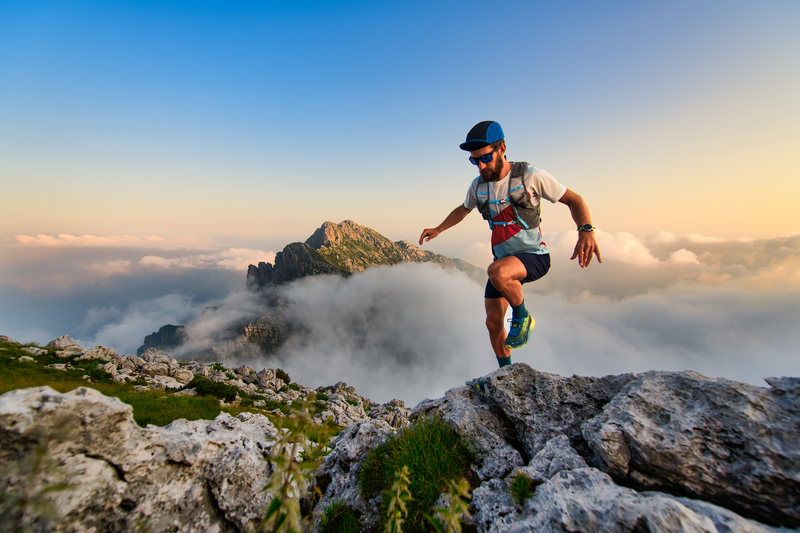
94% of researchers rate our articles as excellent or good
Learn more about the work of our research integrity team to safeguard the quality of each article we publish.
Find out more
ORIGINAL RESEARCH article
Front. Immunol.
Sec. Cancer Immunity and Immunotherapy
Volume 16 - 2025 | doi: 10.3389/fimmu.2025.1559200
This article is part of the Research Topic Cellular Metabolism, the Immune System, and Oncogenesis: Opportunities for Drug Discovery and Development View all 3 articles
The final, formatted version of the article will be published soon.
You have multiple emails registered with Frontiers:
Please enter your email address:
If you already have an account, please login
You don't have a Frontiers account ? You can register here
Breast cancer is a heterogeneous and complex disease with multiple subtypes.Neoadjuvant therapy, administered prior to surgery, aims to shrink tumor size, improve surgical outcomes, and assess tumor response to treatment. Current research on the efficacy of neoadjuvant therapy and its impact on breast cancer subtypes is crucial for precision medicine and personalized treatment. This project leverages multimodal breast cancer data, integrating transcriptomics, proteomics, MRI imaging, and clinical information to develop a diagnostic model for neoadjuvant therapy in breast cancer. Based on these multimodal data, we identified four distinct multimodal breast cancer subtypes with significant prognostic differences. We compared the molecular pathological characteristics of these subtypes at multiple levels and explored the tumor microenvironment, cell-cell communication, and tumor progression differences at the single-cell level. Additionally, we investigated the molecular mechanisms underlying the differential drug response of malignant cells within four multimodal breast cancer subtypes. This study introduces new approaches for multimodal diagnosis of neoadjuvant therapy efficacy in breast cancer, providing valuable evidence and support for future precision and personalized treatments.
Keywords: BRCA, Multimodal Diagnostic Model, Neoadjuvant Therapy, Heterogeneous disease, subtypes, Tumor Microenvironment
Received: 12 Jan 2025; Accepted: 26 Feb 2025.
Copyright: © 2025 Ye, Yuan, Hong, Xu and Liu. This is an open-access article distributed under the terms of the Creative Commons Attribution License (CC BY). The use, distribution or reproduction in other forums is permitted, provided the original author(s) or licensor are credited and that the original publication in this journal is cited, in accordance with accepted academic practice. No use, distribution or reproduction is permitted which does not comply with these terms.
* Correspondence:
Peng Xu, Guangzhou University, Guangzhou, China
Wenbin Liu, Guangzhou University, Guangzhou, China
Disclaimer: All claims expressed in this article are solely those of the authors and do not necessarily represent those of their affiliated organizations, or those of the publisher, the editors and the reviewers. Any product that may be evaluated in this article or claim that may be made by its manufacturer is not guaranteed or endorsed by the publisher.
Research integrity at Frontiers
Learn more about the work of our research integrity team to safeguard the quality of each article we publish.