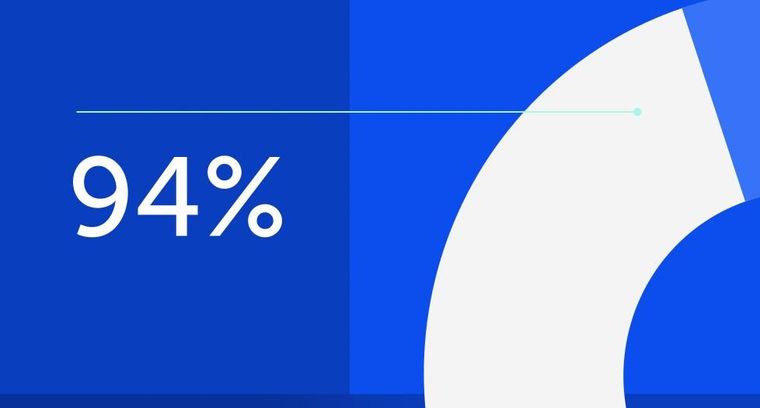
94% of researchers rate our articles as excellent or good
Learn more about the work of our research integrity team to safeguard the quality of each article we publish.
Find out more
MINI REVIEW article
Front. Immunol., 17 March 2025
Sec. Cancer Immunity and Immunotherapy
Volume 16 - 2025 | https://doi.org/10.3389/fimmu.2025.1560257
This article is part of the Research TopicHarnessing Big Data for Precision Medicine: Revolutionizing Diagnosis and Treatment StrategiesView all 39 articles
Breast cancer (BC) is a predominant malignancy among women globally, with its etiology remaining largely elusive. Diagnosis primarily relies on invasive histopathological methods, which are often limited by sample representation and processing time. Consequently, non-invasive imaging techniques such as mammography, ultrasound, and Magnetic Resonance Imaging (MRI) are indispensable for BC screening, diagnosis, staging, and treatment monitoring. Recent advancements in imaging technologies and artificial intelligence-driven radiomics have enhanced precision medicine by enabling early detection, accurate molecular subtyping, and personalized therapeutic strategies. Despite reductions in mortality through traditional treatments, challenges like tumor heterogeneity and therapeutic resistance persist. Immunotherapies, particularly PD-1/PD-L1 inhibitors, have emerged as promising alternatives. This review explores recent developments in BC imaging diagnostics and immunotherapeutic approaches, aiming to inform clinical practices and optimize therapeutic outcomes.
Breast cancer is one of the leading female malignancies globally and the second most prevalent cancer overall (1, 2). The etiology of BC remains unclear, with diagnoses primarily relying on morphological pathology, where histopathological examination is the gold standard (3). However, this invasive method faces limitations such as difficulty in obtaining representative samples and being time-consuming. Consequently, non-invasive imaging techniques like mammography, ultrasound, and MRI are essential for BC screening, diagnosis, staging, and monitoring treatment efficacy. Advances in imaging technologies and artificial intelligence have facilitated the emergence of radiomics, enhancing precision medicine through early detection, accurate molecular subtyping, staging, prognostic evaluations, and personalized treatment plans (4).
Traditional BC treatments include surgical removal, radiotherapy, chemotherapy, targeted therapies, and endocrine treatments, which have collectively reduced mortality rates. Nevertheless, challenges such as tumor heterogeneity, therapeutic resistance, metastasis, and disease recurrence persist, particularly in poor prognostic subtypes like HER-2 positive and triple-negative BC (TNBC). The five-year survival rate for advanced BC patients remains around 20% despite comprehensive treatments. There is an urgent need for innovative therapies, with immunotherapies gaining prominence. PD-1/PD-L1 inhibitors have shown effectiveness in BC immunotherapy, although challenges remain, especially for TNBC (5, 6). This review highlights recent advancements in imaging diagnostics and immunotherapy for BC to inform clinical interventions and therapeutic strategies.
Imaging technologies are essential for the diagnosis, staging, and treatment monitoring of BC. Mammography is the primary screening tool, while ultrasound, including elastography and contrast-enhanced ultrasound (CEUS), aids in assessing tumor characteristics and lymph node involvement. MRI techniques like dynamic contrast-enhanced MRI (DCE-MRI), diffusion-weighted imaging (DWI), provide detailed insights into the tumor’s microenvironment, molecular subtypes, and response to therapy. Radiomics, combined with artificial intelligence and traditional imaging, enhances diagnostic accuracy and supports personalized treatment strategies.
Conventional ultrasound is a cornerstone in BC screening, widely adopted due to its high sensitivity and specificity (7). The assessment of axillary lymph node metastasis is crucial for determining clinical outcomes and patient survival (8). Key ultrasound features, including tumor size, internal echotexture, margins, and Adler blood flow grading, are valuable for predicting axillary node involvement. Additionally, the longitudinal-to-transverse ratio serves as an important indicator for malignancy risk stratification, although its reliability in forecasting lymph node metastasis remains debated (9). Despite its critical role in diagnosing BC, guiding biopsies, localization, axillary evaluation, and follow-up, conventional ultrasound is associated with a high false-positive rate, resulting in numerous unnecessary biopsies. Studes found that recommendations for further assessment after the addition of ultrasonography to mammography screening approximately doubled, and biopsy recommendation rates increased 2- to 3-fold in patients with dense breast tissue (10, 11), potentially increasing the burden of misdiagnosis in these populations.
Ultrasound elastography, including strain and shear wave elastography (SWE), enhances conventional ultrasound by assessing tissue stiffness to differentiate benign from malignant lesions, reducing unnecessary biopsies. The method applies external force to deform tissue, with the probe capturing displacement to generate grayscale or color images (12). Although breast elastography’s accuracy versus traditional B-mode ultrasound is debated, SWE lacks increased sensitivity for ductal/lobular carcinomas (13). Tumor size affects outcomes, with smaller lesions showing better sensitivity and specificity (14). Combining elastography with conventional ultrasound improves diagnostic performance (15, 16).
CEUS visualizes tumor contrast distribution, revealing microvascular architecture and blood supply, thereby differentiating benign from malignant breast tumors with 100% sensitivity and 87.5% specificity, strongly correlating with MRI findings (17). In BC subtype classification, Wen et al. (18) identified enhancement speed and intensity from 116 lesions as subtype indicators. CEUS effectively evaluates axillary lymph node malignancy. Niu et al. (19) found uniform enhancement benign and absent/weak enhancement malignant with high sensitivity. It also predicts sentinel lymph node metastasis (20), depicts lesion reduction and microvascular changes (21), and forecasts neoadjuvant chemotherapy response. Lee et al. (22) and Peng et al. (23) demonstrated CEUS’s superiority over MRI in predicting pathological response and residual tumor size. Thus, CEUS enhances diagnostic accuracy, aids subtype differentiation, evaluates lymph node status, and predicts chemotherapy outcomes, making it essential for post-treatment BC assessment.
ABUS is a 3D ultrasound technique that automatically scans the breast from multiple angles, reducing operator dependence inherent in handheld methods. This enhances examination reproducibility and enables multi-planar reconstruction, with coronal views decreasing interpretation time (24). ABUS allows clinicians to review more images swiftly and demonstrates superior diagnostic performance for lesions smaller than 5 mm (25). However, it excludes axillary regions and lacks tools for assessing vascular distribution and tissue elasticity (26). Gatta et al. (27) showed that combining digital mammography with 3D prone-position ABUS significantly improves BC detection in women with dense breast tissue.
S-detect is a widely utilized AI-assisted system embedded in ultrasound machines, leveraging deep learning algorithms for computer-aided diagnosis. Aligned with the Breast Imaging Reporting and Data System (BI-RADS), it autonomously evaluates key tumor characteristics, including size, shape, depth, margins, and internal structure, and classifies findings as either “possibly benign” or “possibly malignant”. Applied in BC diagnostics, S-detect enhances ultrasound accuracy and clinical diagnostic capabilities (28, 29). When adjunctive for BI-RADS category 4 nodules, it significantly reduces false-positive biopsy rates, minimizing unnecessary invasive procedures (30).
Ultrasound-guided percutaneous biopsy employs real-time ultrasound to precisely locate lesions and guide needle insertion for tissue sampling, enabling accurate pathological examination. This minimally invasive, highly accurate, and low-trauma procedure is widely used in clinical practice (31). Pathological data confirm BC diagnoses and provide critical information on histological types, grading, and molecular tumor features, while also effectively detecting metastatic lymph nodes (32). In cases of ambiguous malignancies, surgical biopsy is often debated; preoperative malignancy assessment could reduce surgeries and patient burden. Girardi et al. (33) found that this technique enhances diagnostic accuracy for uncertain malignancies, with an upgrade rate of approximately 3%, thereby improving management of suspicious lesions.
