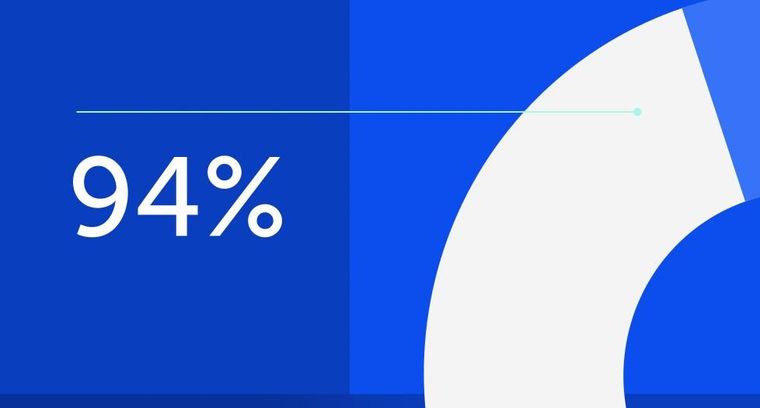
94% of researchers rate our articles as excellent or good
Learn more about the work of our research integrity team to safeguard the quality of each article we publish.
Find out more
ORIGINAL RESEARCH article
Front. Immunol.
Sec. Cancer Immunity and Immunotherapy
Volume 16 - 2025 | doi: 10.3389/fimmu.2025.1540018
This article is part of the Research TopicProtein Lactylation in Disease Progression: Biological Function and Therapeutic TargetsView all 4 articles
The final, formatted version of the article will be published soon.
Select one of your emails
You have multiple emails registered with Frontiers:
Notify me on publication
Please enter your email address:
If you already have an account, please login
You don't have a Frontiers account ? You can register here
Background: Breast cancer is a heterogeneous malignancy with complex molecular characteristics, making accurate prognostication and treatment stratification particularly challenging. Emerging evidence suggests that lactylation, a novel post-translational modification, plays a crucial role in tumor progression and immune modulation. Methods: To address breast cancer heterogeneity, we developed a machine learning-derived lactylation signature (MLLS) using lactylation-related genes selected through random survival forest (RSF) and univariate Cox regression analyses. A total of 108 algorithmic combinations were applied across multiple datasets to construct and validate the model. Immune microenvironment characteristics were analyzed using multiple immune infiltration algorithms. Computational drugrepurposing analyses were conducted to identify potential therapeutic agents for high-risk patients. Results: The MLLS effectively stratified patients into low-and high-risk groups with significantly different prognoses. The model demonstrated robust predictive power across multiple cohorts. Immune infiltration analysis revealed that the low-risk group exhibited higher levels of immune checkpoints (e.g., PD-1, PD-L1) and greater infiltration of B cells, CD4⁺ T cells, and CD8⁺ T cells, suggesting better responsiveness to immunotherapy. In contrast, the high-risk group showed immune suppression features associated with poor prognosis. Methotrexate was computationally predicted as a potential therapeutic candidate for high-risk patients, although experimental validation remains necessary.The MLLS represents a promising prognostic biomarker and may support personalized treatment strategies in breast cancer, particularly for identifying candidates who may benefit from immunotherapy.
Keywords: lactylation, Breast cancer prognosis, machine learning, immune microenvironment, Immunotherapy
Received: 05 Dec 2024; Accepted: 15 Apr 2025.
Copyright: © 2025 Min, Zhang, Liu, Wang, Guan, Chen, Sun, Wang and Wang. This is an open-access article distributed under the terms of the Creative Commons Attribution License (CC BY). The use, distribution or reproduction in other forums is permitted, provided the original author(s) or licensor are credited and that the original publication in this journal is cited, in accordance with accepted academic practice. No use, distribution or reproduction is permitted which does not comply with these terms.
* Correspondence: Tao Wang, Guizhou Provincial People's Hospital, Guiyang, China
Disclaimer: All claims expressed in this article are solely those of the authors and do not necessarily represent those of their affiliated organizations, or those of the publisher, the editors and the reviewers. Any product that may be evaluated in this article or claim that may be made by its manufacturer is not guaranteed or endorsed by the publisher.
Supplementary Material
Research integrity at Frontiers
Learn more about the work of our research integrity team to safeguard the quality of each article we publish.