- Darmstadt University of Applied Sciences, Darmstadt, Germany
This study investigates the diffusion of AI-based service applications within the business models of German manufacturing industries, surveying 162 decision-makers. The integration of AI into business model is assessed through the Business Model Canvas (BMC) framework, evaluating its value in terms of effectiveness as well as efficiency. Rather than focusing on specific use cases, the study delves into the intended usage of value-driven AI services references to enhance effectiveness and efficiency across various elements of the business models. Through this research, eleven service values have been identified. Each service vale corresponds to a distinct element of the BMC. Decision-makers were surveyed using a Confirmation/Disconfirmation (C/D) paradigm to measure the disparities between their current and target performance levels. Consequently, this study provides valuable insights from the perspective of decision makers regarding the current and desired state of AI integration in the German manufacturing industry, taking into account AI usage or no AI usage at the time of data collection.
1 Introduction
The integration and diffusion of AI continue to rising up. Particularly noticeable since the launch of ChatGPT renewed enthusiasm for AI-based applications across various sectors, including Customer-to-Customer (C2C) as well as Business-to-Business (B2B) interactions (von Garrel and Mayer, 2023). Since the 1950s, AI has been a familiar concept but recent advances in model approaches and computing power have significantly bolstered its market readiness. This was evident in the milestones achieved within generative AI in the 2010s (Krizhevsky et al., 2012) and in how businesses began using it as a new tool to redesign various elements of their business models.
Examples therefor are the creation of customized value propositions through the analysis of customer data, thereby adapting offerings to meet individual needs with options like “pay-per-use” or “pay-as-you-go” (Kett et al., 2021; Kahle and Helldorff, 2021). Furthermore, chatbots can be deployed to personalize and automate interactions, ensuring round-the-clock customer service without delays (Schmidt et al., 2021). Additionally, supply chains can be optimized by leveraging AI to analyze supply-chain-data, identifying bottlenecks, exploring new partnerships, optimizing transportation routes, and ultimately enhancing overall efficiency (Neuhüttler et al., 2021; Valentowitsch, 2021). These instances illustrate how AI can profoundly impact and reshape the three pillars of value creation: Value Proposition, Value Chain, and Customer Relationship.
Despite this surge, the manufacturing businesses in Germany still exhibit significant untapped potential (Büchel et al., 2021; von Garrel and Thomas, 2023). However, constraints such as limited capital, technical expertise, and training opportunities for employees impede the widespread adoption and diffusion of AI within the manufacturing sector, particularly with Small and Medium-sized Enterprises (SMEs) (Büchel et al., 2021; von Garrel and Thomas, 2023). Nonetheless, the utilization of AI technologies within German industries is steadily increase. More business organizations are recognizing the potential of AI to enhance their business models (Merkel-Kiss and von Garrel, 2022; von Garrel and Thomas, 2023). It has the capacity to optimize and innovate internal and external operations like enhancing quality, increasing efficiency, and boosting effectiveness. Moreover, AI facilitates the repositioning of value propositions and lead to innovation from traditional products through the addition of supplementary (Smart-) Services (von Garrel and Jahn, 2023).
Against this background, this research delves the use of AI in relation to its value and illustrates the diffusion (current status) and desired integration (target status) of AI-based applications across various elements of manufacturing business models in Germany.
2 Theoretical foundations
Business models (BM) serve as aggregated and complexity-reducing representations of an organization’s value creation logic for a specific target audience, and illustrate how the generated value is transformed in to revenues (Kett et al., 2021). Among other functions, this framework facilitates a critical assessment of the current business operations, revealing strengths, weaknesses, opportunities and threats. Additionally, business models serve to analyze, plan, design and communicate, planning, design and communication and enable the identification of necessary adjustments or further developments (Bruhn and Hadwich, 2021; Kett et al., 2021).
In the literature, several forms of visual representations for business models are documented. Widely used in practice is the Business Model Canvas (BMC) as introduced by Osterwalder and Pigneur (2011) depicted in Figure 1. A significant advantage of the BMC lies in its primary focus on the three pillars of value creation and illustrates the added value (key activities, key resources, key partners), customers (customer relationships, customer segments and channels), and finances (cost structure and revenue streams) in relation to the value proposition (Osterwalder and Pigneur, 2011). Definitions and explanations of the individual elements of the BMC are available in the original literature (Osterwalder and Pigneur, 2011).