DCE-MRI employs high-resolution T1-weighted isotropic sequences and rapid gadolinium-based contrast agent administration via high-pressure injectors to enhance imaging. It exploits tumor-induced angiogenesis, leading to permeable blood vessels where contrast agents leak into the interstitial space, causing localized signal enhancement. By analyzing time-signal intensity curves, parameters such as Ktrans, kep, and ve differentiate enhancement kinetics in breast lesions. Quantitative MRI morphologies of invasive BC correlate significantly with immunohistochemical biomarkers and subtypes (34, 35). Differentiating benign from malignant lesions using Ktrans, kep, and ve achieves accuracy rates of 94.50%, 79.82%, and 87.16%, respectively, with sensitivities up to 99% and specificities as high as 97% (36).
MRS employs point-resolved spectroscopy or stimulated echo acquisition mode voxel sequences to acquire spectroscopic images for tissue chemical analysis (37). It demonstrates high diagnostic sensitivity and stable specificity, particularly effective for early-stage BC, small tumors, and non-mass enhancing lesions (38). Lipid metabolites differentiate benign from malignant conditions, enhancing MRI specificity in fat necrosis identification, reducing unnecessary biopsies. Invasive ductal carcinoma exhibits a higher water-to-fat ratio, indicating BC response to neoadjuvant chemotherapy (39, 40). MRS also evaluates tumor aggressiveness, with elevated total choline (tCho) in highly proliferative tumors and minimal choline peaks in low-activity lobular carcinoma (41). Despite its potential, MRS lacks widespread integration into multiparametric MRI protocols, requiring optimization through multicenter trials for reproducibility and accuracy.
DWI measures the mobility of water molecules within tissues, indirectly reflecting pathological and physiological characteristics influenced by factors such as cellular density, membrane integrity, and microstructural constraints. It is clinically recognized as a highly sensitive method for BC detection (42). Research suggests that DWI may also aid in predicting pathological grading (43). Apparent Diffusion Coefficient (ADC) values are significantly reduced in both estrogen receptor (ER)-positive and ER-negative BC (44). Conversely, HER-2 positive BC exhibit higher ADC values compared to HER-2 negative cases. Interestingly, lower ADC values are observed in ER/PR-positive BC, which is atypical since ER/PR expression is generally associated with slower-growing, lower-grade tumors, indicating an area ripe for further investigation.
IVIM imaging enhances DWI by using a bi-exponential model to separate microcirculatory perfusion from water diffusion. Parameters ADC, coefficient (D), pseudo-diffusion coefficient (D*), and perfusion fraction (F) differentiate benign from malignant breast lesions. Low b-values reflect both diffusion and perfusion, while high b-values mainly indicate diffusion, aiding tumor microcirculation and diffusion analysis (45). IVIM-MRI identifies tumor types, prognostic biomarkers, and therapy response. Lower ADC values link to aggressive invasive BC phenotypes (46, 47). In Luminal B tumors, D and ADC are lower than in Luminal A. ER expression correlates with ADC, D, and F, while D* relates to Ki-67. IVIM complements dynamic contrast-enhanced MRI for precise differentiation (48).
DKI surpasses DWI and DTI by using non-Gaussian diffusion-weighted analysis, calculating diffusion coefficient (D) and kurtosis (K) to quantify tissue water diffusion deviations (49). It detects abnormal water diffusion in tissues, with invasive BC showing lower D values than benign lesions. Ductal carcinoma in situ also has lower D values than benign conditions. The 50th and 75th percentile D values in invasive BC are lower than in ductal carcinoma in situ, offering 95.7% specificity in distinguishing benign lesions from invasive cancer (49).
2D digital mammography (DM) remains the leading modality for BC screening and diagnostic evaluation in recalled patients (50). However, DM’s efficacy is hindered by tissue overlap in 2D images, which diminishes sensitivity (70%, dropping to 30% in highly dense breasts) (51–53) and specificity (92%), causing 8% of healthy women to undergo unnecessary recalls (52, 54). Additionally, DM entails slight radiation exposure from x-rays (55) and significant patient discomfort due to required breast compression (56). Digital breast tomosynthesis (DBT) enhances mammography by acquiring multiple tomographic images per view, generating a “semi-3D” mammogram. This technique produces sequential thin slices, reducing tissue masking, improving cancer detection, and lowering false-positive rates (57).
Breast CT provides comprehensive three-dimensional imaging, thereby reducing the interference of overlapping anatomical tissues in breast evaluations. In this method, patients are positioned prone, allowing the breast to naturally extend away from the chest wall without compression. The x-ray source and flat-panel detector rotate horizontally around the breast, capturing numerous cone-beam projections that are subsequently reconstructed into a 3D CT image (58). While breast CT offers enhanced visualization of mass lesions compared to mammography (58, 59), it exhibits lower spatial resolution (60), is less effective in detecting microcalcifications (58), and involves a higher radiation dose.
Radiomics, introduced by Gillies et al. (61) extracts quantitative features from medical images using high-throughput computing, transforming images into multidimensional datasets for tumor evaluation, diagnosis, and prognosis prediction (62). The workflow includes data acquisition, tumor segmentation, feature extraction, selection, and model development (63, 64). In BC, radiomics applications are diverse: predicting axillary lymph node metastasis [Cui et (65)], combining mammography and MRI for sentinel lymph node prediction [Cheng et al. (66)], distinguishing BC subtypes and receptor status [Fan et al. (67), Leithner et al. (68)], assessing TILs (69), establishing immune scores [Han et al. (70)], and enhancing neoadjuvant chemotherapy (NAC) efficacy evaluation (71, 72). Radiomics also links MRI features with molecular subtypes, pathological complete response (pCR), and residual tumor burden [Choudhery et al. (73)], while predicting axillary metastasis and recurrence risk [Yu et al. (74) and Kim et al. (75)]. Ultrasound and PET radiomics further predict lymph node involvement, molecular subtypes, and recurrence (76–87). These advancements underscore radiomics’ potential as a non-invasive biomarker for precise BC clinical decision-making (Table 1).
Immunotherapy aims to modulate the TME by targeting immune suppression and evasion, employing strategies such as antigen release, PD-1/L1 inhibition, immune activation, T cell infiltration, cancer recognition, and apoptosis induction (88–92), synergistically eliminating BC cells (93). However, the immunosuppressive TME remains a significant barrier to the efficacy of these therapies, especially in aggressive subtypes like TNBC.
PD-1, an immune checkpoint on activated cytotoxic T lymphocytes in the TME, maintains immune tolerance and limits tumor-facilitating responses (94–98). In BC, particularly in TNBC, tumors often overexpress PD-L1, which binds PD-1, inhibiting T cell proliferation and cytokine secretion, enabling immune evasion. Inflammation is pivotal in disease progression (99–102). The immunosuppressive TME, characterized by regulatory T cells (Tregs), myeloid-derived suppressor cells (MDSCs) (103), and tumor-associated macrophages (TAMs) secreting cytokines like TGF-β and IL-10, further suppresses T cell responses and promotes tumor progression (104–107). PD-1/PD-L1 inhibitors disrupt this interaction, reactivating T cells and suppressing MDSCs (108). However, monotherapy efficacy is limited: Avelumab shows 5.2% response in unselected TNBC and 22.2% in PD-L1+ cases (109), pembrolizumab achieves 18.5% in PD-L1+ TNBC (110), and other trials report ≤10% objective response rate (ORR) with no progression-free survival (PFS) or overall survival (OS) benefits (111–113). MDSC accumulation in TNBC further suppresses T cell activation, hindering PD-1/PD-L1 inhibitor efficacy (106). Combining these inhibitors with other immunomodulatory agents may enhance tumor antigen release, immune cell infiltration, and reduce immunosuppressive cell activity, potentially improving outcomes.
Combining ICIs, such as CTLA-4 inhibitors (e.g., ipilimumab) and PD-1/PD-L1 inhibitors, enhances antitumor immune responses by targeting distinct pathways. CTLA-4 inhibitors boost early T-cell activation, while PD-1/PD-L1 inhibitors prevent T-cell suppression in the TME (114, 115). This dual approach shows clinical efficacy across tumors, with metastatic BC achieving a 17% ORR (43% in TNBC, 0% in ER-positive) (116, 117). IDO inhibitors with nivolumab are also under investigation for advanced solid tumors, including BC (118).
