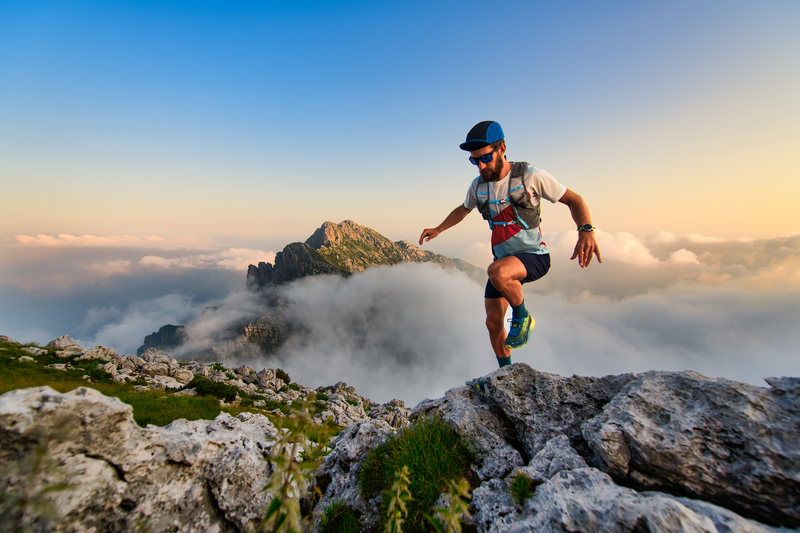
94% of researchers rate our articles as excellent or good
Learn more about the work of our research integrity team to safeguard the quality of each article we publish.
Find out more
ORIGINAL RESEARCH article
Front. Immunol.
Sec. Cancer Immunity and Immunotherapy
Volume 16 - 2025 | doi: 10.3389/fimmu.2025.1560717
This article is part of the Research Topic Exploring the Intersection of Cancer Metabolism, Metastasis and Immunotherapy View all 4 articles
The final, formatted version of the article will be published soon.
You have multiple emails registered with Frontiers:
Please enter your email address:
If you already have an account, please login
You don't have a Frontiers account ? You can register here
Background: Sepsis-induced cardiomyopathy (SIC) presents a critical complication in cancer patients, contributing notably to heart failure and elevated mortality rates. While its clinical relevance is well-documented, the intricate molecular mechanisms that link sepsis, tumor-driven inflammation, and cardiac dysfunction remain inadequately explored. This study aims to elucidate the interaction between post-tumor inflammation, intratumor heterogeneity, and the dysfunction of VSMC in SIC, as well as to evaluate the therapeutic potential of exercise training and specific pharmacological interventions.Methods: Transcriptomic data from NCBI and GEO databases were analyzed to identify differentially expressed genes (DEGs) associated with SIC. Weighted gene co-expression network analysis (WGCNA), gene ontology (GO), and KEGG pathway enrichment analyses were utilized to elucidate the biological significance of these genes. Molecular docking and dynamics simulations were used to investigate drug-target interactions, and immune infiltration and gene mutation analyses were carried out by means of platforms like TIMER 2.0 and DepMap to comprehend the influence of DVL1 on immune responsiveness.Results: Through the utilization of the datasets, we discovered the core gene DVL1 that exhibited remarkable up-regulated expression both in SIC and in diverse kinds of cancers ,which were associated with poor prognosis and inflammatory responses. Molecular docking revealed that Digoxin could bind to DVL1 and reduce oxidative stress in SIC. The DVL1 gene module related to SIC was identified by means of WGCNA, and the immune infiltration analysis demonstrated the distinctive immune cell patterns associated with DVL1 expression and the impact of DVL1 on immunotherapeutic resistance.Conclusions: DVL1 is a core regulator of SIC and other cancers and ,therefore ,can serve as a therapeutic target. The present study suggests that targeted pharmacological therapies to enhance response to exercise regimens may be a novel therapeutic tool to reduce the inflammatory response during sepsis, particularly in cancer patients. The identified drugs, Digoxin , require further in vivo and clinical studies to confirm their effects on SIC and their potential efforts to improve outcomes in immunotherapy-resistant cancer patients.
Keywords: several cancers, DVL1, intratumor heterogeneity, Oxidative Stress, Drug Therapy, Immunotherapy resistance, molecular docking, exercise training
Received: 14 Jan 2025; Accepted: 28 Feb 2025.
Copyright: © 2025 Liu, Jia, Yu, Lai, Li, Zhang, Guo, Xu and Luo. This is an open-access article distributed under the terms of the Creative Commons Attribution License (CC BY). The use, distribution or reproduction in other forums is permitted, provided the original author(s) or licensor are credited and that the original publication in this journal is cited, in accordance with accepted academic practice. No use, distribution or reproduction is permitted which does not comply with these terms.
* Correspondence:
Kailiang Xu, Department of Critical Care Medicine, Shanghai Pudong New Area Gongli Hospital, Shanghai, China
Qiancheng Luo, Department of Critical Care Medicine, Shanghai Pudong New Area Gongli Hospital, Shanghai, China
Disclaimer: All claims expressed in this article are solely those of the authors and do not necessarily represent those of their affiliated organizations, or those of the publisher, the editors and the reviewers. Any product that may be evaluated in this article or claim that may be made by its manufacturer is not guaranteed or endorsed by the publisher.
Research integrity at Frontiers
Learn more about the work of our research integrity team to safeguard the quality of each article we publish.