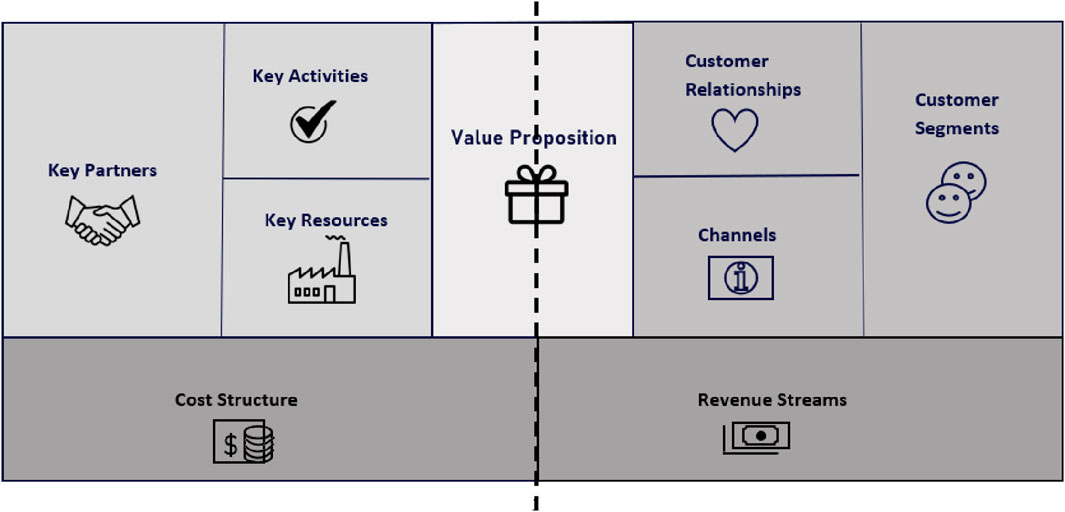
Figure 1. Own design according to Osterwalder and Pigneur (2011).
As Figure 1 illustrates, finances serve as the foundation of the Business Model Canvas (BMC). They profitability is primarily defined by the value proposition in relation to the value chain and the customer. If the graphic would be divided in the middle, two blocks emerge. The left block of value propositions and the associated value chain determine the cost structure. Above all, the use of resources of any kind (material, time, etc.) and the associated activities comprise the financial expenses. The literature also confirms that the use of resources has a significant influence on the cost structure and an efficient use leads to a reduction in costs (Gläß, 2018; Fresner, 2010; Incekara, 2022; Odesola and Daramola, 2010). The block on the right side of value proposition, customers and revenue streams describes where the revenue comes from and how it flows into the BM. It ultimately describes how effectively a business model is able to generate revenue and how effective is performance or the value on the customer side (Ney, 2006). It therefore highlights the business elements that significantly influence the effectiveness (right-hand side) and efficiency (left-hand side) of value creation (Osterwalder and Pigneur, 2010, S. 49).
AI-based business models stand apart from traditional or digital business models in that AI technology is crucial for at least one element, indicating that AI plays a pivotal role in defining the value creation process and shaping the revenue structure (Moll and Lerch, 2021). It fosters adaptability and intelligent interactions with a broad range of stakeholders, enhancing flexibility and agility in the dynamic environments (Bruhn and Hadwich, 2021; Winter, 2021). For instance, traditional value propositions can evolve into adaptive ( = effective) and autonomous ( = efficient) services (Kett et al., 2021). In order to achieve a sustainable increase in performance and further establishment on markets, business models should therefore further develop or change the underlying functional logic of value creation in the long term (Kett et al., 2021; Bruhn and Hadwich, 2021).
AI fundamentally offers the potential to enhance efficiency as well as effectiveness by data utilization. Consequently, information in the form of data and its availability are critical in transforming value creation processes. Data makes it possible to uncover previously unseen patterns and structures (Hu et al., 2022). Essentially, every incident is rooted in cause-effect relationships, which can be captured and represented as digital information (data). In this context, it is crucial to acknowledge the value of data ( = information) and to be aware that business models can be unique. The transformation and integration of AI into business processes depend on the industry, the specific value proposition, the value requirements (from customers and the market) as well as the availability of data within this framework. Based on this principle, models can be adjusted to specific use cases and developed for unique conditions, enabling the analysis of occurrences and supporting predictions, decisions and actions (Rohde et al., 2022).
Following this understanding of the BMC and the idea of the principles of effectiveness and efficiency in relation to the individual availability and usability of data, the study deals with the question:
How does prioritizing the elements of the Business Model Canvas (BMC) based on effective and efficient value-driven data usage influence the transformation of AI-based service business models in the German manufacturing industry?
3 Methodical implementation
The methodology focuses on operationalizing the measurement of distribution of AI usage across business model elements according to the BMC framework. The measurement is carried out by a survey aimed the current and desired utilization of AI-based performance instances within manufacturing business models in Germany.
In this context, the study does not prioritize the distribution of specific use cases across industries. Instead, it emphasizes the service value or references linked to AI-based use cases, which can be attributed to specific elements by the BMC. The investigated items ( = performance case/service values) from Figure 2. Are derived from the findings in the report “Innovations in the German Economy” and transferred into the issue of AI (Rammer et al., 2021, S. 10f). The integration of AI with at least one of the following service values leads to innovative changes that transform a traditional business model into an AI-based business model.
