technique offering high throughput and reusable substrate/microchannel (Kim et al., 2023). This SAW-based mixing enables the creation of droplet libraries with diverse chemical concentrations with high throughput. Chemical composition control opens up a wide range of possibilities for studying various biological, chemical, or biochemical processes in a droplet-based format.
The capability to selectively separate droplets plays a vital role in the development of lab-on-a-chip devices used in screening studies such as single cell analysis (Li et al., 2023), cell sequencing (Kang et al., 2018), disease diagnosis (Sun et al., 2022) and cell biology (Fan et al., 2019), enabling the removal of undesired droplets or the collection of desired ones into a specific outlet. Manipulating droplets within microfluidic systems can be accomplished through various microchannel designs that utilize hydrodynamic forces. Some widely used passive techniques include deterministic lateral displacement (Hochstetter et al., 2020) and pinched flow fractionation (Maenaka et al., 2008) which rely on microchannel geometry and droplet size. But, in addition to the formation of unwanted by-products, few limitations are obvious in these approaches, such as the dependance on fluid properties and flow conditions. Alternatively, external forces such as acoustic, electric, or magnetic fields can be applied to precisely control droplet locations in microfluidic systems. Table 1 provides a comprehensive comparison of essential characteristics, such as throughput, implementation simplicity, and required input power, while also highlighting the limitations associated with these techniques.
TABLE 1. Comparison of key features and limitations of various techniques for droplet library generation and droplet separation.
Typically, the differentiation between desired and undesired droplets is accomplished through droplet sensing and/or labeling step. Droplet microfluidic platforms widely incorporate optical detection methods, such as fluorescence or absorbance measurements, to analyze the droplets and extract relevant information. Fluorescence Activated Droplet Sorting (FADS) (Baret et al., 2009) is a typical example of this category and has significantly advanced the field due to its ability to manipulate samples at the droplet level rather than sorting individual cells. The technique depends on the light scattering or fluorescent properties, offering a high throughput. Despite high sensitivity and impressive readouts, the significant dependence on fluorescence tagging on a target droplet restricts the applicability of FADS in ranges of applications, particularly in cases where labeling with fluorescence is challenging (Toseland, 2013). Furthermore, droplets have the capability to be labeled with magnetic beads or ferrofluid, causing them to exhibit a response to magnetic fields generated within microfluidic devices due to their magnetic properties (Buryk-Iggers et al., 2019). Nevertheless, the process of labeling can alter cell behavior or even be toxic thereby undermining their potential clinical utility in biomedical applications (Frey et al., 2020). While there have been notable advancements in HTS platform using aforementioned approaches ensuring high throughput, ongoing research is currently dedicated to addressing a common limitation associated with these approaches by introducing label-free techniques for droplet separation. Several label-free methods includes, image-activated droplet sorting (Sesen and Whyte, 2020), electrical-impedance based separation (Zhong et al., 2023), mass spectrometry (Holland-Moritz et al., 2020), and acoustic impedance-based technique for droplet separation (Ali and Park, 2023). By avoiding the use of labels, these techniques aid direct analysis of samples, preserving their inherent properties and reducing potential artifacts introduced by labeling. These label-free methods aim to expand the range of applications and provide more flexibility in droplet separation.
In recent years, there have been notable advancements in droplet microfluidic technology, which have proven highly effective addressing numerous challenges encountered in biological research. Areas where these advancements have been particularly impactful are single-cell analysis and combinatorial chemistry. This perspective presents a thorough overview of the progress of droplet microfluidics as a highly promising next-generation HTS platform in two key areas: droplet separation for encapsulated sample analysis and droplet library generation with precise control over chemical concentrations. Table 1 shows the summary of key features and limitations of various techniques for droplet library generation and droplet separation.
