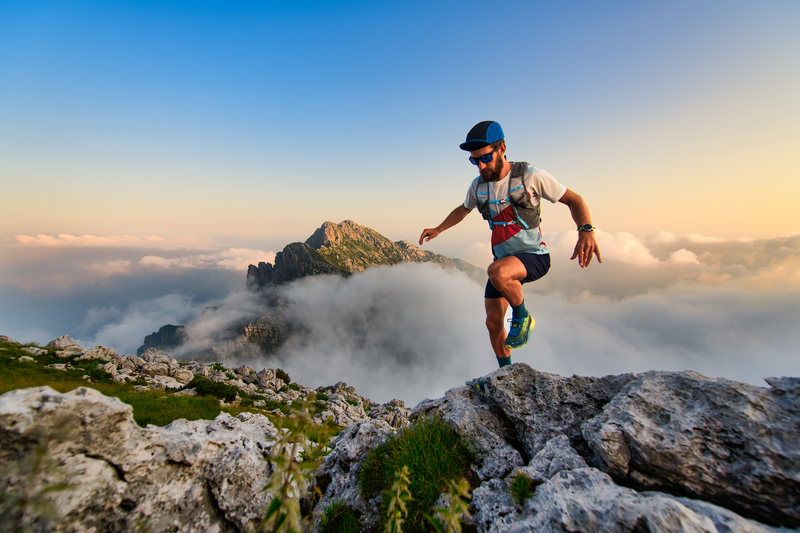
94% of researchers rate our articles as excellent or good
Learn more about the work of our research integrity team to safeguard the quality of each article we publish.
Find out more
ORIGINAL RESEARCH article
Front. Immunol.
Sec. Cancer Immunity and Immunotherapy
Volume 16 - 2025 | doi: 10.3389/fimmu.2025.1558496
This article is part of the Research Topic The Role of Neutrophil Extra Cellular Traps (NETs) Formation in Tumor Microenvironment- from Basic Research to Clinical Applications View all articles
The final, formatted version of the article will be published soon.
You have multiple emails registered with Frontiers:
Please enter your email address:
If you already have an account, please login
You don't have a Frontiers account ? You can register here
BackgroundNeutrophil extracellular traps (NETs) play pivotal roles in various pathological processes. The formation of NETs is impaired in acute myeloid leukemia (AML), which can result in immunodeficiency and increased susceptibility to infection. MethodsThe gene set variation analysis (GSVA) algorithm was employed for the calculation of NET score, while the consensus clustering algorithm was utilized to identify molecular subtypes. Weighted gene coexpression network analysis (WGCNA) revealed potential genes and biological pathways associated with NETs, and a total of 10 machine learning algorithms were applied to construct the optimal prognostic model.ResultsThrough the analysis of multiomics data, we identified two molecular subtypes with high and low NET scores. The low-NET score subgroup exhibited increased infiltration of immune effector cells. Conversely, the high-NET score subtype presented an abundance of monocytes and M2 macrophages, accompanied by elevated expression levels of immune checkpoint genes. These findings suggest that a pronounced immunosuppressive effect is associated with a significantly worse prognosis for this subtype. The optimal risk score model was selected by employing the C-index as the criterion on the basis of training 10 machine learning algorithms on 9 multicenter AML cohorts. Survival analysis confirmed that patients with high-risk scores had considerably poorer prognoses than those with lower scores. Receiver operating characteristic (ROC) curve and Cox regression analyses further validated the strong independent prognostic value of the risk score model. The nomogram, which was constructed by integrating the risk score model and clinicopathological factors, demonstrated high accuracy in predicting the overall survival of AML patients. Moreover, patients with refractory or chemotherapy-unresponsive AML had significantly higher risk scores. By analyzing drug therapy data from in vitro AML cells, we identified a subset of drugs that demonstrated increased sensitivity in the high-risk score group. Additionally, patients with a high risk score were also predicted to exhibit a favorable response to anti-PD-1 therapy, suggesting that these individuals may derive greater benefits from immunotherapy. ConclusionThe NET-related signature, derived from a combination of diverse machine learning algorithms, has promising potential as a valuable tool for prognostic prediction, preventive measures, and personalized medicine in patients with AML.
Keywords: Conceptualization, Supervision, and Project administration, F.Z., B.H., X.W., J.L., F.Y.: Funding acquisition, F.Z., and X.W.: Resources, F.Z., F.Y., Z.W., and J.L.: Validation and Visualization, F.Z.: Methodology, F.Z.: Data Curation, F.Z.: Formal analysis, F.Z.: Software
Received: 10 Jan 2025; Accepted: 10 Feb 2025.
Copyright: © 2025 Zhong, Yao, Zihao, Liu, Huang and Wang. This is an open-access article distributed under the terms of the Creative Commons Attribution License (CC BY). The use, distribution or reproduction in other forums is permitted, provided the original author(s) or licensor are credited and that the original publication in this journal is cited, in accordance with accepted academic practice. No use, distribution or reproduction is permitted which does not comply with these terms.
* Correspondence:
Bo Huang, Department of Clinical Laboratory, The Second Affiliated Hospital, Jiangxi Medical College, Nanchang University, Nanchang, China
Xiaozhong Wang, Department of Clinical Laboratory, The Second Affiliated Hospital, Jiangxi Medical College, Nanchang University, Nanchang, China
Disclaimer: All claims expressed in this article are solely those of the authors and do not necessarily represent those of their affiliated organizations, or those of the publisher, the editors and the reviewers. Any product that may be evaluated in this article or claim that may be made by its manufacturer is not guaranteed or endorsed by the publisher.
Research integrity at Frontiers
Learn more about the work of our research integrity team to safeguard the quality of each article we publish.