- 1 University of Pennsylvania Medical Center, Philadelphia, PA, USA
- 2 Rice University, Houston, TX, USA
We used voxel-based lesion-symptom mapping (VLSM) to determine which brain areas are necessary for discriminating time intervals above and below 1 s. VLSM compares behavioral scores of patients that have damage to a given voxel to those that do not on a voxel-by-voxel basis to determine which voxels are critical for the given behavior. Forty-seven subjects with unilateral hemispheric lesions performed a temporal discrimination task in which a standard stimulus was compared on each trial to a test stimulus. In different blocks of trials, standard stimuli were either 600 or 2000 ms. Behavioral measures included the point of subjective equality, a measure of accuracy, and the coefficient of variation, a measure of variability. Lesions of the right middle and inferior frontal gyri were associated with decrements in performance on both durations. In addition, lesions of the left temporal lobe and right precentral gyrus were associated exclusively with impaired performance for subsecond stimuli. In line with results from other studies, these data suggest that different circuits are necessary for timing intervals in these ranges, and that right frontal areas are particularly important to timing.
Introduction
There has been a surge of interest in the cognitive neuroscience of time perception over the past decade. Whereas potential contributions of the basal ganglia and cerebellum to the perception of short time intervals have been explored by a number of investigators, the cortical basis of interval processing has more recently come to the forefront. For example, models of timing such as striatal beat frequency (SBF; Matell and Meck, 2004) suggest that cortex may be crucial to interval timing. On this account, input from cortical neurons is integrated by spiny neurons in the striatum to encode a remembered duration. Additionally, electrophysiologic investigations of cortical neurons have demonstrated that cells in parietal (Leon and Shadlen, 2003) and frontal (Niki and Watanabe, 1979; Genovesio et al., 2006) cortex, including supplementary motor area (SMA; Macar et al., 2004; Mita et al., 2009) exhibit ramping and other behaviors that are consistent with the hypothesis that these cells mark the passage of time.
Many (Gibbon et al., 1997; Rammsayer, 1999, 2001; Lewis and Miall, 2003) investigators have argued for a fundamental distinction between timing of short (usually less than 1 s) and long (usually more than 1 s) intervals. Although different structures (Lewis and Miall, 2003; Wiener et al., 2010b) and neurotransmitters (Rammsayer, 1999, 2001; Wiener et al., 2011) have been proposed to support these two functions, the degree to which routines involved in sub- and suprasecond timing are distinct remains unclear (e.g., Macar et al., 2002). One reason for the persistent controversy is that few studies of timing have assessed both sub- and suprasecond intervals (e.g., Harrington et al., 1998; Hinton and Meck, 2004; Shih et al., 2009).
There have been exceptions to this generalization, however. For example Lewis and Miall (2003) reported an imaging study in which a temporal discrimination task at 600 and 3000 ms was employed. They found bilateral DLPFC, and right inferior parietal cortex (angular gyrus), among other areas, to be commonly activated during timing of both intervals. Subsecond intervals also activated the cerebellum, right superior temporal gyrus, and prefrontal operculum, among other areas, while suprasecond intervals additionally activated left inferior parietal cortex and the posterior cingulate. More recently, we (Wiener et al., 2010b) performed a meta-analysis using the activation likelihood estimation (ALE) technique in which both sub- and suprasecond timing were included. This analysis showed two brain regions common to timing sub- and suprasecond durations: SMA and right inferior frontal gyrus (IFG). Additionally, when studies were sorted according to stimulus duration, we found that timing of subsecond durations were more likely to activate the cerebellum and basal ganglia, while suprasecond durations were more likely to activate cortical structures.
Transcranial magnetic stimulation (TMS), a technique for producing temporary, virtual lesions in a circumscribed cortical region, has also produced data comparing cortical contributions to timing of durations above and below 1 s. Jones et al. (2004) showed that TMS over right DLPFC disrupts temporal reproduction in the seconds range, but not in the subsecond range. An additional study by Koch et al. (2007) replicated the exclusive effect of right DLPFC stimulation on suprasecond timing and extended the results by demonstrating that stimulation of the cerebellum disrupted performance for subsecond stimuli only.
There have been two studies involving subjects with focal brain lesions in which both sub- and suprasecond intervals have been tested (Nichelli et al., 1995; Mangels et al., 1998). Both studies suggested that lesions of the prefrontal cortex disrupt discrimination of both sub- and suprasecond intervals. Another study examined frontal lesion patients’ performance on a tapping task with a response period of 1.5 s (Picton et al., 2006). They found that participants with damage to right lateral BA 45 had the most difficulty with this task. Patients with damage to right lateral BA 6 also showed a trend toward disruption in performance.
Another cortical lesion study of timing behavior bears noting. Harrington et al. (1998) administered a temporal discrimination task using subsecond stimuli (300 and 600 ms standards) in 37 subjects with cortical lesions. Grouping subjects on the basis of involvement in specified brain regions, they found both frontal and parietal areas in the right hemisphere to be important to timing these intervals. Finally, Coslett et al. (2009) reported data from 31 subjects with focal cortical lesions on temporal production, estimation, and reproduction tasks using stimuli from 2 to 12 s; they found that these patients underproduced and overestimated all but the shortest interval.
