- 1Palestinian Neuroscience Initiative, Faculty of Medicine, Al-Quds University, Jerusalem, State of Palestine
- 2Center for Molecular and Behavioral Neuroscience, Rutgers University, Newark, NJ, USA
- 3Marcs Institute for Brain and Behavior and School of Social Sciences and Psychology, Western Sydney University, Sydney, NSW, Australia
Anxiety disorders, including generalized anxiety disorder (GAD), social anxiety disorder (SAD), and panic anxiety disorder (PAD), are a group of common psychiatric conditions. They are characterized by excessive worrying, uneasiness, and fear of future events, such that they affect social and occupational functioning. Anxiety disorders can alter behavior and cognition as well, yet little is known about the particular domains they affect. In this study, we tested the cognitive correlates of medication-free patients with GAD, SAD, and PAD, along with matched healthy participants using a probabilistic category-learning task that allows the dissociation between positive and negative feedback learning. We also fitted all participants' data to a Q-learning model and various actor-critic models that examine learning rate parameters from positive and negative feedback to investigate effects of valence vs. action on performance. SAD and GAD patients were more sensitive to negative feedback than either PAD patients or healthy participants. PAD, SAD, and GAD patients did not differ in positive-feedback learning compared to healthy participants. We found that Q-learning models provide the simplest fit of the data in comparison to other models. However, computational analysis revealed that groups did not differ in terms of learning rate or exploration values. These findings argue that (a) not all anxiety spectrum disorders share similar cognitive correlates, but are rather different in ways that do not link them to the hallmark of anxiety (higher sensitivity to negative feedback); and (b) perception of negative consequences is the core feature of GAD and SAD, but not PAD. Further research is needed to examine the similarities and differences between anxiety spectrum disorders in other cognitive domains and potential implementation of behavioral therapy to remediate cognitive deficits.
Introduction
Anxiety disorders are a group of common psychiatric conditions. They are characterized by excessive worry, uneasiness, and fear of future events, such that they affect social and occupational functioning. The DSM-V identifies several forms of anxiety within this spectrum based on distinct phenomenological patterns (Rabe-Jablonska and Bienkiewicz, 1994; APA, 2013) including generalized anxiety disorder (GAD), social anxiety disorder (SAD), and panic anxiety disorder (PAD). Nearly one in three individuals will develop an anxiety spectrum disorder during their lifetime (Kessler et al., 2005). The most common form is specific phobias (lifetime prevalence of 12.5%), followed by SAD (12.1%), GAD (5.7%), and PAD (4.7%) (Kessler et al., 2005; Porcelli et al., 2012).
One of the most important features of anxiety disorders is their cognitive component. This component is exhibited in the form of biased information processing of novel social situations as well as specific and general threats. For instance, the exposure of SAD patients to ordinary social settings induces selective retrieval of past negative social memories. This leads to negative interpretation and response to current events in SAD (Grupe and Nitschke, 2013) as well as PAD (Gladsjo et al., 1998; Dupont et al., 2000; Lautenbacher et al., 2002). This excessive worrying about stimuli and threats may exhibit generalized avoidance and safety behaviors (Clark and Wells, 1995). As a consequence of generalized avoidance, repeated exposure to normal life events produces strong feelings of fear that persist and maintain anxiety for years (Salkovskis et al., 1991; Clark and Wells, 1995; Barlow, 2002).
Despite the significant role of cognitive biases in the psychopathology of anxiety disorders (Mathews and MacLeod, 1994; Beck and Clark, 1997; Heinrichs and Hofmann, 2001), the different cognitive domains, aversively motivated reinforcement learning and instrumental avoidance in particular, are under-investigated (Airaksinen et al., 2005). In comparison, impairments in the different cognitive functions have been extensively explored in the less prevalent anxiety disorders such as Obsessive Compulsive Disorder (Greisberg and McKay, 2003; Kuelz et al., 2004; Muller and Roberts, 2005) and Post-Traumatic Stress Disorder (Golier and Yehuda, 2002; Horner and Hamner, 2002). The majority of studies on cognitive functions in PAD showed impairments in verbal episodic memory and executive functioning (Airaksinen et al., 2005), divided attention (Lautenbacher et al., 2002), short-term verbal memory and learning (Asmundson et al., 1994), verbal long-term memory (Lucas et al., 1991), and visual memory (Lucas et al., 1991; Boldrini et al., 2005). In SAD patients, several studies reported deficits in attentive, executive, and visuo-spatial functions (Cohen et al., 1996), in short-term verbal memory (Asmundson et al., 1994), and in avoidance learning (Ly and Roelofs, 2009). In contrast to these findings, other studies found no evidence of most of these impairments in PAD and GAD (Gladsjo et al., 1998; Purcell et al., 1998a,b). Cognitive impairments in GAD are the least addressed in anxiety disorders. The few studies in this area of research have found no correlation between cognitive deficits and GAD (Zalewski et al., 1994; Airaksinen et al., 2005).
Unfortunately, many of the aforementioned cognitive studies reported general findings with contradictory conclusions. Few studies investigated learning from positive and negative feedback in anxiety disorders. Subjects with high anxiety traits showed higher avoidance of negative feedback compared to healthy controls in the context of Pavlovian and instrumental conditioning (Lovibond et al., 2008; Ly and Roelofs, 2009; Vriends et al., 2012; Cremers et al., 2014).
In neurochemical terms, converging evidence has associated dopamine-dependent pathways in the ventral region of the striatum with motivational processing (Haber and Knutson, 2010). In addition, strong evidence suggests that dopamine and serotonin modulate processing of negative feedback (Henkel et al., 2002; Pariante and Lightman, 2008; Moustafa et al., 2013).
Computational models, such as Q-learning, have been used to fit behavioral data (Frank et al., 2007a; Moustafa and Maida, 2007; Rutledge et al., 2009). More recently, actor-critic models (Barto, 1995; Dayan and Balleine, 2002) have provided good fits to behavioral results (Collins et al., 2014). Computational and experimental investigations argue that the ventral and dorsal striatum (particularly the caudate nucleus) play dissociable roles in learning and decision-making. Specifically, many argue that the critic corresponds to the ventral striatum while the actor corresponds to the dorsal striatum (Cardinal et al., 2002; O'Doherty et al., 2004; Guitart-Masip et al., 2014).
In this study, we examined the cognitive effects of the three subtypes of anxiety disorder on learning from positive versus negative feedback. To our knowledge, this might be the first study to address feedback learning in these three disorders in one study using one cognitive task. We tested patients with GAD, SAD, PAD off their medications along with matched healthy controls, using a category-learning task that allows the dissociation between learning from positive and negative feedback (Bódi et al., 2009; Herzallah et al., 2013). In addition, we applied Q-learning and actor-critic models to behavioral data to test the effects of valence vs. action in learning performance. As in our prior models, the actor-critic model in its most extended form included four free parameters, dealing with both learning from positive and negative feedback in both critic and actor. These parameters are related to learning from positive vs. negative prediction error in the critic as well as learning from positive vs. negative prediction error in the actor. We additionally explored the role played by noise and action selection parameters using various other models.