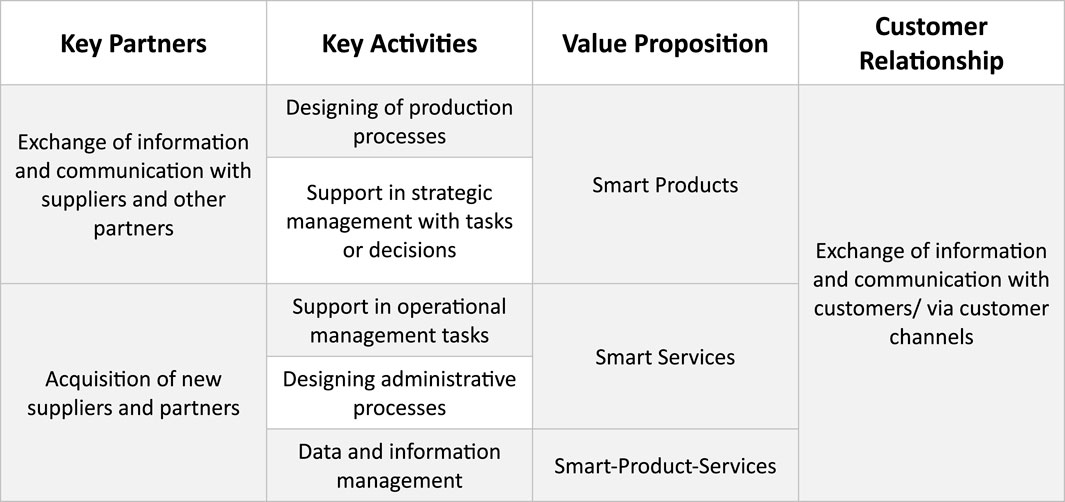
Figure 2. Indicators for service values derived from report “Innovations in the German Economy.2”
The diffusion (current performance) and deficits (target performance) of AI-based applications are assessed using the Confirmation/Disconfirmation-Paradigm (C/D-Paradigm) methodology (Homburg et al., 2019). Originally it is a marketing approach for measuring customer satisfaction (as illustrate in Figure 3). The model compares actual experiences (current performance) with a predefined performance standard. If the comparison aligns with the standard, it is termed “confirmation” which indicates customer satisfaction (confirmation → current = target). Positive disconfirmation affirming customer satisfaction as well and occurs when current performance exceeds target performance (current > target). If the target performance exceeds the current performance (current < target), this means negative disconfirmation and customer dissatisfaction.
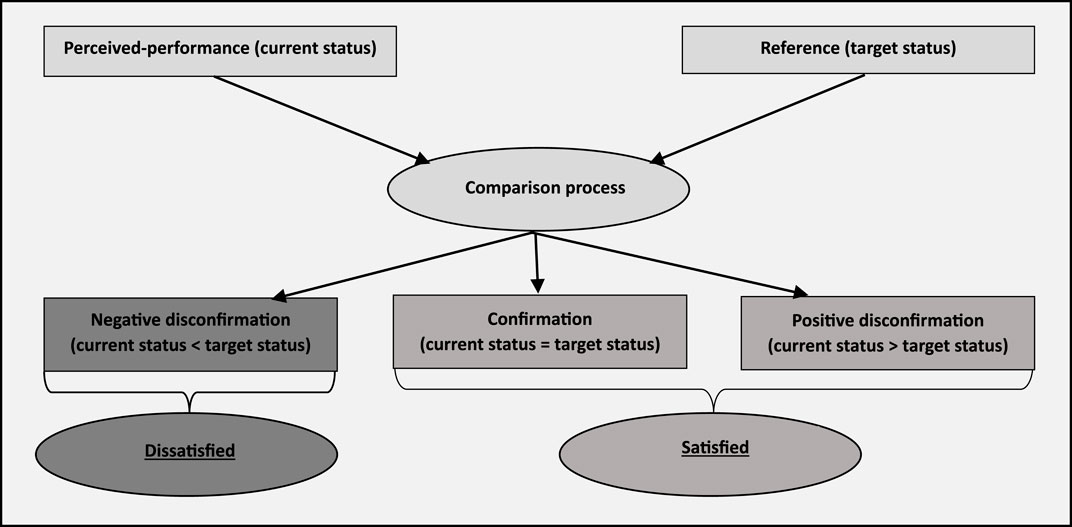
Figure 3. Confirmation-disconfirmation-paradigm according to Homburg et al. (2019).
In the survey, respondents were asked to assess their current and target performance using a 5-level Likert-Scale (1 = “do not agree at all” (2), (3), (4), 5 = “Completely agree”). Evaluating a current performance of AI usage requires the utilization of AI. Therefor a screening question was integrated to obtain conscious use. Here, “conscious use” refers to the deliberate acquisition of AI-based software to achieve performance or business objectives.
Current-performance: “My organization uses AI…”
Target-performance: “My organization should (additionally) use AI…”
E.g.: …to support in operational management tasks.
…to offer Smart-Service-Products.
