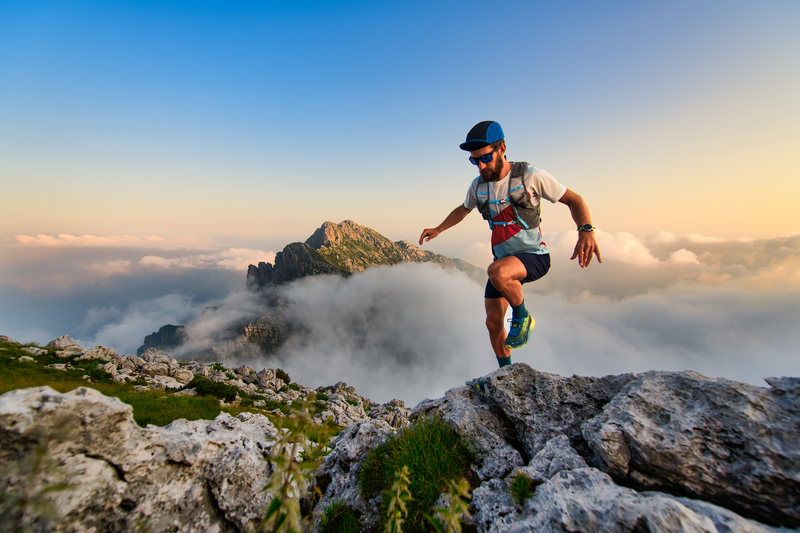
94% of researchers rate our articles as excellent or good
Learn more about the work of our research integrity team to safeguard the quality of each article we publish.
Find out more
PERSPECTIVE article
Front. Ind. Microbiol. , 26 September 2023
Sec. Food
Volume 1 - 2023 | https://doi.org/10.3389/finmi.2023.1255505
Artificial Intelligence (AI) is the creation of intelligent systems able to execute specific tasks which would normally need human intelligence to function. Today, AI is widely used to assist research and development in industrial biotechnology. Many biotech firms rely on AI tools. The food industry is among the industrial sectors where AI has only recently been explored. AI enhances food biotechnology by supporting food enzymes engineering, microbial metabolic engineering, food safety and, in general, food microbiology. The use of AI tools in the food industry ranges from food processing – including all aspects of the production of food ingredients – to food quality and safety. This paper highlights the emerging importance of AI in some specific sectors of food biotechnology: development of new process-aid enzymes, precision fermentation, food safety and toxicity, food microbiology, and the study of the interplay between food and gut microbiome. These represent only a few examples of the myriad of applications that AI is seeing and will see in the food biotech sector. This Perspective paper intends to stimulate the discussion around the intricate relationship between AI and such research topics.
Artificial Intelligence (AI) is an emerging technology that may eventually dominate all aspects of human life. Google, a prolific user of AI, describes this technology as the “ability of systems to synthesize information as the manifestation of intelligence, similar to the way it is defined in biological intelligence”.1 One primary theme within AI is machine learning. Machine learning (ML) combines probability, statistics, and convex optimization to better solve problems related to speech recognition, robot control, and computer vision (Jordan and Mitchell, 2015). Some of the algorithms within AI include reinforcement learning, expert systems (ES), Turing test, artificial neural networks (ANN), and logic programming (Borana and Jodhpur, 2016).
AI is becoming a crucial tool in the field of food biotechnology. Mavani et al. (2022) recently described the application of the different types of AI within the food industry. AI gives strong support to the development of new capabilities, products, and commodities, while reducing processing time and increasing accuracy. Moreover, AI has played a key role for shaping new approaches in the research and development of enzymes for industrial applications, including food enzymes. An outstanding example this application of AI is the development of AlphaFold, a computational tool that can predict the structure of proteins. AlphaFold has been used to determine the structure of over 200 million proteins to date (Jumper et al., 2021).
For the application to enzyme development problems, the role of AI is not confined to the study of protein structure–function relationships. In fact, AI can support each phase of the development of a new enzyme from its discovery by the manipulation of big datasets, for example those generated using metagenomics or classical single enzyme mutational biochemistry. Also important is to understand how variations in enzyme structure impact its application in a specific process of interest: it is crucial to mimic the complex food processing environments where its performance will be ultimately judged.
