- 1Paris-1-Panthéon-Sorbonne University CESSP-UMR 8209, Paris, France
- 2French National Centre for Scientific Research (CNRS), Paris, France
- 3MATRICE Equipex, Seine-Saint-Denis, France
- 4Complex Systems Institute Paris Île-de-France, Paris, France
- 5Graduate School of Cognitive Engineering (ENSC), Talence, France
As the COVID-19 pandemic continues to unfold, numerous neurological symptoms emerge. The literature reports more and more manifestations of severe acute respiratory syndrome coronavirus 2 (SARS-CoV-2) related to headache, dizziness, impaired consciousness, cognitive impairment, and motor disorders. Moreover, the infection of SARS-CoV-2 may have a durable neurological impact. ACE2/TMPRSS2 is the main entry point into cells for some strains of coronaviruses (CoVs), including SARS-CoV-2, which uses it to target the central nervous system (CNS). The aim of this study was to characterize the scope of the potential complex impact of a SARS-CoV-2 infection in the brain. It concerns different scales: the topographic, cognitive, sensorimotor, and genetic one. We investigated which cognitive and sensorimotor functions are associated with the brain regions where ACE2/TMPRSS2 is overexpressed, hypothesising that they might be particularly affected by the infection. Furthermore, overexpressed genes in these regions are likely to be impacted by COVID-19. This general understanding is crucial to establish the potential neurological manifestations of the infection. Data on mRNA expression levels of genes were provided by the Allen Institute for Brain Science (AIBS), and the localisation of brain functions by the LinkRbrain platform. The latter was also used to analyze the spatial overlap between ACE2/TMPRSS2 overexpression, and either function-specific brain activations or regional overexpression of other genes. The characterisation of these overexpressed genes was based on the GeneCards platform and the gene GSE164332 from the Gene Expression Omnibus database. We analysed the cognitive and sensorimotor functions whose role might be impaired, of which 88 have been categorised into seven groups: memory and recollection, motor function, pain, lucidity, emotion, sensory, and reward. Furthermore, we categorised the genes showing a significant increase in concentration of their mRNAs in the same regions where ACE2/TMPRSS2 mRNA levels are the highest. Eleven groups emerged from a bibliographical research: neurodegenerative disease, immunity, inflammation, olfactory receptor, cancer/apoptosis, executive function, senses, ischemia, motor function, myelination, and dependence. The results of this exploration could be in relation to the neurological symptoms of COVID-19. Furthermore, some genes from peripheral blood are already considered as biomarker of COVID-19. This method could generate new hypotheses to explore the neurological manifestations of COVID-19.
Introduction
Recently, an increasing number of neurological symptoms are reported in different COVID-19 studies (Özdağ Acarli et al., 2020; Taquet et al., 2021). Among them, the most widely reported in humans are headache, dizziness, impaired consciousness, cognitive impairment, as well as motor disorders and neuropathic pain. Some studies suggest that the severe acute respiratory syndrome coronavirus 2 (SARS-CoV-2) infects the brain of COVID-19 patients. Especially, autopsies revealed SARS-CoV-2 antigens in the brain parenchyma and cortical neurons of some patients (Meinhardt et al., 2021; Song et al., 2021). The SARS-CoV-2 viral RNA has been detected in the substantia nigra. In animal studies, it is suggested that dopaminergic neurons, cortical neurons, microglia, and astrocytes are susceptible to SARS-CoV-2 infection (Chen S. et al., 2021; Ferren et al., 2021; Song et al., 2021). This infection may have a durable neurological impact (Harmer et al., 2002). Other studies established the hypothesis of a relation between a SARS-CoV-2 infection and some neurological diseases like Alzheimer’s, Parkinson’s, and dementia (Lev et al., 2003; Obulesu and Lakshmi, 2014).
Angiotensin-converting enzyme 2 (ACE2), a novel homologous of ACE expressed in renal and cardiovascular tissues, as well as the gastrointestinal system, was reported to be the main receptor for SARS-CoV-2 entry into cells (Netland et al., 2008). In the brain, several studies indicated that ACE2 was expressed in excitatory and inhibitory neurons, astrocytes, and microglia in the human and mouse brain (Chen R. et al., 2021; Mahalingam et al., 2021; Li et al., 2022). Baig et al. (2020) demonstrated that SARS-CoV-2 also uses ACE2 to target the central nervous system (CNS). Lu et al. (2020) analysed diffusion tensor imaging and 3D high-resolution T1WI sequences of several COVID-19 patients and concluded that significant structural changes happen in different regions, such as olfactory cortices, hippocampi, insula, and cingulate gyrus. These structural changes correspond to brain regions where the gene coding for ACE2 is expressed the most. Therefore, biological, cognitive, and sensorimotor functions that characterize the regions where ACE2 is overexpressed are likely to be affected by the COVID-19.
In addition to ACE2, other works (Hoffmann et al., 2020; Ren et al., 2020) have reported the transmembrane serine protease 2 (TMPRSS2) gene as another potential entry of SARS-CoV-2 in several tissues, such as kidney and lung tissue. For the brain, the potential entry ACE2/TMPRSS2 of the virus has been studied in mice and humans and has been reported by Fodoulian et al. (2020), in GSE151973. Furthermore, in addition to the role of ACE2 and TMPRSS2 as an entry of the virus through the nasal tissue to the brain, several studies have linked their brain distribution to certain neurological effects of COVID-19 (Boldrini et al., 2021; Hernández et al., 2021; Kulkarni et al., 2022).
Although the presence of SARS-CoV-2 in the CNS has been demonstrated, its mode of replication remains unclear. However, the results from modelling SARS-CoV-2 neuroinvasion and cell death using human brain organoids suggested that SARS-CoV-2 is able to use the machinery of neuronal cells to replicate (Song et al., 2021). In addition, Olivarria et al. (2022) revealed that an average of 50% of infected mice exhibited CNS infection characterised by widespread viral replication in neurons.
The aim of this study was to characterize the scope of the potential complex impact of a SARS-CoV-2 infection in the brain. It concerns different scales: the topographic, cognitive, sensorimotor, and genetic one. This characterisation concerns the cognitive and sensorimotor functions, but also the local overexpressed genes. This general understanding is crucial to establish the potential neurological manifestations of the infection (McFarland et al., 2021). Hypotheses were drawn using the genetic base of Allen Institute for Brain Science (AIBS) (Hawrylycz et al., 2012), and the topographical distance was provided by the LinkRbrain platform (Mesmoudi et al., 2015). LinkRbrain is an open-access web tool for multi-scale integration of knowledge on the brain.1 It provides a visualisation of the literature about 460 cognitive and sensorimotor functions of each brain region and integrates this functional information with transcriptome data. The cognitive and sensorimotor database has been automatically extracted from the literature. AIBS atlas is used to cover 20,789 mRNA (messenger ribonucleic acid) expressions in the 947 human brain regions expressed by 947 Montreal Neurological Institute (MNI) coordinates. For each coordinate, the platform maps the cognitive and sensorimotor functions on a brain, as well as the expression scores of 20,789 mRNAs of genes. Based on its topographical calculation, LinkRbrain provides relational graphs of the functions and the genes. The final objective of this platform was to document the cognitive and sensorimotor functions and the genes of each brain coordinate thanks to the topographical similarities and co-expressions of mRNA.
In this work, we especially aimed to document ACE2/TMPRSS2 by identifying the cognitive and sensorimotor functions that activate, separately, the same regions where ACE2 and TMPRSS2 are overexpressed, as well as the other genes co-overexpressed with ACE2 and TMPRSS2. To do so, we first detected and mapped the regions where the mRNAs of ACE2 and TMPRSS2 are overexpressed. Then, based on the topographical distance calculation, we found the cognitive and sensorimotor functions that activate these regions. Finally, we calculate the topographical distance between a specific mRNA (of ACE2 and TMPRSS2) and the localised overexpression of the 20,789 identified mRNAs. This allowed us to identify the most expressed genes in the regions where ACE2 (or TMPRSS2) is overexpressed. The results of this topographical exploration are coherent with observed COVID-19 neurological symptoms. This method could generate new hypotheses to explore the neurological manifestations of COVID-19.
Materials and Methods
Genomic Data Sources
The Allen Brain Atlas (ABA), developed by the AIBS (Hawrylycz et al., 2012), provides a transcriptomic map of the whole human brain. RNAs were extracted from each brain to implement 58,693 complementary RNA hybridisation probes. The ABA dataset consists of two full post-mortem brains and four half brains. In this study, we are interested in the complete brain data H0351.2001 and H0351.2002. The genetic profiles of these two brains are highly similar (Jones et al., 2009; Cioli et al., 2014). In addition, LinkRbrain used ABA H0351.2001, which presents the genetic profiles of a set of 947 samples (Mesmoudi et al., 2015). It represents the structures of the human brain in approximate proportion to the volumetric representation of each cortical, subcortical, cerebellar, and brainstem structure. In this study, all the genetic results are related to ABA H0351.2001.