Resistance to immunotherapy in BC often stems from the immune system’s inability to recognize tumors due to insufficient immunogenic neoantigens (119), which are crucial for personalized cancer vaccines (120). Cryoablation induces cell death, inflammation, and neoantigen exposure, enhancing immune detection (121). A pilot study in ER-positive patients demonstrated that ipilimumab combined with cryoablation significantly increased CD8+ T cells and Th1 cytokines, boosting antitumor immunity (122, 123). T cell receptor sequencing linked TILs to expanded T cell clones, serving as biomarkers (124). Post-translational modifications, like 5-azacytidine-induced hypomethylation of immune genes (125), and preclinical studies suggest PD-L1 upregulation (126). Histone deacetylase inhibitors also exhibit immunomodulatory effects (127), and combining epigenetic therapies with immunotherapies may improve antitumor responses and efficacy (128).
Combining ICIs with chemotherapy results in higher TNBC response rates than ICI alone. Chemotherapy partially reverses the TNBC immunosuppressive microenvironment and upregulates PD-L1 on BC cells, creating synergy (129). A phase Ib trial of atezolizumab with albumin-bound paclitaxel in advanced TNBC showed a 39.4% ORR, likely due to paclitaxel-induced TLR activation and dendritic cell stimulation (130). The I-SPY2 trial indicated that adding anthracyclines and cyclophosphamide to paclitaxel and pembrolizumab increased efficacy from 22% to 60%, likely via anthracycline-induced immune stimulation and antigen presentation (131). Furthermore, KEYNOTE-355 found pembrolizumab with chemotherapy significantly improved PFS in PD-L1+ TNBC, especially CPS≥10 (132).
By inducing antigen release, recruiting antigen-presenting cells, and stimulating T cell responses, radiotherapy enhances synergy with ICIs. In a BC mouse model, radiotherapy combined with PD-L1 blockade slowed tumor growth and activated CD8+ T cells (133). Clinical pilot studies showed partial responses and stable disease in HER2-positive and HER2-negative BC patients treated with ICIs and radiotherapy, with notable abscopal effects in metastatic TNBC patients (134). Despite small sample sizes, these findings underscore durable abscopal effects and the importance of patient selection, prompting further investigation in subsequent trials (135).
Traditional targeted therapies in BC aim to improve patient survival by targeting oncogenes or tumor suppressor genes, though resistance often develops. Combining targeted therapies with immunotherapy can remodel the TME and enhance antitumor immune responses (136). PARP inhibitors targeting BRCA1/2 mutations elevate cytosolic DNA, activating interferon pathways and enhancing type I interferon and T cell infiltration (137). They also upregulate PD-L1, making them apt for immunotherapy combinations (138). The phase II TOPACIO trial reported a 47% response rate with niraparib and pembrolizumab (139), while ipatasertib with atezolizumab and paclitaxel achieved a 73% response (140). Ongoing studies assess MEK inhibitors with ICIs in TNBC, enhancing PD-1/L1 blockade responses (141).
Current ACT strategies for BC include TILs, CAR-T, CAR-NK, and TCR-T cells, each with distinct features. TILs act as prognostic indicators for BC outcomes. CAR-T and CAR-NK cells target solid tumors, with numerous clinical trials addressing various antigens. For example, EGFR-CAR-T cells significantly inhibit TNBC growth in vitro and in vivo (142), and ICAM-1-specific CAR-T cells effectively reduce tumor growth by targeting ICAM-1-expressing TNBC cells (143). MUCl-CAR-T therapy evaluated autologous MUCl-CAR-T cells in relapsed or refractory TNBC (144). CAR-NK therapies utilize NK receptors to induce apoptosis, showing therapeutic promise (145). While CAR-T trials in TNBC are expanding, TCR-T therapies are limited by MHC dependency (146) (Figure 1).
The integration of advanced imaging, radiomics, and artificial intelligence has enhanced BC diagnostics and molecular characterization. Immunotherapies, including PD-1/PD-L1 inhibitors and combination therapies, address tumor heterogeneity and resistance. Liquid biopsy, particularly circulating tumor DNA (ctDNA) detection, enables treatment monitoring and minimal residual disease detection (147). Nanotechnology improves drug delivery, enhancing efficacy and reducing side effects of chemotherapy and immunotherapy (148). Targeting the TME through immune checkpoint inhibition and immune suppression reversal offers transformative potential for BC treatment (149). Combined with genomic sequencing and data-driven models, these innovations promise a more precise and effective therapeutic framework for BC.
JH: Writing – original draft. NL: Writing – original draft. LZ: Writing – original draft, Writing – review & editing.
The author(s) declare that no financial support was received for the research and/or publication of this article.
The authors declare that the research was conducted in the absence of any commercial or financial relationships that could be construed as a potential conflict of interest.
The author(s) declare that no Generative AI was used in the creation of this manuscript.
All claims expressed in this article are solely those of the authors and do not necessarily represent those of their affiliated organizations, or those of the publisher, the editors and the reviewers. Any product that may be evaluated in this article, or claim that may be made by its manufacturer, is not guaranteed or endorsed by the publisher.
1. Sung H, Ferlay J, Siegel RL, Laversanne M, Soerjomataram I, Jemal A, et al. Global cancer statistics 2020: GLOBOCAN estimates of incidence and mortality worldwide for 36 cancers in 185 countries. CA Cancer J Clin. (2021) 71:209–49. doi: 10.3322/caac.21660
2. Sui S, Xu S, Pang D. Emerging role of ferroptosis in breast cancer: New dawn for overcoming tumor progression. Pharmacol Ther. (2022) 232:107992. doi: 10.1016/j.pharmthera.2021.107992
3. Makki J. Diversity of breast carcinoma: histological subtypes and clinical relevance. Clin Med Insights Pathol. (2015) 8:23–31. doi: 10.4137/CPath.S31563
4. Mayerhoefer ME, Materka A, Langs G, Haggstrom I, Szczypinski P, Gibbs P, et al. Introduction to radiomics. J Nucl Med. (2020) 61:488–95. doi: 10.2967/jnumed.118.222893
5. Mina LA, Lim S, Bahadur SW, Firoz AT. Immunotherapy for the treatment of breast cancer: emerging new data. Breast Cancer (Dove Med Press). (2019) 11:321–8. doi: 10.2147/BCTT.S184710
6. Yang Y, Wu Q, Chen L, Qian K, Xu X. Severe immune-related hepatitis and myocarditis caused by PD-1 inhibitors in the treatment of triple-negative breast cancer: a case report. Ann Transl Med. (2022) 10:424. doi: 10.21037/atm-22-1284
7. Sood R, Rositch AF, Shakoor D, Ambinder E, Pool KL, Pollack E, et al. Ultrasound for breast cancer detection globally: A systematic review and meta-analysis. J Glob Oncol. (2019) 5:1–17. doi: 10.1200/JGO.19.00127
8. Mori N, Li L, Matsuda M, Mori Y, Mugikura S. Prospects of perfusion contrast-enhanced ultrasound (CE-US) in diagnosing axillary lymph node metastases in breast cancer: a comparison with lymphatic CE-US. J Med Ultrason (2001). (2024) 51:587–97. doi: 10.1007/s10396-024-01444-w
9. Hu X, Xue J, Peng S, Yang P, Yang Z, Yang L, et al. Preoperative nomogram for predicting sentinel lymph node metastasis risk in breast cancer: A potential application on omitting sentinel lymph node biopsy. Front Oncol. (2021) 11:665240. doi: 10.3389/fonc.2021.665240
10. Rebolj M, Assi V, Brentnall A, Parmar D, Duffy SW. Addition of ultrasound to mammography in the case of dense breast tissue: systematic review and meta-analysis. Br J Cancer. (2018) 118:1559–70. doi: 10.1038/s41416-018-0080-3
11. Lee JM, Arao RF, Sprague BL, Kerlikowske K, Lehman CD, Smith RA, et al. Performance of screening ultrasonography as an adjunct to screening mammography in women across the spectrum of breast cancer risk. JAMA Intern Med. (2019) 179:658–67. doi: 10.1001/jamainternmed.2018.8372
12. Sigrist RMS, Liau J, Kaffas AE, Chammas MC, Willmann JK. Ultrasound elastography: review of techniques and clinical applications. Theranostics. (2017) 7:1303–29. doi: 10.7150/thno.18650
13. Giuseppetti GM, Martegani A, Di Cioccio B, Baldassarre S. Elastosonography in the diagnosis of the nodular breast lesions: preliminary report. Radiol Med. (2005) 110:69–76.