AI technology is especially important to the development of precision fermentation – an emerging field in its own right – by driving the genetic manipulation of “cell factories” (i.e., mainly yeast and fungi) towards the production of custom molecules, including proteins.
Food safety and compliance are key in the food industry and AI tools greatly support the continuous improvement of these standards, paving the way to the development of rapid and cost-effective methods to determine toxic compounds in food.
The interplay between food and gut microbiome is under continuous investigation. AI holds promise in the development of precision nutrition, the branch of science that evaluates individual lifestyles and creates tailor-made nutritional programs that in turn lead to better quality of life.
AI has proven to be a powerful tool for the study of enzyme structure/function relationships. It has driven computational modeling and mutagenesis R&D that has resulted in improved enzymes for food processing.
The application of food enzymes normally occurs in complex environment. AI is envisioned to be able to support the simulation of the most complex reactions performed by process-aid enzymes in food processing. This is a great advantage in comparison to the classic tools used to improve these enzymes, which take under consideration only a limited number of parameters and certainly do not consider the real environment in which the food processing occurs.
Wang J. et al. (2023) have recently reviewed how the AI-assisted design and engineering of enzymes could be beneficial to food processing enzymes. One of the main advantages of using AI in food enzyme engineering is the significant saving in computational time. Much reduced time and resources are used in comparison to the traditional physical methods, while potentially delivering wide sets of information that support the development of new products.
Precision fermentation represents a new trend in the food industry. It is based on the use of microbes – mainly yeasts and fungi – to produce a specific molecule in a scalable process. Protein-based ingredients represent the most common product of precision fermentation in food industry. Precision fermentation is the next step in the evolution of classical submerged fermentation and it is now possible thanks to advances in microbe manipulation and metabolic engineering (Teng et al., 2021) to create synthetic cells factories. Genetic modification of the workhorse microbes so far used in precision fermentation and envisioned to be key players used for this technology relies on the use of traditional metabolic engineering tools for strain improvement. AI-based approaches have attracted considerable attention lately in the industrial microbiology field. AI tools, in fact, favor a much more rapid understanding of the best edits to apply to the microbial genome to improve the yield of a molecule of interest. AI tools help editing and customize microbes for the production of a specific molecules requested in food industry, while also guiding the storage and manipulation of big data sets generated from the integration of experimental and in silico experiments. Today, many food companies are focusing on this new technology to accommodate the ever widening array of food requests.
Food safety is crucial to human survival and health. AI is being used in the areas of food supply chain management, quality control, food sorting, and food hygiene. Food fraud was predicted by a Bayesian network model (MedISyss-FF) developed by the European Union’s Rapid Alert System for Food and Feed (Bouzembrak and Marvin, 2016) whereas the U.S. FDA (Food and Drug Administration) has used AI to develop a model for predicting aflatoxin to help identify low- and high-risk seafood sourcing (FDA, 2022). Similarly, a study conducted in Taiwan by Chang et al. (2020) describes the development of an automated alarm system for food safety.
Microbial toxins and toxic chemicals are certainly the major factor contributing to food safety risks. The methodologies currently used to detect such risks are costly and time-consuming. The integration of AI and machine learning software to the conventional methods used for assessing food toxicity has led to big improvement in terms of rapidity and cost-effectiveness of food analyses to detect toxic compounds of both chemical and biological origins. The management of large dataset enabled by AI tools allows to detect and classify toxic compounds in a rapid and easy manner. In this contest, a risk of food toxicity can be represented also by the chemical migration from package to food. Wang S. S. et al. (2023) have recently developed an AI-based tool that can prevent such issues by utilizing a mix of data related to the chemical properties, material type, food type and temperature. A survey conducted by Zhou et al. (2019) has clearly shown that deep learning is the right approach to outperform the analysis that supports food quality and safety. The rapidity of AI-based tools allow in fact to investigate the presence of toxic compounds at all critical points of food manufacturing.
Developing AI-based food sorting and packaging is often challenging due to variety of shapes and sizes of products. However, when established, AI can aid in decision making and automation, resulting in rapid, efficient, and hygienic practices, amongst other benefits. TOMRA and TensorFlow are two popular AI tools that use a combination of high-resolution cameras, laser technology systems, X-ray systems, and IR spectroscopy. Such tools are thought to have increased productivity in product sorting by ~90% (Kumar et al., 2021).
