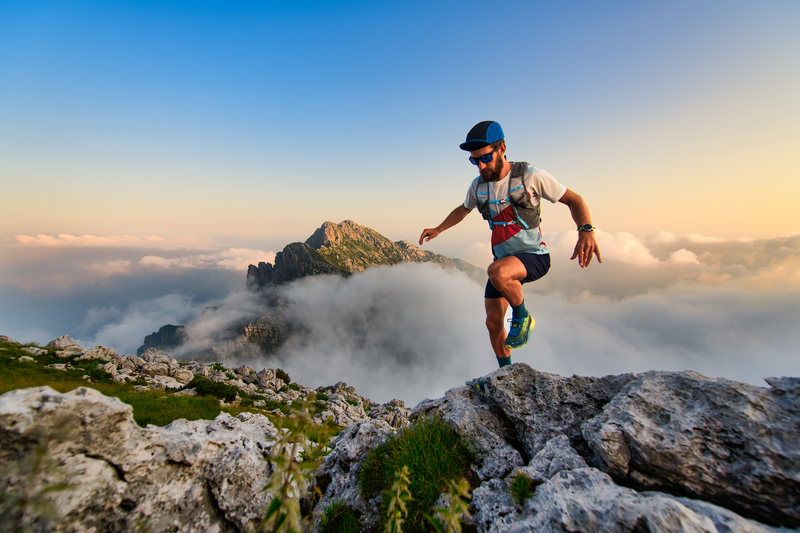
95% of researchers rate our articles as excellent or good
Learn more about the work of our research integrity team to safeguard the quality of each article we publish.
Find out more
ORIGINAL RESEARCH article
Front. Immunol.
Sec. Cancer Immunity and Immunotherapy
Volume 16 - 2025 | doi: 10.3389/fimmu.2025.1583103
The final, formatted version of the article will be published soon.
You have multiple emails registered with Frontiers:
Please enter your email address:
If you already have an account, please login
You don't have a Frontiers account ? You can register here
Background: Breast cancer is the most common malignancy in women globally, with significant heterogeneity affecting prognosis and treatment. RNA-binding proteins play vital roles in tumor progression, yet their prognostic potential remains unclear. This study introduces an Artificial Intelligence-Assisted RBP Signature (AIRS) model to improve prognostic accuracy and guide personalized treatment. Methods: Data from 14 BC cohorts (9,000+ patients) were analyzed using 108 machine learning model combinations. The AIRS model, built on three key RBP genes (PGK1, MPHOSPH10, MAP2K6), stratified patients into high-and low-risk groups. Genomic alterations, single-cell transcriptomics, tumor microenvironment characteristics, and drug sensitivity were assessed to uncover AIRS-associated mechanisms.The AIRS model demonstrated superior prognostic performance, surpassing 106 established signatures. High AIRS scores correlated with elevated tumor mutational burden, specific copy number alterations, and an immune-suppressive TME. Single-cell analysis revealed functional heterogeneity in epithelial cells, linking high AIRS scores to pathways like transcription factor binding. Regulatory network analysis identified key transcription factors such as MYC. Low AIRS scores predicted better responses to immune checkpoint inhibitors, while drug sensitivity analysis highlighted panobinostat and paclitaxel as potential therapies for high-risk patients.The AIRS model offers a robust tool for BC prognosis and treatment stratification, integrating genomic, transcriptomic, and single-cell data. It provides actionable insights for personalized therapy, paving the way for improved clinical outcomes. Future studies should validate findings across diverse populations and expand functional analyses.
Keywords: breast cancer, RNA-Binding Proteins, Prognostic model, personalized treatment, Immunotherapy
Received: 25 Feb 2025; Accepted: 01 Apr 2025.
Copyright: © 2025 Zhao, Li, Yuan, Meng, Xu, Cai, Zhang, Zhang and Wang. This is an open-access article distributed under the terms of the Creative Commons Attribution License (CC BY). The use, distribution or reproduction in other forums is permitted, provided the original author(s) or licensor are credited and that the original publication in this journal is cited, in accordance with accepted academic practice. No use, distribution or reproduction is permitted which does not comply with these terms.
* Correspondence:
Xiaonan Zhang, Bengbu Medical Universiy, Bengbu, Anhui Province, China
Tao Wang, Guizhou Provincial People's Hospital, Guiyang, China
Disclaimer: All claims expressed in this article are solely those of the authors and do not necessarily represent those of their affiliated organizations, or those of the publisher, the editors and the reviewers. Any product that may be evaluated in this article or claim that may be made by its manufacturer is not guaranteed or endorsed by the publisher.
Research integrity at Frontiers
Learn more about the work of our research integrity team to safeguard the quality of each article we publish.