14. Shiina T, Nightingale KR, Palmeri ML, Hall TJ, Bamber JC, Barr RG, et al. WFUMB guidelines and recommendations for clinical use of ultrasound elastography: Part 1: basic principles and terminology. Ultrasound Med Biol. (2015) 41:1126–47. doi: 10.1016/j.ultrasmedbio.2015.03.009
15. Watanabe T, Yamaguchi T, Okuno T, Konno S, Takaki R, Sato M, et al. Utility of B-Mode, color doppler and elastography in the diagnosis of breast cancer: results of the CD-CONFIRM multicenter study of 1351 breast solid masses. Ultrasound Med Biol. (2021) 47:3111–21. doi: 10.1016/j.ultrasmedbio.2021.07.009
16. Golatta M, Pfob A, Busch C, Bruckner T, Alwafai Z, Balleyguier C, et al. The potential of combined shear wave and strain elastography to reduce unnecessary biopsies in breast cancer diagnostics - An international, multicentre trial. Eur J Cancer. (2022) 161:1–9. doi: 10.1016/j.ejca.2021.11.005
17. Ricci P, Cantisani V, Ballesio L, Pagliara E, Sallusti E, Drudi FM, et al. Benign and Malignant breast lesions: efficacy of real time contrast-enhanced ultrasound vs. magnetic resonance imaging. Ultraschall Med. (2007) 28:57–62. doi: 10.1055/s-2006-927226
18. Wen B, Kong W, Zhang Y, Xue H, Wu M, Wang F. Association between contrast-enhanced ultrasound characteristics and molecular subtypes of breast cancer. J Ultrasound Med. (2022) 41:2019–31. doi: 10.1002/jum.15886
19. Niu Z, Xiao M, Ma L, Qin J, Li W, Zhang J, et al. The value of contrast-enhanced ultrasound enhancement patterns for the diagnosis of sentinel lymph node status in breast cancer: systematic review and meta-analysis. Quant Imaging Med Surg. (2022) 12:936–48. doi: 10.21037/qims-21-416
20. Huang C, Luo J, Shan Z, Zhen T, Li J, Ma Q, et al. The value of the improved percutaneous and intravenous contrast-enhanced ultrasound diagnostic classification in sentinel lymph nodes of breast cancer. Quant Imaging Med Surg. (2024) 14:2391–404. doi: 10.21037/qims-23-1210
21. Kinoshita S, Miyake R, Shimada N, Hirano A, Seki Y, Shimizu K, et al. Initial experience with contrast-enhanced ultrasonography in follow-up assessment of small breast cancer treated by cryoablation. Australasian Medical Journal (2017) 10:599. doi: 10.21767/AMJ.2017.3034
22. Lee SC, Grant E, Sheth P, Garcia AA, Desai B, Ji L, et al. Accuracy of contrast-enhanced ultrasound compared with magnetic resonance imaging in assessing the tumor response after neoadjuvant chemotherapy for breast cancer. J Ultrasound Med. (2017) 36:901–11. doi: 10.7863/ultra.16.05060
23. Peng J, Pu H, Jia Y, Chen C, Ke XK, Zhou Q. Early prediction of response to neoadjuvant chemotherapy using contrast-enhanced ultrasound in breast cancer. Med (Baltimore). (2021) 100:e25908. doi: 10.1097/MD.0000000000025908
24. Philadelpho F, Calas MJG, Carneiro GAC, Silveira IC, Vaz ABR, Nogueira AMC, et al. Comparison of automated breast ultrasound and hand-held breast ultrasound in the screening of dense breasts. Rev Bras Ginecol Obstet. (2021) 43:190–9. doi: 10.1055/s-0040-1722156
25. Mostafa AAE, Eltomey MA, Elaggan AM, Hashish AA. Automated breast ultrasound (ABUS) as a screening tool: initial experience. Egyptian Journal of Radiology and Nuclear Medicine (2019) 50:1–6. doi: 10.1186/s43055-019-0032-9
26. Schiaffino S, Gristina L, Tosto S, Massone E, De Giorgis S, Garlaschi A, et al. The value of coronal view as a stand-alone assessment in women undergoing automated breast ultrasound. Radiol Med. (2021) 126:206–13. doi: 10.1007/s11547-020-01250-7
27. Gatta G, Cappabianca S, La Forgia D, Massafra R, Fanizzi A, Cuccurullo V, et al. Second-generation 3D automated breast ultrasonography (Prone ABUS) for dense breast cancer screening integrated to mammography: effectiveness, performance and detection rates. J Pers Med. (2021) 11:875. doi: 10.3390/jpm11090875
28. Xia Q, Cheng Y, Hu J, Huang J, Yu Y, Xie H, et al. Differential diagnosis of breast cancer assisted by S-Detect artificial intelligence system. Math Biosci Eng. (2021) 18:3680–9. doi: 10.3934/mbe.2021184
29. O’Connell AM, Bartolotta TV, Orlando A, Jung SH, Baek J, Parker KJ. Diagnostic performance of an artificial intelligence system in breast ultrasound. J Ultrasound Med. (2022) 41:97–105. doi: 10.1002/jum.15684
30. Li N, Liu W, Zhan Y, Bi Y, Wu X, Peng M. Value of S-Detect combined with multimodal ultrasound in differentiating Malignant from benign breast masses. Egyptian Journal of Radiology and Nuclear Medicine (2024) 55:22. doi: 10.1186/s43055-023-01183-x
31. Newell MS, Mahoney MC. Ultrasound-guided percutaneous breast biopsy. Tech Vasc Interv Radiol. (2014) 17:23–31. doi: 10.1053/j.tvir.2013.12.005
32. Ouyang T, Li JF, Wang TF, Xie YT, Fan ZQ, Lin BY. Clinical study of percutaneous ultrasound-guided or freehand core needle biopsy of primary breast cancer. Zhonghua Wai Ke Za Zhi. (2005) 43:1519–21.