Methods
Participants
We recruited 73 eligible participants from the clinics associated with Cairo and Ain Shams Universities. The participant groups were: GAD (n = 18), SAD (n = 20), PAD (n = 17), or Healthy Controls (HCs; n = 18). HC were either partners of patients or were recruited from the community. All participants underwent clinical diagnostic DSM-IV-TR interviews and strictured clinical interviews using the Mini International Neuropsychiatric Interview (MINI; Amorim et al., 1998) to confirm the diagnosis and absence of comorbidities prior to cognitive testing. Participants' age ranged from 30 to 60 years. Participants were group matched for age (M = 43.10; SD = 5.54), gender (41 males and 32 females), years of education (M = 11.93; SD = 2.99), and disease duration (M = 12.87; SD = 3.66) as shown in Table 1. Inclusion criteria for HC included absence of any psychiatric, neurological, or other disorders that might affect cognition.
Exclusion criteria for all participants included psychotropic drug exposure; major medical, or neurological illness; illicit drug use or alcohol abuse within the past year; lifetime history of alcohol or drug dependence; psychiatric disorders other than the three anxiety disorders; current pregnancy, or breastfeeding. After receiving a complete description of the study, participants provided written informed consent as approved and conformed by both the Ethics committee and the guidelines for protection of human participants.
Neuropsychological Test Battery
All participants completed the Arabic version of neuropsychological tests by the clinicians: the North American Adult Reading Test (NAART; Uttl, 2002), the Wechsler Adult Intelligence Scale—Revised (WAIS-R) Digit Span test (Forward and Backward; Schroeder et al., 2012). Further, all participants completed the Hamilton Anxiety Rating Scale (HAM-A; Hamilton, 1959) to rate the severity of a participant's anxiety.
There was no significant difference between groups in NAART scores (Kruskal-Wallis H = 0.201, df = 3, p = 0.977). However, HAM-A differed significantly across groups (Kruskal-Wallis H = 43.731, df = 3, p < 0.001). Tukey's HSD on HAM-A results revealed significant differences between the control group and PAD, SAD and Gad (p < 0.001), but no significant differences between the three disease groups (PAD, SAD, and GAD; p > 0.05). We used the Kruskal-Wallis test to compare the WAIS forward digit, and WAIS backward digit among groups, which showed no significant effect of group (Forward digit: H = 5.071, df = 3, p = 0.167; Backward digit: H = 1.602, df = 3, p = 0.659), respectively.
Computer-Based Cognitive Task
Learning from Positive and Negative Feedback
All participants were administered a computer-based probabilistic classification task (Bódi et al., 2009). On each trial, participants viewed one of four images (Figure 1), and were asked to guess whether it belonged to category A or B. On any given trial, stimuli S1 and S3 belonged to category A with 80% probability and to category B with 20% probability, while stimuli S2 and S4 belonged to category B with 80% probability and to category A with 20% probability (Table 2). Stimuli S1 and S2 were used in the positive-feedback learning task. Two stimuli per valence were employed in order to balance category outcome frequencies, so that one stimulus in each task would be associated with each outcome. Thus, if the participant correctly guessed category membership on a trial with either of these stimuli, a positive feedback of +25 points was received; if the participant guessed incorrectly, no feedback appeared. Stimuli S3 and S4 were used in the negative-feedback learning task. Thus, if the participant guessed incorrectly on a trial with either of these stimuli, a negative feedback of −25 was received; correct guesses received no feedback.
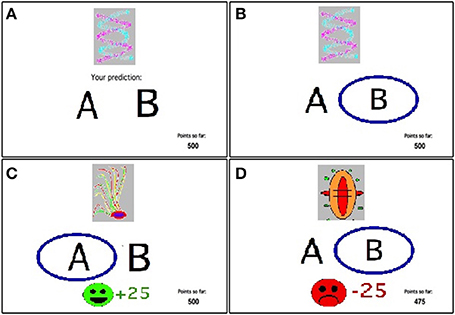
Figure 1. The feedback-based probabilistic classification task. (A) On each trial, the participant sees one of four stimuli and is asked whether this stimulus predicts rain or sun. (B) No feedback is given for incorrect answers in positive feedback stimuli or correct answers in negative feedback stimuli. (C) For positive feedback stimuli, correct responses get rewarded with visual feedback and 25 point winnings. (D) For negative feedback stimuli, incorrect responses get punished with visual feedback and the loss of 25 points.
The experiment was conducted on a Macintosh Macbook, programmed in the SuperCard language. The participant was seated in a quiet testing room at a comfortable viewing distance from the screen. The keyboard was masked except for two keys, labeled “A” and “B” that the participant could use to enter responses. Participants first completed a practice phase which walked the participant through an example of a correct and an incorrect response to a sample trial in the negative-feedback learning task and an example of a correct and incorrect response to a sample trial in the positive-feedback learning task. These examples used images other than those assigned to S1–S4. The actual task contained 160 trials, separated and randomized into four blocks. Trials were separated by a 2 s interval, during which time the screen was blank. Within each block, each stimulus appeared 10 times, 8 times with the more common outcome (e.g. category ‘A’ for S1 and S3 and ‘B’ for S2 and S4) and 2 times with the less common outcome. Thus, training on the positive-feedback learning task (S1 and S2) and negative-feedback learning task (S3 and S4) were intermixed. The no-feedback outcome, when it arrived, was ambiguous, as it could signal lack of positive feedback (if received during a trial with S1 or S2) or lack of negative feedback (if received during a trial with S3 or S4). At the end of the 160 trials, if the participant's running tally of points was less than 500 (i.e., no more than the points awarded at the start of the experiment), additional trials were added on which the participant's response was always taken as correct, until the tally was at least 525. This was done in an attempt to minimize frustration in participants by ensuring that all participants terminated the experiment with more points than they had started with. Data from any such additional trials were not analyzed. On each trial, the computer recorded whether the participant made the optimal response (i.e., Category A for S1 and S3 and Category B for S2 and S4) regardless of actual outcome.
Statistical Analysis
The normality of data distribution was checked using Kolmogorov–Smirnov tests; age, disease duration, overall learning from positive feedback, overall learning from negative feedback, and learning from negative feedback in the 4th block were normally distributed (p > 0.05) and so ANOVA was conducted. Kruskal-Wallis test was used to analyze the data that were not normally distributed (p < 0.05); Education, HAM-A, NAART, Forward digit, Backward digit, and learning from positive feedback in the 4th block.
For the computer-based cognitive task we used mixed-design two way ANOVA, followed by one-way ANOVA and post-hoc tests, Bonferroni post-hoc tests. The level of significance was set at α = 0.05.
Computational Model
We fit each participant's behavioral data using Q-learning and various actor-critic models (see Table 3 for models used), and used a hierarchical Bayesian procedure for estimating model parameter values for each participant (for more details, see Piray et al., 2014).
