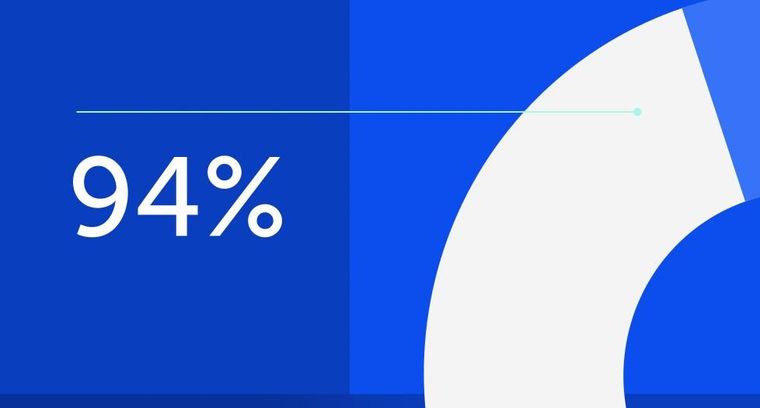
94% of researchers rate our articles as excellent or good
Learn more about the work of our research integrity team to safeguard the quality of each article we publish.
Find out more
ORIGINAL RESEARCH article
Front. Immunol.
Sec. Cancer Immunity and Immunotherapy
Volume 16 - 2025 | doi: 10.3389/fimmu.2025.1544348
This article is part of the Research TopicCrosstalk in Tumor Microenvironments: Shaping Early Drug and Immunotherapy StrategiesView all 8 articles
The final, formatted version of the article will be published soon.
Select one of your emails
You have multiple emails registered with Frontiers:
Notify me on publication
Please enter your email address:
If you already have an account, please login
You don't have a Frontiers account ? You can register here
Background Triple-negative breast cancer (TNBC) is a highly aggressive subtype with limited therapeutic targets and poor immunotherapy outcomes. The tumor microenvironment (TME) plays a key role in cancer progression. Advances in single-cell transcriptomics have highlighted the impact of stromal cells on tumor progression, immune suppression, and immunotherapy. This study aims to identify stromal cell marker genes and develop a prognostic signature for predicting TNBC survival outcomes and immunotherapy response.Methods Single-cell RNA sequencing (scRNA-seq) datasets were retrieved from the Gene Expression Omnibus (GEO) database and annotated using known marker genes. Cell types preferentially distributed in TNBC were identified using odds ratios (OR). Bulk transcriptome data were analyzed using Weighted correlation network analysis (WGCNA) to identify myCAF-, VSMC-, and Pericyterelated genes (MVPRGs). A consensus MVP cell-related signature (MVPRS) was developed using 10 machine learning algorithms and 101 model combinations and validated in training and validation cohorts. Immune infiltration and immunotherapy response were assessed using CIBERSORT, ssGSEA, TIDE, IPS scores, and an independent cohort (GSE91061). FN1, a key gene in the model, was validated through qRT-PCR, immunohistochemistry, RNA interference, CCK-8 assay, apoptosis assay and wound-healing assay. In TNBC, three stromal cell subpopulations-myofibroblastic cancer-associated fibroblasts (myCAF), vascular smooth muscle cells (VSMCs), and pericytes-were enriched, exhibiting high interaction frequencies and strong associations with poor prognosis. A nine-gene prognostic model (MVPRS), developed from 23 prognostically significant genes among the 259 MVPRGs, demonstrated excellent predictive performance and was validated as an independent prognostic factor. A nomogram integrating MVPRS, age, stage, and tumor grade offered clinical utility. High-risk group showed reduced immune infiltration and increased activity in tumor-related pathways like ANGIOGENESIS and HYPOXIA, while low-risk groups responded better to immunotherapy based on TIDE and IPS scores. FN1, identified as a key oncogene, was highly expressed in TNBC tissues and cell lines, promoting proliferation and migration while inhibiting apoptosis.This study reveals TNBC microenvironment heterogeneity and introduces a prognostic signature based on myCAF, VSMC, and Pericyte marker genes. MVPRS effectively predicts TNBC prognosis and immunotherapy response, providing guidance for personalized treatment. FN1 was validated as a key oncogene impacting TNBC progression and malignant phenotype, with potential as a therapeutic target.
Keywords: Triple-negative breast cancer, machine learning, prognosis, Immunotherapy, Tumor Microenvironment
Received: 12 Dec 2024; Accepted: 21 Apr 2025.
Copyright: © 2025 Li, Jin, Pan, Zhang, Liying, Deng, Zhou, Guo and Zhang. This is an open-access article distributed under the terms of the Creative Commons Attribution License (CC BY). The use, distribution or reproduction in other forums is permitted, provided the original author(s) or licensor are credited and that the original publication in this journal is cited, in accordance with accepted academic practice. No use, distribution or reproduction is permitted which does not comply with these terms.
* Correspondence:
Yifeng Zhou, Department of Genetics, School of Basic Medical Sciences, Suzhou Medical College of Soochow University, Suzhou, China
Binbin Guo, Department of Genetics, School of Basic Medical Sciences, Suzhou Medical College of Soochow University, Suzhou, China
Shenghua Zhang, Department of Genetics, School of Basic Medical Sciences, Suzhou Medical College of Soochow University, Suzhou, China
Disclaimer: All claims expressed in this article are solely those of the authors and do not necessarily represent those of their affiliated organizations, or those of the publisher, the editors and the reviewers. Any product that may be evaluated in this article or claim that may be made by its manufacturer is not guaranteed or endorsed by the publisher.
Supplementary Material
Research integrity at Frontiers
Learn more about the work of our research integrity team to safeguard the quality of each article we publish.