33. Girardi V, Guaragni M, Ruzzenenti N, Palmieri F, Fogazzi G, Cozzi A, et al. B3 Lesions at Vacuum-Assisted Breast Biopsy under Ultrasound or Mammography Guidance: A Single-Center Experience on 3634 Consecutive Biopsies. Cancers (Basel). (2021) 13:5443. doi: 10.3390/cancers13215443
34. Bae MS, Seo M, Kim KG, Park IA, Moon WK. Quantitative MRI morphology of invasive breast cancer: correlation with immunohistochemical biomarkers and subtypes. Acta Radiol. (2015) 56:269–75. doi: 10.1177/0284185114524197
35. Liu H, Zhan H, Sun D, Zhang Y. Comparison of BSGI, MRI, mammography, and ultrasound for the diagnosis of breast lesions and their correlations with specific molecular subtypes in Chinese women. BMC Med Imaging. (2020) 20:98. doi: 10.1186/s12880-020-00497-w
36. Machireddy A, Thibault G, Tudorica A, Afzal A, Mishal M, Kemmer K, et al. Early prediction of breast cancer therapy response using multiresolution fractal analysis of DCE-MRI parametric maps. Tomography. (2019) 5:90–8. doi: 10.18383/j.tom.2018.00046
37. Fardanesh R, Marino MA, Avendano D, Leithner D, Pinker K, Thakur SB. Proton MR spectroscopy in the breast: Technical innovations and clinical applications. J Magn Reson Imaging. (2019) 50:1033–46. doi: 10.1002/jmri.26700
38. Baltzer PA, Dietzel M. Breast lesions: diagnosis by using proton MR spectroscopy at 1.5 and 3.0 T–systematic review and meta-analysis. Radiology. (2013) 267:735–46. doi: 10.1148/radiol.13121856
39. Bolan PJ, Kim E, Herman BA, Newstead GM, Rosen MA, Schnall MD, et al. MR spectroscopy of breast cancer for assessing early treatment response: Results from the ACRIN 6657 MRS trial. J Magn Reson Imaging. (2017) 46:290–302. doi: 10.1002/jmri.25560
40. Choi JS, Yoon D, Koo JS, Kim S, Park VY, Kim EK, et al. Magnetic resonance metabolic profiling of estrogen receptor-positive breast cancer: correlation with currently used molecular markers. Oncotarget. (2017) 8:63405–16. doi: 10.18632/oncotarget.18822
41. Gruber S, Debski BK, Pinker K, Chmelik M, Grabner G, Helbich T, et al. Three-dimensional proton MR spectroscopic imaging at 3 T for the differentiation of benign and Malignant breast lesions. Radiology. (2011) 261:752–61. doi: 10.1148/radiol.11102096
42. Sippo DA, Burk KS, Mercaldo SF, Rutledge GM, Edmonds C, Guan Z, et al. Performance of screening breast MRI across women with different elevated breast cancer risk indications. Radiology. (2019) 292:51–9. doi: 10.1148/radiol.2019181136
43. Partridge SC. Amornsiripanitch N: DWI in the assessment of breast lesions. Top Magn Reson Imaging. (2017) 26:201–9. doi: 10.1097/RMR.0000000000000137
44. Jena A, Taneja S, Singh A, Negi P, Mehta SB, Sarin R. Role of pharmacokinetic parameters derived with high temporal resolution DCE MRI using simultaneous PET/MRI system in breast cancer: A feasibility study. Eur J Radiol. (2017) 86:261–6. doi: 10.1016/j.ejrad.2016.11.029
45. You C, Li J, Zhi W, Chen Y, Yang W, Gu Y, et al. The volumetric-tumour histogram-based analysis of intravoxel incoherent motion and non-Gaussian diffusion MRI: association with prognostic factors in HER2-positive breast cancer. J Transl Med. (2019) 17:182. doi: 10.1186/s12967-019-1911-6
46. Suo S, Cheng F, Cao M, Kang J, Wang M, Hua J, et al. Multiparametric diffusion-weighted imaging in breast lesions: Association with pathologic diagnosis and prognostic factors. J Magn Reson Imaging. (2017) 46:740–50. doi: 10.1002/jmri.25612
47. Kawashima H, Miyati T, Ohno N, Ohno M, Inokuchi M, Ikeda H, et al. Differentiation between luminal-A and luminal-B breast cancer using intravoxel incoherent motion and dynamic contrast-enhanced magnetic resonance imaging. Acad Radiol. (2017) 24:1575–81. doi: 10.1016/j.acra.2017.06.016
48. Ma D, Lu F, Zou X, Zhang H, Li Y, Zhang L, et al. Intravoxel incoherent motion diffusion-weighted imaging as an adjunct to dynamic contrast-enhanced MRI to improve accuracy of the differential diagnosis of benign and Malignant breast lesions. Magn Reson Imaging. (2017) 36:175–9. doi: 10.1016/j.mri.2016.10.005
49. Park VY, Kim SG, Kim EK, Moon HJ, Yoon JH, Kim MJ. Diffusional kurtosis imaging for differentiation of additional suspicious lesions on preoperative breast MRI of patients with known breast cancer. Magn Reson Imaging. (2019) 62:199–208. doi: 10.1016/j.mri.2019.07.011
50. Durand MA, Philpotts LE. : Can Tomosynthesis replace 2D mammography as a future breast screening tool? Current Breast Cancer Reports (2016) 8:213–20. doi: 10.1007/s12609-016-0224-5
51. Alakhras M, Bourne R, Rickard M, Ng KH, Pietrzyk M, Brennan PC. Digital tomosynthesis: a new future for breast imaging? Clin Radiol. (2013) 68:e225–236. doi: 10.1056/NEJMoa052911
52. Pisano ED, Gatsonis C, Hendrick E, Yaffe M, Baum JK, Acharyya S, et al. Diagnostic performance of digital versus film mammography for breast-cancer screening. N Engl J Med. (2005) 353:1773–83. doi: 10.1056/NEJMoa052911
53. Mandelson MT, Oestreicher N, Porter PL, White D, Finder CA, Taplin SH, et al. Breast density as a predictor of mammographic detection: comparison of interval- and screen-detected cancers. J Natl Cancer Inst. (2000) 92:1081–7. doi: 10.1093/jnci/92.13.1081
54. Ciatto S, Houssami N, Bernardi D, Caumo F, Pellegrini M, Brunelli S, et al. Integration of 3D digital mammography with tomosynthesis for population breast-cancer screening (STORM): a prospective comparison study. Lancet Oncol. (2013) 14:583–9. doi: 10.1016/S1470-2045(13)70134-7
55. Feig SA, Hendrick RE. Radiation risk from screening mammography of women aged 40-49 years. J Natl Cancer Inst Monogr. (1997) 1997:119–24. doi: 10.1093/jncimono/1997.22.119
56. Boone JM, Nelson TR, Lindfors KK, Seibert JA. Dedicated breast CT: radiation dose and image quality evaluation. Radiology. (2001) 221:657–67. doi: 10.1148/radiol.2213010334
57. Hooley RJ, Durand MA, Philpotts LE. Advances in digital breast tomosynthesis. AJR Am J Roentgenol. (2017) 208:256–66. doi: 10.2214/AJR.16.17127
58. Lindfors KK, Boone JM, Nelson TR, Yang K, Kwan AL, Miller DF. Dedicated breast CT: initial clinical experience. Radiology. (2008) 246:725–33. doi: 10.1148/radiol.2463070410
59. Boone JM, Kwan AL, Yang K, Burkett GW, Lindfors KK, Nelson TR. Computed tomography for imaging the breast. J Mammary Gland Biol Neoplasia. (2006) 11:103–11. doi: 10.1007/s10911-006-9017-1
60. Zhao Y, Brun E, Coan P, Huang Z, Sztrokay A, Diemoz PC, et al. High-resolution, low-dose phase contrast X-ray tomography for 3D diagnosis of human breast cancers. Proc Natl Acad Sci U S A. (2012) 109:18290–4. doi: 10.1073/pnas.1204460109
61. Gillies RJ, Anderson AR, Gatenby RA, Morse DL. The biology underlying molecular imaging in oncology: from genome to anatome and back again. Clin Radiol. (2010) 65:517–21. doi: 10.1016/j.crad.2010.04.005
62. Gillies RJ, Kinahan PE, Hricak H. Radiomics: images are more than pictures, they are data. Radiology. (2016) 278:563–77. doi: 10.1148/radiol.2015151169
63. Liu Z, Wang S, Dong D, Wei J, Fang C, Zhou X, et al. The applications of radiomics in precision diagnosis and treatment of oncology: opportunities and challenges. Theranostics. (2019) 9:1303–22. doi: 10.7150/thno.30309
64. Chen M, Copley SJ, Viola P, Lu H, Aboagye EO. Radiomics and artificial intelligence for precision medicine in lung cancer treatment. Semin Cancer Biol. (2023) 93:97–113. doi: 10.1016/j.semcancer.2023.05.004
