- 1Laboratorio Nacional de Ciencias de la Sostenibilidad (LANCIS), Instituto de Ecología, Universidad Nacional Autónoma de México, Ciudad de México, Mexico
- 2Cluster of Excellence-Controlling Microbes to Fight Infection, Interfaculty Institute of Microbiology and Medicine, University of Tübingen, Tübingen, Germany
- 3Laboratorio de Investigación en Procesos Avanzados de Tratamiento de Aguas, Unidad Académica Juriquilla, Instituto de Ingeniería, Universidad Nacional Autónoma de México, Querétaro, Mexico
Climate change and pollution drive the need for fossil fuel alternatives. Dark fermentation offers promise through the use of microbial consortia to convert organic matter into hydrogen gas. Persisting challenges like instability and low yields may stem from reduced diversity of the anaerobic digestion communities that serve as inoculum and undergo aggressive pretreatments and culturing conditions. This study explores the impact of diversity loss on function, focusing on biogas production and stability. Two treatments, with and without aggressive pretreatment, were tested on 12 replicate bioreactors each, resulting in differing microbial diversity levels. Microbial communities were assessed via 16S amplicon sequencing, monitoring biogas production, volatile fatty acids, and testing invasion susceptibility. The two treatments exhibited divergent assembly and functional trajectories, although replicates within each treatment ultimately converged into similar compositions and stable levels of biogas production. Heat-treated bioreactors showed a 91.5% biogas increase but exhibited higher invasion susceptibility compared to non-treated. Non-treated bioreactors showed unique species associations with biogas production (e.g. Ethanoligenens harbinense and Enterococcus olivae), distinct from the commonly studied Clostridium group. These findings provide insights into the effects of diversity loss on stability, elucidating differences across taxonomic and functional stability as well as invasion susceptibility. Moreover, the identification of novel bacterial groups associated with hydrogen production suggests promising directions for future research to enhance microbial consortia control and design in dark fermentation.
1 Introduction
The phylogenetic and metabolic diversity of microbes has been the source of major biotechnological advances, from harnessing or engineering the metabolic capabilities of single strains (e.g. yeast fermentation) to complex communities (e.g. methane production) (Escalante et al., 2015; Giri et al., 2020; Ergal et al., 2022). Nonetheless, in the pursuit of maximizing yields and operational control, a strong emphasis has been put on single strain cultures or engineered consortia, leading to great success in medical and food industries (i.e. insulin production, alcohol, and bread production) but hindering progress in others that are dependent on more complex biochemical transformations that require the coexistence of multiple microbial groups, in other words, microbial functional diversity (Escalas et al., 2019). It has been long recognized that microbial communities have varying degrees of compositional responses in response to environmental perturbations (Shade et al., 2012). Yet, the impact on function and robustness of, both, compositional changes and the decrease in the different components of diversity (e.g. species richness) has been a matter of debate for a long time (Nemergut et al., 2014; Bier et al., 2015; Lee et al., 2023), specially, given the well-known functional redundancy in microbes (Louca et al., 2018). Function can be understood and measured from different angles. In several systems (either environmental or biotechnological) function is often evaluated, albeit reductively, as specific productivity measures in terms of growth rates or biomass and metabolite yields (Lipson, 2015; Li et al., 2019b; Sarangi and Nanda, 2020). Here, we propose that other aspects of function may also be considered when evaluating the consequences of diversity perturbations, such as stability and resilience. In this sense, it has been suggested that the increase in diversity components (i.e. richness and evenness) leads to higher stability and functional resilience due to functional redundancy, niche complementation and differential response traits against perturbations (Shade et al., 2012). Although almost no consensus has been reached in this sense, microbial interactions are generally regarded as fundamental ecological processes determining microbial community assembly and function (Li and Müller, 2023). Since microbial communities carry out important processes like nutrient cycling, substrate degradation and metabolite production, understanding the mechanisms that drive the diversity-function relationship can help in the management of microbial communities, for example, in therapeutic, biotechnological or bioremediation settings (Shade, 2023; van Leeuwen et al., 2023; Villanueva-Galindo et al., 2023).
Using simplified microbial consortia which show reduced complexity (e.g. in terms of species diversity and interactions) can help understand the mechanisms that link diversity and function (Sun and Sanchez, 2023). For instance, low-diversity microbial consortia (like those used for biofuels and food production or microbiome-based synthetic communities) have been used to test ecological hypotheses and propose generalizable ecological theories since they offer controlled environments and easily measurable functions (Stenuit and Agathos, 2015; Cairns et al., 2018; Michielsen et al., 2024). Ecological mechanisms that have been shown to be important to the assembly of microbial communities can be deterministic (like species interactions and niche differentiation) or stochastic (such as birth-rate drift) (Yuan et al., 2019; Wang et al., 2023). Due to the intrinsic characteristics of microbial organisms (i.e. their small size and chemically-mediated interactions; Schmidt et al., 2015), the exploration of ecological mechanisms (e.g. biotic interactions or the species richness-community function relationship) in microbial communities is frequently investigated via statistical inference. In this regard, to advance the knowledge of the ecological dynamics of microbial consortia, experiments can be specifically designed to this end (Navarro-Díaz et al., 2020). Dark fermentation consortia, a type of hydrogen-producing microbial communities, represent a good system to study ecological hypotheses since they are low-diversity systems with well-studied measurable functions (Wang et al., 2020). Dark fermentation consortia are derived from anaerobic digestion communities in which hydrogen-producing bacteria are selected by using aggressive pretreatments and strictly controlling culture conditions (Wang and Yin, 2017). In hydrogen-producing reactors, instability, invasion by lactic acid bacteria (LAB) and low yield are still unresolved issues and proposed causes might involve biodiversity loss and competitive interactions (Castelló et al., 2020; Thulasisingh et al., 2023). Thus, understanding the ecological mechanisms that underlie population dynamics in hydrogen-producing consortia can provide insights into predicting and controlling community-level properties.
In this work, we studied the effect that changes in microbial diversity (species richness) have on the function and stability of microbial consortia, using hydrogen-producing consortia as a model system. Since species richness is an important component of diversity, we will use both terms interchangeably. We adopted two commonly used strategies for achieving hydrogen production and established two sets of microbial consortia with two levels of species richness from the same inoculum. The first strategy consisted of applying an aggressive heat-shock pretreatment to the inoculum while the second consisted of maintaining specific culture conditions previously reported for hydrogen production. We followed biogas production until stabilization and characterized microbial composition and volatile fatty acids during this time. Then, we performed an invasion experiment to test the invasion susceptibility of each treatment. We hypothesize that since diversity determines the function and stability of microbial consortia, each of the two sets of hydrogen-producing bioreactors will differ not only in their levels of diversity but also in their long-term behavior and resistance to perturbations (like invasion).
2 Materials and methods
2.1 Experimental design
To investigate if microbial diversity affects the ecological robustness of dark fermentation microbial consortia, we used lab-scale bioreactors inoculated with anaerobic digestion granules and treated them to generate contrasting diversity, which permitted to evaluate the functional outcomes of changes in diversity levels. The experiment was conducted in two sequential stages, in the first stage we intended to specifically evaluate the effect of diversity on the functional stability of the bioreactors; in the second stage, we challenged the bioreactors with a controlled invasion of Lactobacillus plantarum culture. For the first stage, we artificially reduced the diversity of the original inoculum with a heat-shock treatment (Valdez-Vazquez and Poggi-Varaldo, 2009) to further compare the microbial community dynamics and performance of the heat-shock-treated microbial communities against the non-treated communities. Twelve replicates per treatment (heat-shock-treated and non-treated) were maintained with daily transfers into fresh medium until biogas production stability was reached. After biogas production stabilized, for each of the two “diversity treatments” we chose six random replicates to start the second stage of the experiment. The six chosen replicates per diversity treatment were divided into two new sets of bioreactors. One set was inoculated with a strain of L. plantarum that was isolated from a hydrogen-producing bioreactor anaerobic bioreactor (Perez-Rangel et al., 2021), the other six were used as non-invasion controls (Figure 1). The function or performance of the bioreactors throughout the experiments was determined based on two parameters: daily biogas production and volatile fatty acids (VFAs) concentration. Microbial diversity and composition were determined by culture-independent 16S rDNA amplicon high throughput sequencing.
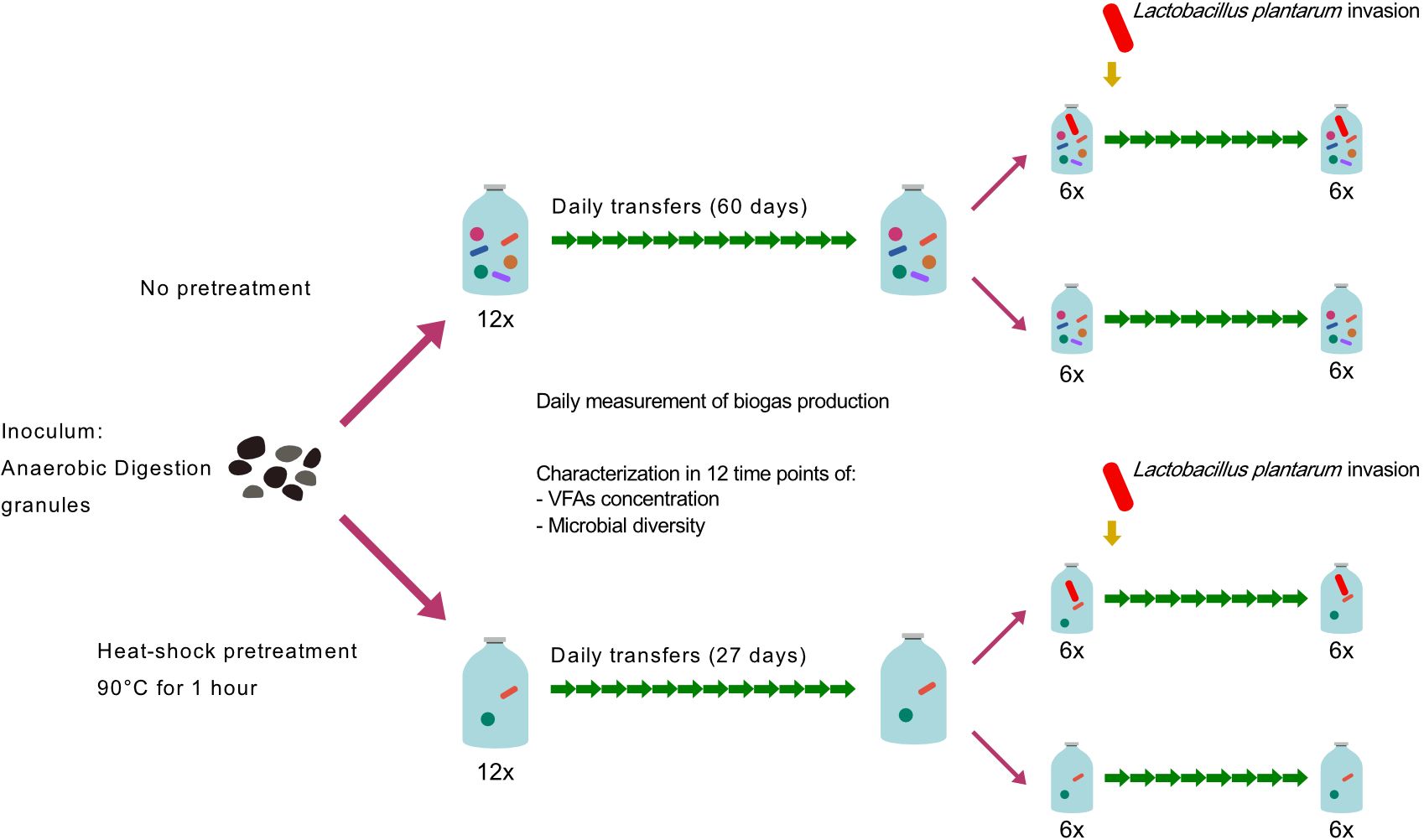
Figure 1 Experimental design. Starting from a single inoculum, we followed two experimental treatments to artificially modify microbial diversity. Each treatment consisted of 12 replicates derived from a single inoculum. To test the stability of reactors in each treatment, we periodically measured the performance of bioreactors until biogas production stabilized. After biogas production stabilization, we challenged the reactors with a controlled invasion and determined the success of the invasive bacteria based on their abundance in the reactors. In the invasion experiment, 6 bioreactors per treatment were selected and divided into two sets of bioreactors. One set was inoculated with Lactobacillus plantarum simulating a biological invasion, while the remaining 6 bioreactors per treatment were used as controls. L. plantarum was used as an invader since Lactobacillus species are well known for causing the collapse of hydrogen-producing bioreactors (Sikora et al., 2013). After biogas production in invaded bioreactors stabilized, the experiment was terminated. All the data on the diversity and performance of the bioreactors were subject to statistical analyses for formal comparisons of the experimental treatments.