…for the exchange of information and communication with customers…
The internal consistency of the items according to efficiency and effectiveness are evaluated by Cronbach’s alpha based on the given answers of the respondents. The scales represent a high or excellent value each Table 1.
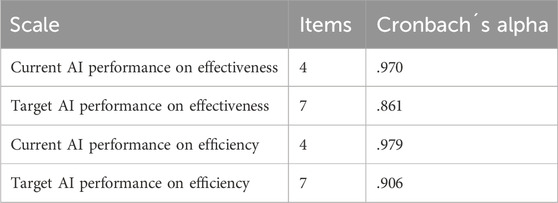
Table 1. Cronbach´s Alpha of the scales current and target performance with regard to effectiveness and efficiency.
The data was collected in an additional follow-up survey of a Choice-based Conjoint (CBC) survey. The CBC revolves around the value-based willingness to pay of cooperative AI1 and presents fictitious purchase offers for an intelligent assistance system for employees (such as Chatbot/Business Bot) as exemplary application. These applications aim to support internal business processes and workflows within organizations:
• “Send mail X to employees involved in process Y.”
• “Make an appointment with XY for *date*/*time*.”
• “Show me live monitoring of process C.”
• “Display me the colleagues/processes that need support.”
• “Show me material inventories and related products (including sales).”
The sample comprises individuals from German manufacturing business organizations who hold decision-making authority. The service provider “Kantar Group” provided a panel of n = 162 respondents that matched the target group. Data collection was collected in March 2022.3
4 Results
The sample consists of 59.26% (n = 96) SMEs and 40.74% (n = 66) large companies. These include various industries by NACE codes, classified by AI usage with absolute and relative frequencies (Figure 4) (German Federal Statistical Office, 2008). The distribution indicates that certain business organizations operate across multiple industries (industry distribution → n = 207). Participants from various positions took part in the survey, including managing directors (14.4%/n = 23), employees with management responsibilities (70%/n = 112), and employees without management responsibilities (15.6%/n = 25).
Almost 60% (n = 96) of the sample indicated AI usage at the time of data collection. Among these, there were n = 59 SMEs and n = 37 major business organizations represented. AI usage is more prevalent in industries such as engineering, vehicle manufacturing, manufacture of furniture and other goods, chemical goods, and electronic equipment. No AI usage (40%, n= 66) is less frequent in manufacturing sectors such as rubber, synthetic materials, glassware, ceramics, supplements, beverages and tobacco.
Table 2 and Figure 5 illustrate the mean current and target distributions of AI usage service values in German manufacturing business models. Table 2 additionally presents positional and scattering measures. As AI usage is a binary issue (yes or no) the metrically collected data (scale 1–5) is dichotomized by rounding to enable meaningful analysis. Values from 1 to 3.44 correspond to unconscious AI usage, while values from 3.45 to 5 indicate conscious use of AI.4
Performance cases in Table 2 suggest the following interpretation: E.g., at the time of data collection the service value of “Designing of production processes” in the business model elements of Key Activities suggest that German manufacturing industries generally do not utilize AI in these cases (M = 2.88 → 3 → no AI usage). This is affirmed by the mode 1 (→ no AI usage) and 50% of the sample reporting no current performance on this issue (x0.5 = 3).
On average, businesses in the sample express a desire for increased integration of AI across service values in all business model elements except for “Support in strategic management with tasks or decisions”. The most widespread of current AI performance is on average observed in the service value of “Designing of production processes” and “Data and information management” in Key activities. In Contrast, the lowest current AI performance besides “Support in strategic management with tasks or decisions” occurs on average in “Acquisition of new suppliers and partners”.
The strongest current diffusion of AI is being observed in the areas of “Designing of production processes” and “Data and information management.” But these are also the areas where on average the greatest desire (target performance) for AI integration. In contrast, the integration of AI service values such as “Exchange of information and communication with suppliers and other partners” and “Smart-Product” are on average the least frequently targeted issues.
The C/D paradigm is evaluated by using a t-test to identify significant differences between current and target performances in terms of effectiveness (t = −9.971, p < .001, n = 162) and efficiency (t = −9.089, p < .001, n = 162). The mean of target performance shows higher values (effectiveness: M = 3.63, SD= .995; efficiency: M = 3.69, SD= .895) than for current performance (effectiveness: M = 2.73, SD = 1.565; efficiency: M= 2.76, SD = 1.575). According to Cohen (1992), the effect size corresponds to a medium to large effect (effectiveness: d = .783; efficiency: d = .714). On average there is a significant difference between current and target performances. Table 3 includes significantly measured gabs for the respective business model elements and performance cases (service values).
Except for “Support in strategic management with tasks or decisions” there is on average a negative disconfirmation across all AI-related services. In terms of negative disconfirmations, the discrepancies are relatively similar in magnitude. However, it is notable that on average the discrepancies in the Key Activities are the largest. This indicates a significant need for improvement in these areas. Conversely, the lowest demand on average is observed in the areas of “Exchange of information and communication with suppliers and other partners” and in providing “Smart-Products.”