Alongside raw materials and food processing, food industry places paramount importance on equipment sanitation and maintenance. Simeone et al. (2020) carried out a study to assess the use of optical and ultrasonic sensors to monitor the removal of food materials fouling a benchtop rig and were able to demonstrate cleaning accuracies as high as 98% using these technologies to monitor cleaning progress. Work done by Friedlander and Zoellner (2020) describe the interesting applications of AI in monitoring food safety at retail outlets.
These kinds of tools will play an important role in the monitoring of food facilities for food safety and future development in this technology will concentrate on developing algorithms to aid real-time determination of high-risk raw material sources (Qian et al., 2023).
AI and machine learning are finding important applications in the area of food pathogen microbiology. Foodborne microbial pathogens can cause food poisoning or food spoilage. In conventional laboratories, food items would be screened for pathogens by isolating contaminants on agar plates followed by biochemical analysis. These methods are slow and are often restricted by the inability to isolate the microorganisms on culture media available in common labs. AI applications can reduce the time for detection and increase sensitivity considerably. Lupolova et al. (2017) showed that through AI based tools, it will be possible to determine host specificity and zoonotic potential of species like Salmonella enterica and Escherichia coli, this holding tremendous importance from a public health perspective.
Using an AI-based technology platform, Wang et al. (2020) were able to detect bacterial growth in less than 3 hours and correctly classify ~80% of bacterial types within ~8 hours, saving more than 12 hours when compared to conventional methods.
AI is helping to implement technologies and instruments which are used in food safety for the rapid and accurate detection of adulterations and microbial contaminants in food, such as hyperspectral imaging (HSI) – a combination of conventional imaging and spectroscopy (Gowen et al., 2007), and novel electronic devices, such as electronic noses (ENs) which are automated odor or microbial growth detectors that combine chemical sensor arrays with a pattern recognition system (Gobbi et al., 2015).
Precision nutrition “integrates genetic, metagenomic, metabolomic, physiopathological, behavioral and sociocultural cues to understand metabolism and human wellbeing and implement health actions” (Livingstone et al., 2022). As such this represents an emerging topic in the food biotechnology realm. Gut microorganisms and dietary components have an inter-dependent relationship. Gut microbes transform food components to produce metabolites that influence and shape the host immune and metabolic responses whereas dietary components influence the type and functional character of the gut microbes. These interactions tend to be very complex and very specific to an individual. AI represents a powerful tool to study large datasets and to carry out comprehensive analyses to develop dietary strategies, with the ultimate goals of combatting disease onset and progression (Danneskiold-Samsøe et al., 2019).
AI is being used increasingly to study gut microbiota and use the information to formulate diagnostic and therapeutic solutions of crucial diseases. In fact, AI algorithms are suited to make connections between nutrition, health and dietary behaviors (Cote and Lamarche, 2021). Microbiome analyses generate large amounts of data sets and AI algorithms allows to break down data into tools that can be used by everyday clinicians to aid diagnosis or prognosis. Whereas traditional methods of data dietary collection often led to errors, machine learning can be used to reduce these error margins and improve the accuracy and authenticity of the data (Morgenstern et al., 2021) despite some limitations on understanding how these predictions would translate into everyday life performance (Cammarota et al., 2020).
This paper highlights a few of the most critical possible applications of AI in food biotechnology. It is clear that AI is shaping new ways of using science for supporting the development of food products and services. This translates to both the rapid advancement of traditional methodologies – such as those related to industrial enzymes development, data management, microbiology – and to the shaping of new advanced technologies, such as precision fermentation.
This innovative way to look at food development, food processing, and food safety interplay with the next frontier in nutrition science such as developing custom-made diets and precision nutrition plans. AI will play a key role in this process as already demonstrated by several studies in progress which are using powerful Al tools to collect large datasets from millions of individuals, interpret them, and include them in biological nutrition studies (Sak and Suchodolska, 2020).
Even though AI has many advantages and holds great promise for food industries, much more work needs to be done for routine application to the various aspects of the industry. And AI cannot work completely without human intervention. Barriers include a lack of data in digital format, concerns about privacy – due to the need to store food safety data on public platforms – and even a reluctance to adopt automated systems in the sector food biotechnology.
