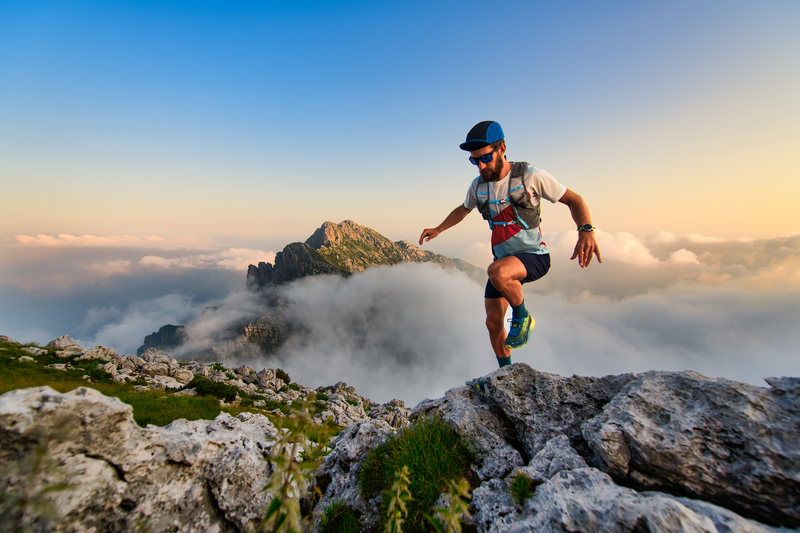
94% of researchers rate our articles as excellent or good
Learn more about the work of our research integrity team to safeguard the quality of each article we publish.
Find out more
ORIGINAL RESEARCH article
Front. Immunol.
Sec. Systems Immunology
Volume 16 - 2025 | doi: 10.3389/fimmu.2025.1533959
The final, formatted version of the article will be published soon.
You have multiple emails registered with Frontiers:
Please enter your email address:
If you already have an account, please login
You don't have a Frontiers account ? You can register here
Recent studies have suggested a potential association between gastric cancer (GC) and myocardial infarction (MI), with shared pathogenic factors. This study aimed to identify these common factors and potential pharmacologic targets.Data from the IEU Open GWAS project were used. Two-sample Mendelian randomization (MR) analysis was used to explore the causal link between MI and GC.Transcriptome analysis identified common differentially expressed genes, followed by enrichment analysis. Drug target MR analysis and eQTLs validated these associations with GC, and the Steiger direction test confirmed their direction. The random forest and Lasso algorithms were used to identify genes with diagnostic value, leading to nomogram construction. The performance of the model was evaluated via ROC, calibration, and decision curves. Correlations between diagnostic genes and immune cell infiltration were analyzed.MI was linked to increased GC risk (OR=1.112, P=0.04). Seventy-four genes, which are related mainly to ubiquitin-dependent proteasome pathways, were commonly differentially expressed between MI and GC. Nine genes were consistently associated with GC, and eight had diagnostic value. The nomogram built on these eight genes had strong predictive performance (AUC=0.950, validation set AUC=0.957). Immune cell infiltration analysis revealed significant correlations between several genes and immune cells, such as T cells, macrophages, neutrophils, B cells, and dendritic cells.MI is associated with an increased risk of developing GC, and both share common pathogenic factors. The nomogram constructed based on 8 genes with diagnostic value had good predictive performance.
Keywords: gastric cancer, Myocardial Infarction, Mendelian randomization, machine learning, Transcriptomics
Received: 25 Nov 2024; Accepted: 28 Feb 2025.
Copyright: © 2025 Ma, Hou, Gu, Guo and Zhu. This is an open-access article distributed under the terms of the Creative Commons Attribution License (CC BY). The use, distribution or reproduction in other forums is permitted, provided the original author(s) or licensor are credited and that the original publication in this journal is cited, in accordance with accepted academic practice. No use, distribution or reproduction is permitted which does not comply with these terms.
* Correspondence:
Junyang Ma, Jining Medical University, Jining, China
Jiankang Zhu, Laboratory of Metabolism and Gastrointestinal Tumor, The First Affiliated Hospital of Shandong First Medical University, Jinan, China, Jinan, China
Disclaimer: All claims expressed in this article are solely those of the authors and do not necessarily represent those of their affiliated organizations, or those of the publisher, the editors and the reviewers. Any product that may be evaluated in this article or claim that may be made by its manufacturer is not guaranteed or endorsed by the publisher.
Research integrity at Frontiers
Learn more about the work of our research integrity team to safeguard the quality of each article we publish.