Figure 6 displays the mean values of target performance according to AI usage and emphasizes significant differences in terms of effectiveness (t(108.64) = 7.946, p < .000) and efficiency (t(108.94) = 5.334, p < .000) between business organizations that use AI and those that do not so far. On average, businesses using AI demonstrate higher target performance in value-driven AI services according to effectiveness (AI usage: M = 4.09, SD = .70, n = 96; No AI usage: M = 2.97, SD = .89, n = 66) as well as in efficiency (AI usage: M = 3.99, SD = .693, n = 96; No AI usage: M = 3.25, SD = .975, n = 66). According to Cohen, the effect size corresponds to a large effect (effectiveness: d = 1.352, efficiency: d = .907). Figure 6 also illustrates the discrepancies for each service value within the business model elements. Table 3 further details the measured discrepancies and effect sizes. The largest discrepancies are observed in “Smart-Products,” “Smart-Product Services,” and “Smart-Services,” while the smallest discrepancies are noted in “Support in strategic management with tasks or decisions” and “Data and information management.”
5 Discussion, conclusion and outlook
The diversity of AI usage across BM´s in German manufacturing industries is steadily increasing. Corresponding to this issue, the framework of the BMC by Osterwalder and Pigneur (2011) is used to classified and demonstrate the logic of value creation by AI in various business model elements. Furthermore, it simplifies the complexity of using digital information (data) to drive effective or efficient changes in business models.
In this context, the Business Model Canvas (BMC) provides a clear illustration of the three pillars of value creation—Value Proposition, Value Chain, and Customer Relationship—categorized into further elements which can be associated with the principals of efficiency (Key Activities, Key Resources, Key Partners, Cost Structure) and effectiveness (Value Proposition, Customer Relationships including Segments, Channels and Revenue Stream) (Osterwalder and Pigneur, 2010, S. 49). From the findings of the report “Innovations in the German Economy,” eleven value-driven AI services were derived. These are classified according to their utility value of the elements of the BMC with reference to the effectiveness and efficiency of the business model. In the framework of the study the reference of effectiveness and efficiency according to the literature by Osterwalder and Pigneur was not carefully validated for the respective value-based services. Even if the results show high Cronbach’s alpha values (>.80) and indicate an internal consistency for the service values, further investigations need to be done for valid proof.12
The sample used in this paper includes various sectors within German manufacturing industries, with a notable proportion of “Engineering,” “Vehicle manufacturing,” and “Production of furniture and other goods.” Among these industries, the percentage distribution of AI usage (AI usage = 60%; No AI usage = 40%) does not align with current scientific findings. In reality, the adoption of AI in German manufacturing business is significantly lower with a larger proportion not using AI so far. This is particularly true for SME´s, which comprise the majority of German manufacturing industries (Büchel et al., 2021; Merkel-Kiss and von Garrel, 2022). Therefor it suggests that the panel of the provider KANTAR is possibly biased towards technology-oriented companies that are pioneers in these areas. A possible bias in this regard cannot be completely ruled out. Nonetheless, the results provide valuable insights into the manufacturing sector of German industries against the current background of increasing willingness-to-accept AI in German businesses (von Garrel and Mayer, 2023).
The analysis of the eleven identified value-driven AI services with regard to the diffusion (current) and desired integration (target) is carried out by the framework and methodology of the C/D-Paradigm, which is generally used in marketing issues. Due to the broad data basis not all findings are presented and discussed in this paper. Rather the reader is shown how data and information should be interpreted based on examples.
Key findings of the sample that are discussed show that German business in manufacturing industries exhibit the highest diffusion of AI in the business model elements according to “Key Activities”. Particularly in the cases of “Designing of production processes” and “Data and information management”. Regarding to this it is important to mentioned that although these elements show the most advanced diffusion, on average there is still the greatest demand. The reason for this may could be that the designation “Data and information management” encompasses a broad spectrum of use cases. Technically, most of the services references can be considered as a kind of data and information management. At this point, the authors therefore appeal for future work to make a further distinction and classification in order to obtain more accurate results.
Further findings apply significant differences between current- and target-performance in each value-based Service reference. The observed gaps (diff) between current-performance and target-performance related to the principals of effectiveness and efficiency correspond to a medium to large effect according to Cohen. Overall, business models in German manufacturing industries exhibit a negative-disconfirmation (current < target) in each service case except in “Support in strategic management with tasks or decisions” (→ current > target). Presumably, decision-makers and managers do not want to hand over decisions with long-term impacts to technology. They want to retain the upper hand in this matter. However, the authors cannot provide a valid and verifiable reason for this but simply provide a starting point for further investigations.