65. Cui X, Wang N, Zhao Y, Chen S, Li S, Xu M, et al. Preoperative prediction of axillary lymph node metastasis in breast cancer using radiomics features of DCE-MRI. Sci Rep. (2019) 9:2240. doi: 10.1038/s41598-019-38502-0
66. Cheng Y, Xu S, Wang H, Wang X, Niu S, Luo Y, et al. Intra- and peri-tumoral radiomics for predicting the sentinel lymph node metastasis in breast cancer based on preoperative mammography and MRI. Front Oncol. (2022) 12:1047572. doi: 10.3389/fonc.2022.1047572
67. Fan M, Zhang P, Wang Y, Peng W, Wang S, Gao X, et al. Radiomic analysis of imaging heterogeneity in tumours and the surrounding parenchyma based on unsupervised decomposition of DCE-MRI for predicting molecular subtypes of breast cancer. Eur Radiol. (2019) 29:4456–67. doi: 10.1007/s00330-018-5891-3
68. Leithner D, Bernard-Davila B, Martinez DF, Horvat JV, Jochelson MS, Marino MA, et al. Radiomic signatures derived from diffusion-Weighted imaging for the assessment of breast cancer receptor status and molecular subtypes. Mol Imaging Biol. (2020) 22:453–61. doi: 10.1007/s11307-019-01383-w
69. Bian T, Wu Z, Lin Q, Mao Y, Wang H, Chen J, et al. Evaluating tumor-infiltrating lymphocytes in breast cancer using preoperative MRI-based radiomics. J Magn Reson Imaging. (2022) 55:772–84. doi: 10.1002/jmri.27910
70. Han X, Cao W, Wu L, Liang C. Radiomics assessment of the tumor immune microenvironment to predict outcomes in breast cancer. Front Immunol. (2021) 12:773581. doi: 10.3389/fimmu.2021.773581
71. Jimenez JE, Abdelhafez A, Mittendorf EA, Elshafeey N, Yung JP, Litton JK, et al. A model combining pretreatment MRI radiomic features and tumor-infiltrating lymphocytes to predict response to neoadjuvant systemic therapy in triple-negative breast cancer. Eur J Radiol. (2022) 149:110220. doi: 10.1016/j.ejrad.2022.110220
72. Zeng Q, Xiong F, Liu L, Zhong L, Cai F, Zeng X. Radiomics based on DCE-MRI for predicting response to neoadjuvant therapy in breast cancer. Acad Radiol. (2023) 30 Suppl 2:S38–49. doi: 10.1016/j.acra.2023.04.009
73. Choudhery S, Gomez-Cardona D, Favazza CP, Hoskin TL, Haddad TC, Goetz MP, et al. MRI radiomics for assessment of molecular subtype, pathological complete response, and residual cancer burden in breast cancer patients treated with neoadjuvant chemotherapy. Acad Radiol. (2022) 29 Suppl 1:S145–54. doi: 10.1016/j.acra.2020.10.020
74. Yu Y, Tan Y, Xie C, Hu Q, Ouyang J, Chen Y, et al. Development and validation of a preoperative magnetic resonance imaging radiomics-Based signature to predict axillary lymph node metastasis and disease-Free survival in patients with early-Stage breast cancer. JAMA Netw Open. (2020) 3:e2028086. doi: 10.1001/jamanetworkopen.2020.28086
75. Kim JH, Ko ES, Lim Y, Lee KS, Han BK, Ko EY, et al. Breast cancer heterogeneity: MR imaging texture analysis and survival outcomes. Radiology. (2017) 282:665–75. doi: 10.1148/radiol.2016160261
76. Zhao M, Zheng Y, Chu J, Liu Z, Dong F. Ultrasound-based radiomics combined with immune status to predict sentinel lymph node metastasis in primary breast cancer. Sci Rep. (2023) 13:16918. doi: 10.1038/s41598-023-44156-w
77. Guo X, Liu Z, Sun C, Zhang L, Wang Y, Li Z, et al. Deep learning radiomics of ultrasonography: Identifying the risk of axillary non-sentinel lymph node involvement in primary breast cancer. EBioMedicine. (2020) 60:103018. doi: 10.1016/j.ebiom.2020.103018
78. Xu R, You T, Liu C, Lin Q, Guo Q, Zhong G, et al. Ultrasound-based radiomics model for predicting molecular biomarkers in breast cancer. Front Oncol. (2023) 13:1216446. doi: 10.3389/fonc.2023.1216446
79. Yu F, Hang J, Deng J, Yang B, Wang J, Ye X, et al. Radiomics features on ultrasound imaging for the prediction of disease-free survival in triple negative breast cancer: a multi-institutional study. Br J Radiol. (2021) 94:20210188. doi: 10.1259/bjr.20210188
80. Cai L, Sidey-Gibbons C, Nees J, Riedel F, Schafgen B, Togawa R, et al. Can multi-modal radiomics using pretreatment ultrasound and tomosynthesis predict response to neoadjuvant systemic treatment in breast cancer? Eur Radiol. (2024) 34:2560–73. doi: 10.1007/s00330-023-10238-6
81. Quiaoit K, DiCenzo D, Fatima K, Bhardwaj D, Sannachi L, Gangeh M, et al. Quantitative ultrasound radiomics for therapy response monitoring in patients with locally advanced breast cancer: Multi-institutional study results. PLoS One. (2020) 15:e0236182. doi: 10.1371/journal.pone.0236182
82. Jiang M, Li CL, Luo XM, Chuan ZR, Lv WZ, Li X, et al. Ultrasound-based deep learning radiomics in the assessment of pathological complete response to neoadjuvant chemotherapy in locally advanced breast cancer. Eur J Cancer. (2021) 147:95–105. doi: 10.1016/j.ejca.2021.01.028
83. Kontos D, Winham SJ, Oustimov A, Pantalone L, Hsieh MK, Gastounioti A, et al. Radiomic phenotypes of mammographic parenchymal complexity: toward augmenting breast density in breast cancer risk assessment. Radiology. (2019) 290:41–9. doi: 10.1148/radiol.2018180179
84. Huang SY, Franc BL, Harnish RJ, Liu G, Mitra D, Copeland TP, et al. Exploration of PET and MRI radiomic features for decoding breast cancer phenotypes and prognosis. NPJ Breast Cancer. (2018) 4:24. doi: 10.1038/s41523-018-0078-2
85. Antunovic L, De Sanctis R, Cozzi L, Kirienko M, Sagona A, Torrisi R, et al. PET/CT radiomics in breast cancer: promising tool for prediction of pathological response to neoadjuvant chemotherapy. Eur J Nucl Med Mol Imaging. (2019) 46:1468–77. doi: 10.1007/s00259-019-04313-8
86. Antunovic L, Gallivanone F, Sollini M, Sagona A, Invento A, Manfrinato G, et al. (18)F]FDG PET/CT features for the molecular characterization of primary breast tumors. Eur J Nucl Med Mol Imaging. (2017) 44:1945–54. doi: 10.1007/s00259-017-3770-9
87. Ha S, Park S, Bang JI, Kim EK, Lee HY. Metabolic radiomics for pretreatment (18)F-FDG PET/CT to characterize locally advanced breast cancer: histopathologic characteristics, response to neoadjuvant chemotherapy, and prognosis. Sci Rep. (2017) 7:1556. doi: 10.1038/s41598-017-01524-7
88. Xia Z, Chen S, He M, Li B, Deng Y, Yi L, et al. Editorial: Targeting metabolism to activate T cells and enhance the efficacy of checkpoint blockade immunotherapy in solid tumors. Front Immunol. (2023) 14:1247178. doi: 10.3389/fimmu.2023.1247178
89. Zhang X, Zhang P, Cong A, Feng Y, Chi H, Xia Z, et al. Unraveling molecular networks in thymic epithelial tumors: deciphering the unique signatures. Front Immunol. (2023) 14:1264325. doi: 10.3389/fimmu.2023.1264325
90. Wang Y, Zhu H, Wang X. Prognosis and immune infiltration analysis of endoplasmic reticulum stress-related genes in bladder urothelial carcinoma. Front Genet. (2022) 13:965100. doi: 10.3389/fgene.2022.965100
91. Jin W, Yang Q, Chi H, Wei K, Zhang P, Zhao G, et al. Ensemble deep learning enhanced with self-attention for predicting immunotherapeutic responses to cancers. Front Immunol. (2022) 13:1025330. doi: 10.3389/fimmu.2022.1025330
92. Zhang J, Peng G, Chi H, Yang J, Xie X, Song G, et al. CD8 + T-cell marker genes reveal different immune subtypes of oral lichen planus by integrating single-cell RNA-seq and bulk RNA-sequencing. BMC Oral Health. (2023) 23:464. doi: 10.1186/s12903-023-03138-0
93. Keenan TE, Tolaney SM. Role of immunotherapy in triple-negative breast cancer. J Natl Compr Canc Netw. (2020) 18:479–89. doi: 10.6004/jnccn.2020.7554
