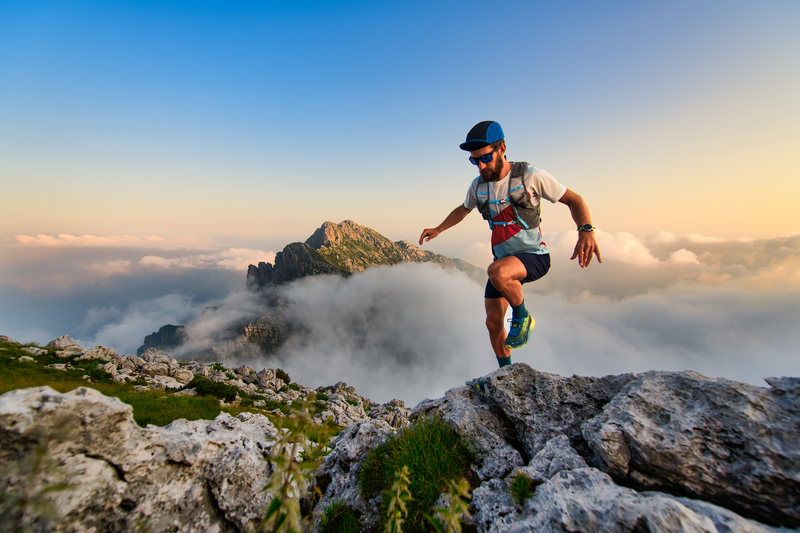
94% of researchers rate our articles as excellent or good
Learn more about the work of our research integrity team to safeguard the quality of each article we publish.
Find out more
ORIGINAL RESEARCH article
Front. Immunol.
Sec. Cancer Immunity and Immunotherapy
Volume 16 - 2025 | doi: 10.3389/fimmu.2025.1541252
The final, formatted version of the article will be published soon.
You have multiple emails registered with Frontiers:
Please enter your email address:
If you already have an account, please login
You don't have a Frontiers account ? You can register here
Background: Hepatocellular carcinoma (HCC) is the leading cause of tumor-related mortality worldwide. There is an urgent need for predictive biomarkers to guide treatment decisions. This study aimed to identify robust prognostic genes for HCC and to establish a theoretical foundation for clinical interventions. Methods: The HCC datasets were obtained from public databases and then differential expression analysis were used to obtain significant gene expression profiles. Subsequently, univariate Cox regression analysis and PH assumption test were performed, and a risk model was developed using an optimal algorithm from 101 combinations on the TCGA-LIHC dataset to pinpoint prognostic genes. Immune infiltration and drug sensitivity analyses were conducted to assess the impact of these genes and to explore potential chemotherapeutic agents for HCC. Additionally, single-cell analysis was employed to identify key cellular players and their interactions within the tumor microenvironment. Finally, reverse transcription-quantitative polymerase chain reaction (RT-qPCR) was utilized to validate the roles of these prognostic genes in HCC. Results: A total of eight prognostic genes were identified (MCM10, CEP55, KIF18A, ORC6, KIF23, CDC45, CDT1, and PLK4). The risk model, constructed based on these genes, was effective in predicting survival outcomes for HCC patients. CEP55 exhibited the strongest positive correlation with activated CD4 T cells. The top 10 drugs showed increased sensitivity in the low-risk group. B cells were identified as key cellular components with the highest interaction numbers and strengths with macrophages in both HCC and control groups. Prognostic genes were more highly expressed in the initial state of B cell differentiation. RT-qPCR confirmed significant upregulation of MCM10, KIF18A, CDC45, and PLK4 in HCC tissues (p < 0.05). Conclusion: This study successfully identified eight prognostic genes (MCM10, CEP55, KIF18A, ORC6, KIF23, CDC45, CDT1, and PLK4), which provided new directions for exploring the potential pathogenesis and clinical treatment research of HCC.
Keywords: Hepatocellular Carcinoma, single-cell sequencing analysis, Combination algorithms, Prognostic genes, drug sensitivity
Received: 07 Dec 2024; Accepted: 03 Mar 2025.
Copyright: © 2025 Wang, Deng, Jiang, Xu, Zhang, Zhou, Gong, Lu, Hou and Zhang. This is an open-access article distributed under the terms of the Creative Commons Attribution License (CC BY). The use, distribution or reproduction in other forums is permitted, provided the original author(s) or licensor are credited and that the original publication in this journal is cited, in accordance with accepted academic practice. No use, distribution or reproduction is permitted which does not comply with these terms.
* Correspondence:
Yi-Fu Hou, Department of Organ Translation Center, Sichuan Provincial People's Hospital, Chengdu, China
Hao Zhang, Department of Hepatobiliary Surgery, Sichuan Provincial People's Hospital, Sichuan, China
Disclaimer: All claims expressed in this article are solely those of the authors and do not necessarily represent those of their affiliated organizations, or those of the publisher, the editors and the reviewers. Any product that may be evaluated in this article or claim that may be made by its manufacturer is not guaranteed or endorsed by the publisher.
Research integrity at Frontiers
Learn more about the work of our research integrity team to safeguard the quality of each article we publish.