2.2 Setup of bioreactors
To compare the effect of diversity on the function and stability of bioreactors, we artificially reduced the diversity of the original inoculum with a heat-shock treatment. The original microbial inoculum consisted of anaerobic digestion granules obtained from a brewery wastewater treatment plant. The total solids (TS) and volatile solids (VS) concentration of the inoculum was 212.61 ± 15.08 mg/g and 195.99 ± 15.14 mg/g respectively. Before the experiment, the inoculum for the two initial cultures for each treatment was prepared by homogenizing 15 g of the anaerobic granules in 20 mL of 1:1 PBS/glycerol solution with steel beads in a Vortex Genie 2 for 5 minutes at max speed. After homogenization, inocula samples were frozen at –80°C until use. To establish the initial cultures for each treatment, first, we thawed the homogenized inoculum and added 20 mL of inoculum to 40 mL of culture medium. We grew the culture overnight at 37°C at 100 rpm. In the case of the heat-shock pretreatment, we heated the overnight culture to 90°C for 1h using a laboratory oven. Since, after the heat-shock pretreatment, no growth was achieved under aerobic conditions, strictly anaerobic conditions had to be used in this treatment by adding 0.5 g/L of cysteine to the culture medium. Once initial cultures were established for both treatments (non-treated and heat-shock-treated bioreactors), the initial cultures were subdivided into 12 replicates per treatment. To partition each culture, every 24 hours, two 20 mL samples of the culture were taken, and each was transferred into a new serum bottle with 40 mL of fresh medium. This partitioning step was repeated 4 times (one partition per day for four days) to achieve 16 replicates from which 12 random replicates were used for each treatment. From that point, every 24 hours, 20 mL of the culture of each replicate culture was reinoculated into 40 mL of fresh medium. After each transfer, the pH was adjusted to 6 as it has been found that a slightly acid pH is favorable for hydrogen production and methanogenesis control (Ghimire et al., 2015). The medium had the following composition per liter: glucose (5 g/L), urea (0.65 g/L), K2HPO4 (0.25 g/L), MgCl2·6H2O (0.376 g/L), FeSO4· 7H2O (0.1 g/L), CoCl2·6H2O (0.0025 g/L), MnCl2·4H2O (0.0025 g/L), KI (0.0025 g/L), NiCl2·6H2O (0.0005 g/L), ZnCl2 (0.0005 mg/L) and yeast extract (0.5 g/L); modified from (Mizuno, 2000). For all the cultures we used 100 mL serum bottles as lab-scale semi-continuous bioreactors with a working volume of 60 mL. Bioreactors were incubated at 37°C and shaken at 100 rpm.
2.3 Characterization of biogas production
To characterize the performance of the bioreactors, we measured daily biogas production. Biogas production was measured in an inverted graduated cylinder using the water displacement technique and, to ensure an exact measurement, we prevented CO2 absorption by using a 5 N HCL solution (pH < 2; Boshagh and Rostami, 2020). We maintained the bioreactors for at least 4 weeks or until stability was achieved. We calculated the biogas production stability index for each replicate using the biogas production of the previous 3 days (stability index measures the variance in biogas production during a period; Tenca et al., 2011). When the mean of the biogas stability index of the 12 replicates was above 0.9 for 3 consecutive days, we considered stability was achieved. We also calculated the final biogas stability index for each replicate and the mean of all replicates for each treatment.
2.4 Invasion test
After the initial stabilization period, 6 random replicates for each treatment were divided again (using the partition methodology described in the Setup of Bioreactors section) and one of the derived bioreactors for each replicate was inoculated with a strain of Lactobacillus plantarum. The L. plantarum strain was isolated from a hydrogen-producing bioreactor degrading wheat straw, operating at 37°C and pH 6.5 (Perez-Rangel et al., 2021). The strain of L. plantarum was grown overnight in LB medium (Sigma Aldrich, USA) and then acclimatized for 3 days in the same medium and conditions that were used in our bioreactors. The invasion was performed at a 10% ratio of the L. plantarum culture at an optical density of 1. For this, we followed the same protocol for daily transfers, but instead of just 40 mL of fresh medium, we used 34 mL of fresh medium and 6 mL of the overnight culture of L. plantarum.
2.5 Characterization of complementary functions of bioreactors
In addition to biogas production, we measured volatile fatty acids (VFAs) concentration as proxy measures of the function of bioreactors. Volatile fatty acids (VFAs) concentration was determined using an SRI 8610-00 gas chromatograph equipped with a Porapak Q column and using Helium at a 30 mL/min flow rate as carrying gas. Temperatures for the injector, column and oven were 150°C, 50°C and 50°C respectively.
2.6 Characterization of microbial diversity
At the same time points in which chemical analyses were performed, we obtained culture samples for microbial composition analyses. Samples were stored at −80°C until processing. DNA was extracted using the Quick-DNA Fungal/Bacterial DNA Microprep Kit (Zymo Research, USA) following the manufacturer’s instructions. Sequencing library preparation was performed targeting the V4 region of the 16S SSU rRNA gene using primers 515F: GTGYCAGCMGCCGCGGTAA (Parada et al., 2016) and 806R: GGACTACNVGGGTWTCTAAT (Apprill et al., 2015). Amplifications were performed in 25 µL reactions with the Platinum Hot Start PCR Master Mix (Invitrogen, USA), 0.5 µL of 10 mM primers, and 1 µL of DNA template at a concentration of 20 ng/µL. Negative controls were included for each PCR reaction to ensure that no contamination occurred. Later, amplification products were visualized using electrophoresis gels. Finally, the sequencing reaction was performed using the Miseq 300PE platform at the Macrogen facilities (Korea) using Reagent V3 and Phix at 30% and with a red length of 250PE.
2.7 16S rRNA sequence data processing
2.7.1 De-multiplexing, filtering, and chimera check
Raw sequences (10,540,264) were demultiplexed using the demuxbyname script from the BBTools suite (Bushnell B., sourceforge.net/projects/bbmap/). Demultiplexed sequences were next processed using QIIME 2 v2021.2 (Bolyen et al., 2019). Sequence quality control (denoising and chimera check) and inference of amplicon sequence variants (ASVs) were performed using the dada2 denoise-paired command. We modified the trim left f, trun len f, trim left r, and trun len r parameters to remove 30 bp from both extremes of the reverse and forward sequences. After quality control, 7,893,591 (74.89%) sequences were retained.
2.7.2 ASV assignment
After quality filtering, samples were rarefied to the average of reads per sample (27,082 reads) using the qiime feature-table rarefy command with the –p-with-replacement argument in QIIME 2 v 2021.2. Taxonomy was assigned to 115 ASVs using BLAST (Altschu et al., 1990) against the NCBI’s 16S ribosomal RNA refseq database (O’Leary et al., 2016). Finally, to improve pattern recognition and reduce technical variability, ASVs with less than 20 reads in 20% of the samples were filtered out (retaining 24 ASVs) of the following analyses based on the filtering criteria published previously (filtering ASVs with less than m = 20 counts in at least k = 20 samples, where m and k were selected as 0.1% of the minimum sample library size which was ~20,000; Cao et al., 2020).
2.8 Statistical analyses
To assess the variability of microbial function over time, for each treatment, we plotted the 12 bioreactors function data (biogas production and VFAs). Similarly, to assess the variation of the average microbial abundance over time we used stacked bar plots of the average abundance of each ASV for the 12 replicates. To investigate how low-abundance ASVs behaved over time the average abundance was plotted in a heatmap, normalizing ASVs abundance across samples. To analyze the relationship between microbial composition and bioreactors we followed two approaches. First, we performed Spearman correlations between ASVs abundance counts and metabolites concentrations and biogas production obtained using the cor function in the stats R package v4.0.5. We represented the correlations as heatmaps for visual interpretation. Then, we used the cca function in the vegan R package v.2.5-7 (Oksanen et al., 2013) to perform a canonical correspondence analysis (CCA) using the abundance counts of ASVs and normalized metabolite concentrations and biogas production. All analyses were performed using R v.4.0.5 (R Core Team, 2013). Finally, to infer the potential interactions occurring between the members of the hydrogen-producing consortia, we used the Lotka-Volterra-based network inference approach implemented in MetaMIS v1.02 (Shaw et al., 2016). For each sampling time point, the average of the microbial abundance of the 12 replicates was computed and used to infer ecological networks. The networks were visualized using Cytoscape 3.0 (Shannon et al., 2003).
3 Results
3.1 Bioreactor’s function
All replicates of both treatments showed increased and stabilized biogas production over time but peaked at different times depending on the treatment. The heat-shock-treated bioreactors showed higher production and stable production was achieved in comparison with the non-treated reactors (916.2 ± 29.9 mL/L vs. 1754.6 ± 2.89 mL/L, a 91.5% increase; Figure 2), biogas stability index was higher in heat-treated bioreactors (0.904 vs. 0.614) than in non-treated reactors. Regarding VFAs, acetic and butyric acids were the most abundant in both treatments indicating hydrogen production (Figure 3), although gas composition wasn’t measured. Both treatments showed high acetic and butyric acid production but the trend in concentration change was different for both treatments. In non-treated bioreactors, acetic and butyric acid concentrations initially increased and then stabilized, while in treated bioreactors, VFAs and biogas showed a consistently stable production pattern. Furthermore, in the non-treated bioreactors, propionic acid was also constantly produced.