DNA Chip and Transcriptomic Map
To obtain the genetic and topographical data of the brains studied by the AIBS (Hawrylycz et al., 2012), several steps of a process have been accomplished and they will be summarised in this section. Each brain studied was cooled and subjected to magnetic resonance imaging (MRI) of the skull, followed by embedding, smashing, and freezing. Cry sections of the entire brain were performed from each plate, after which the plates were subdivided and sectioned on 2 × 3 inch slides for histological analysis to identify the structure. Defined areas of the brain were isolated either by macrodissection (cortical gyri, other large structures) or laser microdissection (Leica LMD6000, Leica Microsystems) from tissue sections on polyethylene naphthalate membrane slides. Any given anatomical structure was first identified on the basis of histological data and then sampled from a series of contiguous coronal plates in both hemispheres. RNA was isolated from each sample and used to generate complementary RNA or complementary RNA (cRNA) probes (approximately 50,000 probes per brain) labelled for hybridisation with customised Agilent 64K microarrays.
Each sample was then placed back into the cortical space using functional MRI data and assigned MNI coordinates. A total of 1,000 MNI coordinates were characterised using concentration rates of approximately 20,789 RNAs.
Cognitive and Sensorimotor Data Sources
LinkRbrain relies on the database of activation peaks generated by the Neurosynth framework2 (Yarkoni et al., 2010). It contains 194,387 activation peaks automatically extracted with text mining from over 14,000 published neuroimaging papers, with 140,974 coordinates correctly labelled in Talairach or MNI (Evan et al., 1993; Talairach and Tournoux, 1993). To extract the terms used by authors to describe the cognitive and sensorimotor functions, we used the abstracts and titles of the neuroimaging articles contained in the Neurosynth database.
The unique relation linking the cognitive and sensorimotor functions is the topographical relations that can be estimated by quantifying the spatial overlap between task-related activations for different functions. In this work, we focus on topographical relations between the functions, the genetic expression profiles, and the neuroanatomical structures. We used a lexical extraction to identify a set of pertinent terms. Text processing involves both grammatical and statistical phases to automatically extract candidate n-grams (also called multi-terms) (Kageura, 1996; Frantzi and Ananiadou, 2000). We analysed the textual content found in titles and abstracts of the 14,000 papers within Neurosynth. The most pertinent noun phrases in our corpus were then curated by a neuroscientist who manually selected the 460 most frequent cognitive and sensorimotor functions. This curation operation simply consists in eliminating n-grams without any relation to cognitive and sensorimotor tasks. In order to link the activation peaks and the function labels, Neurosynth data and software were used to generate a meta-analytic reverse inference map for each of the previously extracted n-grams (Poldrack, 2006; Yarkoni et al., 2011). This map quantifies the degree to which each brain region is preferentially activated in studies tagged with a particular cognitive and sensorimotor functions label. We use the map inference procedure to generate cognitive and sensorimotor networks from the activation peaks (Yarkoni et al., 2010).
LinkRbrain Topographical Distance
The topographical overlap (i.e., correlation) between gene expression regions and cognitive and sensorimotor activations is expressed as a distance based on a correlation metric (Mesmoudi et al., 2015).
All calculations are based on the differential expression rate of genes in different brain regions. Gene expression region is a set of points or nodes. Given two nodes A and B (sets of weighted points), we can represent them as such:
where μi and νj are the weights of the two points Mi and Nj, respectively. The weights express genetic expression values related to the regions. “m” and “n” are the number of points in the sets A and B. The minimum number of points is 12 (coordination task set), and 23079 is the maximum number of points (memory task set).
The correlation score (σ) between the nodes A and B is defined by the formula:
where r is the reference radius (10 mm in this case, consistent with the meta-analysis), and d(Mi,Nj) is the Euclidian distance between Mi and Nj.
The Euclidian distance is calculated by the formula:
The final score between A and B is obtained after normalising the correlation scores.
For readability purposes, this result is displayed as multiplied by 100, which is then rounded to the nearest integer.
The correlation between the selected function(s), gene(s), or neuroanatomical regions and the other functions, genes, or neuroanatomical regions can be represented as a graph. The graph is computed using the force-directed layout algorithm (Fruchterman and Reingold, 1991).
Analysis From the LinkRbrain Platform
LinkRbrain is an open-access web platform integrating anatomical, functional, and genetic knowledge provided by the scientific community (see text footnote 1) (Mesmoudi et al., 2015). As explained above, the genetic data were provided by the AIBS (Hawrylycz et al., 2012), and the cognitive and sensorimotor functions have been automatically extracted from the literature.
The topographical distance described in the previous paragraph has been used to evaluate spatial correlations between the brain areas where mRNAs of ACE2 or TMPRSS2 (separately) are overexpressed, and the localised overexpression of 20,789 identified mRNAs.
The platform allows the comparison of new sets of coordinates with previously published articles. Thus, the insertion of gene names in the platform allows their visualisation as a new set of coordinates. In addition, it establishes correlations with other genes whose level of expression is already identified at the same location.
Furthermore, the platform incorporates a database of cognitive and sensorimotor functions with their coordinates, corresponding to the peaks of brain activation. This allows topographical interactions to be made by calculating the correlations on the overlap between the different activated zones and the localisations of the functions.
Complementary Databases
In order to identify biological functions and pathways, and to compare our results with the immune signature enrichment levels in peripheral blood and brain, we used the following:
• The human gene database GeneCards available at https://www.genecards.org/.
• The Gene Expression Omnibus (GEO) data using SARS-CoV-2 as the keyword.3 Especially the section SARS-CoV-2 and COVID-19 pathway (WP4846).
• The exploration results of expression profiling by high-throughput sequencing of GSE164485 (Fullard et al., 2021) and GSE164332 (Gagliardi et al., 2021) from the Gene Expression Omnibus database.
Visualisations
Thanks to the tools available on LinkRbrain we obtained:
• 3D and 2D visualisations: allow to envision the coordinates of the different transcription levels of ACE2 and TMPRSS2 mRNAs. On each 3D representation, zero coordinates appear at the crossing of vertical and horizontal lines.
• Topographical correlation graphs: illustrate the 20 most closely correlated cognitive and sensorimotor functions with ACE2 or TMPRSS2.
• List of cognitive and sensorimotor functions: based on its topographical distance calculation, LinkRbrain provides among 460 cognitive and sensorimotor functions the ones that activate the same topographical localisations where the ACE2 and TMPRSS2 mRNAs are highly expressed.
• List of genes: based on its topographical distance calculation, LinkRbrain provides among 20,789 mRNA gene expressions a list of mRNAs which share the highest co-expression with the ACE2 and TMPRSS2 mRNAs.
Data Availability
The topographical data that support a part of the findings of this study are the regions of the Talairach atlas openly available at http://www.talairach.org/index.html.
LinkRbrain relies on the database of activation peaks generated by the Neurosynth framework: http://neurosynth.org. The localisation of the cognitive and sensorimotor functions available on LinkRbrain has been automatically extracted with text mining from over 14,000 published neuroimaging papers, with 140,974 coordinates correctly labelled in Talairach or MNI.
The data on mRNA expression levels of genes were provided by the AIBS, which shared their human brain genetic data available at https://human.brain-map.org/.
Thanks to LinkRbrain’s topographical distance calculation, we analysed the correlation between ACE2 overexpression, and either region-specific brain activation, function-specific brain activations, or regional overexpression of other genes. The authors confirm that the lists of regions, functions, and genes found with this correlation are available in Supplementary Tables 1–4.
Results
In this study, we executed several stages of the LinkRbrain process (Mesmoudi et al., 2015). First, we started by mapping the regions where the ACE2 mRNAs are overexpressed in the brain thanks to ABA H0351.2001. Then, we used the topographical distance calculated by LinkRbrain to identify the main brain functions activating the same regions where the ACE2 mRNAs are the most overexpressed. Finally, we identified the other mRNAs that could be co-overexpressed in the same regions of ACE2 mRNAs.
A correlation based on the topographical distance calculates the intersection between two groups of points. Each group represents brain functions or genes, and the points are the activations or AIBS coordinates. This intersection between the groups respects the normalisation of the group sizes; thus the higher correlation indicates a higher intersection between the groups.