There are also significant differences in target performance depending on AI usage. Business models show gabs with regard to all value-driven services according to business model elements. The results show that, on average, business models in the manufacturing industries that already use AI demonstrate a higher demand for integrating value-driven AI services into all elements of the business model compared to businesses that have not yet integrated AI. According to Cohen a medium to large effect is observed for all value-based service types. Particularly notable are the gaps in Value Proposition. On average, decision makers who have already implemented AI into their BM have also a stronger desire to integrate AI into their own value proposition as well. This is evident for “Smart-Products”, “Smart-Product Services”, and “Smart-Services”. This may be due to their own perceived value of services they use. At this point the authors can just speculate but the findings provide a basis for further research.
With regard to the research question, it can be summarized that the paper provides an insight into the diffusion of different AI value-driven service types with classification of effectiveness and efficiency according the framework of the BMC. The value-driven use of data in the shape of AI is desired in all researched elements of the BMC. The data shows that the use of AI not only increases the demand for further AI integration within the business own value creation processes but also enhances the desire to transform its own value proposition based on AI. Furthermore, the descriptive findings show a status quo and difference in that issues and can serve as crucial foundations for further research. In particular, to investigate the motivations and reasons for integrating AI into business model elements and its impact on the overall business models in manufacturing industries. Especially with regard to effectiveness and efficiency.
Data availability statement
The original contributions presented in the study are included in the article/supplementary material, further inquiries can be directed to the corresponding author.
Author contributions
PB: Conceptualization, Data curation, Methodology, Writing–original draft, Writing–review and editing. JV: Project administration, Supervision, Validation, Writing–review and editing.
Funding
The author(s) declare that financial support was received for the research, authorship, and/or publication of this article. The project on which this report is intended was funded by the German Federal Ministry of Education and Research under the grant number 02L19C157. The responsibility for the content of this publication lies with the authors.
Conflict of interest
The authors declare that the research was conducted in the absence of any commercial or financial relationships that could be construed as a potential conflict of interest.
Publisher’s note
All claims expressed in this article are solely those of the authors and do not necessarily represent those of their affiliated organizations, or those of the publisher, the editors and the reviewers. Any product that may be evaluated in this article, or claim that may be made by its manufacturer, is not guaranteed or endorsed by the publisher.
Footnotes
1Cooperative AI as generative AI show features like explaining themselves (“Explainable AI–XAI”) and adapting to humans usage behavior by interacting as collaborators (“Interactive AI”) (Holzinger, 2016; Adadi and Berrada, 2018).
2E.g., “Likewise, 11.8% of companies have made a fundamental change in their relationships with existing customers, for example, by introducing new forms of customer communication or by introducing personalized forms of customer relationship management (Rammer et al. 2021, S.10).” → “Exchange of information and communication with customers/via customer channels”.
3After the official release of ChatGPT but before it went viral.
28German Federal Statistical Office (2008)
4(1) = “Do not agree at all,” (2), (3), (4), (5) = “Completely agree at all”: According to the range of the scale, 3 relates to the zero point (e.g., (3) = “Neither agree or disagree” Such an indication does not represent any conscious of AI use.
5C= Mean of current performance.
6T= Mean of target performance.
7Diff= T - C.
8d= Effect size according to Cohen (1992).
9AI= mean of target performance of BM using AI.
10No AI= mean of target performance of BM do not using AI.
11Diff= AI–No AI.
12The authors recommend a Confirmatory Factor Analysis (CFA) for further researches.
References
Adadi, A., and Berrada, M. (2018). Peeking inside the black-box: a survey on explainable artificial intelligence (XAI). IEEE Access 6, 52138–52160. doi:10.1109/access.2018.2870052
Al-Debei, M. M., and Avison, D. (2010). Developing a unified framework of the business model concept. Eur. J. Inf. Syst. 19 (3), 359–376. doi:10.1057/ejis.2010.21
Berger, P., and von Garrel, J. (2023). How to design a value-based Chatbot for the manufacturing industry: an empirical study of an internal assistance for employees. KI - Künstliche Intell. 37, 203–211. doi:10.1007/s13218-023-00817-6
Bruhn, M., and Hadwich, K. (2021). “Künstliche Intelligenz im Dienstleistungsmanagement – Anwendungen, Einsatzbereiche und Herangehensweisen,” in Künstliche Intelligenz im Dienstleistungsmanagement. Forum Dienstleistungsmanagement. Editors M. Bruhn, and K. Hadwich (Wiesbaden: Springer Gabler). doi:10.1007/978-3-658-34324-8_1
Büchel, J., Goecke, H., Demary, V., and Mertens, A. (2021). KI-Monitor 2021. status quo der Künstlichen Intelligenz in Deutschland Gutachten. Available at: https://www.researchgate.net/publication/355118451_KI-Monitor_2021_Status_quo_der_Kunstlichen_Intelligenz_in_Deutschland/citations.
Bundesministerium fur Bildung und Forschung Hg (2021). Innovationen in der deutschen Wirtschaft. Indikatorenbericht zur Innovationserhebung 2020. Innovationsaktivitäten der Unternehmen in Deutschland im Jahre 2019, mit einem Ausblick für 2020 und 2021.
