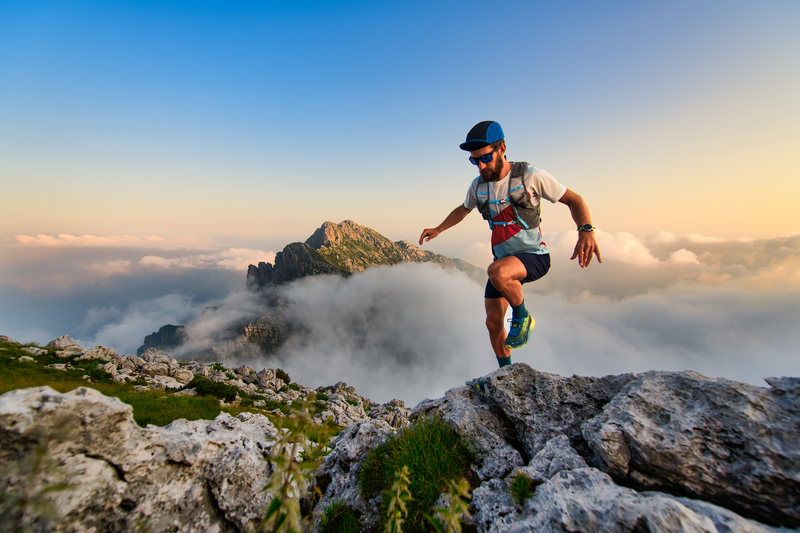
94% of researchers rate our articles as excellent or good
Learn more about the work of our research integrity team to safeguard the quality of each article we publish.
Find out more
ORIGINAL RESEARCH article
Front. Immunol.
Sec. Microbial Immunology
Volume 16 - 2025 | doi: 10.3389/fimmu.2025.1556857
This article is part of the Research Topic Deciphering Host-Pathogen Interactions in Tuberculosis: Implications for Diagnostics and Therapeutics View all 3 articles
The final, formatted version of the article will be published soon.
You have multiple emails registered with Frontiers:
Please enter your email address:
If you already have an account, please login
You don't have a Frontiers account ? You can register here
Blood inflammatory status is closely associated with tuberculosis (TB) progression. Emerging inflammatory indices from different leukocyte subtypes have become a prognostic hotspot for various diseases, yet their application in TB prognosis remains limited. This study aims to assess the impact of inflammatory status on TB patients' prognosis and its potential as a prognostic indicator to optimize prognostic assessment and therapeutic strategies.This study included 4027 TB patients admitted to a tuberculosis-designated hospital in Shenzhen from January 2017 to December 2022. Patients were classified into three inflammatory statuses (Q1-Q3) based on each index's level. We conducted Cox regression and restricted cubic splines (RCS) analyses to evaluate the association between inflammatory status and unfavorable outcome, subgroup analyses to understand heterogeneous associations among subpopulations, and receiver operating characteristic (ROC) analyses to evaluate the prognostic performance of inflammatory status on TB treatment outcomes.During 48991.79 person-months of follow-up involving 4027 patients, 225 unfavorable outcomes occurred. Multivariable Cox regression indicated that the Q3 levels of CAR, CLR, dNLR, NLR, SII, and SIRI increased the risk of unfavorable outcome by 45%-99% (HR: 1.45-1.99, all P<0.050), whereas ENR reduced the risk by 29% (HR: 0.71, P=0.040) compared to Q1. RCS curves revealed linear associations with unfavorable outcome that were positive for CAR, CLR, dNLR, SII, and SIRI, negative for ENR (all P for nonlinear>0.050), and nonlinear for MLR, NLR, and PNI (all P for nonlinear<0.050). Subgroup analyses identified heterogeneous associations across age, sex, BMI, comorbidities, and drug resistance (all P for interaction<0.050), with attenuated risk effects of CAR, CLR, dNLR, and SII in patients aged 30-60 years, male, BMI≥24.0 kg/m², smokers, retreatment cases, and those with tumor. ROC analysis demonstrated stable predictive performances of inflammatory status (AUC: 0.785-0.804 at 6-month, 0.781-0.793 at 9-month, and 0.762-0.773 at 12-month), and the combination of the inflammatory status significantly optimized the prognostic performance of the basic model (9-month AUC: 0.811 vs 0.780, P=0.024; 12-month AUC: 0.794 vs 0.758, P=0.013).Pretreatment blood inflammatory status effectively predicts the treatment outcome of TB patients. Our findings hold significant clinical value for TB patient management and warrant prospective evaluation in future studies.
Keywords: blood inflammatory status, Inflammation, Tuberculosis, prognosis, survival analysis, cohort study
Received: 07 Jan 2025; Accepted: 03 Mar 2025.
Copyright: © 2025 Ji, Xie, Wei, Huang, Liu, Ye, Liu, Lu, Lv, Hou, Zhang, Xu, Yuan, Lu and Yang. This is an open-access article distributed under the terms of the Creative Commons Attribution License (CC BY). The use, distribution or reproduction in other forums is permitted, provided the original author(s) or licensor are credited and that the original publication in this journal is cited, in accordance with accepted academic practice. No use, distribution or reproduction is permitted which does not comply with these terms.
* Correspondence:
JianHui Yuan, Nanshan District Center for Disease Control and Prevention, Shenzhen, China
Shuihua Lu, National Clinical Research Center for Infectious Disease, Shenzhen, 518112, China
Chongguang Yang, School of Public Health (Shenzhen), Shenzhen Key Laboratory of Pathogenic Microbes and Biosafety, Shenzhen Campus of Sun Yat-sen University, Shenzhen, China
Disclaimer: All claims expressed in this article are solely those of the authors and do not necessarily represent those of their affiliated organizations, or those of the publisher, the editors and the reviewers. Any product that may be evaluated in this article or claim that may be made by its manufacturer is not guaranteed or endorsed by the publisher.
Research integrity at Frontiers
Learn more about the work of our research integrity team to safeguard the quality of each article we publish.