Visualisation of Angiotensin-Converting Enzyme 2 Overexpression
Representative points of ACE2 and TMPRSS2 are mapped on LinkRbrain on a 3D and 2D representations of the human brain cortical surface (see Figure 1). The LinkRbrain platform listed the main regions where ACE2 and TMPRSS2 mRNAs are overexpressed (see the list in Supplementary Table 1). The first observation was that ACE2 and TMPRSS2 are predominantly overexpressed in the same regions. We can find the brain stem and some regions from the subcortical, such as putamen, caudate, amygdala, insula, hippocampus, and thalamus. Other cortical regions are also detected: the temporal gyrus, the orbitofrontal lobe (BA10 and BA11), and some occipital regions.
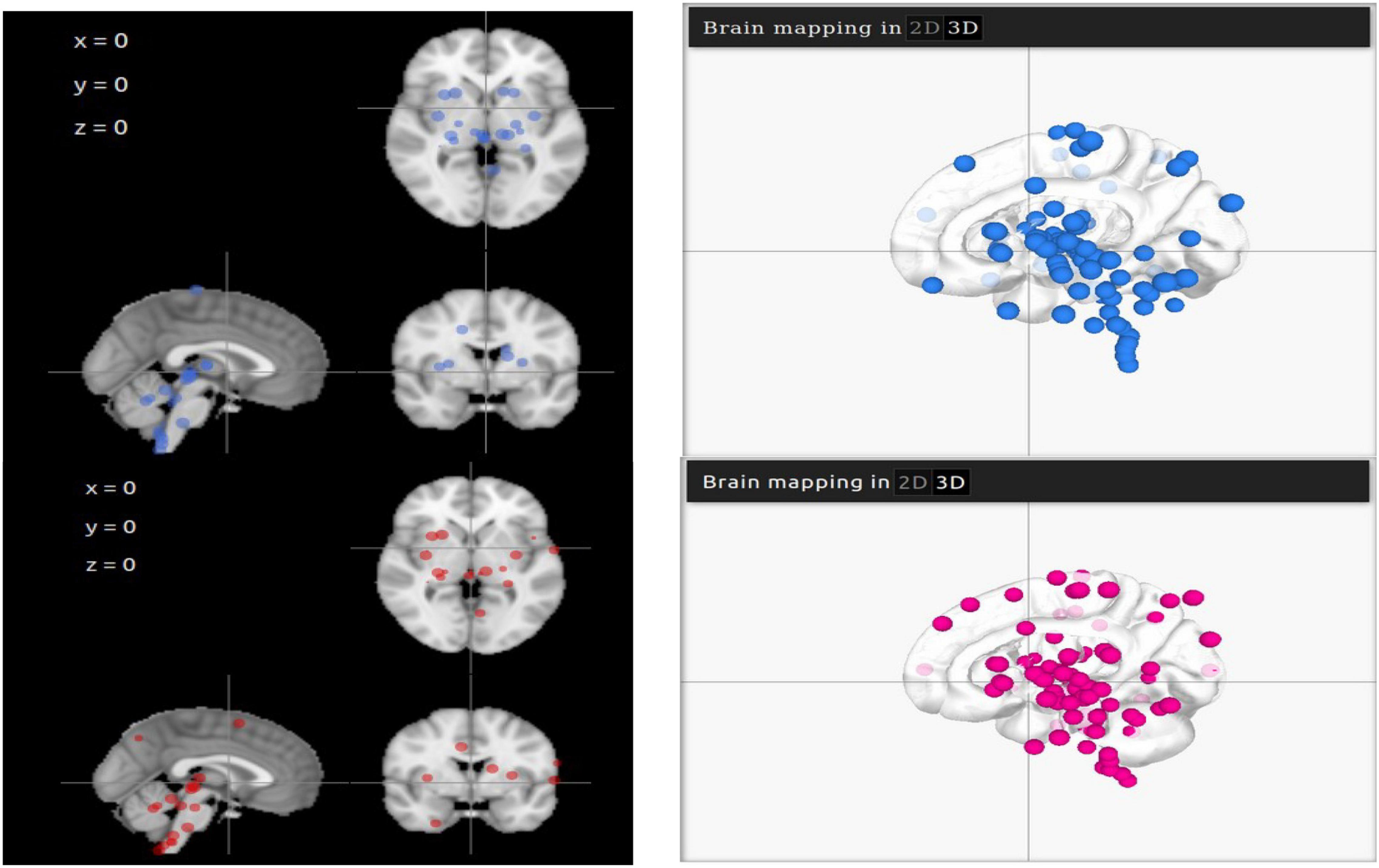
Figure 1. 2D and 3D visualisations of ACE2 (in blue) and TMPRSS2 (in magenta) mRNA overexpressions in the brain. The differences in 3D visualisation color intensity result from the transparency of the brain and the juxtaposition of several layers.
Potential Cognitive and Sensorimotor Functions Affected by Severe Acute Respiratory Syndrome Coronavirus 2
LinkRbrain incorporates a database of cognitive and sensorimotor functions with their coordinates corresponding to their brain activation peaks.
The results of this topographical exploration present some interesting outcomes. For each gene (ACE2/TMPRSS2), we analysed a group of the 100 most correlated functions. As expected, 93% of the functions are identical between the two groups. After merging the two functions groups, the resulting 107 functions whose role might be impaired, of which 104 are categorised into seven groups that could be in relation to the neurological symptoms of COVID-19 (see Table 1).
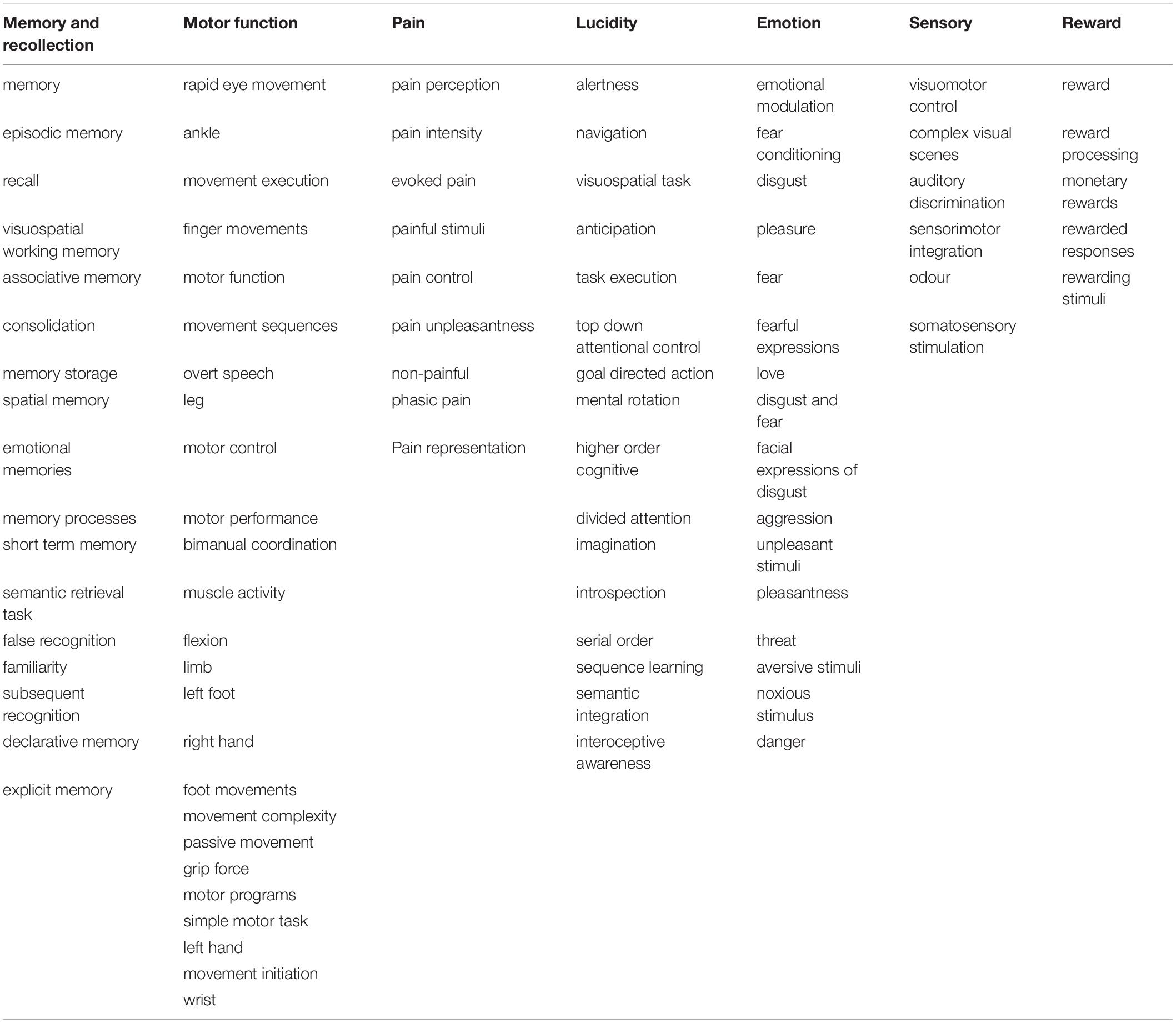
Table 1. Cognitive and sensorimotor functions activating the same regions where ACE2/TMPRSS2 mRNAs are overexpressed, clustered by groups.