We report an additional study of the hemispheric basis of temporal processing. Our study differs from previous investigations in several important respects. First, subjects were not selected on the basis of lesion locus. Using a convenience sample rather than subjects selected on the basis of lesion locus permits us to investigate a wider range of brain structures; whereas selecting subjects on the basis of frontal lobe damage, for example, will permit one to draw conclusions about the role of frontal regions in timing, such an approach offers no information regarding the role of other brain regions. Second, with 47 subjects with focal lesions, our study is the largest to date to explore the hemispheric basis of temporal processing. Third, rather than just group subjects on the basis of a common region of interest (e.g., “predominantly frontal”), we also employ voxel-based lesion-symptom mapping, or VLSM (Bates et al., 2003), a technique derived from work in functional imaging. In this technique, at each voxel the performance of subjects with a lesion at that voxel is compared to the performance of subjects without a lesion at that voxel using a t-test. Voxels at which lesioned subjects perform significantly less well than subjects without lesions are assumed to be important for the behavioral measure. VLSM has a number of advantages over traditional methods of studying behavioral deficits in brain-lesioned patients. It allows us to examine all areas of the brain without a priori assumptions, thereby offering the possibility of finding areas not previously known to be involved in the behavior studied. It also gives a more precise picture of which areas are important to the given behavior than traditional region of interest or overlap studies of brain-lesioned patients.
Materials and Methods
Subjects
Forty-seven subjects (22 female) with a single hemispheric vascular lesion documented by brain imaging participated in this study. Patients were recruited through the University of Pennsylvania Center for Cognitive Neuroscience patient database (Fellows et al., 2008). Sixteen healthy control subjects also participated. The mean age of the patients was 59 ± 11 years, and the mean age of controls was 57 ± 9 years. Patients had suffered their stroke an average of 6.3 ± 5.3 years previously. No subject had a history of substance abuse or psychiatric illness. See Table 1 for details on each patient. The research was approved the Institutional Review Board of the University of Pennsylvania.
Behavioral Task
Subjects were asked to indicate if a test interval was longer or shorter than the standard interval. At the onset of each trial, a central fixation point was presented for 1 s and immediately succeeded by a red square that persisted for either 600 or, in a different block of trials, 2000 ms (standard duration). After a blank screen lasting 1 s, a second red square was presented for a variable duration (comparison duration) as determined by a maximum-likelihood procedure, the Parameter Estimation by Sequential Testing (PEST) algorithm (Pentland, 1980). The current upper or lower threshold value is presented as the comparison duration for each trial. Furthermore, the range and frequency of comparison stimuli is different for each subject, allowing task difficulty to be scaled to individual performance. We set the initial lower and upper thresholds to equal 50 and 150% of the standard interval duration, respectively. The initial stepsize for adjustments in the comparison duration was set to 15% of the standard duration for the first 20 trials, then to 5% for the remaining 40 trials. If the comparison stimulus was judged to be longer, subjects depressed the “L” key whereas if the comparison stimulus was judged to be shorter, subjects depressed the “S” key. Subjects were not told the range of stimulus durations and were not given feedback regarding accuracy. Each interval (600, 2000 ms) was assessed in a separate block of 60 trials. The order of blocks was counterbalanced across subjects.
Data Analysis
Curve fitting
The probability of the subject making a “longer” response choice was plotted as a function of the comparison interval. These data were then fit with a sigmoidal, psychometric curve using the psignifit version 2.5.6 software package (see bootstrap-software.org/psignifit/) for Matlab, which implements the maximum-likelihood method described by Wichmann and Hill (2001a). Upper and lower thresholds, the approximate points at which the subject is 25 and 75% likely to judge the comparison stimulus as longer, were calculated using the bias-corrected bootstrap method implemented by psignifit based on 4999 simulations (Wichmann and Hill, 2001b). For each block of trials for each subject two measures of performance were calculated. First, the point of subjective equality (PSE), or the duration at which participants responded “longer” 50% of the time was determined; this is considered a measure of accuracy. Second, the difference limen (DL), or the interval between the durations at which participants responded “longer” 25 and 75% of the time, was calculated; for example, if subjects indicated that the test interval of 450 ms was longer on 25% of trials and a test interval of 800 ms was longer on 75% of trials, the DL would be 350 ms.
In order to facilitate comparison of performance across different intervals, PSE was transformed into an absolute proportional error (|[PSE–standard]/standard|) value; similarly, the DL was divided by the PSE to generate the coefficient of variation (CV); CV, therefore, is a measure of variability that is independent of stimulus duration.
In order to minimize erroneous results driven by extremely poor participants, the data were winsorized, so that values that were greater than 3 SD from the mean were recoded to be the value at 3 SD from the mean. Although they understood the task and performed adequately in other domains, six subjects performed extremely poorly with the result that a psychometric curve could not be generated; for these individuals, absolute PSE proportional error and CV were coded to be 3 SD from the mean of the patient data on these measures. These patients were not excluded from analysis based on their poor performance because to do so would eliminate those patients whose performance was most aberrant and therefore potentially most informative regarding temporal processing. Absolute proportional error was used as we were unable to determine if the poor performance of the six subjects reflected under- or over-estimation of the intervals. Further, we had no a priori assumptions regarding whether lesions to certain cortical areas would result in an increase or decrease perceived duration, and, it is possible that two patients with a similar lesion could display inaccuracies in time perception in opposite directions. For this reason, using an absolute error measure makes the VLSM process more sensitive to perturbations in time perception that may occur in either direction (speeding up or slowing down), as any disturbance in timing accuracy will contribute to a significant result. It is understood that this gain in sensitivity comes at the cost of making claims regarding lengthening or shortening effects of brain lesions.
Imaging methods
Structural images were acquired using MRI (n = 14) or CT (n = 33). All scans were obtained at least 2 months after the infarct and were judged to be of good quality by an experienced neurologist.
