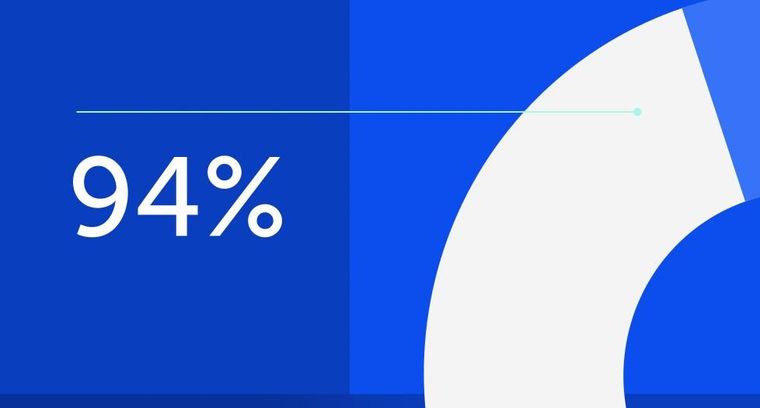
94% of researchers rate our articles as excellent or good
Learn more about the work of our research integrity team to safeguard the quality of each article we publish.
Find out more
ORIGINAL RESEARCH article
Front. Immunol., 11 April 2025
Sec. Cancer Immunity and Immunotherapy
Volume 16 - 2025 | https://doi.org/10.3389/fimmu.2025.1555530
This article is part of the Research TopicThymus Biology: Development, Immunodeficiency and Cancer ProgressionView all 4 articles
Background: High expression levels of programmed death receptor 1 (PD-1) and its ligand 1 (PD-L1) have been observed in thymic epithelial tumors (TET), suggesting their potential as prognostic indicators for disease progression and the effectiveness of immunotherapy in TET. The conventional method obtaining PD-L1 was challenging due to invasive sampling and tumor heterogeneity
Methods: A total of 124 patients with pathologically confirmed TET (57 PD-L1 positive, 67 PD-L1 negative) were retrospectively enrolled and allocated into training and validation cohorts in a ratio of 7:3. Radiomics features were extracted from T1-weighted, T2-weighted fat suppression, and apparent diffusion coefficient (ADC) map images to establish a radiomics signature in the training cohort. Multivariate logistic regression analysis was conducted to develop a combined radiomics nomogram that incorporated clinical, conventional MR features, or ADC model for evaluation purposes. The performance of each model was compared using receiver operating characteristics analysis, while discrimination, calibration, and clinical efficiency of the combined radiomics nomogram were assessed.
Results: The radiomics signature, consisting of four features, demonstrated a favorable ability to predict and differentiate between PD-L1 positive and negative TET patients. The combined radiomics nomogram, which incorporates the peri-cardial invasion sign, ADC value, WHO classification, and radiomics signature, showed excellent performance (training cohort: area under the curve [AUC] = 0.903; validation cohorts: AUC = 0.894). The calibration curve and decision curve analysis further confirmed the clinical usefulness of this combined model. The decision curve analysis demonstrated the clinical utility of the integrated radiomics nomogram.
Conclusions: The radiomics signature serves as a valuable tool for predicting the PD-L1 status of TET patients. Furthermore, the integration of radiomics nomogram enhances the personalized prediction capability.
Thymic epithelial tumors (TET) are the most common anterior mediastinum neoplasms in adults (1, 2). Recently, programmed death receptor 1 (PD-1) and its ligand 1 (PD-L1) based immune checkpoint inhibitors (ICIs) show promising prospects in TET treatment. Additionally, PD-L1 has been proved as a predictor of the response to TET immunotherapy (2). TETs express PD-1/PD-L1 at high levels, which differs between different Masaoka stages and pathological subtypes (3–5). Furthermore, accumulating evidence has revealed high PD-1/PD-L1 expression in TET is worse prognosis (6). Therefore, accurate prediction of PD-L1 expression for TET patient is crucial. The common method to analysis PD-L1 expression is immunohistochemistry (IHC). However, IHC way is time consuming and does not provide a real-time detection (7). Thus, exploring a new approach to predict the expression of PD-L1 level for TETs may be clinical significance.