The first group consists of 17 functions, all related to memory and recollection. Among them are “semantic retrieval task” and “false memory” with one of the highest correlations to ACE2/TMPRSS2. The second group consists of 25 functions connected with motor functions, such as “movement execution,” “movement sequences,” and “motor control,” which can be found in Figure 2. Another group is represented by eight functions and concerns physical and mental suffering, and pain is highly present in the results. The highest scores of correlations are found for “pain perception,” “pain intensity,” and “evoked pain.” For the next group, 16 functions related to the lucidity filling have been grouped. As the COVID-19 pandemic continues to unfold, numerous neurological symptoms are coming to light, among which is the feeling of confusion. The fifth group consists of 16 functions associated with emotional intensity and modulation. Finally, five functions concerning sensory inputs like visual, auditory, and odor tasks have been found in our results.
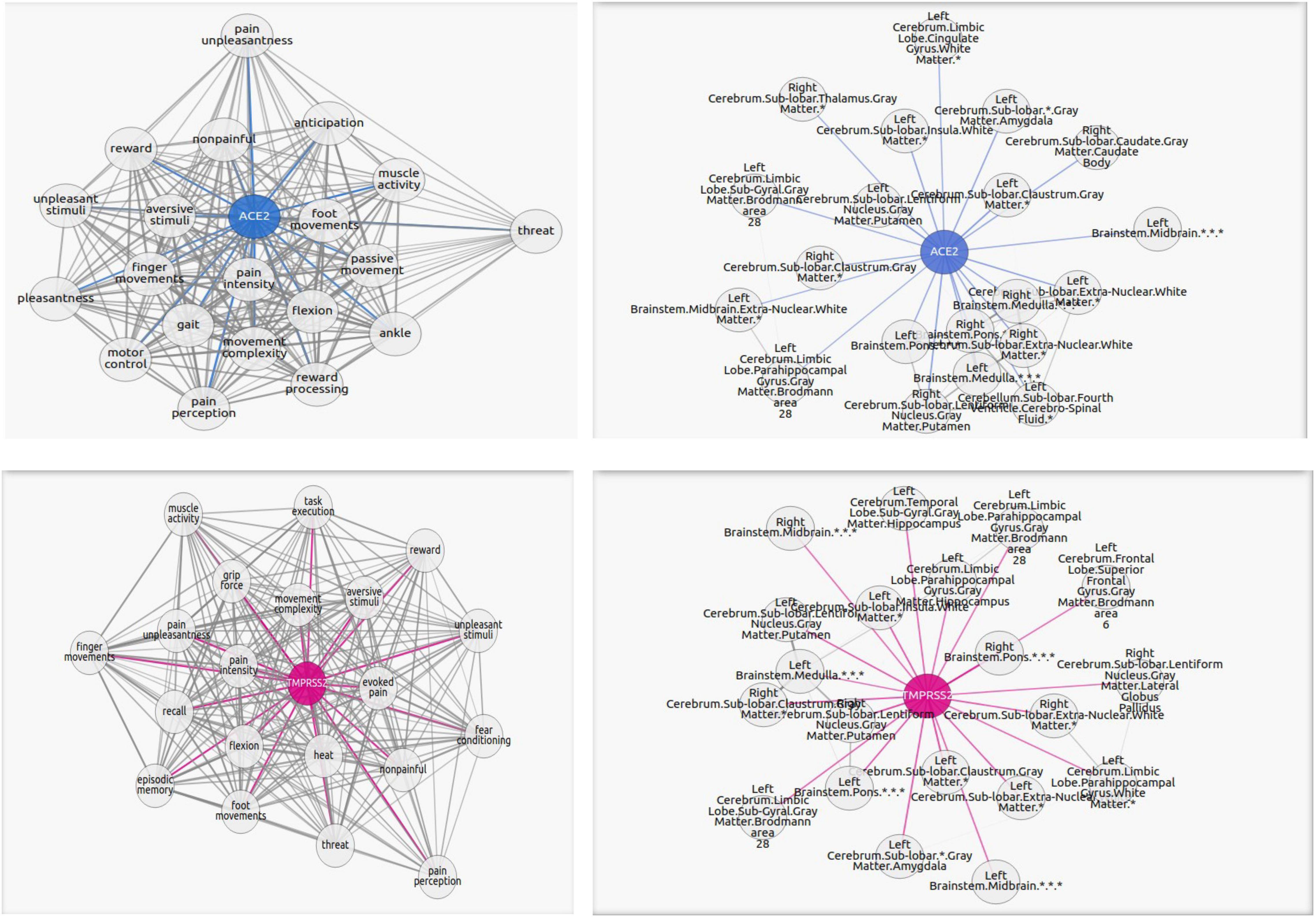
Figure 2. Graphs portraying the regions where the ACE2 (in blue) and TMPRSS2 (in magenta) are the overexpressed and their highest correlated functions. The blue or magenta links connect “ACE2” or “TMPRSS2” network with their correlated tasks. The gray links connect tasks with each other. Each graph shows the 20 most correlated functions/regions. The complete lists of functions and regions are in the Supplementary Materials.
Potential Genes Affected by Severe Acute Respiratory Syndrome Coronavirus 2
The other objective of this work was to explore the genes with mRNAs overexpressed in the same regions as ACE2 and TMPRSS2. LinkRbrain computed correlations between region coordinates where ACE2 mRNAs have the highest expression levels and the coordinates of the overexpression of each of the 20,788 genes on the platform. The same process was applied toTMPRSS2 mRNAs. From this calculation, and for each studied gene RANm (ACE2/TMPRSS2), 100 genes showed a significant increase in concentration of their mRNAs in the same regions where ACE2 or TMPRSS2 mRNA levels are the highest (see the list in Supplementary Table 3).
It has been established by Lu et al. (2020) that SARS-CoV-2 brain infection altered some brain regions. These topographical changes could cause gene transcription perturbations. The results related to the overexpression of mRNA genes, as well as the work presented in this study, are not definitive. All these results have to be used as a possible field to explore.
After merging the two ACE2/TMPRSS2 correlated mRNA groups, we obtained 179 unique genes. From these identified 179 genes, 98 were clustered into 11 groups (see Table 2). Considering their cellular and biological functions, this clusterisation was based on GeneCards platform and bibliographic research.
In Table 2, we identified eight genes coding for olfactory receptors (ORs), such as OR10H1, OR52B6, and OR8S1. Moreover, genes that could be related to inflammation, myelination, immunity, ischemia, and cancer genes have been found, such as CPA2, AADACL3, GRAP2, and REST. The characterisation of these groups naturally leads to the emergence of a cluster composed of genes related to neurodegenerative diseases, such as Alzheimer’s and Parkinson’s diseases. These two diseases do not appear to be the only ones worsening neuroinflammation and decreasing cognitive function as other diseases seem to be correlated with SARS-CoV-2 like dementia. Finally, we found that the AIPL1 and TMC2 genes are related to vision, hearing, and equilibrium perturbations.
In Table 3, we explored the pathways that involved some genes corresponding to the co-expressed mRNAs. We obtained results concerning pathways implicated in apoptosis process, immune system, and also in glycosylation.
Discussion
The aim of this study was to characterize the scope of the potential complex impact of a SARS-CoV-2 infection in the brain. LinkRbrain contains three databases: Talairach atlas of topographical regions, 460 cognitive and sensorimotor functions, and mRNAs gene expression. Each of these databases consists of MNI coordinates related to one or several labels. Depending on the scale, the label(s) will be topographical, cognitive and sensorimotor, or genetic. We started by mapping the regions where ACE2 and MPRSS2 mRNAs are overexpressed thanks to the mRNA expression levels of genes provided by the AIBS. Then, thanks to LinkRbrain’s topographical distance calculation, we analysed the correlation between ACE2 and MPRSS2 overexpression, and either region-specific brain activation, function-specific brain activations, or regional overexpression of other genes. The results of this integration brought to light seven cognitive and sensorimotor categories and 11 genetic groups. The main regions specific to ACE2 and MPRSS2 that we found are localised in the brain stem, the subcortical, the orbitofrontal, and some occipital regions. This is coherent with the literature study of Chen R. et al. (2021) where the spatial distribution analysis demonstrated that the expression of ACE2 and MPRSS2 is relatively high in specific brain locations, such as thalamus, human middle temporal gyrus, posterior cingulate cortex, and hippocampal. Thanks to the correlation calculated by LinkRbrain, it was established that 88 cognitive and sensorimotor functions activate these regions.