Businesses need to be encouraged to focus on research that builds AI applications that are custom made and address specific needs. In fact, the use of AI in food biotechnology paves the way to developing applications focused on specific needs and questions of the food industry.
The original contributions presented in the study are included in the article. Further inquiries can be directed to the corresponding author.
AA: Writing – original draft, Writing – review & editing. SP: Writing – original draft, Writing – review & editing.
Authors AA and SP are employed by the company Biocatalysts Ltd.
The authors declare that the research was conducted in the absence of any commercial or financial relationships that could be construed as a potential conflict of interest.
Author AA was an editorial board member of Frontiers, at the time of submission. This had no impact on the peer review process and the final decision.
All claims expressed in this article are solely those of the authors and do not necessarily represent those of their affiliated organizations, or those of the publisher, the editors and the reviewers. Any product that may be evaluated in this article, or claim that may be made by its manufacturer, is not guaranteed or endorsed by the publisher.
Borana J., Jodhpur N. U. (2016). “Applications of artificial intelligence & associated technologies,” in Proceeding of International Conference on Emerging Technologies in Engineering, Biomedical, Management and Science, ETEBMS-2016, March, 5–6.
Bouzembrak Y., Marvin H. J. P. (2016). Prediction of food fraud type using data from Rapid Alert System for Food and Feed (RASFF) and Bayesian network modelling. Food Control 61, 180–187. doi: 10.1016/j.foodcont.2015.09.026
Cammarota G., Ianiro G., Ahern A., Carbone C., Temko A., Claesson M., et al. (2020). Gut microbiome, big data and machine learning to promote precision medicine for cancer. Nat. Rev. Gastroenterol. Hepatol. 17 (10), 635–648. doi: 10.1038/s41575-020-0327-3
Chang W. T., Yeh Y. P., Wu H. Y., Lin Y. F., Dinh T. S., Lian I. B. (2020). An automated alarm system for food safety by using electronic invoices. PloS One 24, 15(1). doi: 10.1371/journal.pone.0228035
Cote M., Lamarche B. (2021). Artificial intelligence in nutrition research: Perspectives on current and future applications. Appl. Physiol. Nutr. Metabol. 1(15), 1–8. doi: 10.1139/apnm-2021-0448
Danneskiold-Samsøe N. B., de Freitas Queiroz Barros H. D., Santos R., Bicas J. L., Cazarin C. B. B., Madsen L., et al. (2019). Interplay between food and gut microbiota in health and disease. Food Res. Int. 115, 23–31. doi: 10.1016/j.foodres.2018.07.043
FDA. (2022). Import screening pilot unleashes the power of data and leverages artificial intelligence. US Food and Drug Administration. Available at: https://www.fda.gov/news-events/fda-voices/import-screening-pilot-unleashes-power-data-and-leverages-artificial-intelligence (Accessed 22 April, 2023).
Friedlander A., Zoellner C. (2020). Artificial intelligence opportunities to improve food safety at retail. Food Prot. Trends 40 (4), 272–278.