The formation of droplet libraries requires precise control over the chemical content and surface topography in specific spatial patterns. This challenge is effectively resolved by the droplet microfluidic platform, which enables the generation of droplets on a chip while controlling their chemical content. Another common issue when dealing with microscale liquid volume using droplet array is that as the droplet size decreases, they become more prone to evaporation (Berthier et al., 2008). For instance, a 10 nL droplet can evaporate within 10–30 s, and a picoliter droplet can evaporate in less than 1 s under ambient conditions (Jackman et al., 1998). To address this issue, sophisticated and miniaturized technology is required, potentially including a platform that safeguards droplets from local humidity and atmospheric conditions. One approach involves covering the droplets with oil, which helps reduce the rate of evaporation. Another alternative way involves placing the droplet array in a closed environment with controlled humidity and introducing a sacrificial liquid layer around the desired droplets.
Considering the design and fabrication of microfluidic chips, polydimethylsiloxane and soft lithography are commonly used for experimental findings, there is a need for more affordable and stable materials and simplified chip manufacturing processes for commercial and clinical applications. In addition, since droplet microfluidics generates a microscale droplet with high speed and high throughput, it is also challenging to achieve rapid sensing and analysis of numerous microdroplets. While fluorescence currently serves as the predominant screening signal, there are still numerous compounds that cannot be detected using fluorescence-based methods. Therefore, there is a need to explore and develop alternative high-efficiency screening signals. Moreover, the potential of droplet analysis using Raman and Nuclear magnetic resonance spectroscopy as a versatile label-free detection technique exists. However, there is a need to overcome challenges related to detecting droplets in complex samples and addressing issues related to optical and magnetic interrogation. Techniques offered in the field of acoustophoresis have a great potential to manipulate droplets without the need of labeling and detection. However, these techniques are still in the early stages of development and have yet to achieve widespread commercial availability.
Droplet-based microfluidic systems have proven to be highly successful in diverse fields, finding widespread practical applications. Nevertheless, in order to obtain consistent and reproducible data, researchers face the task of continuously monitoring and adjusting parameters throughout their experiments to maintain stable conditions. Furthermore, conducting parametric analysis can be cumbersome since droplets are sensitive to environmental conditions, which is a key hindrance in becoming a droplet microfluidic a robust platform. Another issue while using droplet microfluidic is related to handling large amounts of multiplex data. To address these issues, artificial intelligence (AI) offers a broad range of capabilities, with regression analysis, image recognition, and classification being the most commonly applied functions in the context of droplet microfluidics. The comprehensive overview of the adaptation of AI in the fiend of droplet microfluidics can be found elsewhere (Liu et al., 2023).
Droplet-based microfluidic systems have proven to be highly successful in diverse fields, finding widespread practical applications. As a potential future application, droplet microfluidics can also be utilized for single cell immunology (Chattopadhyay et al., 2014), single cell profiles of transcriptome (Klein et al., 2015), and probably also for proteome (Albayrak et al., 2016). At last, it is important to highlight that the key to effectively utilizing microfluidics to address real-world biological and medical challenges lies in fostering strong collaboration among biologists, chemists, engineers, and clinicians. In addition to fluorescence-based droplet and cell sorting techniques, the collaboration between researchers in the fields of microfluidics, cell biology, clinical diagnostics, and microfabrication has led to the development of droplet microfluidic platforms for single-cell RNA sequencing and digital PCR. Furthermore, the collaboration of microfluidics engineers and synthetic biologists have also successfully created droplet-based platforms for synthetic biology applications. These platforms allow for the compartmentalization of biological reactions within discrete droplets, enabling the investigation of genetic circuits, protein engineering, and the construction of artificial cells. Moreover, droplet microfluidic devices have found utility in producing micro- and nanoscale particles, which are extensively used in various industries such as bio-pharmaceuticals, drug delivery, cosmetics, and the food industry.
The original contributions presented in the study are included in the article/Supplementary Material, further inquiries can be directed to the corresponding author.
MA and WK wrote the manuscript. JP provided the idea, supervision, funding acquisition and approved the final version of the manuscript. All authors contributed to the article and approved the submitted version.
