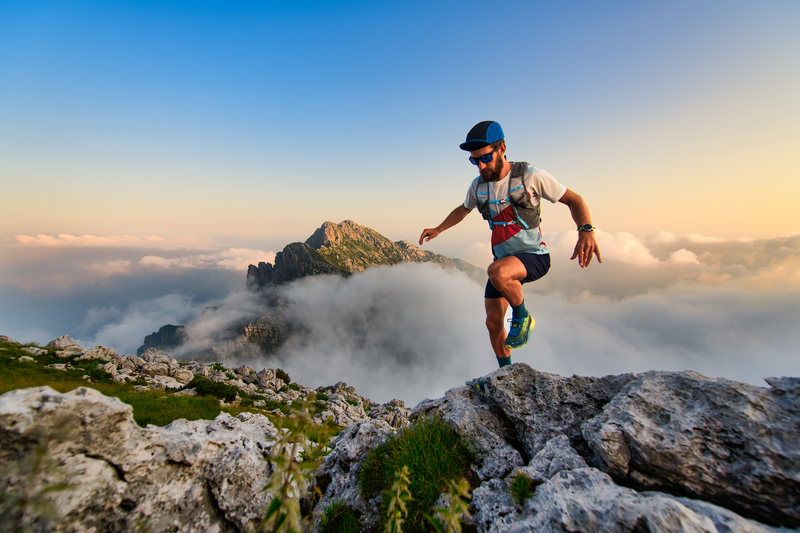
94% of researchers rate our articles as excellent or good
Learn more about the work of our research integrity team to safeguard the quality of each article we publish.
Find out more
ORIGINAL RESEARCH article
Front. Immunol.
Sec. Inflammation
Volume 16 - 2025 | doi: 10.3389/fimmu.2025.1549150
This article is part of the Research Topic Role of bioinformatics and AI in understanding inflammation and immune microenvironment dynamics View all 3 articles
The final, formatted version of the article will be published soon.
You have multiple emails registered with Frontiers:
Please enter your email address:
If you already have an account, please login
You don't have a Frontiers account ? You can register here
Background: Atherosclerosis is a significant contributor to cardiovascular disease, and conventional diagnostic methods frequently fall short in the timely and accurate detection of early-stage atherosclerosis. Abnormal lipid metabolism plays a critical role in the development of atherosclerosis. Consequently, the identification of new diagnostic markers is essential for the precise diagnosis of this condition.The datasets related to atherosclerosis utilized in this research were obtained from the GEO database (GSE2470, GSE24495, GSE100927 and GSE43292). The ssGSEA technique was first utilized to assess lipid metabolism scores in samples affected by atherosclerosis, thereby aiding in the discovery of important regulatory genes linked to lipid metabolism via WGCNA. Following this, differential expression analysis and functional evaluations were carried out, after which various machine learning approaches were employed to determine significant diagnostic genes for atherosclerosis. A diagnostic model was then developed and validated through several machine learning algorithms. Furthermore, molecular docking studies were conducted to analyze the binding affinity of these key markers with therapeutic agents for atherosclerosis. The ssGSEA technique was also used to measure immune cell scores in atherosclerotic samples, aiding the exploration of the connection between key diagnostic markers and immune cells. Finally, the expression variations of the identified pivotal genes were confirmed through experimental validation.Result: WGCNA identified 302 lipid metabolism-related genes in atherosclerotic samples, and functional analysis revealed that these genes are associated with multiple immune pathways. Through further differential analysis and screening using machine learning algorithms, APLNR, PCDH12, PODXL, SLC40A1, TM4SF18, and TNFRSF25 were identified as key diagnostic genes for atherosclerosis. The diagnostic model we constructed was confirmed to predict the occurrence of atherosclerosis with high accuracy, and molecular docking studies indicated that these six key diagnostic genes have potential as drug targets. Additionally, the ssGSEA algorithm further validated the association of these diagnostic genes with various immune cells. Finally, the expression levels of these six genes were experimentally confirmed.Our study introduces novel lipid metabolism-related diagnostic markers for atherosclerosis and emphasizes their potential as immune-related drug targets. This research provides a valuable approach for the predictive diagnosis and targeted therapy of atherosclerosis.
Keywords: Atherosclerosis, Lipid Metabolism, machine learning, Immune infiltration, biomarkers
Received: 20 Dec 2024; Accepted: 05 Feb 2025.
Copyright: © 2025 Chen, Wu, Guan, Chen, Chai, Ying, Zhu, Li and Zhao. This is an open-access article distributed under the terms of the Creative Commons Attribution License (CC BY). The use, distribution or reproduction in other forums is permitted, provided the original author(s) or licensor are credited and that the original publication in this journal is cited, in accordance with accepted academic practice. No use, distribution or reproduction is permitted which does not comply with these terms.
* Correspondence:
Biao Wu, Changhai Hospital, Second Military Medical University, Shanghai, China
Disclaimer: All claims expressed in this article are solely those of the authors and do not necessarily represent those of their affiliated organizations, or those of the publisher, the editors and the reviewers. Any product that may be evaluated in this article or claim that may be made by its manufacturer is not guaranteed or endorsed by the publisher.
Research integrity at Frontiers
Learn more about the work of our research integrity team to safeguard the quality of each article we publish.