VLSM methods
For patients with high-resolution MRI scans (n = 14), lesions were identified in native space (that is, on the subject’s MRI scan) manually by a neurologist (HBC) who was naïve with respect to the behavioral data. The marked structural scans were then registered to a common template using a symmetric diffeomorphic registration algorithm (Avants et al., 2006; see also http://www.picsl.upenn.edu/ANTS/). This same mapping was then applied to the lesion maps. To optimize the automated registration, volumes were first registered to an intermediate template constructed from images acquired on the same scanner. A single mapping from this intermediate template to the Montreal Neurological Institute (MNI) space “Colin27” volume (Holmes et al., 1998) was used to complete the mapping from subject space to MNI space. For patients with CT scans the lesions were rendered on the Colin27 template using MRICro by the same neurologist. Resolution for the Colin27 template was 1 voxel = 1 mm3.
Behavioral Analysis
Separate mixed-model ANOVAs were performed for absolute PSE proportional error and CV. Duration (600 or 2000 ms) was a within-subjects factor and group (patient or control) was a between-subjects factor. In subsequent analyses exploring the hemispheric basis of temporal processing deficits, separate ANOVAs were performed for patients with left and right hemisphere lesions, comparing each to controls.
Although VLSM was the primary technique by which issues of the anatomic bases of performance was analyzed, in an effort to address previously reported findings (e.g., Harrington et al., 1998), we also divided subjects on the basis of the lesion locus as defined by involvement of specific brain regions. In the current study patients were subdivided into those with and without lesions that were predominantly frontal (at least 10% damage to BAs 5, 6, 8, 9, 10, 11, 44, 45, 46, and 47; 13 patients). Also, patients with and without predominantly posterior parietal lesions (at least 10% damage to BAs 39, 40, 5, and 7; seven patients) were compared to controls. In keeping with previous studies, those patients whose lesions involved both frontal and parietal structures were assigned to the frontal or parietal groups on the basis of the relative size of the frontal or parietal involvement. Four patients with very extensive involvement in both regions were omitted from these analyses. For both analyses, percent damage was determined using the Brodmann area map in MRICron. Post hoc t-tests were administered where appropriate. Correlations were also performed between size of lesion (in cc) and performance on the timing tasks, as measured by CV or absolute proportional error of PSE at each interval.
VLSM Analysis
Voxels in which fewer than two patients were lesioned were excluded from the VLSM analyses. At each voxel, a t-test comparing the scores between patients with and without lesions was performed with the MRICron (http://www.sph.sc.edu/comd/rorden/mricron/) brain imaging package. The resulting t-map was thresholded to control the false discovery rate (FDR; Genovese et al., 2002) at q = 0.05, where q is the expected proportion of false positives among supra-threshold voxels.
Results
Behavioral Analysis
Patients versus controls
Data for the CV and absolute proportional error for both controls and patients is presented in Table 2. ANOVA for the CV data demonstrated a main effect of group [F(1,61) = 5.3, p < 0.05], but no significant effect for duration [F(1,61) = 0.1, ns] and no interaction between duration and group [F(1,61) = 1.0, ns]. ANOVA for absolute proportional error demonstrated a significant effect of group [F(1,61) = 11.2, p < 0.05] and there was no main effect of duration [F(1,61) = 0.2, ns]. There was no group by duration interaction [F(1,61) = 2.4, ns].
VLSM Analysis
600 ms interval
In the analysis for the CV measure significant voxels were found in the right precentral gyrus (lateral BA 6, 3231 voxels) and IFG (BA 44, 925 voxels). On the left side, significant areas were the basal ganglia (caudate, putamen, and pallidum, 8802 voxels), the superior and middle temporal lobe (35523 voxels), and hippocampus (2303 voxels, see Figure 1). No significant voxels were found in the absolute PSE proportional error analysis.

Figure 1. Results for the CV measure, 600 ms. Separate analyses were performed for left and right hemisphere-lesioned patients. All FDR cutoff values are q = 0.05. All images are in neurological convention.
2000 ms interval
The CV measure analysis yielded significant voxels in the right hemisphere in the same areas as the 600 ms interval: lateral BA 6 (3444 voxels) and BA 44 (1571 voxels), and also in the right middle frontal gyrus (MFG; BA 9, 73 voxels). No significant voxels were found on the left side for this measure, or for the PSE measure (see Figure 2). No significant voxels were found in the absolute PSE proportional error analysis.
Power
In VLSM analyses, power to detect behavioral differences is due in part to differences in the number of patients with lesions at each voxel. Figure 3 shows a color map of the number of patients with lesions in each voxel and thus provides a measure of the relative (not absolute) power to observe an effect at each voxel, as power is maximized where voxels are lesioned in about half the population for 0/1 lesion scores (Kimberg et al., 2007) As is typical in studies involving VLSM (e.g., Schwartz et al., 2009), the relative power is greatest in the peri-sylvian regions irrigated by the Middle Cerebral Artery.