The first group consists of 15 functions, all related to memory and recollection. This suggests that COVID-19 could lead to memory complications. In fact, some studies (Ragab et al., 2020; Soy et al., 2020) show that SARS-CoV-2 infection entails a cytokine storm, which can later generate cognitive and psychiatric effects (Panoskaltsis et al., 2021). Lu et al. (2020) suggest that memory and concentration problems noticed after the infection are related to the significant structural changes occurred in some specific brain regions. Patients endured decreased concentration, reduced memory, and difficulty retaining information, persisting for over 2 months. Several studies described cognitive impairment in patients infected by SARS-CoV-2 (Almeria et al., 2020; Alemanno et al., 2021). Alemanno et al. (2021) demonstrated that 80% of patients presented cognitive deficits, when Almeria et al. (2020) mentioned deterioration in attention, memory, and executive functions. The second group consists of 25 functions connected with motor functions. Meppiel et al. (2021) reported that some patients showed symptoms like motor or sensitive deficit and/or movement disorder. In addition, aggravation of specific motor functions has been reported for Parkinsonian patients (Sulzer et al., 2020). The “reward” group from our results supports the relation between some Parkinsonian symptoms, such as impulse control disorder and reward, especially monetary reward (Drew et al., 2020). Another group is represented by eight functions and concerns physical and mental suffering. Pain is established as an important symptom of COVID-19, both in the acute phase of the disease and at later stages. Potential mechanisms change nociceptor excitability, which are likely to develop pain, induce neuropathies, and worsen existing pain states (McFarland et al., 2021). Moreover, painful symptoms, as well as muscular, joint, chronic, and neuropathic pain, are clear clinical manifestations of COVID-19 infection (Kemp et al., 2020; Widyadharma et al., 2020). For the next group, 14 functions related to the lucidity filling have been grouped. The dysexecutive syndrome, with attention and orientation disorders, has been documented as a consequence of COVID-19 (Helms et al., 2020; Rogers et al., 2020; Sahoo et al., 2020). This syndrome is directly related to the listed functions “proprioceptive information,” “visuospatial task,” “alertness,” and “executive control.” Mcloughlin et al. (2020) reported an increased rate of delirium and neurological alteration in COVID-19 patients. Other studies described neurological manifestations, such as dizziness, altered consciousness, disorientation, and delirium (Rogers et al., 2020). The fifth group consists of 16 functions associated with emotional intensity and modulation. Literature reviews on coronaviruses (CoVs) reported that during acute illness, patients are suffering from depressed mood, anxiety disorders, psychosis, and irritability (Murray et al., 1992; Paniz-Mondolfi et al., 2020). Finally, cinq functions concerning sensory inputs like visual, auditory, and odor tasks have been found in our results, which are compatible with some reported SARS-CoV-2 symptoms (Helms et al., 2020; Rogers et al., 2020; Sahoo et al., 2020).
Thanks to its topographical distance correlation, the LinkRbrain platform listed the genes co-expressed with ACE2 that could be affected by SARS-CoV-2 infection. One cluster concerns olfactory receptors, but there are also genes in relation to cognitive functions like executive functions and neurodegenerative diseases like Alzheimer’s, Parkinson’s, and dementia. We identified eight genes coding for ORs, such as OR10H1, OR52B6, and OR8S1. Studies demonstrated that in the case of humans and mice (transgenic), the brain was a target organ for infection in SARS-CoV-2 (Netland et al., 2008; Meinhardt et al., 2021). Besides, these studies show that the virus could reach the brain by the olfactory bulb or by the olfactory tract of the CNS (Meinhardt et al., 2021). Furthermore, Kerslake et al. (2020) demonstrated that ORs facilitate the transport of the virus using the vagal nerve in the context of COVID-19. Butler et al. (2006) showed that in mice infected intranasally with neurovirulent strains of HCoV-OC43, virus enters the CNS via the olfactory nerves with subsequent transneuronal retrograde dissemination. Once the brain is infected by SARS-CoV-2, several neurological effects are reported (Özdağ Acarli et al., 2020; Taquet et al., 2021). It has been shown that other CoVs like human HCoV-OC43 or SARS-CoV were associated with neuronal complications, such as inflammation and encephalomyelitis (Paniz-Mondolfi et al., 2020). Groups that could be related to these neurological symptoms emerged in our results, such as inflammation, myelination, immunity, ischemia, and cancer genes. Nonetheless, Murray et al. (1992) demonstrated that murine coronavirus can cause direct lysis of oligodendrocytes and final demyelination in the human CNS. Poyiadji et al. (2020) computed tomography and MRI reports of hemorrhagic necrotising encephalopathy of a COVID-19 female. Other studies described several symptoms of inflammatory-induced nerve dysfunctions (Chen et al., 2020; Xydakis et al., 2020). Furthermore, Toscano et al. (2020) reported that several COVID-19 patients developed the autoimmune Guillain–Barré syndrome after a few days following the onset of SARS-CoV-2 infection. The other well-distinguished cluster is composed of genes related to neurodegenerative diseases, such as Alzheimer’s and Parkinson’s diseases. This group could be explained with the presence of genes related to demyelination and inflammation. In the literature, especially on transgenic mice (Sy et al., 2011), a direct causal relationship has been reported between HCoV-OC43, MHV, certain neuropathogenesis, and degeneration of specific cognitive functions. In addition, Arbour et al. (2000) reported that the genetic material of CoVs is co-localised with Aβ protein in the brain. It is likely that SARS-CoV-2 can induce the accumulation of Aβ protein and may lead to a close relationship between Alzheimer’s disease susceptibility and neuroinflammation due to SARS-CoV-2. Alzheimer’s disease does not appear to be the only one worsening neuroinflammation and decreasing cognitive function. According to our results and the literature, the hypothetical vulnerability of SARS-CoV-2 localised in basal ganglia and dopaminergic brain regions might increase Parkinsonian symptoms. This could be induced by a systemic failure of the dopamine synthesis pathway. It has been established that alterations in apoptosis-related signalling pathways are involved in Alzheimer’s and Parkinson’s diseases (Lev et al., 2003; Obulesu and Lakshmi, 2014). In our results, we found several genes related to cancer. In the literature, some of these genes, such as RBMY1A3P and TP53, are involved in apoptosis (Sutherland et al., 2005). Therefore, modulation of neuronal apoptosis by SARS-CoV-2 during latent infections could possibly be related to alterations in neuronal processes, leading to neuronal degeneration and brain damage (Patatian and Benech, 2020). Furthermore, other diseases seem to be correlated with SARS-CoV-2 like dementia. Taquet et al. (2021) released a meta-analysis on symptoms presented over 236,379 survivors of COVID-19 6 months after their contamination. It was noticed that dementia, anxiety disorder, and psychotic disorder are substantial psychiatric morbidity related to COVID-19 infection. Finally, studies found that other senses are perturbed: vision, hearing, and equilibrium (Sutherland et al., 2005; Helms et al., 2020). In our results, we found several genes related to these symptoms like AIPL1 and TMC2. Moreover, conjunctivitis has been a documented phenomenon in COVID-19, and reverse transcription polymerase chain reaction of conjunctiva and tears has tested positive for SARS-CoV-2 (Chen et al., 2020).
To compare our results with other brain data, we analysed the GSE164332 (Fullard et al., 2021) data that had focused on the frontal cortex. Intersection of a larger set of our results for ACE2/TMPRSS2 (Supplementary Table 4) with that of Gagliardi et al. (2021) (Fullard et al., 2021) provided us with five genes, namely, SLC14A1, HIF3A, RGS5, ZNF622 (instead of ZNF621), and HBA2. The HIF3A, RGS5, and HBA2 genes were validated by quantitative PCR (qPCR) method. In addition, Gagliardi et al. (2021) (Fullard et al., 2021) considered that these genes are the most deregulated (mRNA encoding the HBA hemoglobin subunits upregulated, and the SLC14A1, HIF3A, and RGS5 genes downregulated), and they are associated with hypoxia and inflammation.
Also, we found four common deregulated genes (INP5D, PRKCA, LRP1, and FCGR2A) in another study focused on the following brain regions: the dorsolateral prefrontal cortex, the medulla oblongata, and the choroid plexus GSE164485 (Gagliardi et al., 2021). We also found other representatives of the same family for others genes. For example, we obtained CD81 and CD82 instead of CD83. Similarly, we obtained DOCK1, an important paralog of DOCK2.