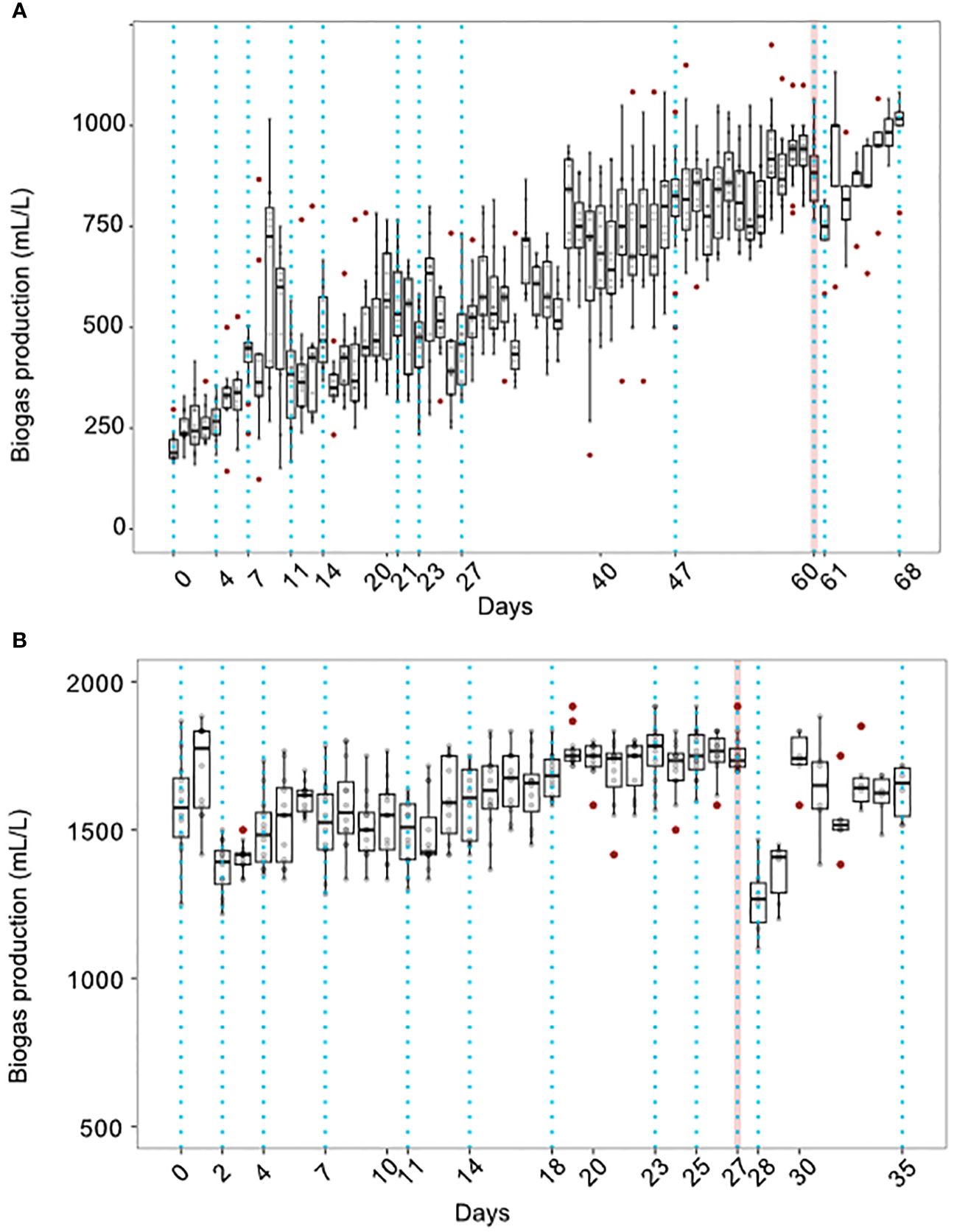
Figure 2 Biogas production over time in the non-treated (A) and heat-shock-treated (B) bioreactors. For each box, red dots represent outliers (1.5 interquartile ranges above or below the first quartile). Dotted vertical lines represent the sampling days for microbial and VFAs characterization. The red vertical line represents the day when the invasion of L. plantarum was performed. 12 replicates were measured per day until the invasion day where only the 6 invaded samples are shown.
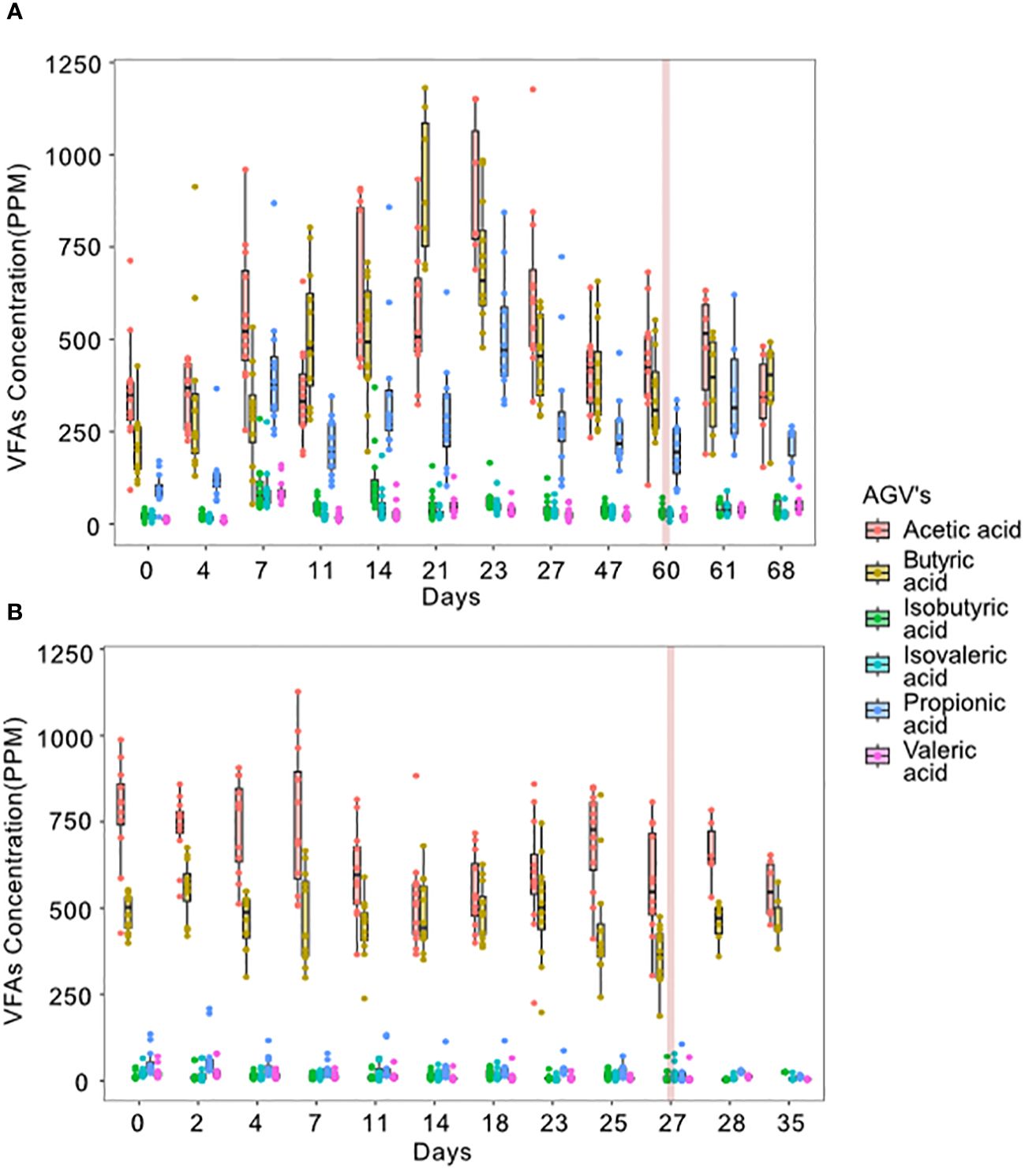
Figure 3 Volatile fatty acids production over time in the non-treated (A) and heat-shock-treated (B) bioreactors. The red vertical line represents the day when the invasion of L. plantarum was performed. 12replicates were measured per day until the invasion day where only the 6 invaded samples are shown.
The effect of the invasion by L. plantarum on the function of the bioreactors was different in each of the treatments. In both experiments, invasion induced a reduction in biogas production observed 24 hours after the inoculation. The reduction was steeper in the heat-shock-treated bioreactors than in the non-treated bioreactors (72.15% vs. 85.03% of the original biogas production respectively). After the initial reduction, biogas production increased again to levels observed before the invasion (93.54% and 92.87% of the original biogas production in the non-treated vs. the heat-shock-treated bioreactors respectively). However, in the non-treated bioreactors, one replicate did not recover from invasion and stopped producing biogas. In Figure 2 the biogas production is shown without the collapsed bioreactor after the invasion. In this figure, it can be observed that, only in the non-treated bioreactors, the biogas recovery even surpassed biogas production before the invasion (reaching 111.49%). In Supplementary Figure 1, the biogas production in the non-treated bioreactors including the non-recovered replicate is also shown. The concentration of the analyzed VFAs was not seemingly affected by the invasion in either treatment (Figure 3).
3.2 Microbial composition
In non-treated bioreactors, the microbial community was initially dominated by species like Citrobacter amalonaticus, Clostridium butyricum, and Enterococcus xiangfangensis (Figure 4). However, as the experiment progressed, their abundance decreased, and Ethanoligenens harbinense, Enterococcus olivae, E. xiangfangensis, and Clostridium pasteurianum became dominant. Additionally, 15 rare ASVs made up a significant portion of bacterial abundance, showing independent dynamics from the dominant species (Figure 5). These rare ASVs maintained stable levels throughout the 60-day experiment. In the heat-shock treatment bioreactors, only two ASVs (C. guangxiense and C. pasteurianum) were present, with C. guangxiense being the dominant one, and their abundance remained stable throughout the experiment.
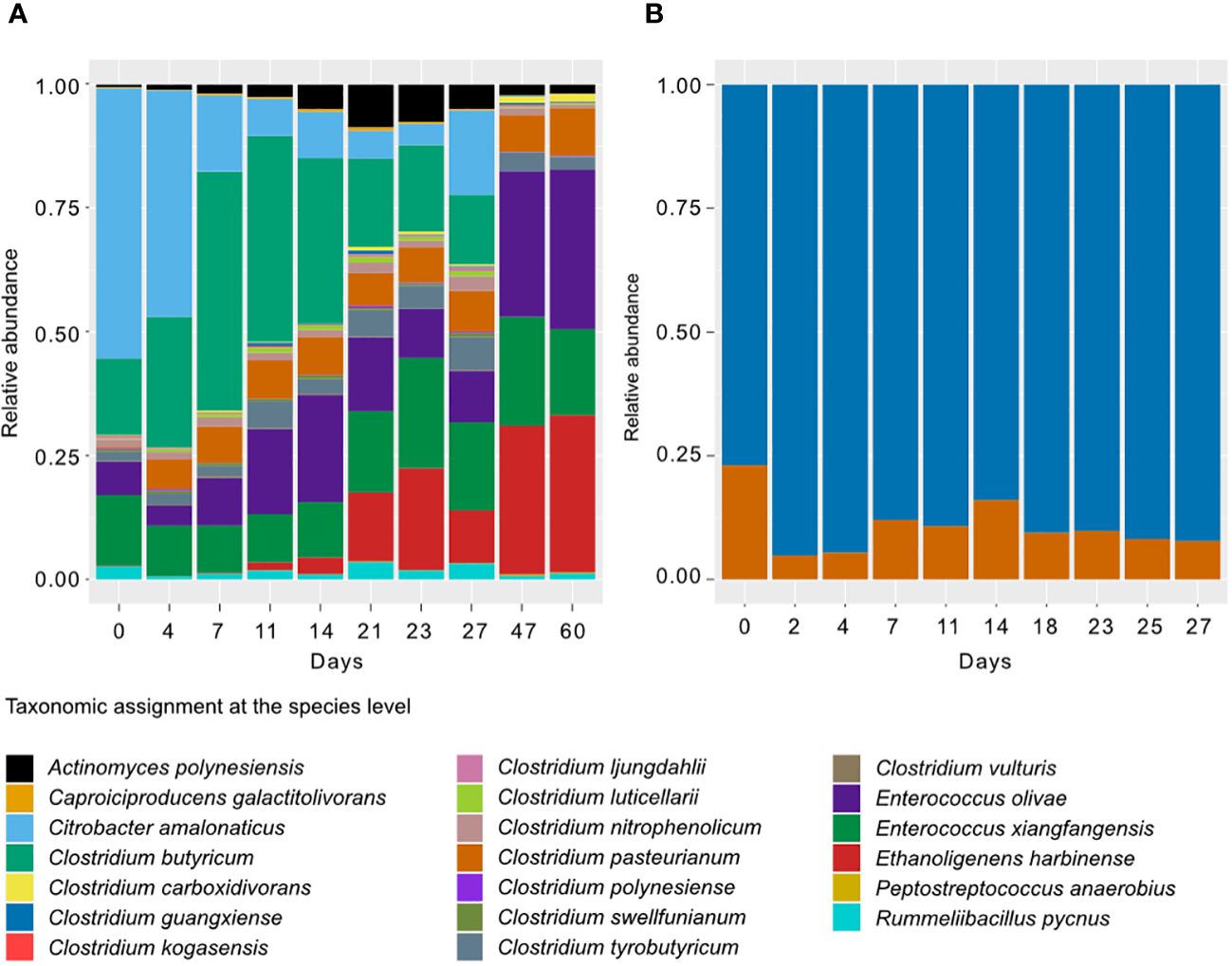
Figure 4 Microbial diversity composition per treatment and over time. Mean relative abundance (N=12) of different amplicon sequence variants (ASVs) in thenon-treated (A) and heat-shock-treated (B) bioreactors.