Engster, F., and Moore, P. (2020). The search for (artificial) intelligence. capitalism. Cap. Cl. 44, 030981682090205. doi:10.1177/0309816820902055
Fresner, J. (2010). Ressourceneffizienz durch Produktionsoptimierung. Z. für Wirtsch. Fabr. 105 (6), 547–550. doi:10.3139/104.110336
Garrel, V. J., and Jahn, C. (2023). Correction to: design framework for the implementation of AI-based (service) business models for Small and medium-sized manufacturing Enterprises. J. Knowl. Econ. 14, 1. doi:10.1007/s13132-023-01125-y
Gassmann, O., Frankenberger, K., and Choudury, M. (2021). “Geschäftsmodelle entwickeln. 55 innovative Konzepte mit dem St. Galler Business Model Navigator,” in überarbeitete und erweiterte Auflage (München: Carl Hanser Verlag GmbH and Co. KG).
German Federal Statistical Office (2008). Klassifikationen - Gliederung der Klassifikation der Wirtschaftszweige, Ausgabe 2008 (WZ 2008). Wiesbaden. Available at: https://www.destatis.de/DE/Methoden/Klassifikationen/Gueter-Wirtschaftsklassifikationen/Downloads/gliederung-klassifikation-wz-3100130089004.pdf?__blob=publicationFile.
Gläß, R. (2018). Künstliche Intelligenz im Handel 2 – Anwendungen. Wiesbaden: Springer Fachmedien Wiesbaden.
Holzinger, A. (2016). Interactive machine learning for health informatics: when do we need the human-in-the-loop? Brain Inf. 3 (2), 119–131. doi:10.1007/s40708-016-0042-6
Homburg, C., Koschate-Fischer, N., and Becker, A. (2019). “Messung von Markenzufriedenheit und Markenloyalität,” in Franz-rudolf esch (Hg.): handbuch markenführung (Wiesbaden: Springer Fachmedien Wiesbaden Springer Reference Wirtschaft), 1289–1306.
Hu, C., Li, Y., and Zheng, X. (2022). Data assets, information uses, and operational efficiency. Appl. Econ. 54 (60), 6887–6900. doi:10.1080/00036846.2022.2084021
Incekara, M. (2022). Türkiye'de Kaynak Verimliliği Yöntemlerinin Analizi: Üretim Maliyeti ve Satış Hacmine Etkisi. Yönetim ve Ekon. Derg. 29 (1), 121–132. doi:10.18657/yonveek.899281
Kahle, E., and Helldorff, S. (2021). “Chancen und Risiken Künstlicher Intelligenz im Dienstleistungsmanagement unter besonderer Berücksichtigung menschlicher Kompetenz und Kommunikation,” in Künstliche Intelligenz im Dienstleistungsmanagement. Forum Dienstleistungsmanagement. Editors M. Bruhn, and K. Hadwich (Wiesbaden: Springer Gabler). doi:10.1007/978-3-658-34324-8_14
Kett, H., Evcenko, D., Falkner, J., Frings, S., and Neuhüttler, J. (2021). “Künstliche Intelligenz als Veränderungstreiber für Geschäftsmodelle,” in Künstliche Intelligenz im Dienstleistungsmanagement. Forum Dienstleistungsmanagement. Editors M. Bruhn, and K. Hadwich (Wiesbaden: Springer Gabler). doi:10.1007/978-3-658-34324-8_2
Krizhevsky, A., Sutskever, I., and Hinton, G. E. (2017). ImageNet classification with deep convolutional neural networks. Commun. ACM 60 (6), 84–90. doi:10.1145/3065386
Merkel-Kiss, M., and von Garrel, J. (2022). Systematische Literaturanalyse zum KI-Einsatz und KI-basierten Geschäftsmodellen in produzierenden kleinen und mittleren Unternehmen. Z. für Arbeitswiss. 77, 1–16. doi:10.1007/s41449-022-00323-9
Moll, C., and Lerch, C. (2021): KI-basierte Geschäftsmodelle im verarbeitenden Gewerbe – Anwendungspotenziale und Ausgestaltungsmöglichkeiten. In: Manfred Bruhn und Karsten Hadwich (Hg.): Künstliche Intelligenz im Dienstleistungsmanagement. Wiesbaden: Springer Fachmedien Wiesbaden (Forum Dienstleistungsmanagement), S. 98–119.