94. Ahmadzadeh M, Johnson LA, Heemskerk B, Wunderlich JR, Dudley ME, White DE, et al. Tumor antigen-specific CD8 T cells infiltrating the tumor express high levels of PD-1 and are functionally impaired. Blood. (2009) 114:1537–44. doi: 10.1182/blood-2008-12-195792
95. Ren Q, Zhang P, Lin H, Feng Y, Chi H, Zhang X, et al. A novel signature predicts prognosis and immunotherapy in lung adenocarcinoma based on cancer-associated fibroblasts. Front Immunol. (2023) 14:1201573. doi: 10.3389/fimmu.2023.1201573
96. Zhang X, Zhuge J, Liu J, Xia Z, Wang H, Gao Q, et al. Prognostic signatures of sphingolipids: Understanding the immune landscape and predictive role in immunotherapy response and outcomes of hepatocellular carcinoma. Front Immunol. (2023) 14:1153423. doi: 10.3389/fimmu.2023.1153423
97. Xiong J, Chi H, Yang G, Zhao S, Zhang J, Tran LJ, et al. Revolutionizing anti-tumor therapy: unleashing the potential of B cell-derived exosomes. Front Immunol. (2023) 14:1188760. doi: 10.3389/fimmu.2023.1188760
98. Gong X, Chi H, Strohmer DF, Teichmann AT, Xia Z, Wang Q. Exosomes: A potential tool for immunotherapy of ovarian cancer. Front Immunol. (2022) 13:1089410. doi: 10.3389/fimmu.2022.1089410
99. Zhai X, Zhang H, Xia Z, Liu M, Du G, Jiang Z, et al. Oxytocin alleviates liver fibrosis via hepatic macrophages. JHEP Rep. (2024) 6:101032. doi: 10.1016/j.jhepr.2024.101032
100. Xiao J, Lin H, Liu B, Xia Z, Zhang J, Jin J. Decreased S1P and SPHK2 are involved in pancreatic acinar cell injury. biomark Med. (2019) 13:627–37. doi: 10.2217/bmm-2018-0404
101. Xiao J, Huang K, Lin H, Xia Z, Zhang J, Li D, et al. Mogroside II(E) inhibits digestive enzymes via suppression of interleukin 9/interleukin 9 receptor signalling in acute pancreatitis. Front Pharmacol. (2020) 11:859. doi: 10.3389/fphar.2020.00859
102. Zhang H, Xia T, Xia Z, Zhou H, Li Z, Wang W, et al. KIF18A inactivates hepatic stellate cells and alleviates liver fibrosis through the TTC3/Akt/mTOR pathway. Cell Mol Life Sci. (2024) 81:96. doi: 10.1007/s00018-024-05114-5
103. Deng Y, Shi M, Yi L, Naveed Khan M, Xia Z, Li X. Eliminating a barrier: Aiming at VISTA, reversing MDSC-mediated T cell suppression in the tumor microenvironment. Heliyon. (2024) 10:e37060. doi: 10.1016/j.heliyon.2024.e37060
104. Chang CH, Qiu J, O’Sullivan D, Buck MD, Noguchi T, Curtis JD, et al. Metabolic competition in the tumor microenvironment is a driver of cancer progression. Cell. (2015) 162:1229–41. doi: 10.1016/j.cell.2015.08.016
105. Zhang L, Gu S, Wang L, Zhao L, Li T, Zhao X, et al. M2 macrophages promote PD-L1 expression in triple-negative breast cancer via secreting CXCL1. Pathol Res Pract. (2024) 260:155458. doi: 10.1016/j.prp.2024.155458
106. Wong RS, Ong RJ, Lim JS. Immune checkpoint inhibitors in breast cancer: development, mechanisms of resistance and potential management strategies. Cancer Drug Resist. (2023) 6:768–87. doi: 10.20517/cdr.2023.58
107. Strati A, Adamopoulos C, Kotsantis I, Psyrri A, Lianidou E, Papavassiliou AG. Targeting the PD-1/PD-L1 signaling pathway for cancer therapy: focus on biomarkers. Int J Mol Sci. (2025) 26:1235. doi: 10.3390/ijms26031235
108. Giannopoulos S, Bozkus CC, Zografos E, Athanasiou A, Bongiovanni AM, Doulaveris G, et al. Targeting both autophagy and immunotherapy in breast cancer treatment. Metabolites. (2022) 12:966. doi: 10.3390/metabo12100966
109. Dirix LY, Takacs I, Jerusalem G, Nikolinakos P, Arkenau HT, Forero-Torres A, et al. Avelumab, an anti-PD-L1 antibody, in patients with locally advanced or metastatic breast cancer: a phase 1b JAVELIN Solid Tumor study. Breast Cancer Res Treat. (2018) 167:671–86. doi: 10.1007/s10549-017-4537-5
110. Nanda R, Chow LQ, Dees EC, Berger R, Gupta S, Geva R, et al. Pembrolizumab in patients with advanced triple-Negative breast cancer: phase Ib KEYNOTE-012 study. J Clin Oncol. (2016) 34:2460–7. doi: 10.1200/JCO.2015.64.8931
111. Adams S, Schmid P, Rugo HS, Winer EP, Loirat D, Awada A, et al. Pembrolizumab monotherapy for previously treated metastatic triple-negative breast cancer: cohort A of the phase II KEYNOTE-086 study. Ann Oncol. (2019) 30:397–404. doi: 10.1093/annonc/mdy517
112. Winer EP, Lipatov O, Im SA, Goncalves A, Munoz-Couselo E, Lee KS, et al. Pembrolizumab versus investigator-choice chemotherapy for metastatic triple-negative breast cancer (KEYNOTE-119): a randomised, open-label, phase 3 trial. Lancet Oncol. (2021) 22:499–511. doi: 10.1016/S1470-2045(20)30754-3
113. Emens LA, Cruz C, Eder JP, Braiteh F, Chung C, Tolaney SM, et al. Long-term clinical outcomes and biomarker analyses of atezolizumab therapy for patients with metastatic triple-Negative breast cancer: A phase 1 study. JAMA Oncol. (2019) 5:74–82. doi: 10.1001/jamaoncol.2018.4224
114. Kern R, Panis C. CTLA-4 expression and its clinical significance in breast cancer. Arch Immunol Ther Exp (Warsz). (2021) 69:16. doi: 10.1007/s00005-021-00618-5
115. Wei SC, Duffy CR, Allison JP. Fundamental mechanisms of immune checkpoint blockade therapy. Cancer Discovery. (2018) 8:1069–86. doi: 10.1158/2159-8290.CD-18-0367
116. Curran MA, Montalvo W, Yagita H, Allison JP. PD-1 and CTLA-4 combination blockade expands infiltrating T cells and reduces regulatory T and myeloid cells within B16 melanoma tumors. Proc Natl Acad Sci U S A. (2010) 107:4275–80. doi: 10.1073/pnas.0915174107
117. Larkin J, Chiarion-Sileni V, Gonzalez R, Grob JJ, Cowey CL, Lao CD, et al. Combined nivolumab and ipilimumab or monotherapy in untreated melanoma. N Engl J Med. (2015) 373:23–34. doi: 10.1056/NEJMoa1504030
118. Peng X, Zhao Z, Liu L, Bai L, Tong R, Yang H, et al. Targeting indoleamine dioxygenase and tryptophan dioxygenase in cancer immunotherapy: clinical progress and challenges. Drug Des Devel Ther. (2022) 16:2639–57. doi: 10.2147/DDDT.S373780
119. Schumacher TN, Schreiber RD. Neoantigens in cancer immunotherapy. Science. (2015) 348:69–74. doi: 10.1126/science.aaa4971
120. Cao Y, Chen C, Tao Y, Lin W, Wang PJP. Immunotherapy for triple-negative breast cancer. Pharmaceutics (2021) 13:2003. doi: 10.3390/pharmaceutics13122003
121. Sabel MS, Nehs MA, Su G, Lowler KP, Ferrara JL, Chang AE. Immunologic response to cryoablation of breast cancer. Breast Cancer Res Treat. (2005) 90:97–104. doi: 10.1007/s10549-004-3289-1
122. McArthur HL, Diab A, Page DB, Yuan J, Solomon SB, Sacchini V, et al. A pilot study of preoperative single-dose ipilimumab and/or cryoablation in women with early-stage breast cancer with comprehensive immune profiling. Clin Cancer Res. (2016) 22:5729–37. doi: 10.1158/1078-0432.CCR-16-0190
123. Xie H, Xi X, Lei T, Liu H, Xia Z. CD8(+) T cell exhaustion in the tumor microenvironment of breast cancer. Front Immunol. (2024) 15:1507283. doi: 10.3389/fimmu.2024.1507283
124. Page DB, Yuan J, Redmond D, Wen YH, Durack JC, Emerson R, et al. Deep sequencing of T-cell receptor DNA as a biomarker of clonally expanded TILs in breast cancer after immunotherapy. Cancer Immunol Res. (2016) 4:835–44. doi: 10.1158/2326-6066.CIR-16-0013
125. Chawla A, Philips AV, Alatrash G, Mittendorf E. Immune checkpoints: A therapeutic target in triple negative breast cancer. Oncoimmunology. (2014) 3:e28325. doi: 10.4161/onci.28325