The actor-critic models used here had different learning rates for positive and negative prediction errors for updating critic's values and actor's decision. So, the models had two critic's learning rates: and ; and two actor's learning rates: and . The model estimates the value of stimulus-outcome pairs for each participant separately. On each trial t the value of the observed stimulus, st, and the chosen response, ct, is updated according to the following rules:
where α+ and α− are the learning rates for positive and negative prediction error, respectively, which determine the degree that recent prediction error affects expected value. Increasing evidence shows that dissociation between learning rates for positive and negative prediction error has a plausible neural substrate (Frank et al., 2007a; Rutledge et al., 2009). δt is the prediction error signal, which is the discrepancy between actual outcome, ot, and expected value:
The probability of chosen option is then computed using softmax equation:
where p(ct = A|st) and p(ct = B|st) are the probabilities of choosing A and B, respectively. β is a noise parameter. Ct(st, A) and Ct(st, B) represents the choice of A and B on the last presentation of st, respectively. Thus, Ct(st, A) = 1 and Ct(st, B) = 0 if A has been chosen in the previous presentation of st before trial t and otherwise if B has been chosen, Ct(st, A) = 0 and Ct(st, B) = 1. So, φ determines how much previous choices, independent of reward history, affect current choice. While positive values of φ represent a tendency to perseverate on previous choices, negative values represent tendency to switch more between options. As shown in Table 3, we ran two different actor-critic models (with fixed and variable noise parameters), as well as additional models for comparison.
Formally, the prediction error signal here is computed according to the following equation:
where Vt(st) is the critic's expected value for st. Then, the critic's value is updated using prediction error signal:
where αc is the critic's learning rate. The prediction error is also conveyed to the actor for updating preferences of the actor for the selected choice:
where αa is the actor's learning rate. Here, the probability of each choice is computed according to actor's preferences:
Further, we fit the data to two simpler models: first, a Q-learning model (Watkins and Dayan, 1992; Sutton and Barto, 1998; Frank et al., 2007b) where there is only one learning rate. The prediction error is therefore calculated based on the values of the stimulus-selected action pair, as opposed to the value of the stimulus independent of the selected action (as in the actor critic model). This model therefore has two free parameters: , β.
Now, we describe the procedure of data fitting. For each subject, we fit the data to each of the models described above. We find best parameter values for each subject. The search for parameter values is conducted using Matlab function fmincon.
After parameter values are determined, for each subject under each model, model fit was assessed by computing negative log likelihood estimates (negLLE) to estimate the a priori probability of the data, given a particular combination of parameter values (see Frank et al., 2007b).
where r is the response made by the subject on trial t [i.e., Pr(r, t)] is the probability that the model makes the same response as the subject on that trial). Estimated parameters for each participant (under each model) were defined as the parameter values of that together resulted in the lowest negLLE for that participant's data.
To compare models, we used the Bayesian Information Criterion (BIC; Schwartz, 1978; Moustafa et al., 2015a,b; Myers et al., 2016), which penalizes models with more free parameters:
where k is the number of free parameters and n is the number of observations (here, n = 160); lower values of BIC indicate fewer explanatory variables, better fit, or both (Table 4). We then used the random effects Bayesian model selection procedure (Stephan et al., 2009; Penny et al., 2010) which takes into account the possibility that different models may have generated different subjects' data, to generate expected posterior probabilities for each model.
Results
Behavioral Results
We used one-sample t-test on the mean accuracy across blocks in both positive- and negative-feedback, with Bonferroni corrected α = 0.0125 to protect the level of significance, to ensure that participants learned significantly better than chance in different groups. In positive-feedback learning, participants in all groups learned significantly better than chance except GAD and SAD [GAD: t(17) = 2.62, p = 0.018; PAD: t(16) = 2.87, p = 0.011; SAD: t(19) = 2.75, p = 0.013; HC: t(17) = 3.77, p = 0.002]. In negative-feedback learning, all groups learned significantly better than chance [GAD: t(17) = 9.48, p < 0.001; PAD: t(16) = 3.23, p = 0.005; SAD: t(19) = 13.47, p < 0.001; HC: t(17) = 6.52, p < 0.001].
Using mixed-design three-way ANOVA, we analyzed the data obtained from the cognitive task with group as the between-subject variable, learning block and feedback type as within-subject variables, and the number of optimal responses on positive and negative-feedback as the dependent variables. There was a significant effect of group [F(3, 69) = 3.036, p = 0.035, η2 = 0.117] and block [F(3, 207) = 6.425, p < 0.001, η2 = 0.085], along with an interaction between feedback type and group [F(3, 69) = 2.797, p = 0.047, η2 = 0.108] and between feedback type, group, and block [F(9, 207) = 3.211, p = 0.001, η2 = 0.122]. However, there was neither a significant effect of feedback type [F(1, 69) = 2.683, p = 0.106] nor an interaction between block and feedback type [F(3, 207) = 1.656, p = 0.178] or between group and block [F(9, 207) = 1.499, p = 0.150]. To examine the interaction between feedback type and group, we used two mixed-design ANOVA post-hoc tests to analyze data from positive-feedback and negative-feedback trials separately, with block as the within-subject variable, and group as the between-subject variable. The first mixed-design ANOVA on positive-feedback trials revealed no significant effect of group [F(3, 69) = 0.089, p = 0.966] and no interaction between group and block [F(3, 207) = 1.708, p = 0.089], as illustrated in Figures 2A, 3A. However, there was a significant effect of block [F(3, 207) = 4.684, p = 0.003, η2 = 0.064]. Results of the mixed-design ANOVA on negative-feedback trials showed a significant effect of group [F(3, 69) = 12.423, p < 0.001, η2 = 0.351], block [F(3, 207) = 3.746, p = 0.012, η2 = 0.051] and interaction between group and block [F(3, 207) = 2.871, p = 0.003, η2 = 0.111]. Tukey's HSD post-hoc analysis revealed a significant difference between GAD and HC, GAD and PAD, SAD and HC, and SAD and PAD (p < 0.001), but not between GAD and SAD, or PAD and HC (p > 0.5), as illustrated in Figures 2B, 3B.
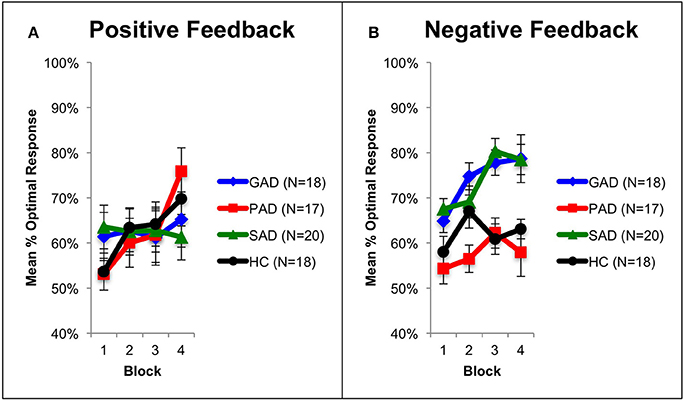
Figure 2. Performance on the positive and negative feedback learning task. (A) The mean number of optimal responses in the four phases for positive feedback stimuli (+SEM). (B) The mean number of optimal responses in the four phases for negative feedback stimuli (+SEM). PAD is Panic Anxiety Disorder, SAD is Social Anxiety Disorder; GAD is Generalized Anxiety Disorder, and HC is healthy controls.
