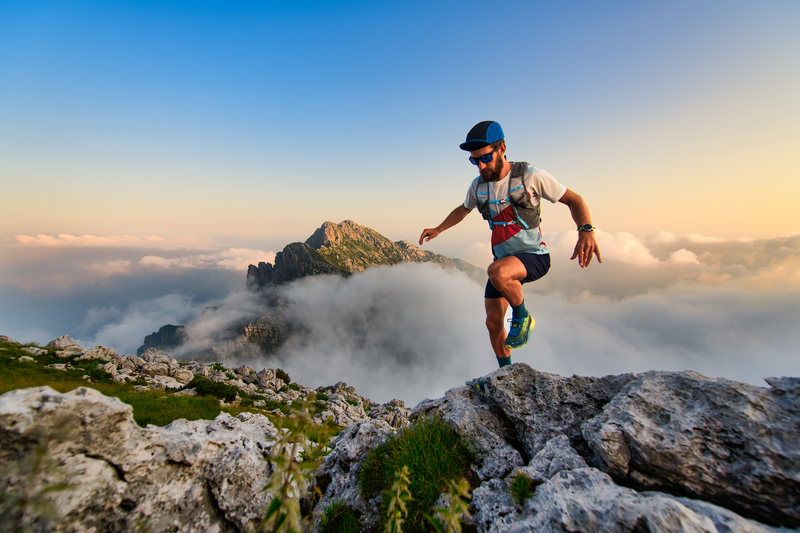
94% of researchers rate our articles as excellent or good
Learn more about the work of our research integrity team to safeguard the quality of each article we publish.
Find out more
ORIGINAL RESEARCH article
Front. Immunol.
Sec. Cancer Immunity and Immunotherapy
Volume 16 - 2025 | doi: 10.3389/fimmu.2025.1552235
This article is part of the Research Topic Community Series in The Immunosuppressive Tumor Microenvironment and Strategies to Revert its Immune Regulatory Milieu for Cancer Immunotherapy: Volume II View all 4 articles
The final, formatted version of the article will be published soon.
You have multiple emails registered with Frontiers:
Please enter your email address:
If you already have an account, please login
You don't have a Frontiers account ? You can register here
Background: γδ T cells are integral elements of the immune system and have shown therapeutic potential in the treatment of AML. Nevertheless, the influence of distinct functional subsets, including the activating marker NKG2D, the immune exhaustion marker TIGIT and the regulatory marker Foxp3, on therapeutic outcomes in AML patients remains unknown.Methods: First, we analyzed RNA-seq data from 167 patients in the TCGA database, concentrating on the correlations between NKG2D, TIGIT, and Foxp3 gene expressions and their association with prognosis in AML. Flow cytometry was employed to assess the expression of these molecular markers on γδ T cells and Vδ1/Vδ2 subsets in the peripheral blood of 25 AML-DN patients, 15 patients in CR, and 27 HCs. We also analyzed the relationship between the expression frequency of NKG2D, TIGIT, and Foxp3 on γδ T cells and their subsets, and their clinical outcomes.Results: Based on data from the TCGA database, we found that a high expression level of NKG2D in combination with a low expression level of TIGIT was significantly associated with longer OS in AML patients. Clinical data revealed that γδ T cells from AML-DN patients exhibited higher expression levels of TIGIT and Foxp3, whereas NKG2D expression was lower compared to HCs. Notably, the expression of the NKG2D + TIGIT -Vδ1 subset was significantly reduced in AML-DN patients compared to CR patients. Univariate logistic regression and Cox regression analyses further indicated that a high expression of the NKG2D + TIGIT -Vδ1 subset was associated with better a clinical prognosis.This study indicates that NKG2D + TIGIT -Vδ1 T cells are strongly correlated with improved prognosis in AML and future research should investigate their potential in adoptive immunotherapy to advance more personalized and precise treatment strategies.
Keywords: Acute Myeloid Leukemia, NKG2D, TIGIT, Foxp3, γδ T cells, prognosis, immune subsets
Received: 27 Dec 2024; Accepted: 10 Mar 2025.
Copyright: © 2025 Jiang, Zheng, Yao, Ning, Zou, Zhan, Lan, Yi, Jin and Wu. This is an open-access article distributed under the terms of the Creative Commons Attribution License (CC BY). The use, distribution or reproduction in other forums is permitted, provided the original author(s) or licensor are credited and that the original publication in this journal is cited, in accordance with accepted academic practice. No use, distribution or reproduction is permitted which does not comply with these terms.
* Correspondence:
Zhenyi Jin, Jinan University, Guangzhou, 510632, Guangdong Province, China
Disclaimer: All claims expressed in this article are solely those of the authors and do not necessarily represent those of their affiliated organizations, or those of the publisher, the editors and the reviewers. Any product that may be evaluated in this article or claim that may be made by its manufacturer is not guaranteed or endorsed by the publisher.
Research integrity at Frontiers
Learn more about the work of our research integrity team to safeguard the quality of each article we publish.