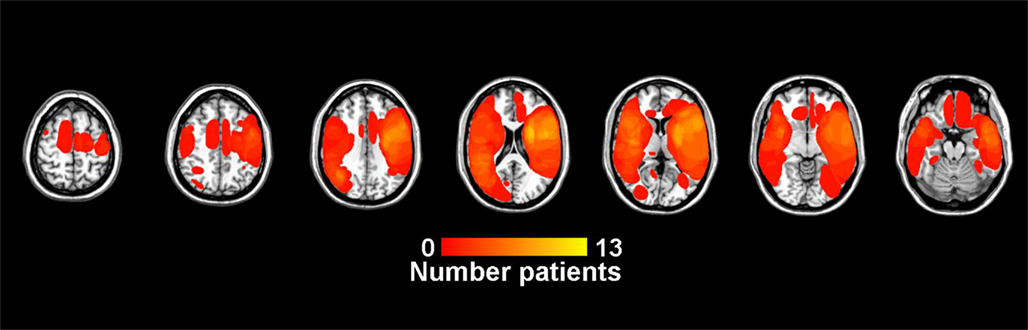
Figure 3. Lesion overlap showing the number of patients with lesions at each voxel. In VLSM analyses, power to detect behavioral differences is due in part to differences in the number of patients with lesions at each voxel. The scale runs from dark red to bright yellow, with brighter yellow indicating a greater number of patients with a lesion at that voxel. Lower right is a legend with number of lesions at each voxel.
Further Breakdown of Behavioral Analysis
Hemispheric differences
Table 3 compares control data to patients with left hemisphere and right hemisphere lesions. ANOVA on CV between patients with right hemisphere lesions and controls showed a significant effect of group [F(1,39) = 5.1, p < 0.05], but no effect of duration or the interaction between duration and group. Left-hemisphere patients differed from controls overall [F(1,36) = 5.5, p < 0.05], but no significant effects of duration or the interaction of duration and group were found.
When comparing patients with right-sided lesions to controls, ANOVA on absolute proportional error demonstrated a main effect of group [F(1,39) = 13.7, ns] but there was no significant main effect of duration [F(1,39) = 0.3, ns] or duration by group interaction [F(1,39) = 2.3, ns]. Left-sided lesion patients were significantly different from controls also [F(1,36) = 9.6, p < 0.05], but no effect of duration or the interaction between duration and group was found.
No significant differences were found between left- and right-sided lesion patients for either the CV measure, or the absolute error of PSE measure.
Frontal damage
Table 4 compares control data to patients with lesions that are predominantly frontal and those with lesions that are primarily posterior parietal. Overlap images of patient lesions contributing to the predominantly frontal group are presented in Figure 4. The overall ANOVA for the CV measure showed a significant difference between controls and patients with predominantly frontal damage [F(1,29) = 7.0, p < 0.05]. The duration factor, and the interaction between group and duration were not significant in this analysis.
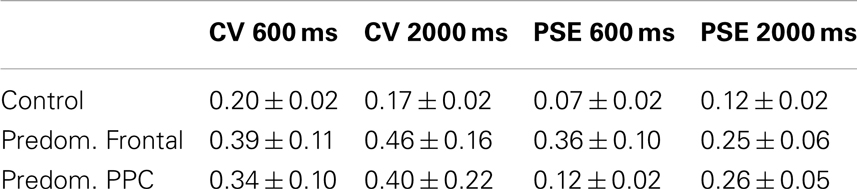
Table 4. Performance of controls versus patients with predominantly frontal lesions and those with predominantly posterior parietal lesions.
For the absolute proportional error of PSE measure, the main effect of group was significant between controls and patients who had frontal damage [F(1,29) = 10.4, p < 0.05]. No effect of duration or the interaction between duration and group was found.
Posterior parietal damage
Figure 5 depicts an overlap image of lesions in patients comprising the posterior parietal damage group. The overall ANOVA for the CV measure showed no significant difference between controls and patients who had damage predominantly to posterior parietal regions [F(1,21) = 4.0, ns], The interaction between group and duration was not significant in this analysis.
For absolute proportional error, a significant main effect of group emerged between patients with significant posterior parietal damage and controls [F(1,21) = 15.1, p < 0.05].
Predominantly frontal versus predominantly posterior parietal damage
There were no significant differences found between patients who had damage that was primarily frontal and those that had damage that was primarily posterior parietal in nature for either the CV or absolute error of PSE measure.
Correlations
Size of lesion correlated with CV at both the 600 ms (Pearson’s r = 0.34, p < 0.05) and the 2000 ms (Pearson’s r = 0.32, p < 0.05) intervals. Size of lesion did not correlate with absolute proportional error of PSE at either interval. Partial correlations with inferior frontal volume as a controlling factor removed the significant correlations of volume with CV at 600 ms (r = 0.26, ns) and 2000 ms (r = 0.15, ns). In contrast, partial correlations between CV and lesion volume with posterior parietal volume as a controlling factor did not remove the significant correlations (600 ms: r = 0.38, p < 0.05; 2000 ms: r = 0.33, p < 0.05). This suggests that, while IFG volume is a significant factor influencing the CV measures, PPC is not.
Discussion
Comparisons of all patients with brain lesions and controls demonstrated significant differences in performance for sub- and suprasecond intervals. Subsequent analyses in which subjects were partitioned on the basis of lesion location further demonstrated significant behavioral differences. Patients with significant frontal damage were less accurate and more variable than controls. Patients with damage to posterior parietal regions were less accurate than controls. These data are in line with previous suggestions that there may be specific cortical regions that are influential in the computation of time. To further examine this possibility, we turned to VLSM as a method to localize lesioned areas important to timing making no a priori assumptions regarding location of interest.
Voxel-based lesion-symptom mapping analysis revealed several areas important to perceptual timing for both 600 and 2000 ms intervals. These were the right precentral gyrus (lateral BA 6), the right MFG (BA 9), and the right IFG (BA 44).