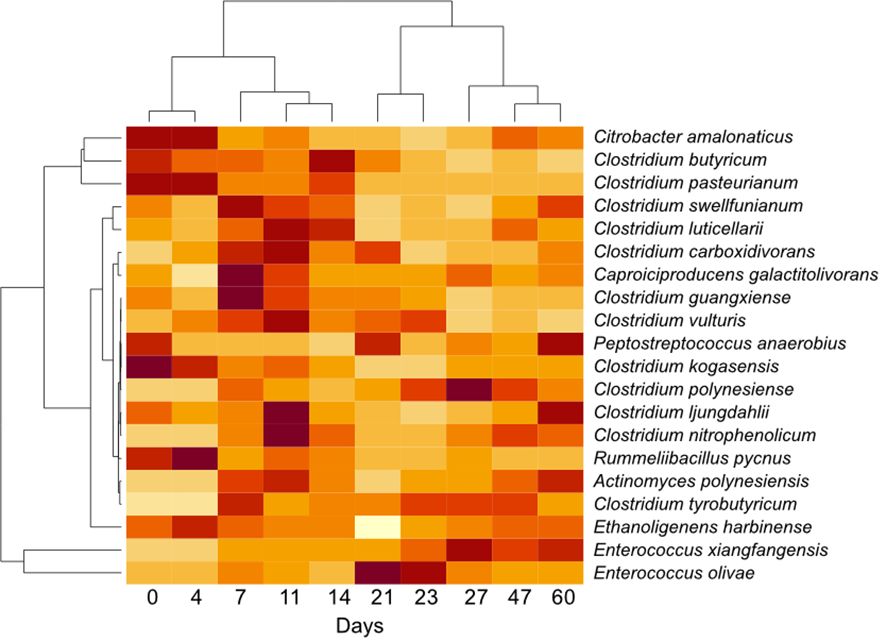
Figure 5 Heatmap showing the variability of the relative bacterial abundance over time in the non-treated bioreactors. Abundance is standardized per ASV to reflect variability between times for each ASV. Each day represents the average abundance per ASV (n = 12).
3.3 Relationship between microbial diversity and function of bioreactors
Since only two species survived in the heat-treated bioreactors and little variation was observed in their function, all correlation and multivariate analyses were performed only for the communities of non-treated bioreactors. Biogas production was positively correlated to the abundance of certain ASVs. For instance, E. harbinense (r = 0.52), C. pasterianum (r = 0.22), E. olivae (r = 0.55), Clostridum carboxidorans (r = 0.51) and Peptostreptococcus anaerobius (r = 0.42) are significatively positively correlated with biogas production (all p-values < 0.05). Contrarily, C. amalonaticus (r = −0.58), C. butyricum (r = −0.36), Clostridium swellfunianum (r = −0.39), Clostridium vulturis (r = −0.24), Rummelibacillus pycnus (r = −0.21) and Clostridium nitrophenolicum (r = 0.25) were negatively correlated (all p-values < 0.05; CCA in Figure 6 and Pearson correlations in Figure 7). The bacteria that had negative correlations with biogas production tended to present positive correlations with, either butyric acid and/or propionic acid. For instance, ASVs like R. pycnus were positively related to butyric acid (r = 0.3, p-value < 0.01), while Clostridium luticellarii was positively related to, both, butyric and propionic acids (r = 0.45 and 0.21, p-values < 0.05). The rest of the analyzed volatile acids (isobutyric acid, isovaleric acid and valeric acid) and microbial ASVs show no clear relationship to biogas production. The CCA (Figure 6), also shows that the structure of the microbial composition of samples in all replicates followed a similar composition and function during the experiment. Thus, samples from earlier times in the experiment showed similar microbial structure and functional performance to samples from the end of the experiment.
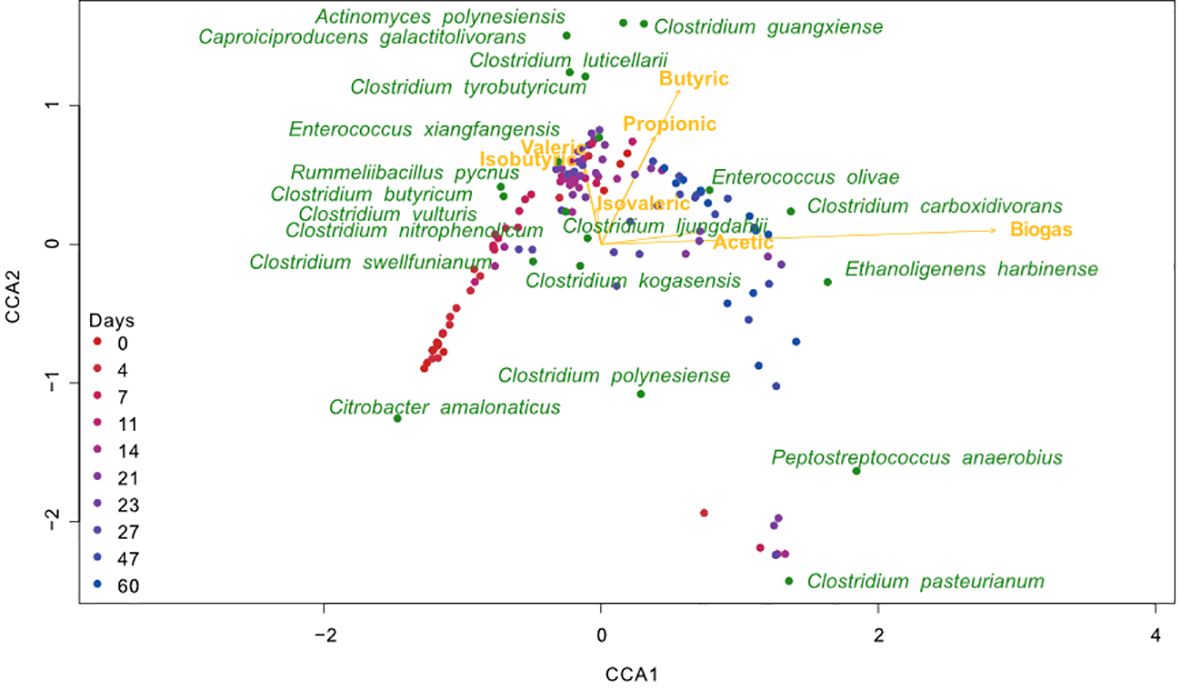
Figure 6 Canonical correspondence analysis (CCA) biplot (n = 120,12 per day). CCA representing the relationship between bioreactors function (biogas and volatile fatty acids) and microbial composition in the non-treated bioreactors. Yellow points represent ASVs, vectors represent metabolites and hollow points represent samples colored by the time of the experiment when they were taken. Eigenvalues: axis 1 = 0.324, axis 2 = 0.111. Percentageof variance explained:15.54(axis 1), 5.33(axis2). Cumulative percentage explained: 20.88. Model significance (p-value < 0.001). Significance of constraining variables: Biogas (p-value < 0.001); Butyric acid (p-value < 0.001); Isovaleric (p-value0.118); Acetic acid (p-value 0.007); Isobutyric acid (p-value 0.154); Valeric acid (p-value 0.273); Propionic acid (p-value < 0.005).
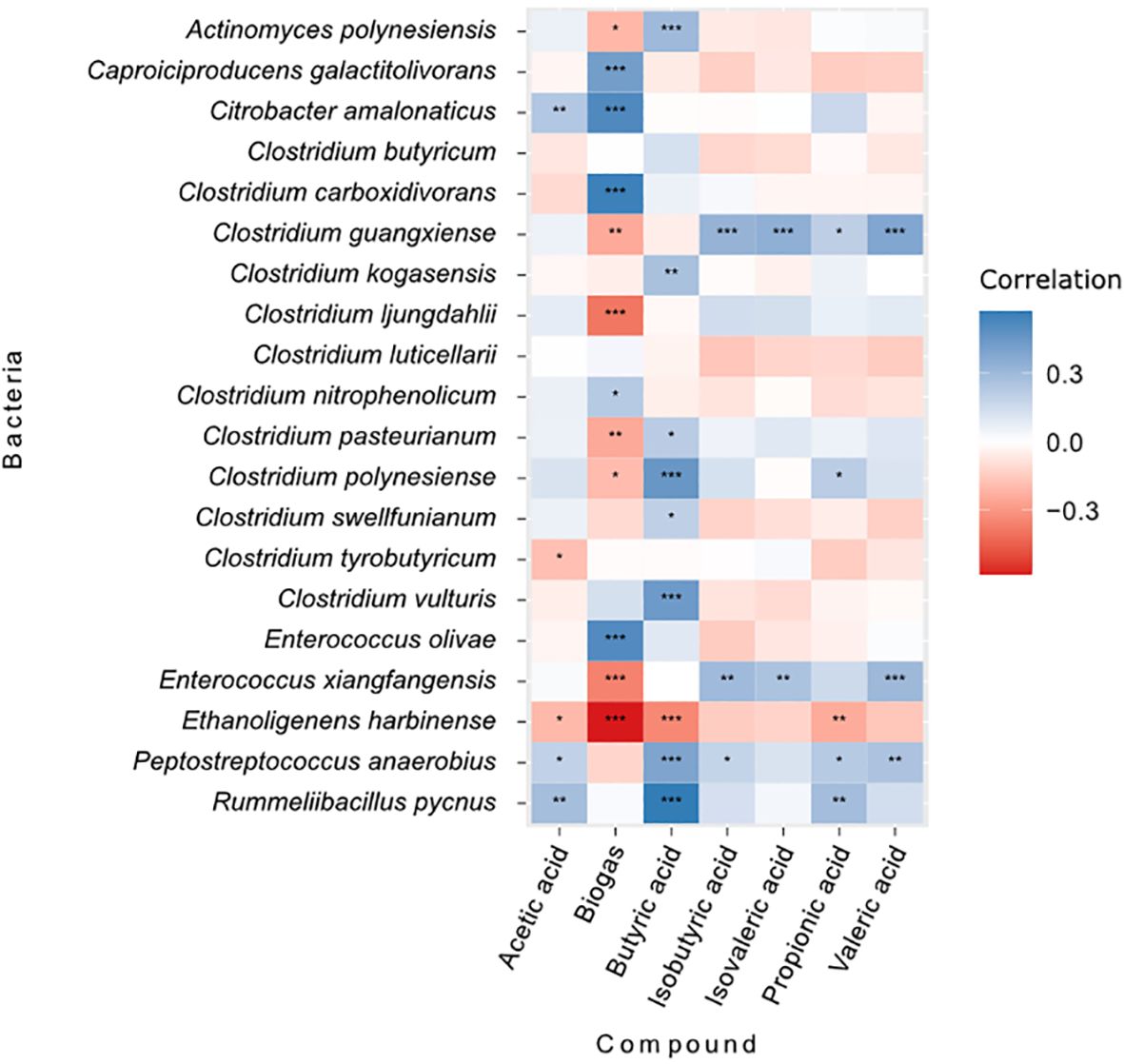
Figure 7 Pearson correlations between microbial abundance, biogas production and volatile fatty acids concentration in the non-treated bioreactors (n=120). Red shades represent negative correlations and blue shades represent positive correlations. Stars inside each cell represent the significance of the correlation (“***” = 0.001; “**” = 0.01; “*” = 0.05).
3.4 Potential ecological interactions
The inferred interactions show potential benefits for certain bacteria positively correlated with biogas production (e.g. E. harbinense and E. olivae) and potential harm for those negatively linked to biogas production (like C. amanoliticus and C. butyricum; Figure 8). For instance, it was inferred that E. harbinense and E. olivae might have benefited from the presence of C. pasteurianum. Conversely, C. amanoliticus showed a potentially negative relationship with C. pasteurianum and R. pycnus, while C. butyricum was affected by E. xiangfangensis. Meanwhile, C. nitrophenolicum, though low in abundance, had several interactions potentially impacting the consortia composition. For example, it might have benefited E. harbinense but was negatively affected by C. amanoliticus and C. butyricum. In turn, C. nitrophenolicum could have benefitted from the presence of E. xiangfangensis and Clostridium tyrobutyricum.