We were also interested in the pathways where our gene results are involved. Based on the exploration of GeneCard, the pathways that we found are implicated in the transport of glucose, glycosylation, innate immune system, GPCR, and also apoptosis, immune response IFN, interferon, and others. These results are confirmed with those obtained from the peripheral blood of convalescent people.
Furthermore, from our results, several genes appear as correlated to this infection as other works based on exploration of data from patient infected by SARS-CoV-2. From these genes, we note the following: CXCR6 (Kasela et al., 2021), CST9L4 (Yan et al., 2020), NOXO1 (Sindona et al., 2021), IL28B (Alothaid et al., 2020), FGF23 (George et al., 2021), and finally, the controverted vitamin D receptor VDR (Ceolin et al., 2021).
In this work, we attempted to relate the information processing performed with the cognitive and sensorimotor functions, and the genes revealed by their mRNA expression level. This type of methodology is used by Cioli et al. (2014) who concluded to a relation between the cognitive function and the genetic expression. Zhou et al. (2020) highlighted the executive functioning impairment, underlining the role of inflammatory processes. They reported a positive association between attentional deficits and inflammatory levels. In our results, both genetic and functional results are consistent. The altered functions on the movement and reward reflect the connection of certain genes with Parkinson’s disease. The functions related to memory and lucidity are directly correlated with inflammation, immunity, and neurodegenerative diseases, such as Alzheimer’s and dementia. Finally, it appears that sensory inputs are impacted, such as visual, auditory, and odor tasks. In our results, we found olfactory receptors and genes related to vision, hearing, and equilibrium disturbances.
Limitations
Even though our results are coherent with observed COVID-19 neurological manifestations, and that some results are validated with infected people, the limit of this method remains in the nature of the cognitive and sensorimotor database and the lack of similar genetic data that cover the whole brain, from infected participant. The cognitive and sensorimotor database is composed with coordinates extracted from the literature. In order to complete this work, it would be necessary to conduct real experiments based on these results as they are not definitive and can only be used as a substantial field of research. This method could generate new hypotheses to explore the neurological manifestations of COVID-19. It presents results in an objective and global manner, based on existing knowledge produced by the scientific community. The technical and methodological contributions of LinkRbrain enable a functional and transcriptome characterisation of certain pathologies, including COVID-19. The creation of large open-source databases is part of the current big data scientific revolution. These techniques produce an intelligible synthesis of results and provide access to cutting-edge knowledge from related fields, which are often fundamental to successful research.
Data Availability Statement
The original contributions presented in this study are included in the article/Supplementary Material, further inquiries can be directed to the corresponding author.
Author Contributions
SM conceived and designed the experiments. SM, MR, and CL performed the experiments and analysed the data. All authors wrote the manuscript, contributed to the article, and approved the submitted version.
Funding
This study was funded by the French General Secretariat for Investment via the National Research Agency (ANR) “Programme d’Investissement d’Avenir (PIA ANR-10-EQPX-0021-01)” and by the CNRS innovation (Prematuration Programme). This study was realised in the framework of the “équipement d’excellence MATRICE” (matricememory.fr). This program was administratively sponsored by the HESAM University.
Conflict of Interest
The authors declare that the research was conducted in the absence of any commercial or financial relationships that could be construed as a potential conflict of interest.
Publisher’s Note
All claims expressed in this article are solely those of the authors and do not necessarily represent those of their affiliated organizations, or those of the publisher, the editors and the reviewers. Any product that may be evaluated in this article, or claim that may be made by its manufacturer, is not guaranteed or endorsed by the publisher.
Acknowledgments
We would like to thank Allen Institute for Brain Science for sharing their human brain genetic expression data. Special thanks go to Clare Mary Puyfoulhoux and Betty Merhi who participated to the success of this study.
Supplementary Material
The Supplementary Material for this article can be found online at: https://www.frontiersin.org/articles/10.3389/fnint.2022.756604/full#supplementary-material
Abbreviations
ABA, Allen Brain Atlas; AIBS, Allen Institute for Brain Science; CNS, central nervous system; CoVs, coronaviruses; MNI, Montreal Neurological Institute; ORs, olfactory receptors.
Footnotes
- ^ https://www.linkrbrain.org
- ^ http://neurosynth.org
- ^ ncbi.nlm.nih.gov/geo/
- ^ https://pubchem.ncbi.nlm.nih.gov/pathway/WikiPathways:WP4846
References
Alemanno, F., Houdayer, E., Parma, A., Spina, A., Del Forno, A., Scatolini, A., et al. (2021). COVID-19 cognitive deficits after respiratory assistance in the subacute phase: a COVID-rehabilitation unit experience. PLoS One 16:e0246590. doi: 10.1371/journal.pone.0246590
Almeria, M., Cejudo, J. C., Sotoca, J., Deus, J., and Krupinski, J. (2020). Cognitive profile following COVID-19 infection: clinical predictors leading to neuropsychological impairment. Brain Behav. Immun. Health 9:100163. doi: 10.1016/j.bbih.2020.100163
Alothaid, H., Aldughaim, M. S. K., El Bakkouri, K., AlMashhadi, S., and Al-Qahtani, A. A. (2020). Similarities between the effect of SARS-CoV-2 and HCV on the cellular level, and the possible role of ion channels in COVID19 progression: a review of potential targets for diagnosis and treatment. Channels 14, 403–412. doi: 10.1080/19336950.2020.1837439
Arbour, N., Day, R., Newcombe, J., and Talbot, P. J. (2000). Neuroinvasion by human respiratory coronaviruses. J. Virol. 74, 8913–8921. doi: 10.1128/jvi.74.19.8913-8921.2000
Baig, A. M., Khaleeq, A., Ali, U., and Syeda, H. (2020). Evidence of the COVID-19 Virus Targeting the CNS: tissue distribution, host-virus interaction, and proposed neurotropic mechanisms. ACS Chem Neurosci. 11, 995–998. doi: 10.1021/acschemneuro.0c00122
Boldrini, M., Canoll, P. D., and Klein, R. S. (2021). How COVID-19 affects the brain. JAMA Psychiatry 78, 682–683. doi: 10.1001/jamapsychiatry.2021.0500
Butler, N., Pewe, L., Trandem, K., and Perlman, S. (2006). Murine encephalitis caused by HCoV-OC43, a human coronavirus with broad species specificity, is partly immune-mediated. Virology 347, 410–421. doi: 10.1016/j.virol.2005.11.044
Ceolin, G., Mano, G. P. R., Hames, N. S., Antunes, L. D. C., Brietzke, E., Rieger, D. K., et al. (2021). Vitamin D, depressive symptoms, and Covid-19 Pandemic. Front. Neurosci. 15:670879. doi: 10.3389/fnins.2021.670879
Chen, L., Liu, M., Zhang, Z., Qiao, K., Huang, T., Chen, M., et al. (2020). Ocular manifestations of a hospitalised patient with confirmed 2019 novel coronavirus disease. Br. J. Ophthalmol. 104, 748–751. doi: 10.1136/bjophthalmol-2020-316304