Gobbi E., Falasconi M., Zambotti G., Sberveglieri V., Pulvirenti A., Sberveglieri G. (2015). Rapid diagnosis of Enterobacteriaceae in vegetable soups by a metal oxide sensor based electronic nose. Sensors Actuators B.: Chem. 207 (B), 1104–1113. doi: 10.1016/j.snb.2014.10.051
Gowen A., O'Donnell C. P., Cullen P. J., Downey G., Frias J. M. (2007). Hyperspectral imaging – an emerging process analytical tool for food quality and safety control. Trends Food Sci. Tech. 18 (12), 590–598. doi: 10.1016/j.tifs.2007.06.001
Jordan M. I., Mitchell T. M. (2015). Machine learning: Trends, perspectives, and prospects. Science. 349(6245), 255–60. doi: 10.1126/science.aaa8415
Jumper J., Evans R., Protzel A., Green T., Figurnov M., Ronnebrger O., et al. (2021). Highly accurate protein structure prediction with AlphaFold. Nature 596, 583–589. doi: 10.1038/s41586-021-03819-2
Kumar I., Rawat J., Mohd N., Husain S. (2021). Opportunities of artificial intelligence and machine learning in the food industry. J. Food Qual. doi: 10.1155/2021/4535567
Livingstone K. M., Ramos-Lopez O., Pérusse L., Kato H., Ordovas J. M., do Martínez J. A. (2022). Precision nutrition: A review of current approaches and future endeavors. Trends Food Sci. Technol. 128(10184). doi: 10.1016/j.tifs.2022.08.017
Lupolova N., Dallman T. J., Holden N. J., Gally D. L. (2017). Patchy promiscuity: machine learning applied to predict the host specificity of Salmonella enterica and Escherichia coli. Microb. Genom. doi: 10.1099/mgen.0.000135
Mavani N. R., Ali J. M., Othman S., Hussain M. A., Hashim H., Rahman N. A. (2022). Application of artificial intelligence in food industry. Food Eng. Rev. 14 (1), 134–175. doi: 10.1007/s12393-021-09290-z
Morgenstern J. D., Rosella L. C., Costa A. P., de Souza R. J., Anderson L. N. (2021). Perspective: Big data and machine learning could help advance nutritional epidemiology. Adv. Nutr. 12 (3), 621–631. doi: 10.1093/advances/nmaa183
Qian C., Murphy S. I., Orsi R. H., Wiedmann M. (2023). How can AI help improve food safety? Ann. Rev. Food Sci. Tech. 14, 517–538. doi: 10.1146/annurev-food-060721-013815
Sak J., Suchodolska M. (2020). Artificial intelligence in nutrients science research: A review. Nutrients 13 (2), 322. doi: 10.3390/nu13020322
Simeone A., Woolley E., Escrig J., Watson N. J. (2020). Intelligent industrial cleaning: A multi-sensor approach utilising machine learning-based regression. Sensors 20 (13), 3642. doi: 10.3390/s20133642
Teng T. S., Chin Y. L., Chai F. K., Chen W. N. (2021). Fermentation for future food systems. EMBO Rep. 22(5), e52680. doi: 10.15252/embr.202152680
Wang H., Ceylan K. H., Qiu Y., Bai B., Zhang Y., Jin Y., et al. (2020). Early detection and classification of live bacteria using time-lapse coherent imaging and deep learning. Light Sci. Appl. 9, 118. doi: 10.1038/s41377-020-00358-9
Wang S. S., Lin P., Wang C. C., Lin Y. C., Tung C. W. (2023). Machine learning for predicting chemical migration from food packaging materials to foods. Food Chem. Toxicol. doi: 10.1016/j.fct.2023.113942
Wang J., Yang P., Zhao B., Liu S. (2023). AI-assisted food enzymes design and engineering: A critical review. Syst. Microb. BiOmanuf. 3, 75–87. doi: 10.1007/s43393-022-00138-z
Keywords: artificial intelligence, food safety, food enzymes, precision fermentation, food microbiology, food biotechnology
Citation: Amore A and Philip S (2023) Artificial intelligence in food biotechnology: trends and perspectives. Front. Ind. Microbiol. 1:1255505. doi: 10.3389/finmi.2023.1255505
Received: 08 July 2023; Accepted: 22 August 2023;
Published: 26 September 2023.
Edited by:
Rajeev Kumar Sukumaran, National Institute for Interdisciplinary Science and Technology (CSIR), IndiaReviewed by:
Branko Velebit, Institute of Meat Hygiene and Technology, SerbiaCopyright © 2023 Amore and Philip. This is an open-access article distributed under the terms of the Creative Commons Attribution License (CC BY). The use, distribution or reproduction in other forums is permitted, provided the original author(s) and the copyright owner(s) are credited and that the original publication in this journal is cited, in accordance with accepted academic practice. No use, distribution or reproduction is permitted which does not comply with these terms.
*Correspondence: Antonella Amore, bGlsbHkuYW1vcmU4NkBnbWFpbC5jb20=
Disclaimer: All claims expressed in this article are solely those of the authors and do not necessarily represent those of their affiliated organizations, or those of the publisher, the editors and the reviewers. Any product that may be evaluated in this article or claim that may be made by its manufacturer is not guaranteed or endorsed by the publisher.
Research integrity at Frontiers
Learn more about the work of our research integrity team to safeguard the quality of each article we publish.