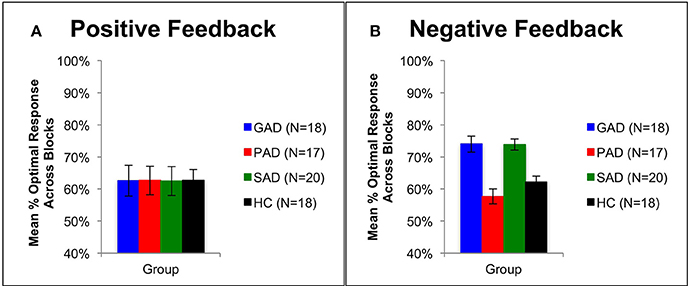
Figure 3. Performance on the positive and negative feedback learning task. (A) The mean number of correct responses across blocks for the positive feedback stimuli (+SEM). (B) The mean number of correct responses across blocks for the negative feedback stimuli (+SEM). PAD is Panic Anxiety Disorder, SAD is Social Anxiety Disorder; GAD is Generalized Anxiety Disorder, and HC is healthy controls.
Computational Results
First, we computed negLLE to find model with best fit to the data. Average model fit, defined in terms of BIC and negLLE is shown for each model in Table 4. Although all models were similarly successful in fitting individual subject data, as indicated by comparable negLLE, BIC values showed that the Q-learning model provided the simplest fit of the data.
Using two-way mixed-design ANOVA, we analyzed results obtained from fitting of the behavioral data to the Q-learning model. The model parameters (learning rate and exploration) were the within-subject variables, while group (GAD, PAD, SAD, HC) was the between-subject variable. There was a significant difference between the model parameters [F(1, 69) = 172.295, p < 0.001, η2 = 0.714], but neither a significant effect of group [F(3, 69) = 0.035, p = 0.991] nor significant interaction between model parameters and group [F(3, 69) = 0.064, p = 0.979]. To further explore the significant difference between parameters, we used two one-way ANOVAs (with Bonferroni corrected α = 0.025 to protect the level of significance) to analyze results from the two model parameters separately with group as the independent variable. There was no effect of group on the learning rate [F(3, 69) = 2.201, p = 0.096, Figure 4A] or exploration parameter [F(3, 69) = 0.036, p = 0.991, Figure 4B].
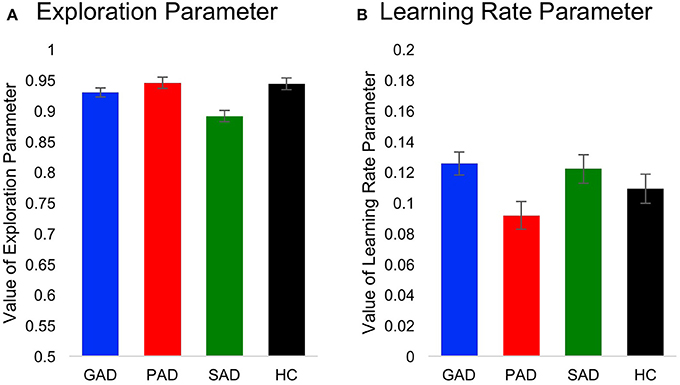
Figure 4. Computational Q-learning model results. Here, we show values for (A) the learning rate parameter and (B) the exploration parameter across groups.
Discussion
To our knowledge, this is the first study to investigate learning from positive and negative feedback across multiple patient groups with anxiety disorders. We found that SAD and GAD patients learned better from negative feedback than either PAD patients or HC participants. However, patient groups did not differ in positive-feedback learning from HC participants. These results suggest a cognitive dissociation between subtypes of anxiety spectrum disorders, which might underlie a difference in the involved neural circuits in these disorders. Results of our computational Q-learning modeling indicated that enhanced learning from negative feedback in patients with GAD and SAD is not attributed to group differences in speed of learning or ability to explore available outcomes.
Behavioral and Neural Correlates of Anxiety Disorders
We observed differences in participants' performance between block 1 and 4 across the different groups (Figure 2). These differences are evident of an overall learning trend by all participants. In addition, we observed that SAD and GAD patients performed significantly better in negative feedback learning compared to HC. While PAD patients did not differ to HC. Therefore, SAD and GAD patients tend to avoid punishment when exposed to ambiguous stimuli, more than PAD patients do. This behavioral bias (hypersensitivity) toward negative feedback in SAD and GAD patients can be explained by the over-evaluation of the ambiguous stimuli, observed in block 1. This in turn necessitates patients to adopt a negative-feedback avoidance strategy to reach the optimal answer. Eventually, this over-evaluation leads to faster learning by SAD and GAD patients as observed in block 4 (Figure 2B). This implies that SAD and GAD patients utilized avoidance learning as a coping mechanism to reduce exposure to negative feedback and thereby counteract anxiety. These behavioral results are consistent with results reported by Lovibond et al. (2008) indicating that the tendency of healthy participants to utilize avoidance behavior is higher in anxious situations. Along the same lines, Ly and Roelofs (2009) revealed that subjects with high socially anxious displayed increased sensitivity to negative feedback. Further, Cremers et al. (2014) found that SAD patients exhibited increased tendency for anticipation of social punishment than social reward. Additionally, Cremers et al. (2014) also reported higher striatal activation to social punishment when compared to social reward.
Past studies suggested the involvement of VTA and its projections to NAc in the processing of aversive stimuli (Wise, 2004; Schultz, 2006). Further, it was shown that stressful and anxious events activate the mesolimbic dopamine pathway (Tidey and Miczek, 1996; Anstrom and Woodward, 2005; Berton et al., 2006; Brischoux et al., 2009; Ungless et al., 2010). Aversive and stressful events are also clearly capable of enhancing dopaminergic function within the mesolimbic system (Anstrom and Woodward, 2005; Berton et al., 2006; Brischoux et al., 2009; Tidey and Miczek, 1996; Ungless et al., 2010).
Aside from dopamine, Deakin and Graeff's theory proposed that serotonergic neuronal activity in the dorsal raphe nucleus facilitates anxiety-like behavior (Deakin and Graeff, 1991). These modulatory actions of serotonin would only be expressed under stressful situations. Deakin and Graeff's theory conjugates inhibitory avoidance and risk assessment to the symptomatology of GAD and SAD (Deakin and Graeff, 1991). Pharmacological studies have shown that anxiolytic drugs like benzodiazepines are effective in the treatment of GAD (Baldwin and Polkinghorn, 2005). Genetic studies indicate that GAD and SAD patients who carry the low activity allele of the serotonin transporter gene (and hence have higher serotonin) show increased sensitivity to fearful and stressful experiences (Furmark et al., 2004; van der Wee et al., 2008). This is inline with our results showing that GAD and SAD patients learned efficiently from negative feedback.
In our study, PAD patients learned similar to healthy controls from negative feedback, but lower than SAD and GAD patients. Results from PAD patients opposed our conjecture based on our retrospective review of literature. In support of our initial hypothesis, Vriends et al. (2012) showed that patients with phobias exhibited higher susceptibility for avoidance behavior, and more biased discrimination of negative stimuli compared to positive ones. In addition, Asmundson et al. (1994) and Lucas et al. (1991) reported impairments in verbal learning, supported by divided attention deficits in PAD patients (Lautenbacher et al., 2002). However, other studies found no evidence of these impairments in PAD (Gladsjo et al., 1998; Purcell et al., 1998a,b). In the light of previous studies, Deakin and Graeff's theory postulated that serotonergic neuronal activity in the dorsal raphe nucleus facilitates anxiety-like behavior, but inhibits fear (Deakin and Graeff, 1991). Hence, selective serotonin reuptake inhibitors are very effective in the treatment of PAD (Bakker et al., 2005). Genetic studies indicate that PAD patients showed no difference between the two alleles of the serotonin transporter gene in fearful and stressful experiences (Blaya et al., 2007; Strug et al., 2010). The difference in PAD patients performance might be attributed to a heightened fear learning effect that might involve the amygdala and the hippocampus (Gorman et al., 2000), rather than the striatum and VTA (LeDoux et al., 1988, 1990; Davis, 1992; Phillips and LeDoux, 1992).