These data are consistent with a number of other demonstrations from functional imaging and brain lesion subjects implicating regions of the right frontal lobe in timing. The IFG, for example, has been found to be active in numerous functional imaging studies of timing. Coull et al. (2004) reported activation of the IFG when subjects directed attention to time. The IFG has also been found to be active during a rhythm monitoring task (Schubotz et al., 2000), a task using tapping procedures in temporal reproduction (Bueti et al., 2008), and many others (e.g., Smith et al., 2003; Lewis and Miall, 2006).
A variety of different roles have been assigned to the IFG in timing. One hypothesis emphasized by Lewis and Miall (2006) is that the IFG and other regions of the DLPFC underlie working memory for time. Several lines of evidence are consistent with this. First, neurons in this region exhibit patterns of discharge that suggest that they monitor interval duration. Pouthas et al. (2005), for example, demonstrated in an fMRI investigation that activation in this area correlates with the length of the duration to be timed. Additionally, it is noteworthy that regions of the IFG, including those implicated in our study, are commonly activated in working memory tasks (Rissman et al., 2008; Lemus et al., 2009). For example, BA 6 has been shown to be related to working memory load (Cohen et al., 1997) and right premotor cortex is involved in spatial working memory storage (Smith and Jonides, 1999).
Other accounts of the role of the frontal lobe have also been proposed. Penhune et al. (1998) suggested that the IFG is responsible for short-term memory retrieval of standard time intervals. Rao et al. (1997) suggest that right IFG is involved, along with STG, in retrieval and rehearsal of representations of time intervals. Still other evidence suggests the IFG is active during recovery from attention lapses (Weissman et al., 2006). Further, right IFG was implicated in temporal decision-making processes by an fMRI study that measured decision-making activity as that activity that correlated with task difficulty (Wencil et al., 2010).
Our data implicate right but not left frontal regions in temporal processing. This finding is consistent with some (e.g., Rao et al., 1997; Brunia et al., 2000) but not all functional imaging studies (Schubotz et al., 2000; Shih et al., 2009). We note, however, that our recent voxel-wise meta-analysis of the functional imaging literature also demonstrated a substantial asymmetry in frontal activation; we found that the right but not left IFG was implicated in both sub- and suprasecond timing (Wiener et al., 2010b). Taken together, the data reported here from brain lesion subjects as well as the functional imaging meta-analysis strongly suggest that the right frontal lobe is a core element of the neural circuitry involved in temporal processing.
Additional subsecond findings
We also found that the basal ganglia, hippocampus, and left temporal lobe are important to discriminating time intervals of around 600 ms. The basal ganglia have long been implicated in timing behavior (for review, see Meck et al., 2008). Although we find a significant effect of basal ganglia damage for the subsecond duration only, the thrust of the literature depicts these structures as necessary for timing longer time intervals as well (e.g., Malapani et al., 1998; Matell et al., 2003). We note, however, that the association of lesions of the left basal ganglia with deficits in timing at 600 ms is inconsistent with a recent report from our lab that two subjects with extensive bilateral striatal lesions performed well on a variety of tasks, including the temporal discrimination task with 600 ms stimuli employed here, assessing timing of both sub- and suprasecond intervals (Coslett et al., 2010). One possible account for this discrepancy is that subjects with damaged voxels in the basal ganglia also had damage in other neural regions; as such, it is possible that damage to the basal ganglia only provided a timing impairment in the context of disruptions in other regions. Currently, VLSM does not allow one to distinguish between these alternatives. However, in light of our earlier findings, we suggest that the basal ganglia, when lesioned in isolation, are not necessary for many timing procedures in which they are nevertheless activated.
The left temporal lobe has been implicated in temporal discrimination by numerous imaging studies (Rao et al., 1997). Left temporal activation has been demonstrated not only in temporal discrimination with auditory stimuli (Rao et al., 2001) but also with visual stimuli as well (Coull et al., 2004, 2008). These findings support the auditory dominance hypothesis, which posits that auditory cortex, by virtue of the temporal acuity developed for language processing, is better tuned for temporal processing and thus is called upon to process time, regardless of the modality presented. Further evidence for this hypothesis comes from a study demonstrating that TMS to auditory cortex during somatosensory timing interferes with precision in a temporal discrimination task (Bolognini et al., 2009). Additionally, a recent study by Kanai et al. (2011) demonstrated that TMS to the auditory cortex disrupted performance on visual and auditory temporal discrimination tasks to the same degree, whereas visual cortex stimulation only disrupted performance for visually timed intervals.
Hippocampal involvement in memory for time has been shown in lesion work in rats (Olton et al., 1987) and humans (Noulhiane et al., 2007), although in humans medial temporal lesions only affected estimations of time intervals of greater than 3 min. The current results suggest a broader contribution of the hippocampus to timing.
Our VLSM analyses yielded no significant effects of parietal lesions on temporal discrimination, failing to replicate the results of numerous imaging (Coull et al., 2004; Shih et al., 2009), as well as TMS (Wiener et al., 2010a) studies. Perhaps of even greater relevance, our findings are inconsistent with several previous studies demonstrating abnormalities in temporal processing in subjects with parietal lesions (Harrington et al., 1998; Oliveri et al., 2009). One possible explanation for this discrepancy appeals to differences between VLSM and alternative methods for grouping subjects according to lesion location that are used to identify the site of pathology associated with a behavioral deficit. As previously described, the level of analysis in VLSM is the single voxel; traditional methods have employed analyses at a much coarser anatomic level (e.g., lobe or hemisphere). One consequence of the fact that the analysis is performed over single voxels is that VLSM is well suited to interrogate cognitive operations that are tightly linked to a specific brain structure. Techniques in which subjects are grouped on the basis of “frontal” or “parietal” involvement, in contrast, may be useful in the identification of brain–behavior relationships that have a more variable brain basis or which depend on neural systems distributed across larger brain regions. For example, if timing is disrupted by lesions anywhere in the posterior parietal cortex, subjects with lesions involving the supramarginal or angular gyri would be expected to exhibit deficits in temporal processing. Such lesions may not involve overlapping voxels, however, and therefore would not be identified with VLSM.