This work was supported by the National Research Foundation of Korea (NRF) grants funded by the Korea government (MSIT) (Nos. RS-2023-00210891 and 2020R1A5A8018367).
The authors declare that the research was conducted in the absence of any commercial or financial relationships that could be construed as a potential conflict of interest.
All claims expressed in this article are solely those of the authors and do not necessarily represent those of their affiliated organizations, or those of the publisher, the editors and the reviewers. Any product that may be evaluated in this article, or claim that may be made by its manufacturer, is not guaranteed or endorsed by the publisher.
Albayrak, C., Jordi, C. A., Zechner, C., Lin, J., Bichsel, C. A., Khammash, M., et al. (2016). Digital quantification of proteins and mRNA in single mammalian cells. Mol. Cell. 61 (6), 914–924. doi:10.1016/j.molcel.2016.02.030
Ali, M., and Park, J. (2023). Ultrasonic surface acoustic wave-assisted separation of microscale droplets with varying acoustic impedance. Ultrason. Sonochemistry 93, 106305. doi:10.1016/j.ultsonch.2023.106305
Atencia, J., Morrow, J., and Locascio, L. E. (2009). The microfluidic palette: A diffusive gradient generator with spatio-temporal control. Lab a Chip 9 (18), 2707–2714. doi:10.1039/B902113B
Baret, J.-C., Miller, O. J., Taly, V., Ryckelynck, M., El-Harrak, A., Frenz, L., et al. (2009). Fluorescence-activated droplet sorting (FADS): Efficient microfluidic cell sorting based on enzymatic activity. Lab a Chip 9 (13), 1850–1858. doi:10.1039/B902504A
Berthier, E., Warrick, J., Yu, H., and Beebe, D. J. (2008). Managing evaporation for more robust microscale assays Part 1. Volume loss in high throughput assays. Lab a Chip 8 (6), 852–859. doi:10.1039/B717422E
Buryk-Iggers, S., Kieda, J., and Tsai, S. S. H. (2019). Diamagnetic droplet microfluidics applied to single-cell sorting. AIP Adv. 9 (7), 075106. doi:10.1063/1.5095884
Cai, L. F., Zhu, Y., Du, G. S., and Fang, Q. (2012). Droplet-based microfluidic flow injection system with large-scale concentration gradient by a single nanoliter-scale injection for enzyme inhibition assay. Anal. Chem. 84 (1), 446–452. doi:10.1021/ac2029198
Chattopadhyay, P. K., Gierahn, T. M., Roederer, M., and Love, J. C. (2014). Single-cell technologies for monitoring immune systems. Nat. Immunol. 15 (2), 128–135. doi:10.1038/ni.2796
Dertinger, S. K. W., Chiu, D. T., Jeon, N. L., and Whitesides, G. M. (2001). Generation of gradients having complex shapes using microfluidic networks. Anal. Chem. 73 (6), 1240–1246. doi:10.1021/ac001132d
Du, G., Fang, Q., and den Toonder, J. M. J. (2016). Microfluidics for cell-based high throughput screening platforms—a review. Anal. Chim. Acta 903, 36–50. doi:10.1016/j.aca.2015.11.023
Fan, W., Chen, X., Ge, Y., Jin, Y., Jin, Q., and Zhao, J. (2019). Single-cell impedance analysis of osteogenic differentiation by droplet-based microfluidics. Biosens. Bioelectron. 145, 111730. doi:10.1016/j.bios.2019.111730
Frey, C., Pfeil, J., Neckernuss, T., Geiger, D., Weishaupt, K., Platzman, I., et al. (2020). Label-free monitoring and manipulation of microfluidic water-in-oil droplets. VIEW 1 (4), 20200101. doi:10.1002/VIW.20200101