Neuhüttler, J., Hermann, S., Ganz, W., Mark, R., and Spath, D. (2021). “Qualitätsbasiertes Testen von Smart Services am Beispiel des Flughafen Stuttgart,” in Künstliche Intelligenz im Dienstleistungsmanagement. Forum Dienstleistungsmanagement. Editors M. Bruhn, and K. Hadwich (Wiesbaden: Springer Gabler). doi:10.1007/978-3-658-34324-8_12
Ney, M. (2006). “Wirtschaftlichkeit von Interaktionsplattformen,” in Effizienz und Effektivität an der Schnittstelle zum Kunden (Wiesbaden: DUV). doi:10.1007/978-3-8350-9091-0
Odesola, I., and Daramola, N. (2010). Activated carbon production from wastes: profitability index and product cost reduction method. AJSIR 1 (2), 332–341. doi:10.5251/ajsir.2010.1.2.332.341
Oliver, R. L. (1980). A cognitive model of the antecedents and consequences of satisfaction decisions. J. Mark. Res. 17 (4), 460–469. doi:10.1177/002224378001700405
Osterwalder, A., and Pigneur, Y. (2010). “Business model generation,” in A handbook for visionaries, game changers, and challengers (Hoboken, NJ: Wiley).
Osterwalder, A., and Pigneur, Y. (2011). Business model generation: Ein Handbuch für Visionäre, Spielveränderer und Herausforderer. Campus Verlag.
Rammer, C., Doherr, T., Krieger, B., Marks, H., Niggemann, H., Peters, B., et al. (2021). Innovationen in der deutschen Wirtschaft – Indikatorenbericht zur Innovationserhebung 2021 – Innovationsaktivitäten der Unternehmen in Deutschland im Jahr 2020, mit einem Ausblick für 2021 und 2022. (Hg): Fraunhofer-Institut für System-und Innovationsforschung ISI. Mannheim: ZEW. Available at: http://ftp.zew.de/pub/zew-docs/mip/20/mip_2020.pdf.
Rohde, M., Bürger, M., Peneva, K., and Mock, J. (2022). Datenwirtschaft und Datentechnologie: Wie aus Daten Wert entsteht. Springer eBooks. doi:10.1007/978-3-662-65232-9
Schmidt, S. L., Li, M. M., Peters, C., and Leimeister, J. M. (2021). “Nutzungszentrierte Gestaltung von HI-basierten Dienstleistungen am Beispiel des IT-Support,” in Manfred Bruhnund Karsten Hadwich (Hg.): Künstliche Intelligenz im Dienstleistungsmanagement (Wiesbaden: Springer Fachmedien Wiesbaden Forum Dienstleistungsmanagement), 184–204. doi:10.1007/978-3-658-34324-8_8
Valentowitsch, J. (2021). “Künstliche Intelligenz als disruptiver Faktor im Dienstleistungsgeschäft,” in Künstliche Intelligenz im Dienstleistungsmanagement. Forum Dienstleistungsmanagement. Editors M. Bruhn, and K. Hadwich (Wiesbaden: Springer Gabler). doi:10.1007/978-3-658-34324-8_5
Venkatesh, V., Morris, M. G., Davis, G. B., and Davis, F. D. (2003). User acceptance of information technology: toward a unified view. MIS Q. 27 (3), 425–478. doi:10.2307/30036540
von Garrel, J., and Mayer, J. (2023). Artificial Intelligence in studies—use of ChatGPT and AI-based tools among students in Germany. Humanit Soc. Sci. Commun. 10, 799. doi:10.1057/s41599-023-02304-7
von Garrel, J., and Thomas, S. (2023). Künstliche Intelligenz und produzierender Mittelstand -Status quo und Potenziale zur Gestaltung und Implementierung von KI- basierten (Service)Geschäftsmodellen.
Winter, J. (2021): Mit Daten und Künstlicher Intelligenz zu digitalen Geschäftsmodellen – Praxisbeispiele aus Produktion, Logistik und Gesundheitswirtschaft. In: Manfred Bruhn und Karsten Hadwich (Hg.): Künstliche Intelligenz im Dienstleistungsmanagement. Wiesbaden: Springer Fachmedien (Forum Dienstleistungsmanagement), S. 76–97.
Keywords: AI-based service, diffusion of AI, German manufacturing industries, AI value-driven services, AI-services, Business Model Canvas (BMC), AI-driven business model
Citation: Berger P and von Garrel J (2024) Diffusion of AI value-driven services in the German manufacturing industries—an empirical examination of value-driven service references classified by the business Model Canvas. Front. Ind. Eng. 2:1407367. doi: 10.3389/fieng.2024.1407367
Received: 26 March 2024; Accepted: 31 May 2024;
Published: 26 July 2024.
Edited by:
Foivos Psarommatis, University of Oslo, NorwayReviewed by:
Tomasz J. Nitkiewicz, Częstochowa University of Technology, PolandIonica Oncioiu, Titu Maiorescu University, Romania
Copyright © 2024 Berger and von Garrel. This is an open-access article distributed under the terms of the Creative Commons Attribution License (CC BY). The use, distribution or reproduction in other forums is permitted, provided the original author(s) and the copyright owner(s) are credited and that the original publication in this journal is cited, in accordance with accepted academic practice. No use, distribution or reproduction is permitted which does not comply with these terms.
*Correspondence: Joerg von Garrel, joerg.vongarrel@h-da.de