The three patient groups showed no difference compared to healthy controls in learning from positive feedback (Figure 2A). These results also opposed our predictions, where we expected that anxiety disorder patients would be impaired in positive feedback learning as supported by the literature. Richey et al. (2014) investigated social and non-social reward in SAD patients using fMRI. They found that SAD patients were impaired in both social and non-social rewards. In comparison, NAc was differentially activated in both types of reward, where patients showed NAc hyperactivation in non-social reward and hypoactivation in social reward. Similarly, Cremers et al. (2014) showed that healthy participants exhibited a motivational preference for social reward, which was absent in SAD patients. In addition, ACC connectivity with striatum decreased in reward trials in SAD patients.
Discrepancies in cognitive functions impairments between GAD and SAD patients have been examined in various studies. Neuroimaging and behavioral studies have shown that patients with GAD and SAD had different responses to social-affective stimuli (Becker et al., 2001; Mennin et al., 2005; Blair et al., 2008). GAD patients were slower than SAD patients in recalling emotional words, while SAD patients were impaired on recognition of speech-related words (Becker et al., 2001). Further, patients with GAD usually report greater emotion intensity and fear of experience than patients with SAD, while patients with SAD are less expressive of positive emotions (Mennin et al., 2005). However, our results showed no difference between GAD and SAD patients in learning from positive or negative feedback.
Computational Analysis of Cognitive Function in Anxiety Disorders
The benefits of fitting computational models to cognitive data is to disentangle behavioral performance into different components, and thus allows a better understanding of the exact information processing mechanism underlying performance. For example, prior fitting models were applied to neurogenetics (Frank et al., 2007b), neuroimaging (Daw et al., 2006), and animal studies (Beeler et al., 2010).
Fitting our behavioral data using a Q-learning model allowed us to tease apart speed of learning from the ability to explore available options. In other words, abnormal performance in the positive and negative feedback task can be due to either slowness to learn stimulus-outcome associations or stimulus valence, or the inability to explore other available outcomes when the outcome in the current trial is not rewarding. By fitting the Q-learning model to the behavioral data, we found that enhanced learning from negative feedback in patients with GAD and SAD is not attributed to a difference in learning rate or exploration. Our data suggest that enhanced focus on the negative in these anxiety disorders is associated with increased stimulus valence and attentional focus on the negative and not necessarily linked to the speed of learning or ability of explore other available options.
A limitation to our study arises from the fact that all recruited subjects in the SAD, GAD, and PAD groups were tested off medications. With the current dataset, we cannot assess the effect of anxiolytic medications affected on feedback-based learning. Another potential limitation of the current study is the low number of recruited subjects. Future studies, however, should address these limitations and better control for possible confounding variables.
In conclusion, our results argue that not all anxiety spectrum disorders share the same cognitive correlates, but are rather different in ways that do not link them to the hallmark of anxiety (higher sensitivity to negative feedback). Further, research is needed to examine the similarities and differences between anxiety spectrum disorders in other cognitive domains. Further, numerous behavioral studies have shown a similar bias to processing negative feedback in patients with major depressive disorder (Hirschfeld, 2001; Eshel and Roiser, 2010; Herzallah et al., 2013), and major depressive disorder is a common comorbidity with anxiety disorders (Sartorius et al., 1996). Therefore, future studies will examine the effects of comorbid depression on cognitive function in patients with anxiety spectrum disorders.
Author Contributions
HK: Data analysis and manuscript writing. OA: Manuscript writing. IM: Manuscript writing. AI: Data analysis and manuscript writing. MG: Manuscript writing. MH: Data analysis and manuscript writing. AM: Research design, computational modeling, and manuscript writing.
Conflict of Interest Statement
The authors declare that the research was conducted in the absence of any commercial or financial relationships that could be construed as a potential conflict of interest.
The handling Editor declared a shared affiliation, though no other collaboration, with three of the authors HK, MG, MH, and states that the process nevertheless met the standards of a fair and objective review.
Acknowledgments
We thank Payam Piray for assistance with Matlab code and models used in this paper. Also we thank Dr. Samih Darwazah (Hikma Pharmaceuticals LLC.), Mr. Ghiath Sukhtian (GMS Holdings), and Mr. Fadi Ghandour (Aramex) for supporting personnel involved in writing this research paper.
References
Airaksinen, E., Larsson, M., and Forsell, Y. (2005). Neuropsychological functions in anxiety disorders in population-based samples: evidence of episodic memory dysfunction. J. Psychiatr. Res. 39, 207–214. doi: 10.1016/j.jpsychires.2004.06.001
Amorim, P., Lecrubier, Y., Weiller, E., Hergueta, T., and Sheehan, D. (1998). DSM-IH-R Psychotic Disorders: procedural validity of the Mini International Neuropsychiatric Interview (MINI). Concordance and causes for discordance with the CIDI. Eur. Psychiatry 13, 26–34. doi: 10.1016/S0924-9338(97)86748-X
Anstrom, K. K., and Woodward, D. J. (2005). Restraint increases dopaminergic burst firing in awake rats. Neuropsychopharmacology 30, 1832–1840. doi: 10.1038/sj.npp.1300730
APA (2013). Diagnostic and Statistical Manual of Mental Disorders, 5th Edn. Washington, DC: American Pyschiatric Association.
Asmundson, G. J., Stein, M. B., Larsen, D. K., and Walker, J. R. (1994). Neurocognitive function in panic disorder and social phobia patients. Anxiety 1, 201–207.
Bakker, A., van Balkom, A. J., and Stein, D. J. (2005). Evidence-based pharmacotherapy of panic disorder. Int. J. Neuropsychopharmacol. 8, 473–482. doi: 10.1017/S1461145705005201
Baldwin, D. S., and Polkinghorn, C. (2005). Evidence-based pharmacotherapy of generalized anxiety disorder. Int. J. Neuropsychopharmacol. 8, 293–302. doi: 10.1017/S1461145704004870
Barlow, J. (2002). Antenatal anxiety, parenting and behavioural/emotional problems in children. Br. J. Psychiatry 181, 440–441; author reply 441. doi: 10.1192/bjp.181.5.440-a
Barto, A. G. (1995). “Adaptive critics and the basal ganglia,” in Models of Information Processing in the Basal Ganglia, eds J. C. Houk, J. L. Davis, and D. G. Beiser (Cambridge: MIT Press), 215–232.
