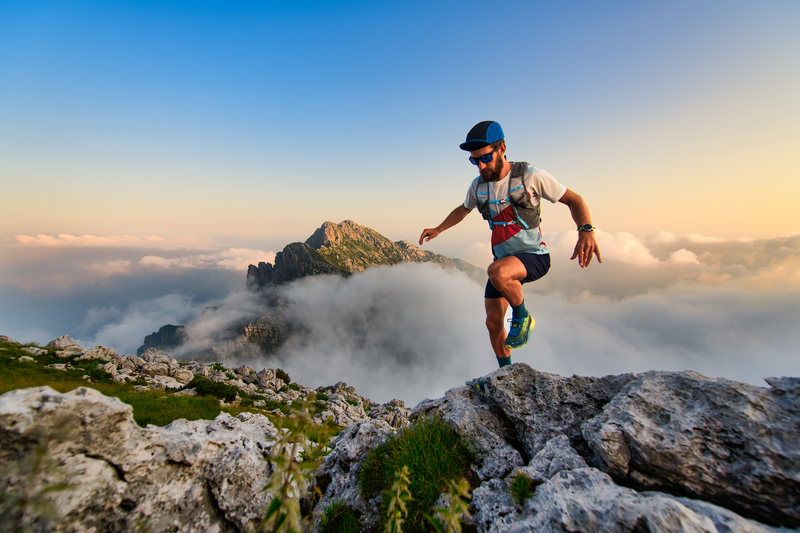
95% of researchers rate our articles as excellent or good
Learn more about the work of our research integrity team to safeguard the quality of each article we publish.
Find out more
EDITORIAL article
Front. Immunol.
Sec. T Cell Biology
Volume 16 - 2025 | doi: 10.3389/fimmu.2025.1591083
This article is part of the Research Topic Mechanistic and Statistical Modeling Approaches to Study Alloimmune T-cell responses View all 7 articles
The final, formatted version of the article will be published soon.
You have multiple emails registered with Frontiers:
Please enter your email address:
If you already have an account, please login
You don't have a Frontiers account ? You can register here
Heart and kidney transplant graft survival is suboptimal, with an average half-life of approximately 10 years. T-cells play a crucial role in graft rejection. Following transplantation, antigen recognition of allo-peptides and HLA molecules leads to the activation of donor-reactive T-cells, which underlie potential T-cell-mediated rejection (TCMR) and can promote donor-specific antibody (DSA) formation. T-cell-targeting immunosuppressive therapies currently used in transplant recipients exert their effect on all T cells. The characteristics and the dynamics of T-cell alloimmune responses remain largely undefined, thus hindering our ability to target them selectively. The advent of single-cell technologies and T-cell receptor (TCR) sequencing has constituted an inflection point in our ability to probe and monitor alloimmune responses. However, these come with the challenge of extracting relevant biological insight from the large datasets generated. Devising novel mechanistic mathematical and statistical modeling approaches is a promising strategy to address this unmet need. The six papers in the "Mechanistic and Statistical Modeling Approaches to Study Alloimmune T-cell Responses" collection explore critical aspects that shape T cell responses that can directly be applied to alloimmune responses in the context of transplantation and immunosuppressive therapies: heterogeneity of human CD8+ T cell responses to antigens, mathematical modeling of T cell recirculation in mice, insights into asymmetric T-cell division and its implications for immunotherapy, identification of diagnostic markers in the context of ischemia, the pre-organized landscape of T cell surfaces, and network analysis of immune repertoires. These studies collectively aim to enhance our understanding of T-cell dynamics and improve therapeutic strategies for transplant recipients. Yifei Geng et al. identified and validated platelet-related diagnostic markers for ischemic stroke (IS) using bioinformatics and machine learning. They integrated datasets to find differentially expressed genes, isolating 51 platelet-related genes. Six key biomarkers (APP, THBS1, F13A1, SRC, PPBP, and VCL) were identified for a diagnostic model validated in rat models. Additionally, they screened potential antiplatelet drugs, highlighting alpha-linolenic acid and ciprofibrate for their efficacy in improving coagulation function and reducing cerebral infarction. This study advances early IS diagnosis and treatment strategies.Yunmin Jung reviews the pre-organized landscape of T cell surfaces, focusing on the spatial arrangement of key receptors and signalling molecules in their resting state. He highlights the role of super-resolution microscopy in revealing nanoscale clusters of T cell receptors (TCRs) and excluding CD45 from microvilli tips. The study emphasizes the importance of these pre-organized structures in optimizing T cell activation and signalling, providing insights into the molecular mechanisms that prepare T cells for rapid and effective immune responses. The studies in this Research Topic, although not all focused directly on alloimmune responses, illustrate how statistical and mechanistic quantitative frameworks can help us understand how these T cell responses are mounted, organized, and evolve over time, thus helping accelerate the process of gleaning biological insight.
Keywords: T cells, statistical methods, computational modeling, alloimmune, Mechanism
Received: 10 Mar 2025; Accepted: 31 Mar 2025.
Copyright: © 2025 Fribourg, Castro and Bassaganya-Riera. This is an open-access article distributed under the terms of the Creative Commons Attribution License (CC BY). The use, distribution or reproduction in other forums is permitted, provided the original author(s) or licensor are credited and that the original publication in this journal is cited, in accordance with accepted academic practice. No use, distribution or reproduction is permitted which does not comply with these terms.
* Correspondence:
Miguel Fribourg, Icahn School of Medicine at Mount Sinai, New York, United States
Disclaimer: All claims expressed in this article are solely those of the authors and do not necessarily represent those of their affiliated organizations, or those of the publisher, the editors and the reviewers. Any product that may be evaluated in this article or claim that may be made by its manufacturer is not guaranteed or endorsed by the publisher.
Research integrity at Frontiers
Learn more about the work of our research integrity team to safeguard the quality of each article we publish.