Radiomics methods have been widely applied with the extraction of numerous quantitative metrics on the entire tumor from radiological images including computed tomography (CT) and magnetic resonance image (MRI) (8, 9). Existing studies recognize the critical role of radiomics in reflecting tissue heterogeneity, staging and risk stratification of TET (10–12). Xiao et al. (10) using a combined radiomics nomogram incorporating tumor shape, apparent diffusion coefficient (ADC) value and radiomics signature differentiating different TET histologic subtypes. Mayoral et al. (11) applied radiomic features, conventional characteristics and both based on CT to differentiate thymoma and thymic carcinoma. A study compared 5 different models based on 13 representative features and a radiomics nomogram combining the selected clinical variables and radiomics signature to predict thymoma risk categorization (12). Besides, radiomics has been successfully employed to predict the level of PD-L1 in lung and esophageal tumors (13, 14). Zhang et al. (15) also reported that MRI radiomics could derive promising biomarker in discriminating PD-1/PD-L1 expression in intrahepatic cholangiocarcinoma.
In this study, we aimed to investigate the potential of multiparametric-MRI based radiomics model in evaluating the expression of PD-1/PD-L1 for TET patients.
The ethical approval for this retrospective study was obtained from the Ethics Committee of our institute, and the requirement for written informed consent was waived. All radiological data and relevant clinical information were reviewed between February 2019 and March 2023. Patients included in this study met the following criteria: 1) confirmed pathological diagnosis of TET through CT-guided fine needle biopsy or surgery; 2) underwent mediastinum MRI with PD-L1 expression level determined by IHC test within one week interval. Patients with the following characteristics were excluded: 1) patients with a history of previous treatment for TET; 2) poor MRI image quality for further analysis. In total, a cohort of 124 TET patients with available PD-L1 results were enrolled in this study. The flowchart illustrating patient enrollment and analysis scheme is presented in Figure 1.
PD-L1 expression levels were detected using the IHC method in this study. PD-L1 expression in tumors was determined by the PD-L1 22C3 pharmDx antibody and characterized by the Combined Positive Score (CPS), which is defined as the sum of PD-L1-positive cells (tumor cells and tumor-associated immune cells) per 100 tumor cells. The CPS cut-off values varied for different cancers, with a CPS of ≥1 defining positivity and a CPS of <1 defining negativity for PD-L1 expression (16). Two pathologists independently evaluated the PD-L1 expression status, with any inconsistent results being further analyzed by a senior pathologist.
All the MRI scans were performed using a 3.0 T MR scanner (MAGNETOM Skyra, Siemens Healthcare, Erlangen, Germany) with a 16-channel torso coil. The following sequences were used: 1) axial T1-weighted imaging with time of repetition [TR]/time of echo [TE], 140/2.5 ms; 2) axial T2-weighted imaging with fat suppression (TR/TE, 1200/93 ms). 3) axial diffusion weighted imaging (DWI) using an echo planar imaging (TR/TE, 4500/63 ms; b value, 0 and 1000 s/mm2), and corresponding ADC maps; 4) Axial dynamic contrast enhanced MR imaging was performed using a T1-weighed volumetric interpolated breath-hold examination with radial acquisition trajectory. Gadolinium-diethylene triamine pentacetic acid (Magnevist; Bayer Schering Pharma AG, Berlin, Germany) was intravenously bolus injected via a power injector at the rate of 4.0 mL/s at the dose of 0.1 mmol/kg, followed by a 20-mL bolus of saline administered at the same injection rate.
The conventional image features and ADC measurement were independently evaluated by two radiologists with 7 and 12 years of experience in chest radiology, respectively. In case of disagreements, a third senior chest radiologist with 30 years of experience was consulted to make the final decision. The evaluation of conventional features included: 1) presence or absence of cystic component; 2) presence or absence of internal septal; 3) presence or absence of pleural invasion; 4) presence or absence of pericardial invasion; and 5) measurement of ADC values. The evaluation of conventional MRI features and ADC measurements are shown in Supplementary Method S1 and S2. All MR images were analyzed using a picture archiving and communication system (Vue PACS, version 12.1.5, Carestream Health, Rochester, NY).