Beck, A. T., and Clark, D. A. (1997). An information processing model of anxiety: automatic and strategic processes. Behav. Res. Ther. 35, 49–58. doi: 10.1016/S0005-7967(96)00069-1
Becker, E. S., Rinck, M., Margraf, J., and Roth, W. T. (2001). The emotional Stroop effect in anxiety disorders: general emotional or disorder specificity? J. Anxiety Disord. 15, 147–159. doi: 10.1038/npjschz.2016.18
Beeler, J. A., Daw, N., Frazier, C. R., and Zhuang, X. (2010). Tonic dopamine modulates exploitation of reward learning. Front. Behav. Neurosci. 4:170. doi: 10.3389/fnbeh.2010.00170
Berton, O., McClung, C. A., Dileone, R. J., Krishnan, V., Renthal, W., Russo, S. J., et al. (2006). Essential role of BDNF in the mesolimbic dopamine pathway in social defeat stress. Science 311, 864–868. doi: 10.1126/science.1120972
Blair, K., Shaywitz, J., Smith, B. W., Rhodes, R., Geraci, M., Jones, M., et al. (2008). Response to emotional expressions in generalized social phobia and generalized anxiety disorder: evidence for separate disorders. Am. J. Psychiatry 165, 1193–1202. doi: 10.1176/appi.ajp.2008.07071060
Blaya, C., Salum, G. A., Lima, M. S., Leistner-Segal, S., and Manfro, G. G. (2007). Lack of association between the Serotonin Transporter Promoter Polymorphism (5-HTTLPR) and Panic Disorder: a systematic review and meta-analysis. Behav. Brain Funct. 3:41. doi: 10.1186/1744-9081-3-41
Bódi, N., Kéri, S., Nagy, H., Moustafa, A., Myers, C. E., Daw, N., et al. (2009). Reward-learning and the novelty-seeking personality: a between- and within-subjects study of the effects of dopamine agonists on young Parkinson's patients. Brain 132, 2385–2395. doi: 10.1093/brain/awp094
Boldrini, M., Del Pace, L., Placidi, G. P., Keilp, J., Ellis, S. P., Signori, S., et al. (2005). Selective cognitive deficits in obsessive-compulsive disorder compared to panic disorder with agoraphobia. Acta Psychiatr. Scand. 111, 150–158. doi: 10.1111/j.1600-0447.2004.00247.x
Brischoux, F., Chakraborty, S., Brierley, D. I., and Ungless, M. A. (2009). Phasic excitation of dopamine neurons in ventral VTA by noxious stimuli. Proc. Natl. Acad. Sci. U.S.A. 106, 4894–4899. doi: 10.1073/pnas.0811507106
Cardinal, R. N., Parkinson, J. A., Hall, J., and Everitt, B. J. (2002). Emotion and motivation: the role of the amygdala, ventral striatum, and prefrontal cortex. Neurosci. Biobehav. Rev. 26, 321–352. doi: 10.1016/S0149-7634(02)00007-6
Clark, D. M., and Wells, A. (1995). “A cognitive model of social phobia,” in Social Phobia: Diagnosis, Assessment, and Treatment, Vol. 1, eds R. G. Heimberg, M. Liebowitz, D. Hope, and F. Schneier (New York, NY: Guilford Press), 24.
Cohen, L. J., Hollander, E., DeCaria, C. M., Stein, D. J., Simeon, D., Liebowitz, M. R., et al. (1996). Specificity of neuropsychological impairment in obsessive-compulsive disorder: a comparison with social phobic and normal control subjects. J. Neuropsychiatry Clin. Neurosci. 8, 82–85. doi: 10.1176/jnp.8.1.82
Collins, A. G., Cavanagh, J. F., and Frank, M. J. (2014). Human EEG uncovers latent generalizable rule structure during learning. J. Neurosci. 34, 4677–4685. doi: 10.1523/JNEUROSCI.3900-13.2014
Cremers, H. R., Veer, I. M., Spinhoven, P., Rombouts, S. A., and Roelofs, K. (2014). Neural sensitivity to social reward and punishment anticipation in social anxiety disorder. Front. Behav. Neurosci. 8:439. doi: 10.3389/fnbeh.2014.00439
Davis, M. (1992). The role of the amygdala in fear and anxiety. Annu. Rev. Neurosci. 15, 353–375. doi: 10.1146/annurev.ne.15.030192.002033
Daw, N. D., O'Doherty, J. P., Dayan, P., Seymour, B., and Dolan, R. J. (2006). Cortical substrates for exploratory decisions in humans. Nature 441, 876–879. doi: 10.1038/nature04766
Dayan, P., and Balleine, B. W. (2002). Reward, motivation, and reinforcement learning. Neuron 36, 285–298. doi: 10.1016/S0896-6273(02)00963-7
Deakin, J. F., and Graeff, F. G. (1991). 5-HT and mechanisms of defence. J. Psychopharmacol. (Oxford). 5, 305–315. doi: 10.1177/026988119100500423
Dupont, H., Mollard, E., and Cottraux, J. (2000). Visuo-spatial attention processes in panic disorder with agoraphobia: a pilot study using a visual target discrimination task. Eur. Psychiatry 15, 254–260. doi: 10.1016/S0924-9338(00)00236-4
Eshel, N., and Roiser, J. P. (2010). Reward and punishment processing in depression. Biol. Psychiatry 68, 118–124. doi: 10.1016/j.biopsych.2010.01.027
Frank, M. J., D'Lauro, C., and Curran, T. (2007a). Cross-task individual differences in error processing: neural, electrophysiological, and genetic components. Cogn. Affect. Behav. Neurosci. 7, 297–308. doi: 10.3758/CABN.7.4.297
Frank, M. J., Moustafa, A. A., Haughey, H. M., Curran, T., and Hutchison, K. E. (2007b). Genetic triple dissociation reveals multiple roles for dopamine in reinforcement learning. Proc. Natl. Acad. Sci. U.S.A. 104, 16311–16316. doi: 10.1073/pnas.0706111104
Furmark, T., Tillfors, M., Garpenstrand, H., Marteinsdottir, I., Langstrom, B., Oreland, L., et al. (2004). Serotonin transporter polymorphism related to amygdala excitability and symptom severity in patients with social phobia. Neurosci. Lett. 362, 189–192. doi: 10.1016/j.neulet.2004.02.070
Gladsjo, J. A., Rapaport, M. H., McKinney, R., Lucas, J. A., Rabin, A., Oliver, T., et al. (1998). A neuropsychological study of panic disorder: negative findings. J. Affect. Disord. 49, 123–131.