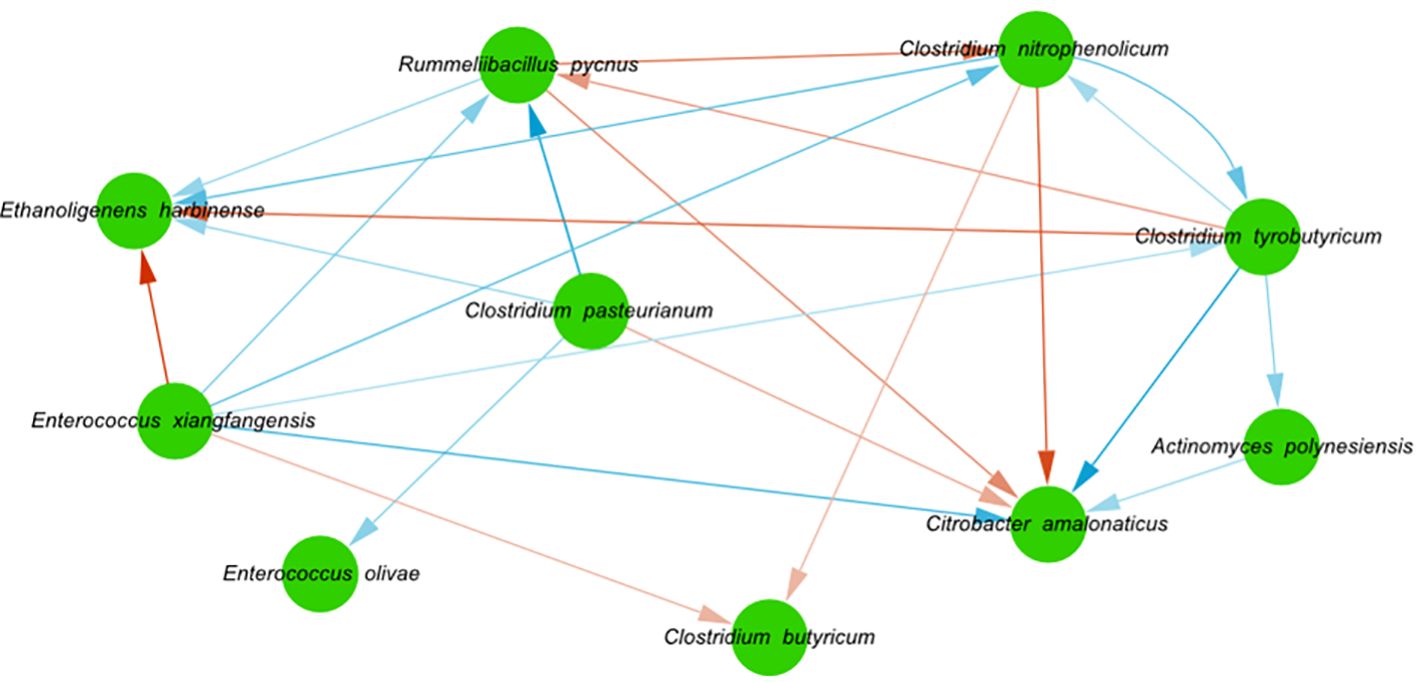
Figure 8 Interactions inferred in the non-treated bioreactors. Nodes denote bacterial species, blue edges denote positive significant interactions, red edges denote negative significant interactions, the direction of the arrows represents the direction of the interaction, and the intensity of the color of the edges represents the strength of the interaction.
3.5 Robustness to invasion
Both treatments resisted the invasion of L. plantarum, but they exhibited distinct responses (Figure 9). In non-treated bioreactors, L. plantarum was initially found in all inoculated bioreactors after 24 hours. However, after a week, it was only detectable in one bioreactor, where it became dominant, leading to a collapse in biogas production. In contrast, in heat-shock-treated bioreactors, L. plantarum was initially present 24 hours after inoculation but never became dominant. Instead, it persisted in small quantities after a week. Ultimately, bioreactors without established L. plantarum maintained a microbial composition similar to pre-inoculation levels.
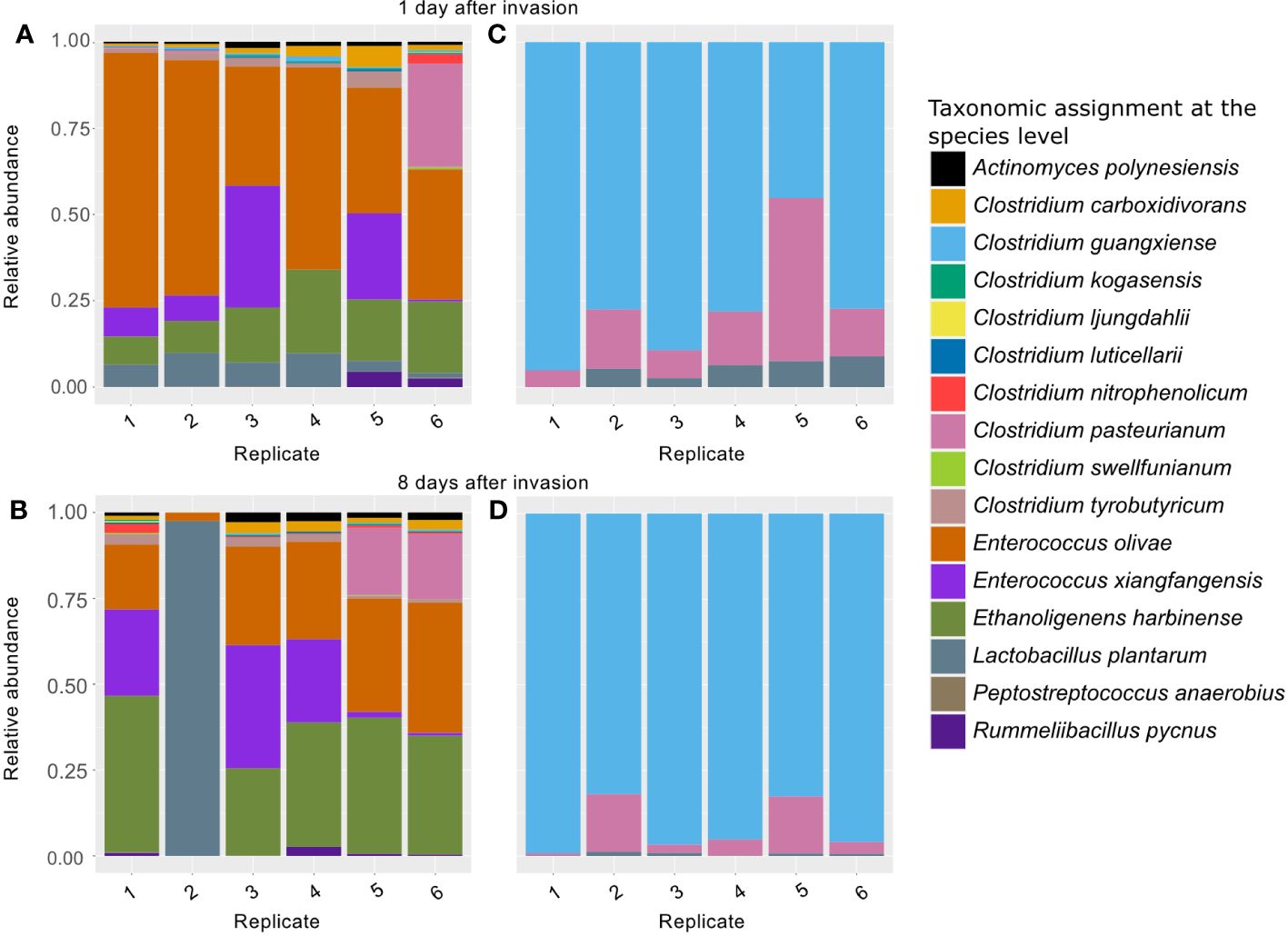
Figure 9 Microbial composition after 6 replicates were divided and inoculated with L. plantarum. Non-treated bioreactors 1 day (A) and 7 days (B) after the inoculation. Heat-shock-treated diversity bioreactors 1 day (C) and 7 days (D) after the inoculation.
4 Discussion
Although microbial composition and function are dependent, not only on the culture conditions but also on the biological characteristics of the microorganisms involved, the mechanisms behind the diversity-function relationship are a matter of investigation (Louca et al., 2018; Escalas et al., 2019; Sanchez et al., 2023). In hydrogen-producing bioreactors, harnessing microbial diversity has been a challenging endeavor, as culture conditions to achieve hydrogen production have proven to have distinctive impacts on microbial diversity (from mild to drastic pretreatments that reduce microbial diversity), altering the structure, function and stability of the microbial communities with unknown ecological consequences (Philippot et al., 2013; Wagg et al., 2014; Hernandez-Teran et al., 2020). In this work, we aimed to analyze the effect of reducing the diversity of microbial consortia on biotic interactions and system-level properties, such as stability and resilience.
4.1 Treatments on inoculum predictably affected the diversity, structure and function
To determine how variation in microbial diversity (i.e. richness) affected function, we periodically tracked the biogas and volatile fatty acid production in both, the non-treated and heat-shock-treated bioreactors. Additionally, we conducted culture-independent molecular analysis on microbial diversity across 10 timepoints in the 12 replicates for each treatment to understand the microbial composition driving biogas production and the differences between the two treatments. In this sense, the two treatments in our work show very contrasting patterns of assembly and functional stabilization (Figures 2, 3), which might have been the result of different mechanisms.
In the heat-treated bioreactors, microbial richness was severely reduced consisting only of C. guangxiense (which dominated the bioreactors) and C. pasteurianum. Although our case is an extreme example of reduced microbial diversity, other experiments also show very few ASVs (e.g. Muñoz-Páez et al., 2019). In terms of biogas production, a quick stability was reached. This function and compositional patterns are in accordance with the previous observations that pretreated communities show long-term biogas production stability under certain conditions (selective enrichment of Clostridum species, and sterile temperature- and pH-controlled conditions) as those used in this work for the heat-treated bioreactors (Singh and Wahid, 2015; Cabrol et al., 2017). It is worth noticing that the stability observed in the heat-shock-treated bioreactors of our experiment might be deceiving. When scaling the process to industrial settings, anaerobic and further controlled conditions and sterility, often needed as a result of selective pretreatments (Hawkes et al., 2002), are difficult to maintain and not cost-effective (Cabrol et al., 2017). High abundance of Clostridum species was long considered as the optimal composition to obtain high hydrogen production in dark fermentation bioreactors (Valdez-Vazquez and Poggi-Varaldo, 2009) until most recent works drove attention to other diversity and abundance patterns in community structure that comprise, not only of hydrogen-producers, but also of other bacterial groups (e.g. Bacillus, lactic acid bacteria or enterobacteria) performing complementary functions like aggregation or detoxification (Cabrol et al., 2017; Lopez-Hidalgo et al., 2022). Other advantages in terms of mixed consortia in hydrogen production include easiness in bioreactor management and substrate utilization but the fact that ecological interactions are not understood makes it difficult to predict an improve their performance (Mohanakrishna and Pengadeth, 2024).