Chen, R., Wang, K., Yu, J., French, L., Chen, Z., Wen, C., et al. (2021). The Spatial and Cell-Type Distribution of SARS-CoV-2 Receptor ACE2 in the Human and Mouse Brains. Front. Neurol. 11:573095. doi: 10.3389/fneur.2020.573095
Chen, S., Han, Y., Yang, L., Kim, T., Nair, M., Harschnitz, O., et al. (2021). SARS-CoV-2 infection causes dopaminergic neuron senescence. Res. Sq. [Preprint]. doi: 10.21203/rs.3.rs-513461/v1
Cioli, C., Abdi, H., Beaton, D., Burnod, Y., and Mesmoudi, S. (2014). Differences in human cortical gene expression match the temporal properties of large-scale functional networks. PLoS One 9:e115913. doi: 10.1371/journal.pone.0115913
Drew, D. S., Muhammed, K., and Baig, F. (2020). Dopamine and reward hypersensitivity in Parkinson’s disease with impulse control disorder. Brain 143, 2502–2518. doi: 10.1093/brain/awaa198
Evan, A., Collins, D., Mills, S., Brown, D., Kelly, R., and Peters, T. (1993). “3D statistical neuroanatomical models from 305 MRI Volumes,” in Proceedings of the 1993 Nuclear Science Symposium and Medical Imaging Conference, San Francisco, CA, 1813–1817. doi: 10.1109/NSSMIC.1993.373602
Ferren, M., Favède, V., Decimo, D., Iampietro, M., Lieberman, N. A. P., Weickert, J. L., et al. (2021). Hamster organotypic modeling of SARS-CoV-2 lung and brainstem infection. Nat. Commun. 12:5809. doi: 10.1038/s41467-021-26096-z
Fodoulian, L., Tuberosa, J., Rossier, D., Boillat, M., Kan, C., Pauli, V., et al. (2020). SARS-CoV-2 receptors and entry genes are expressed in the human olfactory neuroepithelium and brain. iScience 23:101839. doi: 10.1016/j.isci.2020.101839
Frantzi, K., and Ananiadou, S. (2000). Automatic recognition of multi-word terms: the c-value/nc-value method. Int. J. Digit. Libr. 3, 115–130. doi: 10.1007/3-540-49653-X_35
Fruchterman, T. M. J., and Reingold, E. (1991). Graph drawing by force-directed placement. Softw. Pract. Exp. 21, 1129–1164. doi: 10.1002/spe.4380211102
Fullard, J. F., Lee, H. C., Voloudakis, G., Suo, S., Javidfar, B., Shao, Z., et al. (2021). Single-nucleus transcriptome analysis of human brain immune response in patients with severe COVID-19. Genome Med. 13:118. doi: 10.1186/s13073-021-00933-8
Gagliardi, S., Poloni, E. T., Pandini, C., Garofalo, M., Dragoni, F., Medici, V., et al. (2021). Detection of SARS-CoV-2 genome and whole transcriptome sequencing in frontal cortex of COVID-19 patients. Brain Behav. Immun. 97, 13–21. doi: 10.1016/j.bbi.2021.05.012
George, B., Amjesh, R., Paul, A. M., Santhoshkumar, T. R., Pillai, M. R., and Kumar, R. (2021). Evidence of a dysregulated vitamin D endocrine system in SARS-CoV-2 infected patient’s lung cells. Sci. Rep. 11:8570. doi: 10.1038/s41598-021-87703-z
Harmer, D., Gilbert, M., Borman, R., and Clark, K. L. (2002). Quantitative mRNA expression profiling of ACE 2, a novel homologue of angiotensin converting enzyme. FEBS Lett. 532, 107–110. doi: 10.1016/s0014-5793(02)03640-2
Hawrylycz, M. J., Lein, E. S., Guillozet-Bongaarts, A. L., Shen, E. H., Ng, L., Miller, J. A., et al. (2012). An anatomically comprehensive atlas of the adult human brain transcriptome. Nature 489, 391–399. doi: 10.1038/nature11405
Helms, J., Kremer, S., Merdji, H., Clere-Jehl, R., Schenck, M., Kummerlen, C., et al. (2020). Neurologic Features in Severe SARS-CoV-2 Infection. N. Engl. J. Med. 382, 2268–2270. doi: 10.1056/NEJMc2008597
Hernández, V. S., Zetter, M. A., Guerra, E. C., Hernández-Araiza, I., Karuzin, N., and Hernández-Pérez, O. R. (2021). ACE2 expression in rat brain: implications for COVID-19 associated neurological manifestations. Exp. Neurol. 345:113837. doi: 10.1016/j.expneurol.2021.113837
Hoffmann, M., Kleine-Weber, H., Schroeder, S., Krüger, N., Herrler, T., Erichsen, S., et al. (2020). SARS-CoV-2 Cell Entry Depends on ACE2 and TMPRSS2 and Is Blocked by a Clinically Proven Protease Inhibitor. Cell 181, 271–280.e8.
Jones, A. R., Overly, C. C., and Sunkin, S. M. (2009). The Allen Brain Atlas: 5 years and beyond. Nat. Rev. Neurosci. 10, 821–828. doi: 10.1038/nrn2722
Kageura, K. (1996). Methods of automatic term recognition: a review. Terminology 3, 259–289. doi: 10.1075/term.3.2.03kag
Kasela, S., Daniloski, Z., Bollepalli, S., Jordan, T. X., tenOever, B. R., Sanjana, N. E., et al. (2021). Integrative approach identifies SLC6A20 and CXCR6 as putative causal genes for the COVID-19 GWAS signal in the 3p21.31 locus. Genome Biol. 22:242. doi: 10.1186/s13059-021-02454-4
Kemp, H. I., Corner, E., and Colvin, L. A. (2020). Chronic pain after COVID-19: implications for rehabilitation. Br. J. Anaesth. 125, 436–440. doi: 10.1016/j.bja.2020.05.021
Kerslake, R., Hall, M., Randeva, H. S., Spandidos, D. A., Chatha, K., Kyrou, I., et al. (2020). Co-expression of peripheral olfactory receptors with SARS-CoV-2 infection mediators: potential implications beyond loss of smell as a COVID-19 symptom. Int. J. Mol. Med. 46, 949–956. doi: 10.3892/ijmm.2020.4646
Kulkarni, P. G., Sakharkar, A., and Banerjee, T. (2022). Understanding the role of nACE2 in neurogenic hypertension among COVID-19 patients. Hypertens. Res. 45, 254–269. doi: 10.1038/s41440-021-00800-4
Lev, N., Melamed, E., and Offen, D. (2003). Apoptosis and Parkinson’s disease. Prog. Neuropsychopharmacol. Biol. Psychiatry 27, 245–250. doi: 10.1016/S0278-5846(03)00019-8
Li, T., Huang, H. Y., Wang, H. D., Gao, C.-C., Liang, H., Deng, C. L., et al. (2022). Restoration of brain angiotensin-converting enzyme 2 alleviates neurological deficits after severe traumatic brain injury via mitigation of pyroptosis and apoptosis. J. Neurotrauma 39, 423–434. doi: 10.1089/neu.2021.0382
Lu, Y., Li, X., Geng, D., Mei, N., Wu, P. Y., Huang, C. C., et al. (2020). Cerebral Micro-Structural Changes in COVID-19 Patients - An MRI-based 3-month Follow-up Study. EClinicalMedicine 25:100484. doi: 10.1016/j.eclinm.2020.100484
Mahalingam, R., Dharmalingam, P., Santhanam, A., Kotla, S., Davuluri, G., Karmouty-Quintana, H., et al. (2021). Single-cell RNA sequencing analysis of SARS-CoV-2 entry receptors in human organoids. J. Cell. Physiol. 236, 2950–2958. doi: 10.1002/jcp.30054
McFarland, A. J., Yousuf, M. S., Shiers, S., and Price, T. J. (2021). Neurobiology of SARS-CoV-2 interactions with the peripheral nervous system: implications for COVID-19 and pain. Pain Rep. 6:e885. doi: 10.1097/PR9.0000000000000885
Mcloughlin, B. C., Miles, A., Webb, T. E., Knopp, P., Eyres, C., Fabbri, A., Humphries, F., et al. (2020). Functional and cognitive outcomes after COVID-19 delirium. Eur. Geriatr. Med. 11, 857–862. doi: 10.1007/s41999-020-00353-8
Meinhardt, J., Radke, J., Dittmayer, C., Franz, J., Thomas, C., Mothes, R., et al. (2021). Olfactory transmucosal SARS-CoV-2 invasion as a port of central nervous system entry in individuals with COVID-19. Nat. Neurosci. 24, 168–175. doi: 10.1038/s41593-020-00758-5
Meppiel, E., Peiffer-Smadja, N., Maury, A., Bekri, I., Delorme, C., Desestret, V., et al. (2021). Neurologic manifestations associated with COVID-19: a multicentre registry. Clin. Microbiol. Infect. 27, 458–466. doi: 10.1016/j.cmi.2020.11.005
Mesmoudi, S., Rodic, M., Cioli, C., Cointet, J. P., Yarkoni, T., and Burnod, Y. (2015). LinkRbrain: multi-scale data integrator of the brain. J. Neurosci. Methods 241, 44–52. doi: 10.1016/j.jneumeth.2014.12.008
Murray, R. S., Cai, G. Y., Hoel, K., Zhang, J. Y., Soike, K. F., and Cabirac, G. F. (1992). Coronavirus infects and causes demyelination in primate central nervous system. Virology 188, 274–284. doi: 10.1016/0042-6822(92)90757-g
Netland, J., Meyerholz, D. K., Moore, S., Cassell, M., and Perlman, S. (2008). Severe acute respiratory syndrome coronavirus infection causes neuronal death in the absence of encephalitis in mice transgenic for human ACE2. J. Virol. 82, 7264–7275. doi: 10.1128/JVI.00737-08
