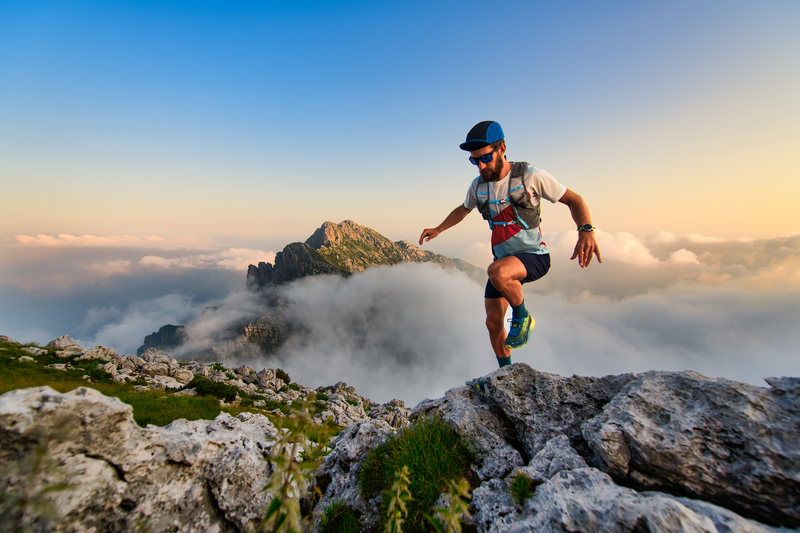
94% of researchers rate our articles as excellent or good
Learn more about the work of our research integrity team to safeguard the quality of each article we publish.
Find out more
ORIGINAL RESEARCH article
Front. Immunol.
Sec. Cytokines and Soluble Mediators in Immunity
Volume 16 - 2025 | doi: 10.3389/fimmu.2025.1554341
This article is part of the Research Topic Cytokine Dynamics in Livestock: From Health to Pathology View all articles
The final, formatted version of the article will be published soon.
You have multiple emails registered with Frontiers:
Please enter your email address:
If you already have an account, please login
You don't have a Frontiers account ? You can register here
Bovine mastitis causes a significant loss to the dairy industry by affecting the quantity and quality of milk. Addressing this challenge, the present study will leverage advanced omics techniques for early mastitis detection in early lactating Sahiwal cows (Bos indicus). This was the first differential transcriptomic study investigating the alterations in gene expression in milk somatic cells during the progression of naturally occurring mastitis in indigenous Sahiwal cows. Cows were grouped into healthy (H), subclinical mastitis (SCM) and clinical mastitis (CM) groups by thoroughly screening them using the California Mastitis Test (CMT) and milk somatic cell counts (SCC). This was followed by detailed milk composition analysis, differential leukocyte counts (DLC), and microbiological culture. The differential gene expression of milk SCs through transcriptome profiling identified 83 and 76, up-regulated and 157 and 192 down-regulated genes in CM vs H and SCM vs H groups (log2 fold change ≥1 and ≤-1, p < 0.05) respectively. Pathway analysis revealed that upregulated genes were enriched in pathways such as phagosome activity, IL-17 signalling, Th1 and Th2 cell differentiation, while downregulated genes were linked to RIG-I-like receptor signalling, NK cell cytotoxicity, and Toll-like receptor signalling and Cytokine-cytokine receptor interactions. Notably, the study underscores the roles of chemokines CCL8, CCL2, and CXCL10 in immune cell recruitment during mastitis, where their downregulation suggests impaired mammary immune defense that governs Chemokine signalling pathways. Further, the comparative analysis with the previously available milk SCs proteome data identified the downregulation of chemokines signalling pathways during mastitis. Overall, this research enhances our understanding of mastitis pathogenesis and emphasizes that these targeted chemokines may boost mammary resilience through immunomodulation, genetic selection and genome editing or by utilising adjuvants in vaccine development that restore chemokine signalling offers a potential strategy to improve mastitis resistance in dairy cattle.
Keywords: Bovine Mastitis, Transcriptomics, differential expressed genes, Cytokine interactions, Chemokine markers, mRNA sequencing
Received: 01 Jan 2025; Accepted: 04 Mar 2025.
Copyright: © 2025 Satheesan, Dang and Alex. This is an open-access article distributed under the terms of the Creative Commons Attribution License (CC BY). The use, distribution or reproduction in other forums is permitted, provided the original author(s) or licensor are credited and that the original publication in this journal is cited, in accordance with accepted academic practice. No use, distribution or reproduction is permitted which does not comply with these terms.
* Correspondence:
Ajay Kumar Dang, National Dairy Research Institute (ICAR), Karnal, India
Disclaimer: All claims expressed in this article are solely those of the authors and do not necessarily represent those of their affiliated organizations, or those of the publisher, the editors and the reviewers. Any product that may be evaluated in this article or claim that may be made by its manufacturer is not guaranteed or endorsed by the publisher.
Research integrity at Frontiers
Learn more about the work of our research integrity team to safeguard the quality of each article we publish.