Golier, J., and Yehuda, R. (2002). Neuropsychological processes in post-traumatic stress disorder. Psychiatr. Clin. North Am. 25, 295–315, vi. doi: 10.1016/S0193-953X(01)00004-1
Gorman, J. M., Kent, J. M., Sullivan, G. M., and Coplan, J. D. (2000). Neuroanatomical hypothesis of panic disorder, revised. Am. J. Psychiatry 157, 493–505. doi: 10.1176/appi.ajp.157.4.493
Greisberg, S., and McKay, D. (2003). Neuropsychology of obsessive-compulsive disorder: a review and treatment implications. Clin. Psychol. Rev. 23, 95–117. doi: 10.1016/S0272-7358(02)00232-5
Grupe, D. W., and Nitschke, J. B. (2013). Uncertainty and anticipation in anxiety: an integrated neurobiological and psychological perspective. Nat. Rev. Neurosci. 14, 488–501. doi: 10.1038/nrn3524
Guitart-Masip, M., Duzel, E., Dolan, R., and Dayan, P. (2014). Action versus valence in decision making. Trends Cogn. Sci. (Regul. Ed). 18, 194–202. doi: 10.1016/j.tics.2014.01.003
Haber, S. N., and Knutson, B. (2010). The reward circuit: linking primate anatomy and human imaging. Neuropsychopharmacology 35, 4–26. doi: 10.1038/npp.2009.129
Hamilton, M. (1959). The assessment of anxiety states by rating. Br. J. Med. Psychol. 32, 50–55. doi: 10.1111/j.2044-8341.1959.tb00467.x
Heinrichs, N., and Hofmann, S. G. (2001). Information processing in social phobia: a critical review. Clin. Psychol. Rev. 21, 751–770. doi: 10.1016/S0272-7358(00)00067-2
Henkel, V., Bussfeld, P., Möller, H. J., and Hegerl, U. (2002). Cognitive-behavioural theories of helplessness/hopelessness: valid models of depression? Eur. Arch. Psychiatry Clin. Neurosci. 252, 240–249. doi: 10.1007/s00406-002-0389-y
Herzallah, M. M., Moustafa, A. A., Natsheh, J. Y., Abdellatif, S. M., Taha, M. B., Tayem, Y. I., et al. (2013). Learning from negative feedback in patients with major depressive disorder is attenuated by SSRI antidepressants. Front. Integr. Neurosci. 7:67. doi: 10.3389/fnint.2013.00067
Hirschfeld, R. M. (2001). The comorbidity of major depression and anxiety disorders: recognition and management in primary care. Prim. Care Companion J. Clin. Psychiatry 3, 244–254. doi: 10.4088/PCC.v03n0609
Horner, M. D., and Hamner, M. B. (2002). Neurocognitive functioning in posttraumatic stress disorder. Neuropsychol. Rev. 12, 15–30. doi: 10.1023/A:1015439106231
Kessler, R. C., Berglund, P., Demler, O., Jin, R., Merikangas, K. R., and Walters, E. E. (2005). Lifetime prevalence and age-of-onset distributions of DSM-IV disorders in the National Comorbidity Survey Replication. Arch. Gen. Psychiatry 62, 593–602. doi: 10.1001/archpsyc.62.6.593
Kuelz, A. K., Hohagen, F., and Voderholzer, U. (2004). Neuropsychological performance in obsessive-compulsive disorder: a critical review. Biol. Psychol. 65, 185–236. doi: 10.1016/j.biopsycho.2003.07.007
Lautenbacher, S., Spernal, J., and Krieg, J. C. (2002). Divided and selective attention in panic disorder. A comparative study of patients with panic disorder, major depression and healthy controls. Eur. Arch. Psychiatry. Clin. Neurosci. 252, 210–213. doi: 10.1007/s00406-002-0382-5
LeDoux, J. E., Cicchetti, P., Xagoraris, A., and Romanski, L. M. (1990). The lateral amygdaloid nucleus: sensory interface of the amygdala in fear conditioning. J. Neurosci. 10, 1062–1069.
LeDoux, J. E., Iwata, J., Cicchetti, P., and Reis, D. J. (1988). Different projections of the central amygdaloid nucleus mediate autonomic and behavioral correlates of conditioned fear. J. Neurosci. 8, 2517–2529.
Lovibond, P. F., Saunders, J. C., Weidemann, G., and Mitchell, C. J. (2008). Evidence for expectancy as a mediator of avoidance and anxiety in a laboratory model of human avoidance learning. Q. J. Exp. Psychol. (Hove). 61, 1199–1216. doi: 10.1080/17470210701503229
Lucas, J. A., Telch, M. J., and Bigler, E. D. (1991). Memory functioning in panic disorder: a neuropsychological perspective. J. Anxiety Disord. 5, 20. doi: 10.1016/0887-6185(91)90013-J
Ly, V., and Roelofs, K. (2009). Social anxiety and cognitive expectancy of aversive outcome in avoidance conditioning. Behav. Res. Ther. 47, 840–847. doi: 10.1016/j.brat.2009.06.015
Mathews, A., and MacLeod, C. (1994). Cognitive approaches to emotion and emotional disorders. Annu. Rev. Psychol. 45, 25–50. doi: 10.1146/annurev.ps.45.020194.000325
Mennin, D. S., Heimberg, R. G., Turk, C. L., and Fresco, D. M. (2005). Preliminary evidence for an emotion dysregulation model of generalized anxiety disorder. Behav. Res. Ther. 43, 1281–1310. doi: 10.1016/j.brat.2004.08.008
Moustafa, A. A., and Maida, A. S. (2007). Using TD learning to simulate working memory performance in a model of the prefrontal cortex and basal ganglia. Cogn. Syst. Res. 8, 262–281. doi: 10.1016/j.cogsys.2007.02.001
Moustafa, A. A., Gluck, M. A., Herzallah, M. M., and Myers, C. E. (2015a). The influence of trial order on learning from reward versus punishment in a probabilistic categorization task: experimental and computational analyses. Front. Behav. Neurosci. 9:153. doi: 10.3389/fnbeh.2015.00153
Moustafa, A. A., Herzallah, M. M., and Gluck, M. A. (2013). Dissociating the cognitive effects of levodopa versus dopamine agonists in a neurocomputational model of learning in Parkinson's disease. Neurodegener. Dis. 11, 102–111. doi: 10.1159/000341999
Moustafa, A. A., Sheynin, J., and Myers, C. E. (2015b). The role of informative and ambiguous feedback in avoidance behavior: empirical and computational findings. PLoS ONE 10:e0144083. doi: 10.1371/journal.pone.0144083
Muller, J., and Roberts, J. E. (2005). Memory and attention in Obsessive-Compulsive Disorder: a review. J. Anxiety Disord. 19, 1–28. doi: 10.1016/j.janxdis.2003.12.001
Myers, C. E., Sheynin, J., Balsdon, T., Luzardo, A., Beck, K. D., Hogarth, L., et al. (2016). Probabilistic reward- and punishment-based learning in opioid addiction: experimental and computational data. Behav. Brain Res. 296, 240–248. doi: 10.1016/j.bbr.2015.09.018
O'Doherty, J., Dayan, P., Schultz, J., Deichmann, R., Friston, K., and Dolan, R. J. (2004). Dissociable roles of ventral and dorsal striatum in instrumental conditioning. Science 304, 452–454. doi: 10.1126/science.1094285
Pariante, C. M., and Lightman, S. L. (2008). The HPA axis in major depression: classical theories and new developments. Trends Neurosci. 31, 464–468. doi: 10.1016/j.tins.2008.06.006
Penny, W. D., Stephan, K. E., Daunizeau, J., Rosa, M. J., Friston, K. J., Schofield, T. M., et al. (2010). Comparing families of dynamic causal models. PLoS Comput. Biol. 6:e1000709. doi: 10.1371/journal.pcbi.1000709
Phillips, R. G., and LeDoux, J. E. (1992). Differential contribution of amygdala and hippocampus to cued and contextual fear conditioning. Behav. Neurosci. 106, 274–285. doi: 10.1037/0735-7044.106.2.274
Piray, P., Zeighami, Y., Bahrami, F., Eissa, A. M., Hewedi, D. H., and Moustafa, A. A. (2014). Impulse control disorders in Parkinson's disease are associated with dysfunction in stimulus valuation but not action valuation. J. Neurosci. 34, 7814–7824. doi: 10.1523/JNEUROSCI.4063-13.2014
Porcelli, S., Fabbri, C., and Serretti, A. (2012). Meta-analysis of serotonin transporter gene promoter polymorphism (5-HTTLPR) association with antidepressant efficacy. Eur. Neuropsychopharmacol. 22, 239–258. doi: 10.1016/j.euroneuro.2011.10.003
Purcell, R., Maruff, P., Kyrios, M., and Pantelis, C. (1998a). Cognitive deficits in obsessive-compulsive disorder on tests of frontal-striatal function. Biol. Psychiatry 43, 348–357. doi: 10.1016/S0006-3223(97)00201-1
Purcell, R., Maruff, P., Kyrios, M., and Pantelis, C. (1998b). Neuropsychological deficits in obsessive-compulsive disorder: a comparison with unipolar depression, panic disorder, and normal controls. Arch. Gen. Psychiatry 55, 415–423. doi: 10.1001/archpsyc.55.5.415
Rabe-Jablonska, J., and Bienkiewicz, W. (1994). [Anxiety disorders in the fourth edition of the classification of mental disorders prepared by the American Psychiatric Association: diagnostic and statistical manual of mental disorders (DMS-IV – options book]. Psychiatr. Pol. 28, 255–268.