The radiomics signature workflow is presented in Figure 2. The volume of interest (VOI) encompassing the tumor was manually delineated along the tumor contour on axial T2WI fat-suppression, axial T1WI without contrast enhanced, and ADC map images using ITK-SNAP software (version 4.0.0; University of Pennsylvania, Philadelphia, USA, http://www.itksnap.org/). This segmentation process included the cystic component of the tumor as well. Two experienced chest radiologists (with 7 and 11 years of experience respectively) completed the segmentation process. In case of any disagreement in determining the tumor mask, a final discussion was conducted to reach a consensus. Radiomics features were extracted from these ROIs using an in-house Python software (Pyradiomics version 2.12; http://pyradiomics.readthedocs.io/en/2.1.2/). A total of 788 features were extracted from T2WI fat-suppression, T1WI, and ADC map images individually. These radiomics features comprised four groups: Shape (n=14), first-order statistics (n=18), textural features (n=68), and wavelet features (n=688). The textural features consisted of Gray Level Run Length Matrix (GLRLM), Gray Level Size Zone Matrix (GLSZM), Gray Level Co-occurrence Matrix(GLCM), and Gray Level Dependence Matrix(GLDM) features. The detailed information regarding feature extraction is provided in Supplementary Table S1.
To ensure the stability of radiomics features extraction, a cohort of 20 TETs was randomly selected for a repeat segmentation. The first reader repeated the tumor segmentation after a week, while the second reader independently performed the segmentation to assess intra- and inter-class consistency.
All enrolled patients were randomly divided into a training cohort and a validation cohort at a ratio of 7:3. To address the variations in index dimensions of the data, the radiomics features extracted were normalized to standard normal distribution using z-scores. Consequently, a three-stage methodology was devised to reduce dimensionality and ascertain resilient attributes. The features selected for further analysis were those exhibiting high stability, as indicated by intra- and inter-class correlation coefficient values exceeding 0.80 (17). Furthermore, Pearson’s correction analysis was utilized to identify redundant and collinear features. Features exhibiting mutual correlation coefficients greater than 0.9 were subsequently excluded (18). In the third step of the analysis, we used the least absolute shrinkage and selection operator (LASSO) regression method to determine which features were the most closely associated with positive for PD-L1 expression. To ensure optimal performance, we employed 10-fold cross-validation for penalty parameter tuning. Thus, the resulting radiomics signature was created by combining these selected features in a linear fashion and weighting them according to their respective coefficients. This allowed us to calculate the corresponding Rad-score for each patient (18).
To assess the stability of radiomics feature extraction, a cohort of 20 PD-L1 positive patients were randomly selected for repeat segmentation. Reader 1 performed tumor segmentation twice within one week, while Reader 2 independently conducted the segmentation to calculate intra- and inter-correlation coefficients (ICC).
We employed statistical tests (chi-squared for categorial variables and Wilcoxon for continuous variables) with a significant level of p < 0.05 to identify significant clinical features in the training group, as highlighted in Table 1. Subsequently, we performed univariate logistic regression analysis to assess the discriminatory ability of these features between two groups at a significance level of p < 0.05. Finally, employing backward stepwise multivariate logistic regression analysis, we identified independent predictive risk factors and constructed a predictive nomogram that incorporated both discriminative clinical features and Rad-score. Additionally, collinearity analysis using variance inflation factor (VIF) was conducted, leading to the removal of factors with VIF >10.
The accuracy of the radiomics signature was initially evaluated in the training cohort using the area under the curve (AUC) of the receiver operating characteristic curve (ROC), and subsequently validated in an independent validation cohort. To enhance the clinical applicability of our model, we incorporated patients’ clinical characteristics and constructed a nomogram-based risk scoring system. Details regarding the calibration curve and decision curve analysis (DCA) of the combined radiomics nomogram are shown in Supplementary Method S3.
The chi-square test or Fisher exact test was employed to compare categorical variables, as appropriate. For the analysis of continuous variables, either the student’s t-test or Mann-Whitney U test was utilized. Statistical analyses were performed using SPSS (version 22.0; IBM) and R software (Version 3.5.1; http://www.Rproject.org). All tests were two-tailed, and a significance level of p<0.05 was considered statistically significant. Univariable and multivariable logistic regression analyses were employed to identify significant risk factors. Following the multivariable analysis, the remaining variables were considered as potential risk factors and included in the clinical modeling of the training cohort. The DCA were employed to assess the clinical impact of the radiomics model, clinical model, and radiomics-clinical model in the training cohort using the “dca.R” package. The Delong non-parametric test was conducted to assess the statistical significance of the differences in AUC values among different models.
The comparation for the differentiation of baseline characteristics of both the training and validation cohorts are presented in Table 1. The study population comprised 67 males and 57 females with a mean age of 53.1 ± 13.1 years (range, 26-80 years). Among these patients, 67 were negative and 57 were positive in PD-L1 expression. The detailed characteristics are shown in Table 2. The patients were randomly assigned to the training cohort and the validation cohort using computer-generated randomization.
