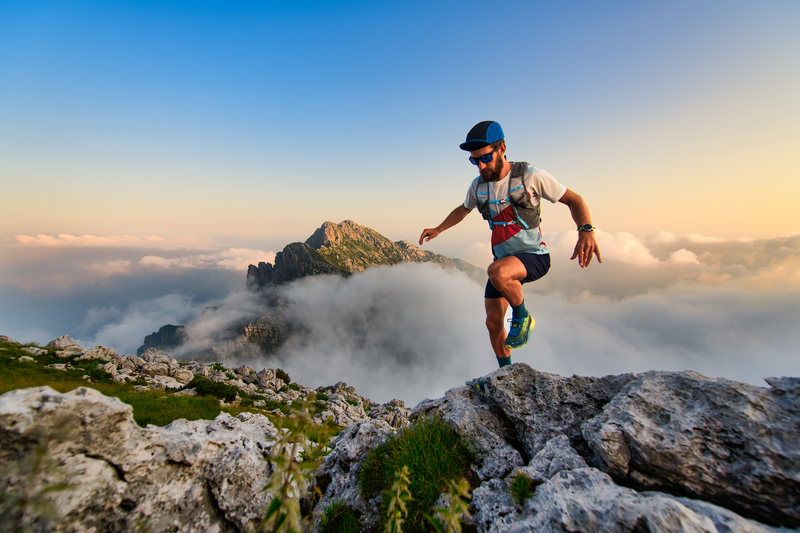
95% of researchers rate our articles as excellent or good
Learn more about the work of our research integrity team to safeguard the quality of each article we publish.
Find out more
ORIGINAL RESEARCH article
Front. Immunol.
Sec. Inflammation
Volume 16 - 2025 | doi: 10.3389/fimmu.2025.1565945
This article is part of the Research Topic Regulation of intervertebral disc homeostasis and the pathological or pathophysiological alterations under various harmful stimuli during aging process View all 3 articles
The final, formatted version of the article will be published soon.
You have multiple emails registered with Frontiers:
Please enter your email address:
If you already have an account, please login
You don't have a Frontiers account ? You can register here
Aging is characterized by gradual structural and functional changes in the body over time, with intervertebral disc degeneration (IVDD) representing a key manifestation of spinal aging and a major contributor to low back pain (LBP). This study utilized bioinformatics and machine learning approaches to identify aging-related biomarkers associated with IVDD in whole blood samples. By analyzing GEO datasets alongside aging-related databases such as GeneCards, HAGR, and AgeAnno, we identified 15 aging-related differentially expressed genes (AIDEGs). Correlation and immune infiltration analyses were conducted on these AIDEGs, and diagnostic models were developed using WGCNA, logistic regression, random forest, support vector machine, k-nearest neighbors, and LASSO regression to identify key genes. Among these, FCGR1A, CBS, and FASLG emerged as significant biomarkers with strong predictive capabilities for IVDD. Further exploration of biological , 215009
Keywords: Intervertebral Disc Degeneration, Aging-related biomarkers, bioinformatics, machine learning, immune infiltration analysis, whole blood samples 200032, 325000
Received: 24 Jan 2025; Accepted: 27 Mar 2025.
Copyright: © 2025 Li, Li, Li, Wang, Tang, Xu, Li, Tan, Pan, Liu, Jiang, Ma, Dai and Yu. This is an open-access article distributed under the terms of the Creative Commons Attribution License (CC BY). The use, distribution or reproduction in other forums is permitted, provided the original author(s) or licensor are credited and that the original publication in this journal is cited, in accordance with accepted academic practice. No use, distribution or reproduction is permitted which does not comply with these terms.
* Correspondence:
Yu-xiang Dai, Spinal Disease Research Institute, Longhua Hospital, Shanghai University of Traditional Chinese Medicine, Shanghai, Shanghai Municipality, China
Peng-fei Yu, Suzhou TCM Hospital Affiliated to Nanjing University of Chinese Medicine, Suzhou, China
Disclaimer: All claims expressed in this article are solely those of the authors and do not necessarily represent those of their affiliated organizations, or those of the publisher, the editors and the reviewers. Any product that may be evaluated in this article or claim that may be made by its manufacturer is not guaranteed or endorsed by the publisher.
Research integrity at Frontiers
Learn more about the work of our research integrity team to safeguard the quality of each article we publish.