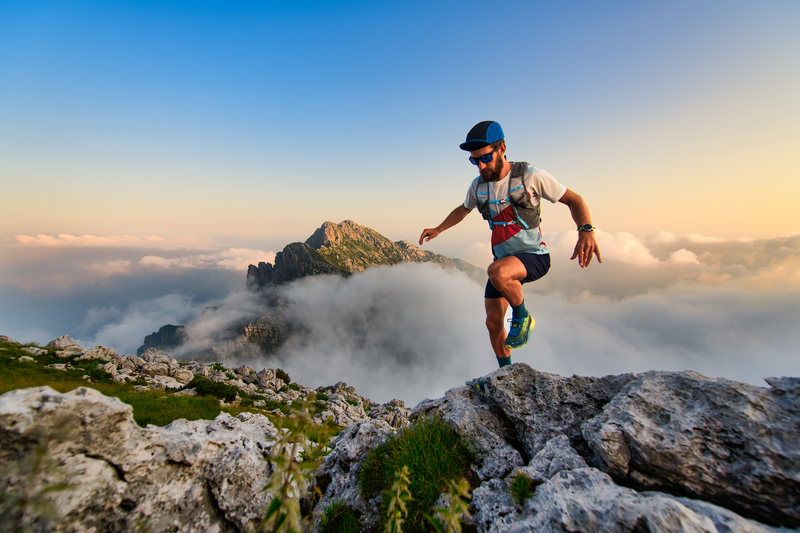
94% of researchers rate our articles as excellent or good
Learn more about the work of our research integrity team to safeguard the quality of each article we publish.
Find out more
ORIGINAL RESEARCH article
Front. Immunol.
Sec. Viral Immunology
Volume 16 - 2025 | doi: 10.3389/fimmu.2025.1549570
This article is part of the Research Topic The Influence of SARS-CoV-2 Infection and Long-COVID on The Incidence of Viral Coinfection. View all articles
The final, formatted version of the article will be published soon.
You have multiple emails registered with Frontiers:
Please enter your email address:
If you already have an account, please login
You don't have a Frontiers account ? You can register here
SARS-CoV-2 has claimed more than 7 million lives worldwide and has been associated with prolonged inflammation, immune dysregulation and persistence of symptoms following severe infection. Understanding the T cell mediated immune response and factors impacting development and continuity of SARS-CoV-2 specific memory T cells is pivotal for developing better therapeutic and monitoring strategies for those most at risk from COVID-19. Here we present a comprehensive analysis of memory T cells in a convalescent cohort (n=20), three months post Omicron infection. Utilising flow cytometry to investigate CD4+CD45RO+ and CD8+CD45RO+ memory T cell IL-2 expression following Omicron (B.1.1.529/BA.1) peptide pool stimulation, alongside T cell receptor repertoire profiling and RNA-Seq analysis, we have identified several immunological features associated with hospitalised status. We observed that while there was no significant difference in median CD4+CD45RO+ IL-2+ and CD8+ CD45RO+ IL-2+ memory T cell count between subgroups, the hospitalised subgroup expressed significantly more IL-2 per cell following Omicron peptide pool exposure in the CD8+CD45RO+ population (p <0.03) and trended toward significance in CD4+CD45RO+ cells (p <0.06). T cell receptor repertoire analysis found that the non-hospitalised subgroup had a much higher number of circulating clonotypes, targeting a wider range of predominantly MHC-I epitopes across the SARS-CoV-2 genome. Several immunodominant epitopes, conserved between both subgroups, were observed, however hospitalised individuals were less likely to express putative HLA alleles responsible for pMHC presentation which may impact TCR affinity. We observed a bias towards shorter CDR3 segments in TCRβ repertoire analysis within the hospitalised subgroup, alongside lower rates of repertoire overlap in CDR3 sequences compared to the non-hospitalised subgroup. We found a significant proportion of TCRs targeted epitopes along the SARS-CoV-2 genome including non-structural proteins, responsible for viral replication and immune evasion. These findings highlight how the continuity of T cell based protective immunity is impacted by both the viral replication cycle of SARS-CoV-2 upon intracellular and innate immune responses, and HLA-type upon TCR affinity and clonotype formation. Our novel Epitope Target Analysis Pipeline (Epi-TAP) could prove beneficial in development of new therapeutic strategies through rapid identification of shared immunodominant epitopes across non-hospitalised and hospitalised subgroups.
Keywords: SARS-CoV-2, COVID-19, T Cell Immunity, T cell receptor (TCR) recognition, Antigen Presentation, Adaptive Immunity, immuno-informatics
Received: 21 Dec 2024; Accepted: 20 Feb 2025.
Copyright: © 2025 Markey, Mclaughlin, Mcdaid, Lynch, English, Alexander, Kelly, Bhavsar, McGilligan, Zhang, Murray, Singh, Walsh, Bjourson, Shukla and Gibson. This is an open-access article distributed under the terms of the Creative Commons Attribution License (CC BY). The use, distribution or reproduction in other forums is permitted, provided the original author(s) or licensor are credited and that the original publication in this journal is cited, in accordance with accepted academic practice. No use, distribution or reproduction is permitted which does not comply with these terms.
* Correspondence:
Priyank Shukla, Personalised Medicine Centre, School of Medicine, Ulster University, Derry/Londonderry, United Kingdom
David Gibson, Personalised Medicine Centre, School of Medicine, Ulster University, Derry/Londonderry, United Kingdom
Disclaimer: All claims expressed in this article are solely those of the authors and do not necessarily represent those of their affiliated organizations, or those of the publisher, the editors and the reviewers. Any product that may be evaluated in this article or claim that may be made by its manufacturer is not guaranteed or endorsed by the publisher.
Research integrity at Frontiers
Learn more about the work of our research integrity team to safeguard the quality of each article we publish.