Table 2. Comparison of conventional MRI features and radiomics score for TET patients of different PD-L1 expression levels.
Significant differences were observed in the prediction of PD-L1 expression in TET based on pericardial invasion sign, ADC value, and world health organization (WHO) pathological classification. The relationship between all these conventional features and PD-L1 expression was presented in Table 2 for both the training and validation cohorts.
Among the 2364 extracted radiomics features, a total of 2086 highly stable features (with inter- and intra-observer ICC values were 0.872 and 0.834, respectively) were selected for further analysis. After conducting pearson correlation analysis, an independent set of 4 features were identified. Subsequently, utilizing LASSO regression on the training cohort (Figure 3), four optimal features were chosen to construct the radiomics signature. The corresponding Rad-score for each patient was then calculated using the following formula:
Figure 3. The least absolute shrinkage and selection operator (LASSO) regression is employed for the selection of radiomics features and construction of a signature. (A) In the LASSO model, the penalization parameter λ selection used 10-fold cross-validation as the minimum criteria. The log (λ) (x-axis) was plotted against the partial likelihood deviance (y-axis). Dotted vertical lines were drawn at the minimum criteria and the 1-SE criteria. λ value of 0.098, with log (λ), −2.317 was chosen (1-SE criteria). (B) LASSO coefficient profiles of the radiomics features. Ten-fold cross-validation in the log (λ) sequence was used to draw the vertical line at the value selected; also indicated are 4 features with nonzero coefficients.
The multivariate logistic regression analysis revealed that the ADC value, pericardial effusion, and radiomics signature emerged as independent predictors of PD-L1 expression in the training cohort (Table 3). The combined model containing three indicators was shown in the nomogram (Figure 4). The discriminating efficiency of combined radiomics nomogram was confirmed in the ROC analysis with an AUC of 0.903 and 0.894 for the training and validation cohort, respectively (Table 4, Figure 5).
Table 3. Results of univariate and multivariate logistic regression for predicting PD-L1 expression in TET.
Figure 4. Nomogram of radiomics-clinical combined model for predicting PD_L1 expression level in TET.
Table 4. The performance of radio_clinical combined model, radiomics model, ADC model and conventional MRI sign for the differentiating PD_L1 expression level in TET.
Figure 5. ROC curve of combined model, radiomics model, ADC and pericardial effusion sign for discriminating different PD_L1 expression (a training cohort, b validation cohort).
The results of the DCAs for conventional MR imaging, ADC model, radiomics signature model, and combined radiomics nomogram in both the combined and validation cohorts can be observed in Figure 6. The DCA curves demonstrate that the combined radiomics nomogram exhibits significant clinical usefulness when considering threshold probabilities exceeding 5%. This suggests that the combined radiomics nomogram serves as a dependable clinical tool for predicting PD-L1 status among TET patients.
Figure 6. Decision curve analysis for conventional MRI, apparent diffusion coefficient, radiomics signature model, and combined radiomics nomogram for the combined training and validation cohort.
Regarding immune checkpoints in TETs, considerable attention has been directed towards PD-1 and its ligand 1, eliciting escalating interest (2, 19). Previous studies have demonstrated a significant correlation between high PD-L1 IHC expression and enhanced response to pembrolizumab in patients with TET (20). A non-invasive, real-time monitoring approach for PD-L1 expression of TET capable of capturing spatio-temporal heterogeneity is imperative. In our study, the radiomics signature model based on multi-sequence MRI exhibited promising predictive performance in determining PD-L1 expression in TET. Furthermore, by integrating additional factors such as peri-cardial invasion sign, ADC value, and radiomics signature into a comprehensive nomogram, we observed further enhancement in the efficacy of the combined model. Consequently, we propose that utilizing a combined nomogram with radiomics could serve as an effective approach for predicting PD-L1 expression in TET.