Richey, J. A., Rittenberg, A., Hughes, L., Damiano, C. R., Sabatino, A., Miller, S., et al. (2014). Common and distinct neural features of social and non-social reward processing in autism and social anxiety disorder. Soc. Cogn. Affect. Neurosci. 9, 367–377. doi: 10.1093/scan/nss146
Rutledge, R. B., Lazzaro, S. C., Lau, B., Myers, C. E., Gluck, M. A., and Glimcher, P. W. (2009). Dopaminergic drugs modulate learning rates and perseveration in Parkinson's patients in a dynamic foraging task. J. Neurosci. 29, 15104–15114. doi: 10.1523/JNEUROSCI.3524-09.2009
Salkovskis, P. M., Clark, D. M., and Hackmann, A. (1991). Treatment of panic attacks using cognitive therapy without exposure or breathing retraining. Behav. Res. Ther. 29, 161–166. doi: 10.3109/02770903.2015.1033724
Sartorius, N., Ustün, T. B., Lecrubier, Y., and Wittchen, H. U. (1996). Depression comorbid with anxiety: results from the WHO study on psychological disorders in primary health care. Br. J. Psychiatry Suppl. 30, 38–43.
Schroeder, R. W., Twumasi-Ankrah, P., Baade, L. E., and Marshall, P. S. (2012). Reliable digit span: a systematic review and cross-validation study. Assessment 19, 21–30. doi: 10.1177/1073191111428764
Schultz, W. (2006). Behavioral theories and the neurophysiology of reward. Annu. Rev. Psychol. 57, 87–115. doi: 10.1146/annurev.psych.56.091103.070229
Schwartz, G. (1978). Estimating the dimensions of a model. Ann. Stat. 2, 461–464. doi: 10.1214/aos/1176344136
Stephan, K. E., Penny, W., Daunizeau, J., Moran, R. J., and Friston, K. J. (2009). Bayesian model selection for group studies. Neuroimage 46, 1004–1017. doi: 10.1016/j.neuroimage.2009.03.025
Strug, L. J., Suresh, R., Fyer, A. J., Talati, A., Adams, P. B., Li, W., et al. (2010). Panic disorder is associated with the serotonin transporter gene (SLC6A4) but not the promoter region (5-HTTLPR). Mol. Psychiatry 15, 166–176. doi: 10.1038/mp.2008.79
Sutton, R. S., and Barto, A. G. (1998). Reinforcement Learning: An Introduction. Cambridge, MA: MIT Press.
Tidey, J. W., and Miczek, K. A. (1996). Social defeat stress selectively alters mesocorticolimbic dopamine release: an in vivo microdialysis study. Brain Res. 721, 140–149. doi: 10.1016/0006-8993(96)00159-X
Ungless, M. A., Argilli, E., and Bonci, A. (2010). Effects of stress and aversion on dopamine neurons: implications for addiction. Neurosci. Biobehav. Rev. 35, 151–156. doi: 10.1016/j.neubiorev.2010.04.006
Uttl, B. (2002). North American Adult Reading Test: age norms, reliability, and validity. J. Clin. Exp. Neuropsychol. 24, 1123–1137. doi: 10.1076/jcen.24.8.1123.8375
van der Wee, N. J., van Veen, J. F., Stevens, H., van Vliet, I. M., van Rijk, P. P., and Westenberg, H. G. (2008). Increased serotonin and dopamine transporter binding in psychotropic medication-naive patients with generalized social anxiety disorder shown by 123I-beta-(4-iodophenyl)-tropane SPECT. J. Nucl. Med. 49, 757–763. doi: 10.2967/jnumed.107.045518
Vriends, N., Michael, T., Schindler, B., and Margraf, J. (2012). Associative learning in flying phobia. J. Behav. Ther. Exp. Psychiatry 43, 838–843. doi: 10.1016/j.jbtep.2011.11.003
Watkins, C. J. C. H., and Dayan, P. (1992). Q-learning. Mach. Learn. 8, 279–292. doi: 10.1007/BF00992698
Wise, R. A. (2004). Dopamine, learning and motivation. Nat. Rev. Neurosci. 5, 483–494. doi: 10.1038/nrn1406
Keywords: generalized anxiety disorder, social anxiety disorder, panic anxiety disorder, striatum, dopamine, learning, positive feedback, negative feedback
Citation: Khdour HY, Abushalbaq OM, Mughrabi IT, Imam AF, Gluck MA, Herzallah MM and Moustafa AA (2016) Generalized Anxiety Disorder and Social Anxiety Disorder, but Not Panic Anxiety Disorder, Are Associated with Higher Sensitivity to Learning from Negative Feedback: Behavioral and Computational Investigation. Front. Integr. Neurosci. 10:20. doi: 10.3389/fnint.2016.00020
Received: 18 November 2015; Accepted: 26 May 2016;
Published: 29 June 2016.
Edited by:
Elizabeth B. Torres, Rutgers University, USAReviewed by:
Catalin V. Buhusi, Utah State University, USAAlexander J. Shackman, University of Maryland, USA
Copyright © 2016 Khdour, Abushalbaq, Mughrabi, Imam, Gluck, Herzallah and Moustafa. This is an open-access article distributed under the terms of the Creative Commons Attribution License (CC BY). The use, distribution or reproduction in other forums is permitted, provided the original author(s) or licensor are credited and that the original publication in this journal is cited, in accordance with accepted academic practice. No use, distribution or reproduction is permitted which does not comply with these terms.
*Correspondence: Mohammad M. Herzallah, bW9oYW1tYWQubS5oZXJ6YWxsYWhAZ21haWwuY29t