Gielen, F., van Vliet, L., Koprowski, B. T., Devenish, S. R. A., Fischlechner, M., Edel, J. B., et al. (2013). A fully unsupervised compartment-on-demand platform for precise nanoliter assays of time-dependent steady-state enzyme kinetics and inhibition. Anal. Chem. 85 (9), 4761–4769. doi:10.1021/ac400480z
Hochstetter, A., Vernekar, R., Austin, R. H., Becker, H., Beech, J. P., Fedosov, D. A., et al. (2020). Deterministic lateral displacement: Challenges and perspectives. ACS Nano 14 (9), 10784–10795. doi:10.1021/acsnano.0c05186
Holden, M. A., Kumar, S., Castellana, E. T., Beskok, A., and Cremer, P. S. (2003). Generating fixed concentration arrays in a microfluidic device. Sensors Actuators B Chem. 92 (1), 199–207. doi:10.1016/S0925-4005(03)00129-1
Holland-Moritz, D. A., Wismer, M. K., Mann, B. F., Farasat, I., Devine, P., Guetschow, E. D., et al. (2020). Mass activated droplet sorting (MADS) enables high-throughput screening of enzymatic reactions at nanoliter scale. Angew. Chem. Int. Ed. 59 (11), 4470–4477. doi:10.1002/anie.201913203
Jackman, R. J., Duffy, D. C., Ostuni, E., Willmore, N. D., and Whitesides, G. M. (1998). Fabricating large arrays of microwells with arbitrary dimensions and filling them using discontinuous dewetting. Anal. Chem. 70 (11), 2280–2287. doi:10.1021/ac971295a
Jung, J. H., Lee, K. H., Lee, K. S., Ha, B. H., Oh, Y. S., and Sung, H. J. (2014). Optical separation of droplets on a microfluidic platform. Microfluid. Nanofluidics 16 (4), 635–644. doi:10.1007/s10404-013-1263-0
Kang, H. M., Subramaniam, M., Targ, S., Nguyen, M., Maliskova, L., McCarthy, E., et al. (2018). Multiplexed droplet single-cell RNA-sequencing using natural genetic variation. Nat. Biotechnol. 36 (1), 89–94. doi:10.1038/nbt.4042
Kim, W., Cha, B., Jeon, J. S., and Park, J. (2023). Acoustofluidic control of chemical concentration within picoliter droplets in a disposable microfluidic chip. Sensors Actuators B Chem. 393, 134132. doi:10.1016/j.snb.2023.134132
Klein, A. M., Mazutis, L., Akartuna, I., Tallapragada, N., Veres, A., Li, V., et al. (2015). Droplet barcoding for single-cell transcriptomics applied to embryonic stem cells. Cell. 161 (5), 1187–1201. doi:10.1016/j.cell.2015.04.044
Li, B., Ma, X., Cheng, J., Tian, T., Guo, J., Wang, Y., et al. (2023). Droplets microfluidics platform—a tool for single cell research. Front. Bioeng. Biotechnol. 11, 1121870. doi:10.3389/fbioe.2023.1121870
Liu, H., Nan, L., Chen, F., Zhao, Y., and Zhao, Y. (2023). Functions and applications of artificial intelligence in droplet microfluidics. Lab a Chip 23, 2497–2513. doi:10.1039/D3LC00224A
Liu, R., Li, X., and Lam, K. S. (2017). Combinatorial chemistry in drug discovery. Curr. Opin. Chem. Biol. 38, 117–126. doi:10.1016/j.cbpa.2017.03.017
Maenaka, H., Yamada, M., Yasuda, M., and Seki, M. (2008). Continuous and size-dependent sorting of emulsion droplets using hydrodynamics in pinched microchannels. Langmuir 24 (8), 4405–4410. doi:10.1021/la703581j
Marre, S., and Jensen, K. F. (2010). Synthesis of micro and nanostructures in microfluidic systems. Chem. Soc. Rev. 39 (3), 1183–1202. doi:10.1039/B821324K
Park, J., Destgeer, G., Afzal, M., and Sung, H. J. (2020). Acoustofluidic generation of droplets with tunable chemical concentrations. Lab a Chip 20 (21), 3922–3929. doi:10.1039/D0LC00803F