In recent years, relevant studies on radiomics for immune therapy of tumor have been increasingly reported (7, 21, 22). Several studies have utilized radiomics methods to investigate the potential of predicting immune status in thoracic tumors (21, 23). Tian et al. (21) employed a CT based radiomics signature and predicted high PD-L1 expression of non-small cell lung cancer and to deduce clinical outcomes in response to immunotherapy. Zheng et al. (7) developed and validated a radiomic model based on contrast-enhanced computed tomography to predict the expression of PD-L1 in head and neck squamous cell carcinoma. Additionally, previous studies have demonstrated the robust performance of MRI-based radiomics biomarkers in predicting PD-L1 expression levels across various tumor type including hepatocellular carcinoma, cholangiocarcinoma and breast cancer (15, 23, 24). Specifically, our study was the first to employ a multi-sequence MRI radiomics integrated model for predicting the PD-L1 level in TETs. The observed variations in cut-off values could potentially be attributed to the diverse spectrum of cancer types and different imaging methods. In our study, we employed a CPS threshold of ≥ 1 as the cut-off value for determining PD-L1 positive expression in patients with TETs, aligning with treatment strategies established by previous studies (2, 25).
Radiomics has emerged as a burgeoning field that transforms medical images into an extensive array of high-dimensional imaging descriptors for oncological tissue (26). In our study, the radiological signature was constructed using a feature set consisting of four radiomics features, resulting in an AUC of 0.903 and 0.894 in the training and validation cohorts, respectively. Our model selected three distinct wavelet features and one traditional feature extracted from T1WI images and ADC maps. The wavelet features were extracted from the decomposed images, which undergo decomposition through the application of a wavelet transform filter. GLSZM (zone entropy and gray-level nonuniformity normalized) mainly describe patterns or the spatial distribution of voxel intensities. The first-order statistics features provide information related to the gray-level distribution of the image (7, 27). These features can comprehensively capture the essence of the original image and effectively extract intratumor heterogeneity information (28). Zhou et al. (18) have reported a radiomics signature based on MRI imaging incorporating 7 wavelet features in intrahepatic cholangiocarcinoma stratification, suggesting that wavelet transformation serves as a multiscale approach enabling comprehensive investigation of tumor biological characteristics and spatial heterogeneity.
According to the literature (29), a substantial proportion of tumors derived from patients with thymoma and thymic carcinoma exhibit expression of both PD-L1 and PD-1. Additionally, the PD-L1 positive group exhibited a higher proportion of high-grade TET patients compared to the negative group in our observation. In the previous study, Padda et al. (30) evaluated PD-L1 expression and also observed a positive correlation between higher PD-L1 expression levels and advanced WHO histologic grades (B2, B3, C), as well as an association with worse overall survival. Katsuya et al. (31) reported a significantly higher expression of PD-L1 in thymic carcinoma compared to thymoma. Finally, we developed a radiomics nomogram by integrating the radiomics signature, ADC value, and peri-cardial invasion sign, which exhibited improved calibration and discriminating efficiency in both training and validation cohorts. Several studies have demonstrated a positive correlation between higher PD-L1 expression and increased tumor aggressiveness in TET (25–32). Furthermore, a meta-analysis revealed significantly elevated levels of PD-L1 positivity in thymoma type B2/B3 or thymic carcinoma compared to thymoma types A/AB/B1 (5). This may elucidate the decreased ADC value and presence of pericardial invasion sign observed in conventional MRI of the PD-L1 positive group in TET.
Our study had some limitations and prospects. Firstly, our radiomics analysis was conducted on a single machine at a single institution. To provide high-level evidence for clinical practice, external validation in a larger sample from multiple institutions is necessary. Secondly, the cohort size, particularly the limited number of PD-L1 positive cases, was relatively small in this study. Thirdly, we only included a broad group of TET patients; however, future studies focusing on specific pathological subgroups of TET may yield more significant findings. Fourthly, this study focuses on the validation of PD-L1 prediction methodology. It mainly explores the feasibility of using MRI to predict PD-L1 expression and provides a non-invasive and rapid PD-L1 status evaluation tool for clinical practice to aid decision-making in precision diagnosis and treatment. In the subsequent prospective studies, we will include patients with high homogeneity and perform prognostic correlation analysis on the model.
In our study, we have developed a radiomics signature based on multi-parametric MRI as a non-invasive and reliable method for predicting the expression level of PD-L1 in TET patients. Additionally, we have established a nomogram model that integrates the radiomics signature with clinical data, ADC values, and conventional MRI factors to enable personalized evaluation of PD-L1 expression levels in TET patients.
The original contributions presented in the study are included in the article/Supplementary Material. Further inquiries can be directed to the corresponding author.
The ethical approval for this retrospective study was obtained from the Ethics Committee of our institute, and the requirement for written informed consent was waived.
