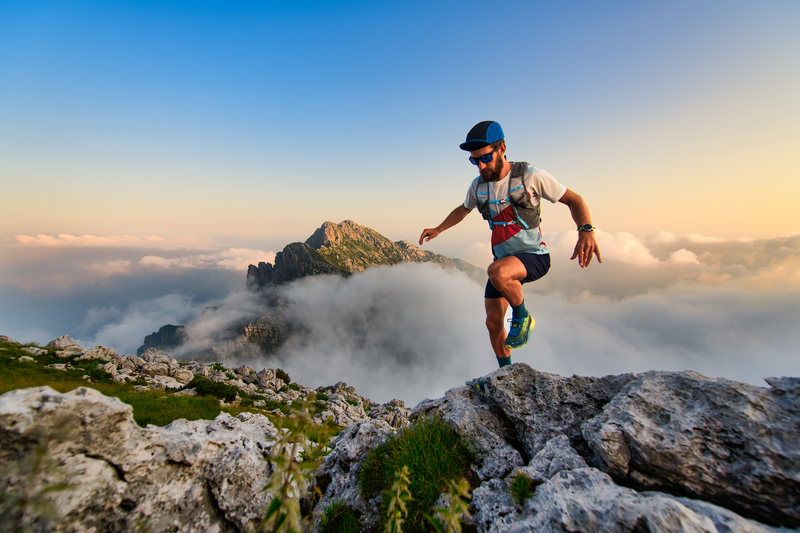
94% of researchers rate our articles as excellent or good
Learn more about the work of our research integrity team to safeguard the quality of each article we publish.
Find out more
ORIGINAL RESEARCH article
Front. Immunol.
Sec. Cancer Immunity and Immunotherapy
Volume 16 - 2025 | doi: 10.3389/fimmu.2025.1544104
This article is part of the Research Topic Community Series in Beyond PD-1: Novel Checkpoint Receptors and Ligands as Targets for Immunotherapy, Volume II View all 3 articles
The final, formatted version of the article will be published soon.
You have multiple emails registered with Frontiers:
Please enter your email address:
If you already have an account, please login
You don't have a Frontiers account ? You can register here
The widespread use of immune checkpoint inhibitors (anti-CTLA4 or PD-1) has opened a new chapter in tumor immunotherapy by providing long-term remission for patients. Unfortunately, however, these agents are not universally available and only a minority of patients respond to them.Therefore, there is an urgent need to develop novel therapeutic strategies targeting other co-inhibitory molecules, such as LAG3, TIM3, TIGIT, NRP1, VISTA. In addition, combination therapies including combined blockade and chemo-immunotherapy are promising topics in this field, such as the recent FDA approval of combination therapy targeting both LAG3 and PD-1. However, comprehensive information on the expression and prognostic value of co-inhibitory molecules, including co-inhibitory receptors and their ligands, in different cancers is not yet available. For this reason, we performed the present analysis to assess the expression patterns and correlations of co-inhibitory molecules, and further evaluated their relationships with patient prognosis, tumor microenvironment and drug sensitivity. These co-inhibitory molecules have the potential to be prognostic biomarkers and drivers of individualized therapy for a wide range of cancers, as they are closely associated with these metrics.
Keywords: Immunotherapy, immune checkpoint inhibitors, Co-inhibitory molecules, Pan-cancer, biomarkers
Received: 12 Dec 2024; Accepted: 03 Mar 2025.
Copyright: © 2025 Ren, Guo, Geng, Chen, Wang, SHI and Zhou. This is an open-access article distributed under the terms of the Creative Commons Attribution License (CC BY). The use, distribution or reproduction in other forums is permitted, provided the original author(s) or licensor are credited and that the original publication in this journal is cited, in accordance with accepted academic practice. No use, distribution or reproduction is permitted which does not comply with these terms.
* Correspondence:
LEI SHI, Chongqing University, Chongqing, China
Lian Zhou, Cancer Hospital, Chongqing University, Chongqing, 400030, Anhui, China
Disclaimer: All claims expressed in this article are solely those of the authors and do not necessarily represent those of their affiliated organizations, or those of the publisher, the editors and the reviewers. Any product that may be evaluated in this article or claim that may be made by its manufacturer is not guaranteed or endorsed by the publisher.
Research integrity at Frontiers
Learn more about the work of our research integrity team to safeguard the quality of each article we publish.