In the non-treated bioreactors, a diverse community was established in the bioreactors and underwent a complex process of assembly and stabilization. This gradual shift in microbial composition was accompanied by a subsequential increase in biogas production. The pattern of increasing biogas production shown by the non-treated bioreactors has been reported in previous experiments (Kim and Shin, 2008; Kannaiah Goud et al., 2014) being the main cause of the aforementioned shift in microbial composition (Palomo-Briones et al., 2018; Yang and Wang, 2019; Castelló et al., 2020). For instance, E. harbinense and C. pasteurianum, were the two most dominant species toward the end of the experiment when biogas production increased, and are known as hydrogen-rich biogas producers (Wang et al., 2009; Masset et al., 2012; Srivastava et al., 2017). Also, Enterococcus species, commonly found in hydrogen-producing consortia (Lopez-Hidalgo et al., 2022), where identified. Species of these genera are known to be hydrogen producers (Laxman Pachapur et al., 2015; Wang and Yin, 2017; Braga et al., 2018) but are not generally studied in hydrogen-producing consortia and further investigation on their role on hydrogen production is needed. In this sense, E. olivae was the most abundant Enterococcus species in the non-treated bioreactors and although it has been reported to produce biogas on glucose (Lucena-Padros et al., 2014) this gas was not identified so, although possible, it is not clear if and how E. olivae could have contributed to biogas production. On the other hand, C. carboxidivorans is a homoacetogen capable of utilizing H2 and CO2 for growth (Fuentes et al., 2021). The positive relationship between C. carboxidivorans and biogas production might be due to increased growth fueled by the capacity of utilizing hydrogen-rich biogas.
Importantly, the mechanisms giving rise to a change in community composition (and thus function) during the assembly of microbial communities in bioreactors are not widely explored. In other type of communities several mechanisms ranging from stochastic to deterministic (from which one of the most influential are biotic interactions) have been identified (Estrela et al., 2022) and thus are discussed further in the next section.
4.2 Stability in diversity treatments was the result of distinctive ecological interactions
The impact of ecological microbial interactions on composition and function has been studied in several systems. Resolving how these ecological interactions work during the assembly of microbial communities might help to improve our predictive power over them allowing us to harness their capabilities. In our work, we statistically inferred the potential microbial interactions of the non-treated bioreactors to understand the reasons behind the assembly process. Microbial interactions have been found to be important in determining community-level functions such as antibiotic resistance, host-pathogen resistance and the result of biotechnological processes (Marcelino et al., 2023; Melkonian et al., 2023; Mizrahi et al., 2023).
Our results indicate that ecological interactions could have influenced the observed dynamics in contrasting ways. In the non-treated bioreactors, more ASVs meant more chances that interactions with strong effects on composition and function occurred. In particular, we hypothesize that positive interactions toward hydrogen producers from the rest of the community resulted in their gradual abundance increase (e.g. Clostridium tyrobutyricum and Enterococcus xiangfangensis toward directed toward E. harbinense). Moreover, negative interactions such as the one from C. pasteurianum toward C. amanolyticus might have decreased the abundance of the later. These interactions might have resulted in the high abundance of E. harbinense and E. olivae toward the end of the experiment. Importantly. Enterococcus species frequently coexist with Clostridium and Ethanoligenens bacteria (the main three groups at the end of our experiment) in several anaerobic environments such as dark fermentation bioreactors (Gabriel‐Barajas et al., 2022; Yang et al., 2022; Zhang et al., 2022) but their interactions have not been studied. Although statistical inference of microbial interactions has limitations and coexistence (or coexclusion) does not necessarily imply any type of interaction, it has been used successfully in different microbial systems to identify important interactions and community-level properties that result from these interactions (Guo et al., 2022; Lin, 2022). As such, our data and the previously mentioned reports indicate that such potential interactions should be extensively studied, especially, since lactic acid bacteria (like E. olivae) show metabolic capacities such as degradation of complex substrates and oxygen consumption might have a positive effect in hydrogen production (Sikora et al., 2013). Further, these interactions might be species or even strain specific (Goyal et al., 2022) and our experiment exemplifies this since we found that other enterococci (E. xiangfangensis) had a negative relationship with, both, E. harbinense and C. butyricum. Lastly, low-abundant bacteria (like C. nitrophenolicum and Rummeliibacillus pycnus) might have established positive interactions with hydrogen producers (like E. harbinense) that could favor the change in the overall composition. Low-abundance bacteria should not be overlooked since they are able to affect the whole structure of microbial communities either by acting as keystone species, being highly active or by serving as functional reservoirs against environmental perturbations (Pascoal et al., 2020; Jia et al., 2022).
In contrast, for heat-shock-treated bioreactors the only two ASVs led the consortia to reach stability faster than non-treated bioreactors. We hypothesize that the compositional and functional stability was the result of the two ASVs showing a mostly neutral interaction. We deduce this neutrality since the two ASVs coexisted without many changes in abundance during the whole experiment. Also, based on the interactions inferred for the non-treated bioreactors, no significant interaction was found between C. pasteurianum and C. guangxiense. This observation is consistent with previous studies in other communities, both natural and artificial, where several species of Clostridium can coexist (Graf et al., 2015; Thi Hoang et al., 2018). Since not every Clostridium (or for that matter any bacterial group) contributes equally to community-level function, our results draw attention to fully understanding the reasons behind the presence of certain species in microbial consortia. In the case of the heat-shock-treated bioreactors, the presence of C. pasteurianum and C. guangxiense might be the result of deterministic processes that increased their fitness like different spore germination times and growth rates that have been noted to be important in community assembly (Hawkes et al., 2002; Jaspers and Overmann, 2004; Menéndez-Serra et al., 2023). As mentioned before, the drastic reduction in ASVs in the heat-shock-treated bioreactors might give the impression of increased stability in the bioreactors. However, in addition to the fact that strict anaerobic conditions had to be used, the diminished diversity can lead to less long-term instability, due to, for example, a lack of functional redundancy in case of perturbations (Louca et al., 2018).
Invasion by competitive species in non-sterilized substrates is one of the reasons for the instability of the bioreactors (Castelló et al., 2020). Several factors have been shown to influence the resistance to invasions of microbial communities. For example, an increase in the richness component of diversity has been positively linked with a reduced probability of invasion while stochastic processes have been linked to invasion success (Mallon et al., 2015; Renault et al., 2022). In this sense, the function and structure of the microbial communities of the two treatments showed different responses to invasion (i.e. the effect on biogas production, the ratio or number of bioreactors where the invader established and the abundance of the invader) that could be related to specific community-level properties of each of them. In the non-treated bioreactors, only in one replicate, the invader became dominant while in the rest of the replicates no presence of the invader was observed after one week. In contrast, in the heat-shock-treated bioreactors, L. plantarum established in at least four replicates (although in low abundances) but never dominated. Similarly, biogas production was affected more severely in the heat-treated bioreactors, indicating that the alien species had a competitive effect on the microbial communities of such bioreactors. As such, in the heat-shock-treated bioreactors, given the small number of species, this community was susceptible of L. plantarum which might have managed to exploit an available niche which is an important factor regarding the establishment of invaders in low diversity communities (Kinnunen et al., 2016;Renault et al., 2022). In the non-treated bioreactors, since the establishment of L. plantarum occurred only once, it is possible that random processes like drift, which refers to random changes in microbial composition through cell division and death, contributed to invasion success in the particular bioreactor where it established. Although stochastic processes like drift are often overlooked, it has been noted earlier for influencing the assembly of microbial communities in bioreactors (Liebana et al., 2019) and other systems (Fodelianakis et al., 2021; Rose et al., 2023). Additionally, the strong effect of L. plantarum in the community might be explained by the similarity in fitness with the resident community, which might have increased the invasion effect (Li et al., 2019a). Thus, our results show that the relationship between microbial diversity, community function and invasion success is complex even in simple communities. As future research continues the test of dark fermentation on an industrial scale, the study of the effect of microbial diversity on the stability and function of bioreactors is going to be of extreme importance. Several challenges on scalability have been identified such as inhibitors accumulation, complex substrates pretreatment requirement and oxygen control (Teke et al., 2024) which can be diminished with the use of the correct management of microbial consortia. Also, further studies must be performed using complex substrates (like agro-industrial effluents) and varying conditions like those intended to be used in industrial-scale bioreactors. Strategies like bioaugmentation (e.g. inoculation of hydrogen strains into mixed cultures) or the use of current technologies like CRISPR are promising to improve the performance of the microbial consortia inhabiting bioreactors. Yet, these strategies still will require the use of ecological information to design strains and ensure their success during the transition from lab to industrial settings.
Despite a long line of evidence showing that diversity has a strong influence on the function and stability of bacterial communities, it is not clear how ecological mechanisms (from which function and stability are dependent) are related to diversity and how they are affected when diversity changes. The results in this work show that the establishment and medium-term behavior of bacterial communities are the outcomes of the interplay between the number and identity of ASVs, biotic interactions and culture conditions. In particular, we found that: (1) higher diversity slowed the stabilization of microbial abundance and dynamics but was ultimately predictable as biotic interactions were important for the assembly of microbial consortia, (2) loss of diversity made stabilization faster but less resilient against adverse culture conditions (aerobic conditions) and (3) higher diversity made invasion success less probable. Further studies investigating the molecular basis of interactions and confirmatory experiments on the nature of the interactions and their effect could help identify mechanisms that caused changes in function and invasion behaviors. Finally, due to the importance of microbial diversity for various applications, particularly in biotechnological settings, this study provides a baseline to incorporate ecological theory and experimental designs into the study of biotechnological settings like bioreactors. Further, this type of study might increase the knowledge of the relationship between function and diversity, which in turn will help improve the yield and stability of hydrogen-producing bioreactors.
Data availability statement
The datasets presented in this study can be found in online repositories. The names of the repository/repositories and accession number(s) can be found below: https://www.ncbi.nlm.nih.gov/genbank/, PRJNA814689.
Author contributions
MN-D: Conceptualization, Writing – review & editing, Data curation, Formal Analysis, Investigation, Methodology, Writing – original draft. VA-T: Writing – review & editing. IV-V: Writing – review & editing, Conceptualization, Funding acquisition, Supervision. JC-R: Conceptualization, Writing – review & editing. MA: Writing – review & editing, Project administration, Investigation. AE: Project administration, Writing – review & editing, Conceptualization, Funding acquisition, Supervision.
Funding
The author(s) declare financial support was received for the research, authorship, and/or publication of this article. MN-D was supported by Consejo Nacional de Ciencia y Tecnología (CONACyT), scholarship no. 594615. Financial support for this work was received from the “Fondo de Sustentabilidad Energética SENER-CONACYT (Mexico)”, Clúster de Bioalcoholes (Grant No. 249564).
Acknowledgments
We thank Marisol Pérez-Rangel for technical assistance during bioreactors establishment and for providing the strain Lactobacillus plantarum used in this work. The authors acknowledge Adalberto Noyola Robles and Margarita Cisneros Ortiz for their support in the laboratory work at the Laboratorio de Ingeniería Ambiental (LIA), Instituto de Ingeniería, UNAM. MN-D is a doctoral student from the Programa de Doctorado en Ciencias Biomédicas, Universidad Nacional Autónoma de México (UNAM).
Conflict of interest
The authors declare that the research was conducted in the absence of any commercial or financial relationships that could be construed as a potential conflict of interest.
The author(s) declared that they were an editorial board member of Frontiers, at the time of submission. This had no impact on the peer review process and the final decision.
Publisher’s note
All claims expressed in this article are solely those of the authors and do not necessarily represent those of their affiliated organizations, or those of the publisher, the editors and the reviewers. Any product that may be evaluated in this article, or claim that may be made by its manufacturer, is not guaranteed or endorsed by the publisher.
