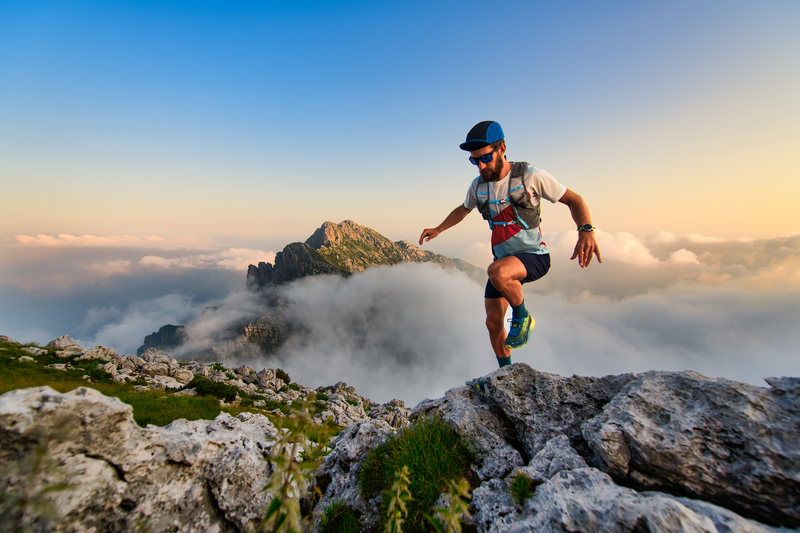
94% of researchers rate our articles as excellent or good
Learn more about the work of our research integrity team to safeguard the quality of each article we publish.
Find out more
ORIGINAL RESEARCH article
Front. Immunol.
Sec. Inflammation
Volume 16 - 2025 | doi: 10.3389/fimmu.2025.1558139
This article is part of the Research Topic Characterizing the Functional Heterogeneity of Synovial Macrophages View all articles
The final, formatted version of the article will be published soon.
You have multiple emails registered with Frontiers:
Please enter your email address:
If you already have an account, please login
You don't have a Frontiers account ? You can register here
Background Osteoarthritis (OA) is a chronic joint disease that significantly impairs quality of life. Synovitis plays a pivotal role in OA progression, and pyroptosis, a form of programmed cell death associated with innate immune inflammation, may contribute to the pathogenesis of OA synovitis. Nevertheless, the precise role of pyroptosis in OA pathogenesis remains poorly understood.We performed an analysis of bulk RNA sequencing data to examine the expression profiles of pyroptosis-related genes in the OA synovium. A LASSO-Cox regression model was employed to identify pivotal genes. Single-cell RNA sequencing data were used to validate the expression of these genes in specific synovial cell clusters.Differentially expressed genes (DEGs) in macrophages with high or low expression levels of core genes were subjected to enrichment analysis. A protein-protein interaction (PPI) network was constructed to identify hub genes, and potential therapeutic compounds were predicted. Consensus clustering analysis was performed to examine the correlations between hub genes and disease status. After identifying PYCARD as the core pyroptosis gene in OA macrophages, we assessed the expression levels of PYCARD in the OA synovium and validated the expression of PYCARD and its related core genes in M1 macrophages.Results A total of twenty pyroptosis-related DEGs were identified, and six core genes were selected through LASSO regression. PYCARD was identified as the key pyroptosis gene in macrophages. Furthermore, 57 therapeutic compounds targeting these genes were predicted. Validation confirmed the upregulation of PYCARD in the OA synovium and M1 macrophages.Conclusion PYCARD was identified as the core pyroptosis gene in OA macrophages, 2 and 57 potential therapeutic compounds were identified. This study offers valuable insights into potential treatment targets for OA.
Keywords: pyroptosis, Inflammation, Pycard, Osteoarthritis, Synovial macrophages
Received: 09 Jan 2025; Accepted: 10 Mar 2025.
Copyright: © 2025 Yao, Yuexin, Mai, Wang, Zhang, Cai and Xiangjiang. This is an open-access article distributed under the terms of the Creative Commons Attribution License (CC BY). The use, distribution or reproduction in other forums is permitted, provided the original author(s) or licensor are credited and that the original publication in this journal is cited, in accordance with accepted academic practice. No use, distribution or reproduction is permitted which does not comply with these terms.
* Correspondence:
Haiyan Zhang, Third Affiliated Hospital, Southern Medical University, Guangzhou, Guangdong Province, China
Wang Xiangjiang, Qingyuan People's Hospital, Qingyuan, China
Disclaimer: All claims expressed in this article are solely those of the authors and do not necessarily represent those of their affiliated organizations, or those of the publisher, the editors and the reviewers. Any product that may be evaluated in this article or claim that may be made by its manufacturer is not guaranteed or endorsed by the publisher.
Research integrity at Frontiers
Learn more about the work of our research integrity team to safeguard the quality of each article we publish.