Obulesu, M., and Lakshmi, M. J. (2014). Apoptosis in Alzheimer’s disease: an understanding of the physiology, pathology and therapeutic avenues. Neurochem. Res. 39, 2301–2312. doi: 10.1007/s11064-014-1454-4
Olivarria, G. M., Cheng, Y., Furman, S., Pachow, C., Hohsfield, L. A., Smith-Geater, C., et al. (2022). Microglia do not restrict SARS-CoV-2 replication following infection of the central nervous system of K18-Human ACE2 Transgenic Mice. J. Virol. 96:e01969–21. doi: 10.1128/jvi.01969-21
Özdağ Acarli, A. N., Samanci, B., Ekizoğlu, E., Çakar, A., Şirin, N. G., Gündüz, T., et al. (2020). Coronavirus Disease 2019 (COVID-19) from the point of view of neurologists: observation of neurological findings and symptoms during the combat against a pandemic. Noro Psikiyatr. Ars. 57, 154–159. doi: 10.29399/npa.26148
Paniz-Mondolfi, A., Bryce, C., Grimes, Z., Gordon, R. E., Reidy, J., Lednicky, J., et al. (2020). Central nervous system involvement by severe acute respiratory syndrome coronavirus-2 (SARS-CoV-2). J. Med. Virol. 92, 699–702. doi: 10.1002/jmv.25915
Panoskaltsis, N., McCarthy, N. E., Stagg, A. J., Mummery, C. J., Husni, M., Arebi, N., et al. (2021). Immune reconstitution and clinical recovery following anti-CD28 antibody (TGN1412)-induced cytokine storm. Cancer Immunol. Immunother. 70, 1127–1142. doi: 10.1007/s00262-020-02725-2
Patatian, A., and Benech, P. (2020). Identifying potential clues on Covid-19 through coronavirus-related literature using a data-driven approach with the help of a text mining-based software, Predictsearch. Int. J. Sci. Res. Publ. 10, 2250–3153. doi: 10.29322/IJSRP.10.09.2020.p10560
Poldrack, R. A. (2006). Can cognitive processes be inferred from neuroimaging data? Trends Cogn. Sci. 10, 59–63. doi: 10.1016/j.tics.2005.12.004
Poyiadji, N., Shahin, G., Noujaim, D., Stone, M., Patel, S., and Griffith, B. (2020). COVID-19-associated acute hemorrhagic necrotizing encephalopathy: imaging features. Radiology 296, E119–E120. doi: 10.1148/radiol.2020201187
Ragab, D., Salah Eldin, H., Taeimah, M., Khattab, R., and Salem, R. (2020). The COVID-19 Cytokine Storm; What We Know So Far. Front. Immunol. 11:1446. doi: 10.3389/fimmu.2020.01446
Ren, X., Wang, S., Chen, X., Wei, X., Li, G., Ren, S., et al. (2020). Multiple Expression Assessments of ACE2 and TMPRSS2 SARS-CoV-2 Entry Molecules in the Urinary Tract and Their Associations with Clinical Manifestations of COVID-19. Infect. Drug Resist. 13, 3977–3990. doi: 10.2147/IDR.S270543
Rogers, J. P., Chesney, E., Oliver, D., Pollak, T. A., McGuire, P., Fusar-Poli, P., et al. (2020). Psychiatric and neuropsychiatric presentations associated with severe coronavirus infections: a systematic review and meta-analysis with comparison to the COVID-19 pandemic. Lancet Psychiatry 7, 611–627. doi: 10.1016/S2215-0366(20)30203-0
Sahoo, S., Mehra, A., Dua, D., Suri, V., Malhotra, P., Yaddanapudi, L. N., et al. (2020). Psychological experience of patients admitted with SARS-CoV-2 infection. Asian J. Psychiatr. 54:102355. doi: 10.1016/j.ajp.2020.102355
Sindona, C., Schepici, G., Contestabile, V., Bramanti, P., and Mazzon, E. (2021). NOX2 Activation in COVID-19: possible implications for neurodegenerative diseases. Medicina 57:604. doi: 10.3390/medicina57060604
Song, E., Zhang, C., Israelow, B., Lu-Culligan, A., Prado, A. V., Skriabine, S., et al. (2021). Neuroinvasion of SARS-CoV-2 in human and mouse brain. J. Exp. Med. 218:e20202135. doi: 10.1084/jem.20202135
Soy, M., Keser, G., Atagündüz, P., Tabak, F., Atagündüz, I., and Kayhan, S. (2020). Cytokine storm in COVID-19: pathogenesis and overview of anti-inflammatory agents used in treatment. Clin. Rheumatol. 39, 2085–2094. doi: 10.1007/s10067-020-05190-5
Sulzer, D., Antonini, A., Leta, V., Nordvig, A., Smeyne, R. J., Goldman, J. E., et al. (2020). COVID-19 and possible links with Parkinson’s disease and parkinsonism: from bench to bedside. NPJ Parkinsons Dis. 6:18. doi: 10.1038/s41531-020-00123-0
Sutherland, L. C., Rintala-Maki, N. D., White, R. D., and Morin, C. D. (2005). RNA binding motif (RBM) proteins: a novel family of apoptosis modulators? J. Cell. Biochem. 94, 5–24. doi: 10.1002/jcb.20204
Sy, M., Kitazawa, M., Medeiros, R., Whitman, L., Cheng, D., Lane, T. E., et al. (2011). Inflammation induced by infection potentiates tau pathological features in transgenic mice. Am. J. Pathol. 178, 2811–2822. doi: 10.1016/j.ajpath.2011.02.012
Talairach, J., and Tournoux, P. (1993). Referentially Oriented Cerebral MRI Anatomy: An Atlas of Stereotaxic Anatomical Correlations for Gray and White Matter. New York, NY: Thieme Medical Publishers.
Taquet, M., Geddes, J. R., Husain, M., Luciano, S., and Harrison, P. J. (2021). 6-month neurological and psychiatric outcomes in 236 379 survivors of COVID-19: a retrospective cohort study using electronic health records. Lancet Psychiatry 8, 416–427. doi: 10.1016/S2215-0366(21)00084-5
Toscano, G., Palmerini, F., Ravaglia, S., Ruiz, L., Invernizzi, P., Cuzzoni, M. G., et al. (2020). Guillain-Barré syndrome associated with SARS-CoV-2. N. Engl. J. Med. 382, 2574–2576. doi: 10.1056/NEJMc2009191
Widyadharma, I. P. E., Sari, N. N. S. P., Pradnyaswari, K. E., Yuwana, K. T., Adikarya, I. P. G. D., Tertia, C., et al. (2020). Pain as clinical manifestations of COVID-19 infection and its management in the pandemic era: a literature review. Egypt. J. Neurol. Psychiatr. Neurosurg. 56:121. doi: 10.1186/s41983-020-00258-0
Xydakis, M. S., Dehgani-Mobaraki, P., Holbrook, E. H., Holbrook, E. H., Geisthoff, U. W., Bauer, C., et al. (2020). Smell and taste dysfunction in patients with COVID-19. Lancet Infect. Dis. 20, 1015–1016. doi: 10.1016/S1473-3099(20)30293-0
Yan, R., Zhang, Y., Li, Y., Xia, L., Guo, Y., and Zhou, Q. (2020). Structural basis for the recognition of SARS-CoV-2 by full-length human ACE2. Science 367, 1444–1448. doi: 10.1126/science.abb2762
Yarkoni, T., Poldrack, R. A., Nichols, T. E., Van Essen, D. C., and Wager, T. D. (2011). Large-scale automated synthesis of human functional neuroimaging data. Nat. Methods 8, 665–670. doi: 10.1038/nmeth.1635
Yarkoni, T., Poldrack, R. A., Van Essen, D. C., and Wager, T. D. (2010). Cognitive neuroscience 2.0: building a cumulative science of human brain function. Trends Cogn. Sci. 14, 489–496. doi: 10.1016/j.tics.2010.08.004
Keywords: ACE2, COVID-19, multi-data integration, neurological symptom, SARS-CoV-2, TMPRSS2, LinkRbrain
Citation: Mesmoudi S, Lapina C, Rodic M and Peschanski D (2022) Multi-Data Integration Towards a Global Understanding of the Neurological Impact of Human Brain Severe Acute Respiratory Syndrome Coronavirus 2 Infection. Front. Integr. Neurosci. 16:756604. doi: 10.3389/fnint.2022.756604
Received: 10 August 2021; Accepted: 30 May 2022;
Published: 13 July 2022.
Edited by:
Philip Tseng, Taipei Medical University, TaiwanReviewed by:
Elias Manjarrez, Meritorious Autonomous University of Puebla, MexicoJian-Ying Chuang, Taipei Medical University, Taiwan
Copyright © 2022 Mesmoudi, Lapina, Rodic and Peschanski. This is an open-access article distributed under the terms of the Creative Commons Attribution License (CC BY). The use, distribution or reproduction in other forums is permitted, provided the original author(s) and the copyright owner(s) are credited and that the original publication in this journal is cited, in accordance with accepted academic practice. No use, distribution or reproduction is permitted which does not comply with these terms.
*Correspondence: Salma Mesmoudi, salma.mesmoudi@univ-paris1.fr
†These authors have contributed equally to this work