Supplementary material
The Supplementary Material for this article can be found online at: https://www.frontiersin.org/articles/10.3389/finmi.2024.1386726/full#supplementary-material
References
Altschu S. F., Gish W., Miller W., Myers E. W., Lipman D. J. (1990). Basic local alignment search tool. J. Mol. Biol. 215, 403–410. doi: 10.1016/S0022-2836(05)80360-2
Apprill A., Mcnally S., Parsons R., Weber L. (2015). Minor revision to V4 region SSU rRNA 806R gene primer greatly increases detection of SAR11 bacterioplankton. Aquat. Microbial Ecol. 75, 129–137. doi: 10.3354/ame01753
Bier R. L., Bernhardt E. S., Boot C. M., Graham E. B., Hall E. K., Lennon J. T., et al. (2015). Linking microbial community structure and microbial processes: an empirical and conceptual overview. FEMS Microbiol. Ecol. 91 (10), fiv113. doi: 10.1093/femsec/fiv113
Bolyen E., Rideout J. R., Dillon M. R., Bokulich N. A., Abnet C. C., Al-Ghalith G. A., et al. (2019). Reproducible, interactive, scalable and extensible microbiome data science using QIIME 2. Nat. Biotechnol. 37, 852–857. doi: 10.1038/s41587-019-0209-9
Boshagh F., Rostami K. (2020). A review of measurement methods of biological hydrogen. Int. J. Hydrogen Energy 45, 24424–24452. doi: 10.1016/j.ijhydene.2020.06.079
Braga J. K., Motteran F., Sakamoto I. K., Varesche M. B. A. (2018). Bacterial and archaeal community structure involved in biofuels production using hydrothermal- and enzymatic-pretreated sugarcane bagasse for an improvement in hydrogen and methane production. Sustain. Energy Fuels 2, 2644–2660. doi: 10.1039/C8SE00312B
Cabrol L., Marone A., Tapia-Venegas E., Steyer J. P., Ruiz-Filippi G., Trably E. (2017). Microbial ecology of fermentative hydrogen producing bioprocesses: useful insights for driving the ecosystem function. FEMS Microbiol. Rev. 41, 158–181. doi: 10.1093/femsre/fuw043
Cairns J., Jokela R., Hultman J., Tamminen M., Virta M., Hiltunen T. (2018). Construction and characterization of synthetic bacterial community for experimental ecology and evolution. Front. Genet. 9, 312. doi: 10.3389/fgene.2018.00312
Cao Q., Sun X., Rajesh K., Chalasani N., Gelow K., Katz B., et al. (2020). Effects of rare microbiome taxa filtering on statistical analysis. Front. Microbiol. 11, 607325. doi: 10.3389/fmicb.2020.607325
Castelló E., Nunes Ferraz-Junior A. D., Andreani C., Anzola-Rojas M. D. P., Borzacconi L., Buitrón G., et al. (2020). Stability problems in the hydrogen production by dark fermentation: Possible causes and solutions. Renewable Sustain. Energy Rev. 119, 109602. doi: 10.1016/j.rser.2019.109602
Ergal İ., Bochmann G., Fuchs W., Rittmann S. K.-M. R. (2022). Design and engineering of artificial microbial consortia for biohydrogen production. Curr. Opin. Biotechnol. 73, 74–80. doi: 10.1016/j.copbio.2021.07.010
Escalante A. E., Rebolleda-Gomez M., Benitez M., Travisano M. (2015). Ecological perspectives on synthetic biology: insights from microbial population biology. Front. Microbiol. 6, 143. doi: 10.3389/fmicb.2015.00143
Escalas A., Hale L., Voordeckers J. W., Yang Y., Firestone M. K., Alvarez-Cohen L., et al. (2019). Microbial functional diversity: From concepts to applications. Ecol. Evol. 9, 12000–12016. doi: 10.1002/ece3.5670
Estrela S., Vila J. C. C., Lu N., Bajić D., Rebolleda-Gómez M., Chang C., et al. (2022). Functional attractors in microbial community assembly. Cell Syst. 13, 29–42.e7. doi: 10.1016/j.cels.2021.09.011
Fodelianakis S., Valenzuela-Cuevas A., Barozzi A., Daffonchio D. (2021). Direct quantification of ecological drift at the population level in synthetic bacterial communities. ISME J. 15 (1), 55–66. doi: 10.1038/s41396-020-00754-4
Fuentes L., Palomo-Briones R., De Jesus Montoya-Rosales J., Braga L., Castello E., Vesga A., et al. (2021). Knowing the enemy: homoacetogens in hydrogen production reactors. Appl. Microbiol. Biotechnol. 105, 8989–9002. doi: 10.1007/s00253-021-11656-6
Gabriel-Barajas J. E., Arreola-Vargas J., Toledo-Cervantes A., Méndez-Acosta H. O., Rivera-González J. C., Snell-Castro R. (2022). Prokaryotic population dynamics and interactions in an AnSBBR using tequila vinasses as substrate in co-digestion with acid hydrolysates of Agave tequilana var. azul bagasse for hydrogen production. J Appl. Microbiol. 132 (1), 413–428. doi: 10.1111/jam.15196
Ghimire A., Frunzo L., Pirozzi F., Trably E., Escudie R., Lens P. N. L., et al. (2015). A review on dark fermentative biohydrogen production from organic biomass: Process parameters and use of by-products. Appl. Energy 144, 73–95. doi: 10.1016/j.apenergy.2015.01.045
Giri S., Shitut S., Kost C. (2020). Harnessing ecological and evolutionary principles to guide the design of microbial production consortia. Curr. Opin. Biotechnol. 62, 228–238. doi: 10.1016/j.copbio.2019.12.012
Goyal A., Bittleston L. S., Leventhal G. ,. E., Lu L., Cordero O. X. (2022). Interactions between strains govern the eco-evolutionary dynamics of microbial communities. eLife 11, e74987. doi: 10.7554/eLife.74987.sa2
Graf D., Di Cagno R., Fak F., Flint H. J., Nyman M., Saarela M., et al. (2015). Contribution of diet to the composition of the human gut microbiota. Microb. Ecol. Health Dis. 26, 26164. doi: 10.3402/mehd.v26.26164
Guo B., Zhang L., Sun H., Gao M., Yu N., Zhang Q., et al. (2022). Microbial co-occurrence network topological properties link with reactor parameters and reveal importance of low-abundance genera. NPJ Biofilms Microbiomes. 8 (1), 3. doi: 10.1038/s41522-021-00263-y
Hawkes F. R., Dinsdale R., Hawkes D. L., Hussy I. (2002). Sustainable fermentative hydrogen production: challenges for process optimisation. Int. J. Hydrogen Energy 27, 1339–1347. doi: 10.1016/S0360-3199(02)00090-3
Hernandez-Teran A., Navarro-Diaz M., Benitez M., Lira R., Wegier A., Escalante A. E. (2020). Host genotype explains rhizospheric microbial community composition: the case of wild cotton metapopulations (Gossypium hirsutum L.) in Mexico. FEMS Microbiol. Ecol. 96. doi: 10.1093/femsec/fiaa109
Jaspers E., Overmann J. (2004). Ecological significance of microdiversity: identical 16S rRNA gene sequences can be found in bacteria with highly divergent genomes and ecophysiologies. Appl. Environ. Microbiol. 70, 4831–4839. doi: 10.1128/AEM.70.8.4831-4839.2004
Jia X., Dini-Andreote F., Falcão Salles J. (2022). Unravelling the interplay of ecological processes structuring the bacterial rare biosphere. ISME Commun. 2, 96. doi: 10.1038/s43705-022-00177-6
Kannaiah Goud R., Sarkar O., Venkata Mohan S. (2014). Regulation of biohydrogen production by heat-shock pretreatment facilitates selective enrichment of Clostridium sp. Int. J. Hydrogen Energy 39, 7572–7586. doi: 10.1016/j.ijhydene.2013.10.046
Kim S.-H., Shin H.-S. (2008). Effects of base-pretreatment on continuous enriched culture for hydrogen production from food waste. Int. J. Hydrogen Energy 33, 5266–5274. doi: 10.1016/j.ijhydene.2008.05.010
Kinnunen M., Dechesne A., Proctor C., Hammes F., Johnson D., Quintela-Baluja M., et al. (2016). A conceptual framework for invasion in microbial communities. ISME J. 10, 2773–2775. doi: 10.1038/ismej.2016.75
Laxman Pachapur V., Jyoti Sarma S., Kaur Brar S., Le Bihan Y., Ricardo Soccol C., Buelna G., et al. (2015). Co-culture strategies for increased biohydrogen production. Int. J. Energy Res. 39, 1479–1504. doi: 10.1002/er.v39.11
Lee K., Park Y., Kuehn S. (2023). Robustness of microbiome function. Curr. Opin. Syst. Biol. 36, 100479. doi: 10.1016/j.coisb.2023.100479
Li S. P., Tan J., Yang X., Ma C., Jiang L. (2019a). Niche and fitness differences determine invasion success and impact in laboratory bacterial communities. ISME J. 13, 402–412. doi: 10.1038/s41396-018-0283-x
Li Y., Chen Y., Wu J. (2019b). Enhancement of methane production in anaerobic digestion process: A review. Appl. Energy 240, 120–137. doi: 10.1016/j.apenergy.2019.01.243
Li S., Müller S. (2023). Ecological forces dictate microbial community assembly processes in bioreactor systems. Curr. Opin. Biotechnol. 81, 102917. doi: 10.1016/j.copbio.2023.102917
Liebana R., Modin O., Persson F., Szabo E., Hermansson M., Wilen B. M. (2019). Combined deterministic and stochastic processes control microbial succession in replicate granular biofilm reactors. Environ. Sci. Technol. 53, 4912–4921. doi: 10.1021/acs.est.8b06669
Lin L. (2022). Bottom-up synthetic ecology study of microbial consortia to enhance lignocellulose bioconversion. Biotechnol Biofuels Bioprod. 15 (1), 14. doi: 10.1186/s13068-022-02113-1
Lipson D. A. (2015). The complex relationship between microbial growth rate and yield and its implications for ecosystem processes. Front. Microbiol. 6, 615. doi: 10.3389/fmicb.2015.00615
Lopez-Hidalgo A. M., Smoliński A., Sanchez A. (2022). A meta-analysis of research trends on hydrogen production via dark fermentation. Int. J. Energy Res. 47, 13300–13339. doi: 10.1016/j.ijhydene.2022.02.106
Louca S., Polz M. F., Mazel F., Albright M. B. N., Huber J. A., O'connor M. I., et al. (2018). Function and functional redundancy in microbial systems. Nat. Ecol. Evol. 2, 936–943. doi: 10.1038/s41559-018-0519-1
Lucena-Padros H., Gonzalez J. M., Caballero-Guerrero B., Ruiz-Barba J. L., Maldonado-Barragan A. (2014). Enterococcus olivae sp. nov., isolated from Spanish-style green-olive fermentations. Int. J. Syst. Evol. Microbiol. 64, 2534–2539. doi: 10.1099/ijs.0.062208-0
Mallon C. A., Elsas J. D. V., Salles J. F. (2015). Microbial invasions: the process, patterns, and mechanisms. Trends Microbiol. 23, 719–729. doi: 10.1016/j.tim.2015.07.013
Marcelino V., Welsh C., Diener C., Gulliver E. L., Rutten E., Young R. B., et al. (2023). Disease-specific loss of microbial cross-feeding interactions in the human gut. Nat. Commun. 14, 6546. doi: 10.1038/s41467-023-42112-w
Masset J., Calusinska M., Hamilton C., Hiligsmann S., Joris B., Wilmotte A., et al. (2012). Fermentative hydrogen production from glucose and starch using pure strains and artificial co-cultures of Clostridium spp. Biotechnol. Biofuels 5, 35. doi: 10.1186/1754-6834-5-35
Melkonian C., Zorrilla F., Kjærbølling I., Blasche S., MaChado D., Junge M., et al. (2023). Microbial interactions shape cheese flavour formation. Nat. Commun. 14, 8348. doi: 10.1038/s41467-023-41059-2
Menéndez-Serra M., Ontiveros V., Cáliz J., Alonso D., Casamayor E. O. (2023). Understanding stochastic and deterministic assembly processes in microbial communities along temporal, spatial and environmental scales. Mol. Ecol. 32, 1629–1638. doi: 10.1111/mec.16842
Michielsen S., Vercelli G., Cordero O. X., Bachmann H. (2024). Spatially structured microbial consortia and their role in food fermentations. Curr. Opin. Biotechnol. 87, 103102. doi: 10.1016/j.copbio.2024.103102
Mizrahi S., Goyal A., Gore J. (2023). Community interactions drive the evolution of antibiotic tolerance in bacteria. Proc. Natl. Acad. Sci. 120, e2209043119. doi: 10.1073/pnas.2209043119
Mizuno O. (2000). Enhancement of hydrogen production from glucose by nitrogen gassparging. Bioresource Technology. 73 (1), 59–65. doi: 10.1016/S0960-8524(99)00130-3
Mohanakrishna G., Pengadeth D. (2024). Mixed culture biotechnology and its versatility in dark fermentative hydrogen production. Bioresource Technol. 394, 130286. doi: 10.1016/j.biortech.2023.130286
Muñoz-Páez K. M., Alvarado-Michi E. L., Buitrón G., Valdez-Vazquez I. (2019). Distinct effects of furfural, hydroxymethylfurfural and its mixtures on dark fermentation hydrogen production and microbial structure of a mixed culture. Int. J. Hydrogen Energy 44, 2289–2297. doi: 10.1016/j.ijhydene.2018.04.139
Navarro-Díaz M., Martinez-Sanchez M. E., Valdez-Vazquez I., Escalante A. E. (2020). A framework for integrating functional and microbial data: The case of dark fermentation H2 production. Int. J. Hydrogen Energy 45, 31706–31718. doi: 10.1016/j.ijhydene.2020.08.189
Nemergut D. R., Shade A., Violle C. (2014). When, where and how does microbial community composition matter? Front. Microbiol. 5, 497. doi: 10.3389/fmicb.2014.00497
O’Leary N. A., Wright M. W., Brister J. R., Ciufo S., Haddad D., Mcveigh R., et al. (2016). Reference sequence (RefSeq) database at NCBI: current status, taxonomic expansion, and functional annotation. Nucleic Acids Res. 44, D733–D745. doi: 10.1093/nar/gkv1189
Oksanen J., Blanchet F., Kindt R., Legendre P. (2013). vegan: Community Ecology Package. R package version 2.0-10. Available at: http://cran.r-project.org.