If multiple regions or large areas of abnormality in the posterior parietal lobe are necessary to generate abnormalities in timing, then, one might expect subjects grouped on the basis of damage in the posterior parietal cortex to be impaired on timing tasks in the absence of a discrete locus of abnormality on a VLSM analysis. Consistent with this speculation, we, like Harrington et al. (1998) observe deficits in subjects with lesions involving the posterior parietal cortex. Thus, although our VLSM analysis does not identify specific voxels in the parietal lobe that are associated with impaired performance on our timing task, these data do not permit one to infer that the parietal lobe is not relevant to timing.
Finally, we note that the failure to identify voxels in the parietal lobe that are associated with poor performance on the timing tasks is unlikely to be attributable solely to a lack of power. As demonstrated in the overlap map (Figure 4), there were as many subjects with lesions in this region as in the frontal regions in which significant effects were observed.
Several weaknesses of the study should be acknowledged. First, although we report the largest series of brain lesion subjects tested on a timing task to date, we are unable to offer any information about a number of brain regions that may be relevant to temporal processing. As we only included patients with hemispheric lesions, we are unable to assess the potential contributions of the cerebellum to timing. Furthermore, because most vascular lesions involve the peri-sylvian regions irrigated by the Middle Cerebral Artery, we lack power to detect effects of lesions involving the SMA, a structure that has been implicated in timing in a number of studies (Macar et al., 1999, 2004; Ferrandez et al., 2003; Wiener et al., 2010b).
Second, the temporal discrimination task utilized in the current study requires participants to compare two time intervals presented in close succession. Because the same “clock” is used to measure both intervals, this task is unlikely to be sensitive to differences in clock speed (cf, Wiener and Coslett, 2008).
In summary, VLSM analysis showed regions in the right frontal lobe to be common to timing intervals above and below 1 s. Lesions involving the left superior temporal cortex, hippocampus, and basal ganglia were found to be correlated with the ability to time the 600 ms interval only. The demonstration that subjects with posterior parietal lesions do not exhibit an abnormality on the VLSM analysis but perform abnormally relative to controls is consistent with the hypothesis that the posterior parietal lobe is important for interval timing but that the procedure(s) supported by the parietal lobe are not tightly localized.
Conflict of Interest Statement
The authors declare that the research was conducted in the absence of any commercial or financial relationships that could be construed as a potential conflict of interest.
References
Avants, B., Schoenemann, P. T., and Gee, J. C. (2006). Lagrangian frame diffeomorphic image registration: morphometric comparison of human and chimpanzee cortex. Med. Image Anal. 10, 397–412.
Bates, E., Wilson, S. M., Saygin, A. P., Dick, F., Sereno, M. I., Knight, R. T., and Dronkers, N. F. (2003). Voxel-based lesion-symptom mapping. Nat. Neurosci. 6, 448–450.
Bolognini, N., Papagno, C., Moroni, D., and Maravita, A. (2009). Tactile temporal processing in the auditory cortex. J. Cog. Neurosci. 22, 1201–1211.
Brunia, C. H., de Jong, B. M., van den Berg-Lenssen, M. M., and Paans, A. M. (2000). Visual feedback about time estimation is related to a right hemisphere activation measured by pet. Exp. Brain Res. 130, 328–337.
Bueti, D., Walsh, V., Frith, C., and Rees, G. (2008). Different brain circuits underlie motor and perceptual representations of temporal intervals. J. Cog. Neurosci. 20, 204–214.
Cohen, J. D., Perlstein, W. M., Braver, T. S., Nystrom, L. E., Noll, D. C., Jonides, J., and Smith, E. E. (1997). Temporal dynamics of brain activation during a working memory task. Nature 386, 604–608.
Coslett, H. B., Shenton, J., Dyer, T., and Wiener, M. (2009). Cognitive timing: neuropsychology and anatomic basis. Brain Res. 1254, 38–48.
Coslett, H. B., Wiener, M., and Chatterjee, A. (2010). Dissociable neural systems for timing: evidence from subjects with basal ganglia lesions. PLoS ONE 5, e10324. doi:10.1371/journal.pone.0010324
Coull, J. T., Nazarian, B., and Vidal, F. (2008). Timing, storage, and comparison of stimulus duration engage discrete anatomical components of a perceptual timing network. J. Cogn. Neurosci. 20, 1–12.
Coull, J. T., Vidal, F., Nazarian, B., and Macar, F. (2004). Functional anatomy of the attentional modulation of time estimation. Science 303, 1506–1508.
Fellows, L. K., Stark, M., Berg, A., and Chatterjee, A. (2008). Patient registries in cognitive neuroscience research: advantages, challenges, and practical advice. J. Cogn. Neurosci. 20, 1107–1113.
Ferrandez, A. M., Hugueville, L., Lehericy, S., Poline, J. B., Marsault, C., and Pouthas, V. (2003). Basal ganglia and supplementary motor area subtend duration perception: an FMRI study. Neuroimage 19, 1532–1544.
Genovese, C. R., Lazar, N. A., and Nichols, T. (2002). Thresholding of statistical maps in functional neuroimaging using the false discovery rate. Neuroimage 15, 870–878.