JS: Data curation, Investigation, Methodology, Software, Writing – original draft. LZ: Data curation, Project administration, Writing – original draft. SL: Methodology, Writing – original draft. XM: Resources, Software, Writing – original draft. TY: Investigation, Methodology, Resources, Writing – review & editing. WZ: Conceptualization, Methodology, Project administration, Visualization, Writing – original draft. YY: Funding acquisition, Project administration, Resources, Writing – review & editing. JH: Funding acquisition, Methodology, Resources, Writing – review & editing. WG: Conceptualization, Data curation, Formal Analysis, Funding acquisition, Resources, Supervision, Writing – review & editing.
The author(s) declare that financial support was received for the research and/or publication of this article. National Natural Science Foundation of China (82473414,82141121), Beijing Xisike Clinical Oncology Research Foundation (Y-MSDPU2021-0223, Y-QL2019-0364), Bethune Young and middle aged Scientific Research training project (BQE-TY-SSPC (2)-N-01), The Friendship Hospital of Ili Kazakh Autonomous Prefecture,Ili & Jiangsu Joint Institute of Health (yl2023zd02),Scientific research project of Ili Prefecture Clinical Research Institute (yl2020ms11),Jiangsu Province’s Key provincial Talents Program (ZDRCA2016028), The Open Project of Jiangsu Biobank of Clinical Resources (SBK202004003), The Specialized Diseases Clinical Research Fund of Jiangsu Province Hospital (DL202412),The Joint Open Research Fund of Nanjing University of Posts and Telecommunications & Jiangsu Province Hospital. The Medical Engineering Translational Fund of Jiangsu Province Hospital (RG202401).
The authors declare that the research was conducted in the absence of any commercial or financial relationships that could be construed as a potential conflict of interest.
The author(s) declare that no Generative AI was used in the creation of this manuscript.
All claims expressed in this article are solely those of the authors and do not necessarily represent those of their affiliated organizations, or those of the publisher, the editors and the reviewers. Any product that may be evaluated in this article, or claim that may be made by its manufacturer, is not guaranteed or endorsed by the publisher.
The Supplementary Material for this article can be found online at: https://www.frontiersin.org/articles/10.3389/fimmu.2025.1555530/full#supplementary-material
1. Marx A, et al. The 2021 WHO classification of tumors of the thymus and mediastinum: what is new in thymic epithelial, germ cell, and mesenchymal tumors? J Thorac Oncol. (2022) 17:200–13.
2. Ao YQ, Gao J, Wang S, Jiang JH, Deng J, Wang HK, et al. Immunotherapy of thymic epithelial tumors: molecular understandings and clinical perspectives. Mol Cancer. (2023) 22:70. doi: 10.1186/s12943-023-01772-4
3. Weissferdt A, Fujimoto J, Kalhor N, Rodriguez J, Bassett R, Wistuba II, et al. Expression of PD-1 and PD-L1 in thymic epithelial neoplasms. Mod Pathol. (2017) 30:826–33. doi: 10.1038/modpathol.2017.6
4. Arbour KC, Naidoo J, Steele KE, Ni A, Moreira AL, Rekhtman N, et al. Expression of PD-L1 and other immunotherapeutic targets in thymic epithelial tumors. PloS One. (2017) 12:e182665. doi: 10.1371/journal.pone.0182665
5. Chen HF, Wu LX, Li XF, Zhu YC, Pan WW, Wang WX, et al. PD-L1 expression level in different thymoma stages and thymic carcinoma: a meta-analysis. Tumori. (2020) 106:306–11. doi: 10.1177/0300891620915788
6. Berardi R, Goteri G, Brunelli A, Pagliaretta S, Paolucci V, Caramanti M, et al. Prognostic relevance of programmed cell death protein 1/programmed death-ligand 1 pathway in thymic Malignancies with combined immunohistochemical and biomolecular approach. Expert Opin Ther Targets. (2020) 24:937–43. doi: 10.1080/14728222.2020.1790529