Palomo-Briones R., Trably E., Lopez-Lozano N. E., Celis L. B., Mendez-Acosta H. O., Bernet N., et al. (2018). Hydrogen metabolic patterns driven by Clostridium-Streptococcus community shifts in a continuous stirred tank reactor. Appl. Microbiol. Biotechnol. 102, 2465–2475. doi: 10.1007/s00253-018-8737-7
Parada A. E., Needham D. M., Fuhrman J. A. (2016). Every base matters: assessing small subunit rRNA primers for marine microbiomes with mock communities, time series and global field samples. Environ. Microbiol. 18, 1403–1414. doi: 10.1111/1462-2920.13023
Pascoal F., Costa R., Magalhães C. (2020). The microbial rare biosphere: current concepts, methods and ecological principles. FEMS Microbiol. Ecol. 97, fiaa227. doi: 10.1093/femsec/fiaa227
Perez-Rangel M., Barboza-Corona J. E., Navarro-Diaz M., Escalante A. E., Valdez-Vazquez I. (2021). The duo Clostridium and Lactobacillus linked to hydrogen production from a lignocellulosic substrate. Water Sci. Technol. 83, 3033–3040. doi: 10.2166/wst.2021.186
Philippot L., Spor A., Henault C., Bru D., Bizouard F., Jones C. M., et al. (2013). Loss in microbial diversity affects nitrogen cycling in soil. ISME J. 7, 1609–1619. doi: 10.1038/ismej.2013.34
R Core Team. (2013). R: A language and environment for statistical computing. Available at: https://www.R-project.org/.
Renault D., Hess M. C. M., Braschi J., Cuthbert R. N., Sperandii M. G., Bazzichetto M., et al. (2022). Advancing biological invasion hypothesis testing using functional diversity indices. Sci Total Environ. 834, 155102. doi: 10.1016/j.scitotenv.2022.155102
Rose C., Lund M. B., Schramm A., Bilde T., Bechsgaard J. (2023). Does ecological drift explain variation in microbiome composition among groups in a social host species? J Evol Biol. 36 (12), 1684–1694. doi: 10.1111/jeb.14228
Sanchez A., Bajic D., Diaz-Colunga J., Skwara A., Vila J. C. C., Kuehn S. (2023). The community-function landscape of microbial consortia. Cell Syst. 14, 122–134. doi: 10.1016/j.cels.2022.12.011
Sarangi P. K., Nanda S. (2020). Biohydrogen production through dark fermentation. Chem. Eng. Technol. 43, 601–612. doi: 10.1002/ceat.201900452
Schmidt R., Cordovez V., De Boer W., Raaijmakers J., Garbeva P. (2015). Volatile affairs in microbial interactions. ISME J. 9, 2329–2335. doi: 10.1038/ismej.2015.42
Shade A. (2023). Microbiome rescue: directing resilience of environmental microbial communities. Curr. Opin. Microbiol. 72, 102263. doi: 10.1016/j.mib.2022.102263
Shade A., Peter H., Allison S. D., Baho D. L., Berga M., Burgmann H., et al. (2012). Fundamentals of microbial community resistance and resilience. Front. Microbiol. 3, 417. doi: 10.3389/fmicb.2012.00417
Shannon P., Markiel A., Ozier O., Baliga N. S., Wang J. T., Ramage D., et al. (2003). Cytoscape: a software environment for integrated models of biomolecular interaction networks. Genome Res. 13, 2498–2504. doi: 10.1101/gr.1239303
Shaw G. T., Pao Y. Y., Wang D. (2016). MetaMIS: a metagenomic microbial interaction simulator based on microbial community profiles. BMC Bioinf. 17, 488. doi: 10.1186/s12859-016-1359-0
Sikora A., Baszczyk M., Jurkowski M., Zielenkiewicz U. (2013). “Lactic acid bacteria in hydrogen-producing consortia: on purpose or by coincidence?,” in Lactic acid bacteria – R & D for food, health and livestock purposes. InTech. doi: 10.5772/50364
Singh L., Wahid Z. A. (2015). Methods for enhancing bio-hydrogen production from biological process: A review. J. Ind. Eng. Chem. 21, 70–80. doi: 10.1016/j.jiec.2014.05.035
Srivastava N., Srivastava M., Kushwaha D., Gupta V. K., Manikanta A., Ramteke P. W., et al. (2017). Efficient dark fermentative hydrogen production from enzyme hydrolyzed rice straw by Clostridium pasteurianum (MTCC116). Bioresour Technol. 238, 552–558. doi: 10.1016/j.biortech.2017.04.077
Stenuit B., Agathos S. N. (2015). Deciphering microbial community robustness through synthetic ecology and molecular systems synecology. Curr. Opin. Biotechnol. 33, 305–317. doi: 10.1016/j.copbio.2015.03.012
Sun X., Sanchez A. (2023). Synthesizing microbial biodiversity. Curr. Opin. Microbiol. 75, 102348. doi: 10.1016/j.mib.2023.102348
Tenca A., Schievano A., Perazzolo F., Adani F., Oberti R. (2011). Biohydrogen from thermophilic co-fermentation of swine manure with fruit and vegetable waste: maximizing stable production without pH control. Bioresour Technol. 102, 8582–8588. doi: 10.1016/j.biortech.2011.03.102
Teke G. M., Anye Cho B., Bosman C. E., Mapholi Z., Zhang D., Pott R. W. M. (2024). Towards industrial biological hydrogen production: a review. World J. Microbiol. Biotechnol. 40, 37. doi: 10.1007/s11274-023-03845-4
Thi Hoang V., Huong Hoang D., Duc Pham N., My Tran H., Thi Viet Bui H., Anh Ngo T. (2018). Hydrogen production by newly isolated Clostridium species from cow rumen in pure- and co-cultures on a broad range of carbon sources. AIMS Energy 6, 846–865. doi: 10.3934/energy.2018.5.846
Thulasisingh A., Ananthakrishnan K., Nandakumar J., Kannaiyan S. (2023). Fermentative biohydrogen fuel production utilizing wastewater: A review. CLEAN - Soil Air Water 51, 2300112. doi: 10.1002/clen.202300112
Valdez-Vazquez I., Poggi-Varaldo H. M. (2009). Hydrogen production by fermentative consortia. Renewable Sustain. Energy Rev. 13, 1000–1013. doi: 10.1016/j.rser.2008.03.003
van Leeuwen P., Brul S., Zhang J., Wortel M. T. (2023). Synthetic microbial communities (SynComs) of the human gut: design, assembly, and applications. FEMS Microbiol. Rev. 47, fuad012. doi: 10.1093/femsre/fuad012
Villanueva-Galindo E., Vital-Jácome M., Moreno-Andrade I. (2023). Dark fermentation for H2 production from food waste and novel strategies for its enhancement. Int. J. Hydrogen Energy. 48, 9957–9970. doi: 10.1016/j.ijhydene.2022.11.339
Wagg C., Bender S. F., Widmer F., van der Heijden M. G. (2014). Soil biodiversity and soil community composition determine ecosystem multifunctionality. Proc. Natl. Acad. Sci. U.S.A. 111, 5266–5270. doi: 10.1073/pnas.1320054111
Wang A., Gao L., Ren N., Xu J., Liu C. (2009). Bio-hydrogen production from cellulose by sequential co-culture of cellulosic hydrogen bacteria of Enterococcus gallinarum G1 and Ethanoigenens harbinense B49. Biotechnol. Lett. 31, 1321–1326. doi: 10.1007/s10529-009-0028-z
Wang J., Pan Z., Yu J., Zhang Z., Li Y. (2023). Global assembly of microbial communities. mSystems 8, e01289–e01222. doi: 10.1128/msystems.01289-22
Wang J., Yin Y. (2017). Principle and application of different pretreatment methods for enriching hydrogen-producing bacteria from mixed cultures. Int. J. Hydrogen Energy 42, 4804–4823. doi: 10.1016/j.ijhydene.2017.01.135
Wang S., Zhang T., Bao M., Su H., Xu P. (2020). Microbial production of hydrogen by mixed culture technologies: A review. Biotechnol. J. 15, e1900297. doi: 10.1002/biot.201900297
Yang G., Wang J. (2019). Changes in microbial community structure during dark fermentative hydrogen production. Int. J. Hydrogen Energy 44, 25542–25550. doi: 10.1016/j.ijhydene.2019.08.039
Yang M., Watson J., Wang Z., Si B., Jiang W., Zhou Z., et al. (2022). “Understanding and design of two-stage fermentation: A perspective of interspecies electron transfer,” In Renewable and Sustainable Energy Reviews. Elsevier, vol. 168(C).
Yuan H., Mei R., Liao J., Liu W. T. (2019). Nexus of stochastic and deterministic processes on microbial community assembly in biological systems. Front. Microbiol. 10, 1536. doi: 10.3389/fmicb.2019.01536
Keywords: dark fermentation, microbial interactions, microbial ecology, diversity and function relationship, ecological network
Citation: Navarro-Díaz M, Aparicio-Trejo V, Valdez-Vazquez I, Carrillo-Reyes J, Avitia M and Escalante AE (2024) Levels of microbial diversity affect the stability and function of dark fermentation bioreactors. Front. Ind. Microbiol. 2:1386726. doi: 10.3389/finmi.2024.1386726
Received: 15 February 2024; Accepted: 09 April 2024;
Published: 25 April 2024.
Edited by:
Simona Rossetti, National Research Council (CNR), ItalyReviewed by:
Yogesh K. Ahlawat, University of Florida, United StatesKusum Dhakar, University of Thessaly, Greece
Copyright © 2024 Navarro-Díaz, Aparicio-Trejo, Valdez-Vazquez, Carrillo-Reyes, Avitia and Escalante. This is an open-access article distributed under the terms of the Creative Commons Attribution License (CC BY). The use, distribution or reproduction in other forums is permitted, provided the original author(s) and the copyright owner(s) are credited and that the original publication in this journal is cited, in accordance with accepted academic practice. No use, distribution or reproduction is permitted which does not comply with these terms.
*Correspondence: Ana E. Escalante, YWVzY2FsYW50ZUBpZWNvbG9naWEudW5hbS5teA==; Marcelo Navarro-Díaz, bWFyY2Vsby5uLmRAb3V0bG9vay5jb20=