Genovesio, A., Tsujimoto, S., and Wise, S. P. (2006). Neuronal activity related to elapsed time in prefrontal cortex. J. Neurophysiol. 95, 3281–3285.
Gibbon, J., Malapani, C., Dale, C. L., and Gallistel, C. (1997). Toward a neurobiology of temporal cognition: advances and challenges. Curr. Opin. Neurobiol. 7, 170–184.
Harrington, D. L., Haaland, K. Y., and Knight, R. T. (1998). Cortical networks underlying mechanisms of time perception. J. Neurosci. 18, 1085–1095.
Hinton, S. C., and Meck, W. H. (2004). Frontal-striatal circuitry activated by human peak-interval timing in the supra-seconds range. Brain Res. Cogn. Brain Res. 21, 171–182.
Holmes, C. J., Hoge, R., Collins, L., Woods, R., Toga, A. W., and Evans, A. C. (1998). Enhancement of MR images using registration for signal averaging. J. Comput. Assist. Tomogr. 22, 324–333.
Jones, C. R. G., Rosenkranz, K., Rothwell, J. C., and Jahanshahi, M. (2004). The right dorsolateral prefrontal cortex is essential in time reproduction: an investigation with repetitive transcranial magnetic stimulation. Exp. Brain Res. 158, 366–372.
Kanai, R., Lloyd, H., Bueti, D., and Walsh, V. (2011). Modality-independent role of the primary auditory cortex in time estimation. Exp. Brain Res. 209, 465–471.
Kimberg, D. Y., Coslett, H. B., and Schwartz, M. F. (2007). Power in voxel-based lesion-symptom mapping. J. Cogn. Neurosci. 19, 1067–1080.
Koch, G., Oliveri, M., Torriero, S., Salerno, S., Lo Gerfo, E., and Caltagirone, C. (2007). Repetitive TMS of cerebellum interferes with millisecond time processing. Exp. Brain Res. 179, 291–299.
Lemus, L., Hernandez, A., and Romo, R. (2009). Neural encoding of auditory discrimination in ventral premotor cortex. Proc. Natl. Acad. Sci. U.S.A. 106, 14640–14645.
Leon, M. I., and Shadlen, M. N. (2003). Representation of time by neurons in the posterior parietal cortex of the macaque. Neuron 38, 317–327.
Lewis, P. A., and Miall, R. C. (2003). Distinct systems for automatic and cognitively controlled time measurement: evidence from neuroimaging. Curr. Opin. Neurobiol. 13, 250–255.
Lewis, P. A., and Miall, R. C. (2006). A right hemispheric prefrontal system for cognitive time measurement. Behav. Processes 71, 226–234.
Macar, F., Anton, J.-L., Bonnet, M., and Vidal, F. (2004). Timing functions of the supplementary motor area: an event-related fmri study. Brain Res. Cogn. Brain Res. 21, 206–215.
Macar, F., Lejeune, H., Bonnet, M., Ferrara, A., Pouthas, V., Vidal, F., and Maquet, P. (2002). Activation of the supplementary motor area and of attentional networks during temporal processing. Exp. Brain Res. 142, 475–485.
Macar, F., Vidal, F., and Casini, L. (1999). The supplementary motor area in motor and sensory timing: evidence from slow brain potential changes. Exp. Brain Res. 125, 271–280.
Malapani, C., Rakitin, B., Levy, R., Meck, W. H., Deweer, B., Dubois, B., and Gibbon, J. (1998). Coupled temporal memories in parkinson’s disease: a dopamine-related dysfunction. J. Cogn. Neurosci. 10, 316–331.
Mangels, J. A., Ivry, R. B., and Shimizu, N. (1998). Dissociable contributions of the prefrontal and neocerebellar cortex to time perception. Brain Res. Cogn. Brain Res. 7, 15–39.
Matell, M. S., and Meck, W. H. (2004). Cortico-striatal circuits and interval timing: coincidence detection of oscillatory processes. Brain Res. Cogn. Brain Res. 21, 139–170.
Matell, M. S., Meck, W. H., and Nicolelis, M. A. (2003). Interval timing and the encoding of signal duration by ensembles of cortical and striatal neurons. Behav. Neurosci. 117, 760–773.
Meck, W. H., Penney, T. B., and Pouthas, V. (2008). Cortico-striatal representation of time in animals and humans. Curr. Opin. Neurobiol. 18, 145–152.
Mita, A., Mushiake, H., Shima, K., Mitsuzaka, Y., and Tanji, J. (2009). Interval time coding by neurons in the presupplementary and supplementary motor areas. Nat. Neurosci. 12, 502–507.
Nichelli, P., Clark, K., Hollnagel, C., and Grafman, J. (1995). Duration processing after frontal lobe lesions. Ann. N. Y. Acad. Sci. 769, 183–190.
Niki, H., and Watanabe, M. (1979). Prefrontal and cingulate unit activity during timing behavior in the monkey. Brain Res. 171, 213–224.
Noulhiane, M., Pouthas, V., Hasboun, D., Baulac, M., and Samson, S. (2007). Role of the medial temporal lobe in time estimation in the range of minutes. Neuroreport 18, 1035–1038.
Oliveri, M., Koch, G., Salerno, S., Torriero, S., Lo Gerfo, E., and Caltagirone, C. (2009). Representation of time intervals in the right posterior parietal cortex: implications for a mental time line. Neuroimage 46, 1173–1179.
Olton, D. S., Meck, W. H., and Church, R. M. (1987). Separation of hippocampal and amygdaloid involvement in temporal memory dysfunctions. Brain Res. 404, 180–188.