7. Zheng YM, Yuan MG, Zhou RQ, Hou F, Zhan JF, Liu ND, et al. A computed tomography-based radiomics signature for predicting expression of programmed death ligand 1 in head and neck squamous cell carcinoma. Eur Radiol. (2022) 32:5362–70. doi: 10.1007/s00330-022-08651-4
8. Yip SS, Aerts HJ. Applications and limitations of radiomics. Phys Med Biol. (2016) 61:R150–66. doi: 10.1088/0031-9155/61/13/R150
9. Liu Z, Wang S, Dong D, Wei J, Fang C, Zhou X, et al. The applications of radiomics in precision diagnosis and treatment of oncology: opportunities and challenges. Theranostics. (2019) 9:1303–22. doi: 10.7150/thno.30309
10. Xiao G, Hu YC, Ren JL, Qin P, Han JC, Qu XY, et al. MR imaging of thymomas: a combined radiomics nomogram to predict histologic subtypes. Eur Radiol. (2021) 31:447–57. doi: 10.1007/s00330-020-07074-3
11. Mayoral M, Pagano AM, Araujo-Filho J, Zheng J, Perez-Johnston R, Tan KS, et al. Conventional and radiomic features to predict pathology in the preoperative assessment of anterior mediastinal masses. Lung Cancer. (2023) 178:206–12. doi: 10.1016/j.lungcan.2023.02.014
12. Dong W, Xiong S, Lei P, Wang X, Liu H, Liu Y, et al. Application of a combined radiomics nomogram based on CE-CT in the preoperative prediction of thymomas risk categorization. Front Oncol. (2022) 12:944005. doi: 10.3389/fonc.2022.944005
13. Yoon J, Suh YJ, Han K, Cho H, Lee HJ, Hur J, et al. Utility of CT radiomics for prediction of PD-L1 expression in advanced lung adenocarcinomas. Thorac Cancer. (2020) 11:993–1004. doi: 10.1111/1759-7714.13352
14. Wen Q, Yang Z, Zhu J, Qiu Q, Dai H, Feng A, et al. Pretreatment CT-based radiomics signature as a potential imaging biomarker for predicting the expression of PD-L1 and CD8+TILs in ESCC. Onco Targets Ther. (2020) 13:12003–13. doi: 10.2147/OTT.S261068
15. Zhang J, Wu Z, Zhang X, Liu S, Zhao J, Yuan F, et al. Machine learning: an approach to preoperatively predict PD-1/PD-L1 expression and outcome in intrahepatic cholangiocarcinoma using MRI biomarkers. Esmo Open. (2020) 5:e910. doi: 10.1136/esmoopen-2020-000910
16. Teixido C, Vilarino N, Reyes R, Reguart N. PD-L1 expression testing in non-small cell lung cancer. Ther Adv Med Oncol. (2018) 10:433588763. doi: 10.1177/1758835918763493
17. Landis JR, Koch GG. The measurement of observer agreement for categorical data. Biometrics. (1977) 33:159–74.
18. Zhou Y, Zhou G, Zhang J, Xu C, Wang X, Xu P. Radiomics signature on dynamic contrast-enhanced MR images: a potential imaging biomarker for prediction of microvascular invasion in mass-forming intrahepatic cholangiocarcinoma. Eur Radiol. (2021) 31:6846–55. doi: 10.1007/s00330-021-07793-1
19. Tateo V, Manuzzi L, De Giglio A, Parisi C, Lamberti G, Campana D, et al. Immunobiology of thymic epithelial tumors: implications for immunotherapy with immune checkpoint inhibitors. Int J Mol Sci. (2020) 21. doi: 10.3390/ijms21239056
20. Cho J, Kim HS, Ku BM, Choi YL, Cristescu R, Han J, et al. Pembrolizumab for patients with refractory or relapsed thymic epithelial tumor: an open-label phase II trial. J Clin Oncol. (2019) 37:2162–70. doi: 10.1200/JCO.2017.77.3184
21. Tian P, He B, Mu W, Liu K, Liu L, Zeng H, et al. Assessing PD-L1 expression in non-small cell lung cancer and predicting responses to immune checkpoint inhibitors using deep learning on computed tomography images. Theranostics. (2021) 11:2098–107. doi: 10.7150/thno.48027
22. Hectors SJ, Lewis S, Besa C, King MJ, Said D, Putra J, et al. MRI radiomics features predict immuno-oncological characteristics of hepatocellular carcinoma. Eur Radiol. (2020) 30:3759–69. doi: 10.1007/s00330-020-06675-2
23. Yin X, Liao H, Yun H, Lin N, Li S, Xiang Y, et al. Artificial intelligence-based prediction of clinical outcome in immunotherapy and targeted therapy of lung cancer. Semin Cancer Biol. (2022) 86:146–59. doi: 10.1016/j.semcancer.2022.08.002