Payne, E. M., Holland-Moritz, D. A., Sun, S., and Kennedy, R. T. (2020). High-throughput screening by droplet microfluidics: Perspective into key challenges and future prospects. Lab a Chip 20 (13), 2247–2262. doi:10.1039/D0LC00347F
Sesen, M., Alan, T., and Neild, A. (2017). Droplet control technologies for microfluidic high throughput screening (μHTS). Lab a Chip 17 (14), 2372–2394. doi:10.1039/C7LC00005G
Sesen, M., and Whyte, G. (2020). Image-based single cell sorting automation in droplet microfluidics. Sci. Rep. 10 (1), 8736. doi:10.1038/s41598-020-65483-2
Sun, C., Liu, L., Pérez, L., Li, X., Liu, Y., Xu, P., et al. (2022). Droplet-microfluidics-assisted sequencing of HIV proviruses and their integration sites in cells from people on antiretroviral therapy. Nat. Biomed. Eng. 6 (8), 1004–1012. doi:10.1038/s41551-022-00864-8
Toseland, C. P. (2013). Fluorescent labeling and modification of proteins. J. Chem. Biol. 6 (3), 85–95. doi:10.1007/s12154-013-0094-5
Tu, R., Zhang, Y., Hua, E., Bai, L., Huang, H., Yun, K., et al. (2021). Droplet-based microfluidic platform for high-throughput screening of Streptomyces. Commun. Biol. 4 (1), 647. doi:10.1038/s42003-021-02186-y
Wu, Y., Hu, B., Ma, X., Zhang, H., Li, W., Wang, Y., et al. (2022). Generation of droplets with adjustable chemical concentrations based on fixed potential induced-charge electro-osmosis. Lab a Chip 22 (2), 403–412. doi:10.1039/D1LC00983D
Zeng, S., Li, B., Su, X. o., Qin, J., and Lin, B. (2009). Microvalve-actuated precise control of individual droplets in microfluidic devices. Lab a Chip 9 (10), 1340–1343. doi:10.1039/B821803J
Zhong, J., Liang, M., Tang, Q., and Ai, Y. (2023). Selectable encapsulated cell quantity in droplets via label-free electrical screening and impedance-activated sorting. Mater. Today Bio 19, 100594. doi:10.1016/j.mtbio.2023.100594
Keywords: microfluidics, droplet microfluidics, high-throughput screening, drug screening, drug discovery, single-cell analysis
Citation: Ali M, Kim W and Park J (2023) Droplet microfluidic technologies for next-generation high-throughput screening. Front. Lab. Chip. Technol. 2:1230791. doi: 10.3389/frlct.2023.1230791
Received: 29 May 2023; Accepted: 10 July 2023;
Published: 18 July 2023.
Edited by:
Samar Damiati, University of Sharjah, United Arab EmiratesReviewed by:
Ahmad Saleem Akhtar, Royal Institute of Technology, SwedenCopyright © 2023 Ali, Kim and Park. This is an open-access article distributed under the terms of the Creative Commons Attribution License (CC BY). The use, distribution or reproduction in other forums is permitted, provided the original author(s) and the copyright owner(s) are credited and that the original publication in this journal is cited, in accordance with accepted academic practice. No use, distribution or reproduction is permitted which does not comply with these terms.
*Correspondence: Jinsoo Park, amluc29vcGFya0BqbnUuYWMua3I=
Disclaimer: All claims expressed in this article are solely those of the authors and do not necessarily represent those of their affiliated organizations, or those of the publisher, the editors and the reviewers. Any product that may be evaluated in this article or claim that may be made by its manufacturer is not guaranteed or endorsed by the publisher.
Research integrity at Frontiers
Learn more about the work of our research integrity team to safeguard the quality of each article we publish.