126. Wrangle J, Wang W, Koch A, Easwaran H, Mohammad HP, Vendetti F, et al. Alterations of immune response of Non-Small Cell Lung Cancer with Azacytidine. Oncotarget. (2013) 4:2067–79. doi: 10.18632/oncotarget.v4i11
127. Tomita Y, Lee MJ, Lee S, Tomita S, Chumsri S, Cruickshank S, et al. The interplay of epigenetic therapy and immunity in locally recurrent or metastatic estrogen receptor-positive breast cancer: Correlative analysis of ENCORE 301, a randomized, placebo-controlled phase II trial of exemestane with or without entinostat. Oncoimmunology. (2016) 5:e1219008. doi: 10.1080/2162402X.2016.1219008
128. Yin J, Gu T, Chaudhry N, Davidson NE, Huang Y. Epigenetic modulation of antitumor immunity and immunotherapy response in breast cancer: biological mechanisms and clinical implications. Front Immunol. (2023) 14:1325615. doi: 10.3389/fimmu.2023.1325615
129. Majidi M, Safaee S, Amini M, Baghbanzadeh A, Hajiasgharzadeh K, Hashemzadeh S, et al. The effects of chemotherapeutic drugs on PD-L1 gene expression in breast cancer cell lines. Med Oncol. (2021) 38:147. doi: 10.1007/s12032-021-01556-0
130. Adams S, Diamond JR, Hamilton E, Pohlmann PR, Tolaney SM, Chang CW, et al. Atezolizumab plus nab-Paclitaxel in the treatment of metastatic triple-Negative breast cancer with 2-Year survival follow-up: A phase 1b clinical trial. JAMA Oncol. (2019) 5:334–42. doi: 10.1001/jamaoncol.2018.5152
131. Nanda R, Liu MC, Yau C, Shatsky R, Pusztai L, Wallace A, et al. Effect of pembrolizumab plus neoadjuvant chemotherapy on pathologic complete response in women with early-Stage breast cancer: an analysis of the ongoing phase 2 adaptively randomized I-SPY2 trial. JAMA Oncol. (2020) 6:676–84. doi: 10.1001/jamaoncol.2019.6650
132. Cortes J, Cescon DW, Rugo HS, Nowecki Z, Im SA, Yusof MM, et al. Pembrolizumab plus chemotherapy versus placebo plus chemotherapy for previously untreated locally recurrent inoperable or metastatic triple-negative breast cancer (KEYNOTE-355): a randomised, placebo-controlled, double-blind, phase 3 clinical trial. Lancet. (2020) 396:1817–28. doi: 10.1016/S0140-6736(20)32531-9
133. Deng L, Liang H, Burnette B, Beckett M, Darga T, Weichselbaum RR, et al. Irradiation and anti-PD-L1 treatment synergistically promote antitumor immunity in mice. J Clin Invest. (2014) 124:687–95. doi: 10.1172/JCI67313
134. Santa-Maria CA, Nanda R. Immune checkpoint inhibitor therapy in breast cancer. J Natl Compr Canc Netw. (2018) 16:1259–68. doi: 10.6004/jnccn.2018.7046
135. Ho AY, Tabrizi S, Dunn SA, McArthur HL. Current advances in immune checkpoint inhibitor combinations with radiation therapy or cryotherapy for breast cancer. Breast Cancer Res Treat. (2022) 191:229–41. doi: 10.1007/s10549-021-06408-z
136. Salemme V, Centonze G, Cavallo F, Defilippi P, Conti L. The crosstalk between tumor cells and the immune microenvironment in breast cancer: implications for immunotherapy. Front Oncol. (2021) 11:610303. doi: 10.3389/fonc.2021.610303
137. Pantelidou C, Sonzogni O, De Oliveria Taveira M, Mehta AK, Kothari A, Wang D, et al. PARP inhibitor efficacy depends on CD8(+) T-cell recruitment via intratumoral STING pathway activation in BRCA-deficient models of triple-negative breast cancer. Cancer Discovery. (2019) 9:722–37. doi: 10.1158/2159-8290.CD-18-1218
138. Jiao S, Xia W, Yamaguchi H, Wei Y, Chen MK, Hsu JM, et al. PARP inhibitor upregulates PD-L1 expression and enhances cancer-associated immunosuppression. Clin Cancer Res. (2017) 23:3711–20. doi: 10.1158/1078-0432.CCR-16-3215
139. Vinayak S, Tolaney SM, Schwartzberg L, Mita M, McCann G, Tan AR, et al. Open-label clinical trial of niraparib combined with pembrolizumab for treatment of advanced or metastatic triple-Negative breast cancer. JAMA Oncol. (2019) 5:1132–40. doi: 10.1001/jamaoncol.2019.1029
140. Kwapisz D. Pembrolizumab and atezolizumab in triple-negative breast cancer. Cancer Immunol Immunother. (2021) 70:607–17. doi: 10.1007/s00262-020-02736-z
141. Loi S, Dushyanthen S, Beavis PA, Salgado R, Denkert C, Savas P, et al. RAS/MAPK activation is associated with reduced tumor-infiltrating lymphocytes in triple-negative breast cancer: therapeutic cooperation between MEK and PD-1/PD-L1 immune checkpoint inhibitors. Clin Cancer Res. (2016) 22:1499–509. doi: 10.1158/1078-0432.CCR-15-1125
142. Xia L, Zheng ZZ, Liu JY, Chen YJ, Ding JC, Xia NS, et al. EGFR-targeted CAR-T cells are potent and specific in suppressing triple-negative breast cancer both in vitro and in vivo. Clin Transl Immunol. (2020) 9:e01135. doi: 10.1002/cti2.1135
143. Wei H, Wang Z, Kuang Y, Wu Z, Zhao S, Zhang Z, et al. Intercellular adhesion molecule-1 as target for CAR-T-Cell therapy of triple-Negative breast cancer. Front Immunol. (2020) 11:573823. doi: 10.3389/fimmu.2020.573823
144. Tchou J, Zhao Y, Levine BL, Zhang PJ, Davis MM, Melenhorst JJ, et al. Safety and efficacy of intratumoral injections of chimeric antigen receptor (CAR) T cells in metastatic breast cancer. Cancer Immunol Res. (2017) 5:1152–61. doi: 10.1158/2326-6066.CIR-17-0189
145. Hu Z. Tissue factor as a new target for CAR-NK cell immunotherapy of triple-negative breast cancer. Sci Rep. (2020) 10:2815. doi: 10.1038/s41598-020-59736-3
146. Xu Y, Yang Z, Horan LH, Zhang P, Liu L, Zimdahl B, et al. A novel antibody-TCR (AbTCR) platform combines Fab-based antigen recognition with gamma/delta-TCR signaling to facilitate T-cell cytotoxicity with low cytokine release. Cell Discovery. (2018) 4:62. doi: 10.1038/s41421-018-0066-6
147. Ho HY, Chung KK, Kan CM, Wong SC. Liquid biopsy in the clinical management of cancers. Int J Mol Sci. (2024) 25:8594. doi: 10.3390/ijms25168594
148. Verma R, Rao L, Nagpal D, Yadav M, Kumar V, Kumar V, et al. Emerging nanotechnology-based therapeutics: A new insight into promising drug delivery system for lung cancer therapy. Recent Pat Nanotechnol. (2024) 18:395–414. doi: 10.2174/1872210517666230613154847
Keywords: breast cancer, PD-1, immune checkpoint, immunotherapy, radiotherapy, imaging diagnosis
Citation: He J, Liu N and Zhao L (2025) New progress in imaging diagnosis and immunotherapy of breast cancer. Front. Immunol. 16:1560257. doi: 10.3389/fimmu.2025.1560257
Received: 14 January 2025; Accepted: 03 March 2025;
Published: 17 March 2025.
Edited by:
Dan Liu, Wuhan University, ChinaReviewed by:
Benhua Li, First Affiliated Hospital of Chongqing Medical University, ChinaCopyright © 2025 He, Liu and Zhao. This is an open-access article distributed under the terms of the Creative Commons Attribution License (CC BY). The use, distribution or reproduction in other forums is permitted, provided the original author(s) and the copyright owner(s) are credited and that the original publication in this journal is cited, in accordance with accepted academic practice. No use, distribution or reproduction is permitted which does not comply with these terms.
*Correspondence: Li Zhao, MjY5OTcyNjA1QHFxLmNvbQ==
Disclaimer: All claims expressed in this article are solely those of the authors and do not necessarily represent those of their affiliated organizations, or those of the publisher, the editors and the reviewers. Any product that may be evaluated in this article or claim that may be made by its manufacturer is not guaranteed or endorsed by the publisher.
Research integrity at Frontiers
Learn more about the work of our research integrity team to safeguard the quality of each article we publish.