24. Lo GR, Wen H, Reiner JS, Hoda R, Sevilimedu V, Martinez DF, et al. Assessing PD-L1 expression status using radiomic features from contrast-enhanced breast MRI in breast cancer patients: initial results. Cancers (Basel). (2021) 13. doi: 10.3390/cancers13246273
25. Katsuya Y, Horinouchi H, Asao T, Kitahara S, Goto Y, Kanda S, et al. Expression of programmed death 1 (PD-1) and its ligand (PD-L1) in thymic epithelial tumors: Impact on treatment efficacy and alteration in expression after chemotherapy. Lung Cancer. (2016) 99:4–10. doi: 10.1016/j.lungcan.2016.05.007
26. Lambin P, Rios-Velazquez E, Leijenaar R, Carvalho S, van Stiphout RG, Granton P, et al. Radiomics: extracting more information from medical images using advanced feature analysis. Eur J Cancer. (2012) 48:441–46. doi: 10.1016/j.ejca.2011.11.036
27. Xie W, Jiang Z, Zhou X, Zhang X, Zhang M, Liu R, et al. Quantitative radiological features and deep learning for the non-invasive evaluation of programmed death ligand 1 expression levels in gastric cancer patients: A digital biopsy study. Acad Radiol. (2023) 30:1317–28. doi: 10.1016/j.acra.2022.10.012
28. Chen J, Lu S, Mao Y, Tan L, Li G, Gao Y, et al. An MRI-based radiomics-clinical nomogram for the overall survival prediction in patients with hypopharyngeal squamous cell carcinoma: a multi-cohort study. Eur Radiol. (2022) 32:1548–57. doi: 10.1007/s00330-021-08292-z
29. Owen D, Chu B, Lehman AM, Annamalai L, Yearley JH, Shilo K, et al. Expression patterns, prognostic value, and intratumoral heterogeneity of PD-L1 and PD-1 in thymoma and thymic carcinoma. J Thorac Oncol. (2018) 13:1204–12. doi: 10.1016/j.jtho.2018.04.013
30. Padda SK, Riess JW, Schwartz EJ, Tian L, Kohrt HE, Neal JW, et al. Diffuse high intensity PD-L1 staining in thymic epithelial tumors. J Thorac Oncol. (2015) 10:500–08. doi: 10.1097/JTO.0000000000000429
31. Katsuya Y, Fujita Y, Horinouchi H, Ohe Y, Watanabe S, Tsuta K. Immunohistochemical status of PD-L1 in thymoma and thymic carcinoma. Lung Cancer. (2015) 88:154–59. doi: 10.1016/j.lungcan.2015.03.003
Keywords: MRI, thymic epitelial tumors, radiomics, PD-L1, immunotharapy
Citation: Shen J, Zhang L, Li S, Mu X, Yu T, Zhang W, Yu Y, He J and Gao W (2025) Multi-sequence MRI based radiomics nomogram for prediction expression of programmed death ligand 1 in thymic epithelial tumor. Front. Immunol. 16:1555530. doi: 10.3389/fimmu.2025.1555530
Received: 04 January 2025; Accepted: 20 March 2025;
Published: 11 April 2025.
Edited by:
Yong Fan, Institute of Cellular Therapeutics, United StatesReviewed by:
Tong Zhang, Sichuan University, ChinaCopyright © 2025 Shen, Zhang, Li, Mu, Yu, Zhang, Yu, He and Gao. This is an open-access article distributed under the terms of the Creative Commons Attribution License (CC BY). The use, distribution or reproduction in other forums is permitted, provided the original author(s) and the copyright owner(s) are credited and that the original publication in this journal is cited, in accordance with accepted academic practice. No use, distribution or reproduction is permitted which does not comply with these terms.
*Correspondence: Wen Gao, Z2Fvd2VuQGpzcGgub3JnLmNu; Jing He, aGVqaW5nZ3lAMTYzLmNvbQ==; Yue Yu, eXV5dWUyMDE0QG5qbXUuZWR1LmNu; Wei Zhang, ZnNremhhbmd3ZWlAMTI2LmNvbQ==
†These authors have contributed equally to this work
Disclaimer: All claims expressed in this article are solely those of the authors and do not necessarily represent those of their affiliated organizations, or those of the publisher, the editors and the reviewers. Any product that may be evaluated in this article or claim that may be made by its manufacturer is not guaranteed or endorsed by the publisher.
Research integrity at Frontiers
Learn more about the work of our research integrity team to safeguard the quality of each article we publish.