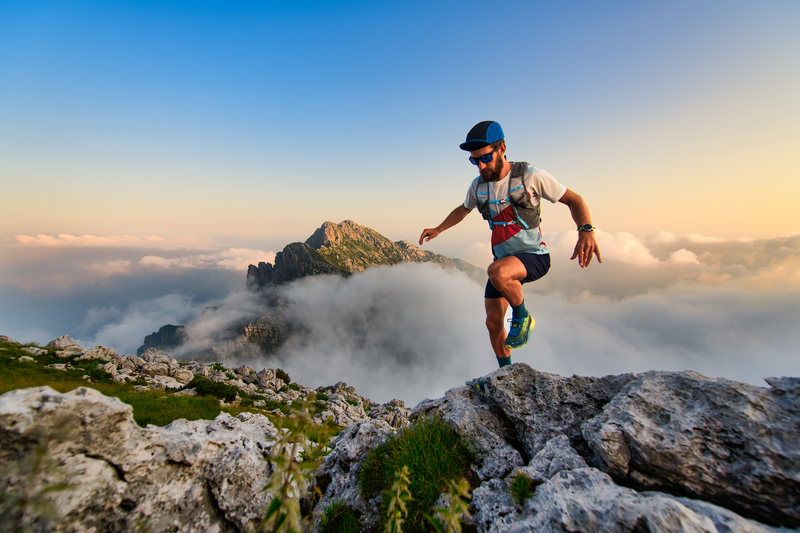
94% of researchers rate our articles as excellent or good
Learn more about the work of our research integrity team to safeguard the quality of each article we publish.
Find out more
BRIEF RESEARCH REPORT article
Front. Immunol.
Sec. T Cell Biology
Volume 16 - 2025 | doi: 10.3389/fimmu.2025.1553554
This article is part of the Research Topic Thymus Research and Development: A New Look to the Past, Current Knowledge, and Future Perspectives View all 8 articles
The final, formatted version of the article will be published soon.
You have multiple emails registered with Frontiers:
Please enter your email address:
If you already have an account, please login
You don't have a Frontiers account ? You can register here
Thymically committed regulatory CD4 T cells (tTregs) are essential for immune homeostasis and selftolerance. We established the human tTreg Expression Signature by comparing genome-wide transcriptomic profiles between tTregs and their conventional counterparts (tTconvs). We further exploited the high sequencing depth of our bulk RNA-seq data to identify a subset of 250 genes significantly expressed in human tTregs and with neglectable expression in tTconvs, defined as below the levels of expression of IL2RA, that we named thymic Treg "private" genes. Notably, pathways related to cell motility, inflammation and T-cell effector specification were overrepresented within the tTreg private genes. We found that 163 of these genes were significantly less expressed in circulating naïve and memory Treg when compared to peripheral data generated in parallel. This result suggested a higher activity for most of the "private" genes in the thymus when compared to the peripheral compartments. Altogether, we provide a unique resource to inform future studies, such as improving annotation in single-cell and spatial transcriptional data, or help designing human studies to validate putative biomarkers for thymically committed Tregs, a priority in the field.
Keywords: Human thymic CD4 Treg "private" genes Human Thymus, Human T-cell development, CD4 T cells, regulatory T cells, Foxp3, RNA-Seq
Received: 30 Dec 2024; Accepted: 03 Mar 2025.
Copyright: © 2025 Raposo, Paço, Ângelo-Dias, Rosmaninho, Almeida and Sousa. This is an open-access article distributed under the terms of the Creative Commons Attribution License (CC BY). The use, distribution or reproduction in other forums is permitted, provided the original author(s) or licensor are credited and that the original publication in this journal is cited, in accordance with accepted academic practice. No use, distribution or reproduction is permitted which does not comply with these terms.
* Correspondence:
Alexandre ASF Raposo, GIMM - Gulbenkian Institute for Molecular Medicine, Lisbon, Portugal
Disclaimer: All claims expressed in this article are solely those of the authors and do not necessarily represent those of their affiliated organizations, or those of the publisher, the editors and the reviewers. Any product that may be evaluated in this article or claim that may be made by its manufacturer is not guaranteed or endorsed by the publisher.
Research integrity at Frontiers
Learn more about the work of our research integrity team to safeguard the quality of each article we publish.