Penhune, V. B., Zatorre, R. J., and Evans, A. C. (1998). Cerebellar contributions to motor timing: a pet study of auditory and visual rhythm reproduction. J. Cogn. Neurosci. 10, 752–765.
Pentland, A. (1980). Maximum likelihood estimation: the best PEST. Percept. Psychophys. 28, 377–379.
Picton, T. W., Stuss, D. T., Shallice, T., Alexander, M. P., and Gillingham, S. (2006). Keeping time: effects of focal frontal lesions. Neuropsychologia 44, 1195–1209.
Pouthas, V., George, N., Poline, J.-B., Pfeuty, M., VandeMoorteele, P.-F., Hugueville, L., Ferrandez, A.-M., Lehericy, S., LeBihan, D., and Renault, B. (2005). Neural network involved in time perception: an fMRI study comparing long and short interval estimation. Hum. Brain Map. 25, 433–441.
Rammsayer, T. H. (1999). Neuropharmacological evidence for different timing mechanisms in humans. Q. J. Exp. Psych. B 52, 273–286.
Rammsayer, T. H. (2001). Effects of pharmacologically induced changes in NMDA-receptor activity on long-term memory in humans. Learn. Mem. 8, 20–25.
Rao, S. M., Harrington, D. L., Haaland, K. Y., Bobholz, J. A., Cox, R. W., and Binder, J. R. (1997). Distributed neural systems underlying the timing of movements. J. Neurosci. 17, 5528–5535.
Rao, S. M., Mayer, A. R., and Harrington, D. L. (2001). The evolution of brain activation during temporal processing. Nat. Neurosci. 4, 317–323.
Rissman, J., Gazzaley, A., and D’Esposito, M. (2008). Dynamic adjustments in prefrontal, hippocampal, and inferior temporal interactions with increasing visual working memory load. Cereb. Cortex 18, 1618–1629.
Schubotz, R. I., Friederici, A. D., and Yves von Cramon, D. (2000). Time perception and motor timing: a common cortical and subcortical basis revealed by FMRI. Neuroimage 11, 1–12.
Schwartz, M. F., Kimberg, D. Y., Walker, G. M., Faseyitan, O., Brecher, A., Dell, G. S., and Coslett, H. B. (2009). Anterior temporal involvement in semantic word retrieval: voxel-based lesion-symptom mapping evidence from aphasia. Brain 132, 3411–3427.
Shih, L. Y. L., Kuo, W.-J., Yeh, T.-C., Tzeng, O. J. L., and Hsieh, J.-C. (2009). Common neural mechanisms for explicit timing in the sub-second range. Neuroreport 20, 897–901.
Smith, A., Taylor, E., Lidzba, K., and Rubia, K. (2003). A right hemispheric frontocerebellar network for time discrimination of several hundreds of milliseconds. Neuroimage 20, 344–350.
Smith, E. E., and Jonides, J. (1999). Storage and executive processes in the frontal lobes. Science 283, 1657–1661.
Weissman, D. H., Roberts, K. C., Visscher, K. M., and Woldorff, M. G. (2006). The neural basis of momentary lapses in attention. Nat. Neurosci. 9, 971–978.
Wencil, E. B., Coslett, H. B., Aguirre, G. K., and Chatterjee, A. (2010). Carving the clock at its component joints: neural bases for interval timing. J. Neurophysiol. 104, 160–168.
Wichmann, F. A., and Hill, N. J. (2001a). The psychometric function: I. Fitting, sampling, and goodness of fit. Percept. Psychophys. 63, 1293–1313.
Wichmann, F. A., and Hill, N. J. (2001b). The psychometric function: II. Bootstrap-based confidence intervals and sampling. Percept. Psychophys. 63, 1314–1329.
Wiener, M., and Coslett, H. B. (2008). Disruption of temporal processing in a subject with probable frontotemporal dementia. Neuropsychologia 46, 1927–1939.
Wiener, M., Hamilton, R., Turkeltaub, P., Matell, M. S., and Coslett, H. B. (2010a). Fast forward: supramarginal gyrus stimulation alters time measurement. J. Cogn. Neurosci. 22, 23–31.
Wiener, M., Turkeltaub, P., and Coslett, H. B. (2010b). The image of time: a voxel-wise meta-analysis. Neuroimage 49, 1728–1740.
Keywords: time perception, lesion, temporal discrimination, VLSM
Citation: Gooch CM, Wiener M, Hamilton AC and Coslett HB (2011) Temporal discrimination of Sub- and suprasecond time intervals: a voxel-based lesion mapping analysis. Front. Integr. Neurosci. 5:59. doi: 10.3389/fnint.2011.00059
Received: 30 June 2011;
Paper pending published: 21 July 2011;
Accepted: 14 September 2011;
Published online: 04 October 2011.
Edited by:
Warren H. Meck, Duke University, USAReviewed by:
Catalin V. Buhusi, Medical University of South Carolina, USASara Cordes, Boston College, USA
Copyright: © 2011 Gooch, Wiener, Hamilton and Coslett. This is an open-access article subject to a non-exclusive license between the authors and Frontiers Media SA, which permits use, distribution and reproduction in other forums, provided the original authors and source are credited and other Frontiers conditions are complied with.
*Correspondence: Cynthia M. Gooch, Department of Neuroscience, Temple University, Room 818 Weiss Hall, 1701 N. 13th Street, Philadelphia, PA 19122-6085, USA. e-mail:Y2dvb2NoQHRlbXBsZS5lZHU=